Open sourcing the AI ecosystem ft. Arthur Mensch of Mistral AI and Matt Miller
发布时间 2024-03-26 21:18:16 来源
摘要
Arthur Mensch, founder of Mistral AI, speaks with Matt Miller at Sequoia Capital's AI Ascent about his mission to bring AI to all ...
GPT-4正在为你翻译摘要中......
中英文字稿 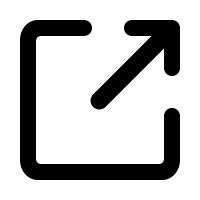
I'm excited to introduce our first speaker, Arthur from Mistral. Arthur is the founder and CEO of Mistral AI despite just being nine months old as a company and having many fewer resources than some of the large foundation model companies so far. I think they've really shocked everybody by putting out incredibly high quality models approaching GPT4 and caliber out into the open. So we're thrilled to have Arthur with us today all the way from France to share more about the opportunity behind building an open source. And please, in interviewing Arthur will be my partner Matt Miller who is dressed in his best French wear to honor Arthur today and helps lead our efforts in Europe. So please welcome Matt and Arthur. With all the efficiency of a French train, right? Right on time. Right on time. We were sweating a little bit back there. Just walked on the door. But good to see you. Thanks for coming all this way. Thanks for being with us here at AI Sense today. Thank you for hosting us. Absolutely. Would love to maybe start with the background story of why you chose to start Mistral and maybe just take us to the beginning. We all know about your career, your successful career at DeepMind, your work on the Chinchilla paper. Tell us maybe share with us. We always love to hear at Sequoia and I know that our founder can also love to hear that spark that gave you the idea to launch and to start to break out and start your own company.
我很高兴介绍我们的第一位演讲者Arthur来自Mistral。尽管作为一家只有九个月历史、资源比一些大型基本模型公司少得多的公司的创始人兼首席执行官,Arthur是Mistral AI的创始人和首席执行官。我认为,他们通过推出接近GPT4和同等水平的高质量模型真的让所有人都感到震惊。因此,我们非常高兴今天能有来自法国的Arthur与我们分享建立开源的机会。请欢迎Matt和Arthur进行访谈。像法国火车一样高效,准时到达。非常准时。我们在那边有点紧张。刚走进门。但很高兴见到你。感谢你这么远跋山涉水来到这里。感谢你今天在AI Sense与我们共度时光。谢谢你们的招待。当然。也许可以从你选择创办Mistral的背景故事开始,然后带我们回到开始。我们都知道你在DeepMind的成功职业生涯,以及你在Chinchilla论文上的工作。也许可以告诉我们一下。我们在Sequoia总是乐意倾听,我知道我们的创始人也乐意听到,是什么点燃了你创立公司的想法,并开始突围,创办自己的公司。
Yeah, sure. So we started the company in April, but I guess the idea was out there for a couple of months before. Simu, Tien and I were in Master Together, Guillem and I were in school together. So we knew each other from before and we had been in the field for like 10 years doing research. And so we loved the way AI progressed because of the open exchanges that occurred between academic labs, industrial labs and how everybody was able to build on top of one another.
是的,当然。我们在四月份成立了公司,但我想这个想法在几个月前就出现了。西姆、天恩和我一起念硕士,吉列姆和我一起上学。所以我们彼此早有认识,并且在同一领域做研究已经有10年了。我们喜欢人工智能的发展方式,因为学术实验室和工业实验室之间开放的交流,每个人都能借助其他人不断进步。
And it was still the case, I guess, even in the beginning of the LLM era, we were opening the AI and DeepMind were actually contributing to one another roadmap. And this kind of stopped in 2022. So basically one of the last paper doing important changes to the way we trained models was Chinchilla. And that was the last model that Google ever published, last important model in the field that Google published. And so for us, it was a bit of a shame that the field stopped doing open contributions that early in the AI journey because we're very far away from finishing it.
我想这种情况仍然存在,即使在LLM时代初期,我们正在开启AI,DeepMind实际上正在为彼此贡献路线图。这种情况在2022年停止了。因此,基本上最后一个对我们训练模型方式进行重要改变的论文是Chinchilla。这是谷歌发布的最后一个模型,在谷歌发布的领域中是最重要的模型。对我们来说,遗憾的是,该领域在AI之旅早期就停止了开放贡献,因为我们离完成它还有很长的路要走。
And so when we saw Chinchilla at the end of the year, and I think we reflected on the fact that there was some opportunity for doing things differently, for doing things from friends because in France you have, as it turned out, there was a lot of talented people that were a bit bored in big tech companies. And so that's how we figured out that there was an opportunity for building very strong open source models going very fast with a lean team of experienced people and try to correct the direction that the field was taking.
因此,当我们在年底看到了柳鼠(Chinchilla)时,我认为我们反思了一件事实,那就是有机会以不同的方式做事,以朋友们之所长来做事,因为在法国,我们发现有很多才华横溢的人在大型科技公司稍感无聊。因此,我们想到可以建立一个由经验丰富的精干团队组成、快速发展的非常强大的开源模式,尝试纠正领域发展的方向。
So we wanted to push the open source model much more. I think we did a good job at that because we've been followed by various companies in our trajectory. Wonderful. And so it was really a lot of the open source movement was a lot of the drive behind starting the company. Yeah, that's one of the driver, our intention and the mission that we gave ourselves is really to bring AI to the hands of every developer.
因此,我们希望更多地推动开源模式。我认为我们在这方面做得很好,因为我们在发展过程中得到了各种公司的追随。很棒。因此,开源运动在很大程度上推动了公司的创立。是的,这是我们的一个动力之一,我们的意图和使命是将人工智能带入每一位开发者的手中。
And the way it was done and the way it is still done by our competitors is very close. And so we want to push a much more open platform and we want to spread the adoption and accelerate the adoption through that strategy. So that's very much at the core. The reason why we started the company did wonderful.
我们的竞争对手做事的方式,以及他们现在的做事方式都很相似。因此,我们希望推动一个更加开放的平台,并通过这种策略来推动采用和加速推广。这在我们公司的核心地位,也是我们创立公司的初衷。
And just recently, I mean, fast forward to today, you released Mistral Large. You've been on this tear of like amazing partnerships with Microsoft, Snowflake, Databricks, announcements. So how do you balance the what you're going to do open source with what you're going to do commercially and how you're going to think about the trade off?
最近,也就是说,快速来到今天,你发布了Mistral Large。你与微软、Snowflake、Databricks等合作伙伴持续推出了一系列惊人的合作消息。那么你是如何平衡你要开源的项目和商业项目以及如何权衡二者之间的取舍呢?
Because that's something that many open source companies contend with. How do they keep their community thriving? But then how do they also build a successful business to contribute to their community? Yeah, it's a hard question. And the way we've addressed it is currently through two families of model, but this might evolve with time. We intend to stay the leader in open source. So that kind of puts a pressure on the open source family because there's obviously some contenders out there.
因为这是许多开源公司所面临的问题。他们如何保持社区的蓬勃发展?但是他们又如何建立一个成功的业务来为社区做出贡献呢?是的,这是一个难题。我们目前通过两种模式来解决这个问题,但随着时间的推移,这种模式可能会发生变化。我们打算继续保持在开源领域的领导地位。这给开源社区带来了一定的压力,因为显然还有一些竞争者。
I think compared to how various software providers playing this strategy developed, we need to go faster because AI develops faster than software. It develops faster than Databricks. Like MongoDB played a very good game at that. And this is a good example of what we could do, but we need to adapt faster. So yeah, there's obviously this tension and we're constantly thinking on how we should contribute to the community, but also how we should show and start getting some commercial adoption, enterprise deals, etc.
我认为与各种软件提供商玩这种策略发展相比,我们需要走得更快,因为人工智能的发展速度比软件更快。它比Databricks发展得更快。就像MongoDB在那方面打得很好。这是我们可以做的一个很好的例子,但我们需要更快地适应。所以是的,显然存在这种紧张感,我们一直在思考我们应该如何为社区做贡献,但同时也在思考我们应该如何展示并开始获取一些商业应用、企业交易等。
And this is obviously a tension. And for now, I think we've done a good job at doing it, but it's a very dynamic thing to think through. So it's basically every week we think of what we should release next on both families. And you have been the fastest in developing models, fastest reaching different benchmarking levels, one of the most leanest in amount of expenditure to reach these benchmarks out of any of the foundational model companies.
这显然是一个紧张的状况。目前为止,我认为我们在处理这个问题上做得不错,但这是一个需要不断思考的动态过程。基本上,每周我们都在考虑在两个系列上接下来该发布什么。你在开发模型方面是最快的,最快达到了不同的基准水平,是所有基础模型公司中在达到这些基准水平所需支出最少的之一。
What do you think has given you that advantage to move quicker than your predecessors and more efficiently? I think we like to get our hands dirty. It's machine learning has always been about crunching numbers, looking at your data, doing a lot of extract, transform and load and things that are oftentimes not fascinating. And so we hired people that were willing to do that stuff. And I think that has been critical to our speed and that's something that we want to keep.
你认为是什么让你比前人更快更有效地前进?我认为我们喜欢动手实践。机器学习一直是关于处理数据,分析数据,进行大量的提取、转换和加载等操作,这些往往并不吸引人。因此,我们雇用愿意做这些工作的人。我认为这对于我们的速度至关重要,这也是我们希望保持的东西。
Awesome. And in addition to the large model, you also have several small models that are extremely popular. When would you tell people that they should spend their time working with you on the small models? When would you tell them working on the large models? Where do you think the economic opportunity for Mistral lies? Is it doing more at the big or doing more of the small?
太棒了。除了大型模型外,您还有几个非常受欢迎的小型模型。您何时会告诉人们他们应该花时间与您一起研究小模型?何时会告诉他们应该致力于大模型?您认为Mistral的经济机会在哪里?是在扩大大型模型的业务还是专注于更多的小模型?
I think this is an observation that every LLM provider has made that one size does not fit all. Depending on what you want to. When you make an application, you typically have different large language model calls. And some should be low latency and because they don't require a lot of intelligence, but some should be higher latency and require more intelligence. An efficient application should leverage both of them, potentially using the large models as an orchestrator for the small ones.
我认为这是每个LLM提供商都会注意到的观察结果,即一刀切并不适用于所有情况。取决于你想要什么。当你提交申请时,通常会有不同的大语言模型调用。有些应该是低延迟的,因为它们不需要太多智能,但有些则应该是高延迟的,需要更多智能。一个高效的应用程序应该充分利用两者,可能使用大模型作为小模型的编排程序。
And I think the challenge here is how do you make sure that everything works? So you end up with a system that is not only a model, but it's really like two models plus an author loop of calling your model, calling systems, calling functions. And I think some of the developer challenge that we also want to address is how do you make sure that this works, that you can evaluate it properly, how do you make sure that you can do continuous integration? Or do you change like. Or do you move from one version to another of a model and make sure that your application has actually improved and not deteriorated?
我认为这里的挑战是如何确保一切都能正常运作?因此,你最终会得到一个不仅是一个模型,而且实际上是两个模型加一个作者循环调用你的模型、调用系统、调用函数。我认为我们也想要解决一些开发者挑战,即如何确保这一切都能正常运作,你可以正确评估它,如何确保你可以进行持续集成?或者你改变或者从一个版本转移到另一个模型,并确保你的应用实际上有所改善而非恶化。
So all of these things are addressed by various companies, but these are also things that we think should be core to our value proposition. And what are some of the most exciting things you see being built on Mistral? Like what are the things that you get really excited about that you see the community doing or customers doing? I think pretty much every young startup in the Bay Area has been using it for like fine-tuning purposes for fast application making. So really, I think one part of the value of Mistral, for instance, is that it's very fast.
因此,各家公司都致力于解决这些问题,但我们认为这些问题也应是我们核心的价值主张。你认为在Mistral上构建的最令人兴奋的事情是什么?比如说,你最为激动的见到社区或客户在做的事情是什么?我认为旧金山湾区的几乎每家年轻初创公司都在使用它进行快速应用程序制作的微调。因此,我认为Mistral的价值之一就是它非常快速。
And so you can make applications that are more involved. And so we've seen web search companies using us. We've seen all of the standard enterprise stuff as well, like knowledge management, marketing. The fact that you have access to the weights means that you can pour in your editorial tone much more. So that's, yeah, we see the typical use cases. I think the value is that for the open source part is that developers have control so they can deploy it everywhere.
因此,您可以制作更复杂的应用程序。我们已经看到网络搜索公司在使用我们。我们也看到了所有标准的企业应用,如知识管理、营销等。您可以访问权重这一事实意味着您可以更多地灌注自己的编辑风格。所以,是的,我们看到了典型的使用案例。我认为开源部分的价值在于开发者有控制权,因此他们可以在任何地方部署它。
They can have very high quality of service because they can use their dedicated instances, for instance. And they can modify the weights to suit their needs and to bump the performance to a level which is close to the largest models while being much cheaper. And what's the next big thing that we're going to get to see from you guys? Can you give us a sneak peek of what might be coming soon or how we should be expecting from Mistral? Yeah, for sure.
他们可以提供非常高质量的服务,因为他们可以使用专用实例。并且他们可以修改权重以适应自己的需求,从而提高性能,使其接近最大模型的水平,同时价格更便宜。接下来我们从你们这里可以看到什么大事件?能透露一下即将到来的消息或者我们应该对Mistral有什么期待吗?当然。
So we have, so Mr. Laj was good but not good enough so we are working on improving it quite heavily. We have interesting open source models on various vertical domains that we'll be announcing very soon. The platform is currently just APIs, like your serverless APIs. And so we are working on making customization part of it, so we like the fine tuning part. And obviously, and I think as many other companies, we are heavily betting on multi-lingual data and multi-lingual model because as a European company, we're also well positioned.
所以我们现在正在努力改进,因为Laj先生很好,但还不够好。我们在各个垂直领域有一些有趣的开源模型,很快会公布。这个平台目前只是API,就像您的无服务器API一样。所以我们正在努力让定制成为其中的一部分,我们喜欢微调的过程。显然,和许多其他公司一样,我们正在大力押注多语言数据和多语言模型,因为作为一个欧洲公司,我们处于一个很好的位置。
And this is the demand of our customers that I think is higher than here. And then, yeah, eventually in the months to come, we will also release some multi-lingual models. Okay, exciting. We'll look forward to that. As you mentioned, many of the people in this room are using Mistral models. Many of the companies we work with here every day here in the Silicon Valley ecosystem are already working with Mistral. How should they work with you and how should they work with the company and what type of, what's the best way for them to work with you? Well, they can reach out.
这是我们客户的需求,我认为比这里更高。然后,在未来几个月,我们也会发布一些多语言模型。好的,很令人兴奋,我们期待着这一点。正如你所提到的,这个房间里的许多人正在使用Mistral模型。我们在硅谷生态系统每天与许多公司合作,他们已经在使用Mistral。他们应该如何与你合作,如何与公司合作,最好的方式是什么?嗯,他们可以联系我们。
So we have some developer relations that are really pushing the community forward, making guides, also gathering use cases to showcase where they can build with Mistral models. So this is, we're very like investing a lot on the community. Something that basically makes the model better and that we are trying to set up is our ways for us to get evaluations, benchmarks, actual use cases on which we can evaluate our models on.
因此,我们有一些开发者关系团队正在推动社区前进,制作指南,收集使用案例来展示他们可以如何使用Mistral模型进行构建。因此,我们在很大程度上正在投资于社区。我们正在努力打造一些方法,使模型变得更好,我们正在尝试建立的是为我们获取评估、基准测试、实际使用案例的方式,以便我们对模型进行评估。
And so having like a mapping of what people are building with our model is also a way for us to make a better generation of new open source models. And so please engage with us to discuss how we can help, how, discuss your use cases. We can advertise it. We can also gather some insight of the new evaluations that we should add to our evaluation to verify that our models are getting better all the time.
因此,了解人们如何使用我们的模型进行建模也是我们制定新一代开源模型的一种方式。因此,请与我们沟通讨论,讨论我们如何能够帮助您解决问题,讨论您的具体应用场景。我们可以进行宣传,也可以收集一些见解,以确定我们应该添加到评估中的新评估指标,以验证我们的模型始终在不断改进。
And on the commercial side, our models are available on our platform. So the commercial models are actually working better than the open source ones. They're also available on various cloud providers so that it facilitates adoption for enterprises. And customization capabilities like fine tuning, which really made the value of the open source models are actually coming very soon. Wonderful. And you talked a little bit about the benefits of being in Europe. You touched on it briefly. There are already this example, global example of the great innovations that can come from Europe and are coming from Europe. But talk a little bit more about the advantages of building a business from France and like building this company from Europe. The advantage in drawbacks, I guess. Yeah, both. I guess one advantage is that you have a very strong junior pool of talent. So there's a lot of people coming from masters in France, in Poland, in the UK that we can train in three months and get them up to speed, get them basically producing as much as a million dollar engineer in the Bay Area for 10 times. Sometimes there's the cost, so that's kind of efficient. Don't tell them all that. They're going to hire people in France. The workforces are very good. Engineers and machine learning engineers. Generally speaking, we have a lot of support from the state, which is actually more important in Europe than in the US. They tend to over-regulate a bit too fast. We've been telling them not to, but they don't always listen. And then generally, European companies like to work with us because we're European and we are better in European languages, as it turns out. The French mistrialage is actually probably the strongest French model out there. So that's not an advantage, but at least there's a lot of opportunities that are going to be a little bit more difficult and it's really emerging.
在商业方面,我们的模型可以在我们的平台上使用。所以商业模型实际上比开源模型运行更好。它们也可以在各种云服务提供商上使用,这样有利于企业采用。并且像微调这样的定制能力,真正增加了开源模型的价值,实际上很快就会到来。太棒了。您稍微谈到了在欧洲的好处。您简要地提到了一下。已经有这个例子,全球范围内可以从欧洲出现并正在出现的伟大创新。但谈一下从法国建立业务和从欧洲建立这家公司的优势。优点和缺点,我想。是的,两者都有。我想一个优点是你有一个非常强大的初级人才池。所以有很多来自法国、波兰、英国的硕士毕业生,我们可以在三个月内进行培训,并快速提升他们的速度,让他们基本上达到在湾区的一名百万美元工程师的生产力十倍。有时候是成本,所以这种效率很高。别告诉他们所有人都会在法国雇用人。在法国,工作力量非常出色。工程师和机器学习工程师。总的来说,我们得到了很多国家的支持,这在欧洲比在美国更重要。他们倾向于过快过度规范。我们一直告诉他们不要这样做,但他们并不总是听取建议。总的来说,欧洲公司喜欢与我们合作,因为我们是欧洲人,我们在欧洲语言方面更擅长,事实证明是如此。法国人的技术其实可能是最强大的法国模型之一。所以这不是一个优势,但至少有很多机会比较困难,而且真的正在兴起。
Wonderful. And paint the picture for us five years from now. I know that this world is moving so fast and you just think about all the things you've gone through in the two years. It's not even two years old as a company. Almost two years old as a company. But five years from now, where does mistrialage said, what do you think you have achieved? What does this landscape look like? So our bet is that basically the platform and the infrastructure of artificial intelligence will be open. And based on that, we'll be able to create assistance and then potentially autonomous agent. And we believe that we can become this platform by being the most open platform out there, by being independent from cloud providers, etc. So in five years from now, I have literally no idea of what this is going to look like. If you looked at the field in like 2019, I don't think you could bet on where we would be today. But we are evolving toward more and more autonomous agents. We can do more and more tasks. I think the way we work is going to be changed profoundly. And making such agents and assistants is going to be easier and easier. So right now we're focusing on the developer world. But I expect that AI technology is in itself so easily controllable through human languages, human language that potentially at some point the developer becomes a user. And so we are evolving toward any user being able to create its own assistant or its own autonomous agent. I'm pretty sure that in five years from now, this will be something that you learn to do at school.
太棒了。那么请为我们勾勒一下五年后的景象。我知道这个世界发展如此之快,你只需想一想在这两年里经历的所有事情。作为一家公司,还不到两岁。几乎是刚刚两岁。但是五年后,mistrialage说,你认为你已经实现了什么?这个领域会是什么样子?所以我们的打赌基本上是人工智能的平台和基础设施会是公开的。基于此,我们将能够创建助手,潜在地是自主代理。我们相信通过成为最开放的平台,独立于云服务提供商等,我们可以成为这个平台。所以说,五年后,我真的完全不知道这会是什么样子。如果你看看2019年的领域,我认为你不会猜到我们今天会在哪里。但我们正在向着越来越多的自主代理发展。我们可以完成越来越多的任务。我认为我们的工作方式会发生深刻变化。制作这样的代理和助手会变得越来越简单。所以现在我们专注于开发者领域。但我期望人工智能技术本身是如此容易通过人类语言来控制,潜在地,在某个时候开发者会成为用户。因此我们正在朝着任何用户能够创建自己的助手或自主代理的方向发展。我非常确信,五年后,这将成为学校里的一项必学技能。
Awesome. Well, we have about five minutes left. Just want to open up in case there's any questions from the audience. Don't be shy. Sony's got a question. How do you see the future of open source versus commercial models playing out for your company? I think you made a huge splash with open source at first. As you mentioned, some of the commercial models are even better now. How do you imagine that plays out over the next handful of years? Well, I guess the one thing we optimize for is to be able to continuously produce open models with a sustainable business model to actually fuel the development of the next generation. And so that's, I think that's, as I've said, this is going to evolve with time. But in order to stay relevant, we need to stay the best at producing open source models, at least on some part of the spectrum. So that can be the small models. That can be the very big models.
太棒了。我们还有大约五分钟的时间。只是想开放一下,看看是否有观众有任何问题。不要害羞。索尼有一个问题。您如何看待公司未来的开源与商业模式之间的发展?我认为您最初推出的开源模式引起了巨大轰动。正如您所提到的,一些商业模式现在甚至更好。您如何想象未来几年内这种情况会发生?嗯,我想我们优化的一个方面是能够持续生产具有可持续商业模式的开放模式,以实际推动下一代的发展。所以,我认为,正如我所说的,这将随着时间的推移而发展。但为了保持相关性,我们需要在某个方面始终保持制作开源模型的最佳水平。这可以是小型模型。也可以是非常大型的模型。
And so that's very much something that basically that sets a constraint on whatever we can do. Staying relevant in the open source world, staying the best solution for developers is really our mission and we'll keep doing it. David. There's got to be questions from more than just the Sequoia partners, guys. You talked a little bit about Loma3 and Facebook and how you think about competition with them? Well, Loma3 is working on, I guess, making models. I'm not sure that we will be open source. I have no idea of what's going on there. So far, I think we've been delivering faster and smaller models. So we expect to be continuing doing it.
因此,这实际上设定了我们所能做的一些约束。保持在开源世界中保持相关性,对开发人员来说是最佳解决方案,这确实是我们的使命,我们将继续努力。大卫。不仅有 Sequoia 合作伙伴提问。你谈到了 Loma3 和 Facebook,你怎么看待与他们的竞争?嗯,Loma3 正在进行,我想,制作模型。我不确定我们会是开源的。我不知道他们在做什么。到目前为止,我认为我们提供更快、更小的模型。因此,我们希望继续这样做。
But generally, the good thing about open source is that it's never too much for competition because once you have several actors, normally that should actually benefit to everybody. And so there should be some, if they turn out to be very strong, there will be some cosperination and we will welcome it. One thing that's made you guys different from other proprietary model providers is the partnerships with Snowflakes and Databricks, for example, and running natively in their clouds as opposed to just having API connectivity.
总的来说,开源软件的好处在于它永远不会因为竞争而过度发展,因为一旦有多个参与者,通常这实际上应该对每个人都有利。因此,如果它们表现出色,将会有一些合作,我们会欣然接受。你们与其他专有模型提供商不同的一点是与Snowflakes和Databricks等合作伙伴关系,并在其云中本地运行,而不仅仅是具有API连接。
Curious if you can talk about why you did those deals and then also what you see is the future of say Databricks or Snowflake in the Brave New Loma world. I guess you should ask them. But I think, generally speaking, AI models become very strong. They are connected to data and grounding information. As it turns out, the enterprise that I oftentimes either on Snowflake or on Databricks or sometimes on AWS. And so being able for customers to be able to deploy the technology exactly where their data is is, I think, quite important.
想知道你能否谈谈为什么做了那些交易,以及你认为Databricks或Snowflake在这个新充满可能的世界中的未来会怎样。我猜你应该问问他们。但我认为,一般来说,人工智能模型变得非常强大。它们与数据和基础信息相连。事实证明,企业往往要么使用Snowflake,要么使用Databricks,有时使用AWS。因此,顾客能够将技术部署在他们的数据所在的地方,我认为这是非常重要的。
I expect that this will continue doing the case, being the case, especially as I believe we'll move on to more stateful AI deployment. So today we deploy several SAPIs with not much state. It's really like lambda functions. But as we go forward and as we make models more and more specialized, as we make them more tuned to use cases and as we make them self-improving, you will have to manage state and those could actually be part of the data cloud. So there's an open question of where do you put the AI state? And I think that's my understanding is that Snowflake and Databricks would like it to be on the other data cloud.
我预计这种情况会继续下去,尤其是因为我相信我们将继续推进更多有状态的人工智能部署。所以今天我们部署了几个没有太多状态的SAPIs。这真的很像lambda函数。但随着我们不断向前推进,随着我们将模型变得越来越专业化,将它们调整为更适合用例,并让它们自我改进,您将不得不管理状态,这些状态实际上可能是数据云的一部分。所以现在有一个未解之谜,AI状态应该存放在哪里?我认为我的理解是Snowflake和Databricks希望它存在于另一个数据云中。
I think there's a question right behind him, the gray switch. I'm curious where you draw the line between openness and proprietary. So you're releasing the weights. Would you also be comfortable sharing more about how you train the models, the recipe for how you collect the data, how you do mixture of experts training, or do you draw the line at like we release the weights and the rest is proprietary? So that's where we draw the line. And I think the reason for that is that it's a very competitive landscape. And so it's similar to the tensions there is in between having some form of revenue to stay in the next generation.
我认为在他身后右边,那个灰色的开关后面有一个问题。我很好奇你在开放性和专有性之间如何划定界限。所以你释放了权重。你是否也愿意分享更多关于如何训练模型、如何收集数据的配方、如何进行专家混合训练,或者你对像我们释放权重这样的界限感到满意?这就是我们的界限所在。我认为这样做的原因是竞争格局非常激烈。就像在保持下一代的一种形式收入之间存在的紧张关系一样。
And there's also a tension between what you actually disclose and everything that, yeah, in order to stay ahead of the curve and not to give your recipe to your competitors. And so again, this is the moving line. If there's also some game theory at stake, like if everybody starts doing it, then we could do it. But for now, for now we are not taking this reskinvity. I'm curious when another company releases weights for a model like Groc, for example, and you only see the weights, what kinds of practices do you guys do internally to see what you can learn from it? You can't tell on a lot of things from weights. We don't even look at it.
在你实际披露的东西和为了保持领先地位而不将你的配方透露给竞争对手之间存在着紧张关系。因此,这是一个不断变化的界限。如果涉及到一些博弈论,比如如果每个人都开始这样做,那么我们也可以这样做。但是目前我们并没有采取这种反应性。例如,当另一家公司发布了像Groc这样的模型的权重,你只看到了权重时,你们内部会进行怎样的实践来看看能从中学到什么?从权重中无法得知许多事情,我们甚至不看它。
It's actually too big for us to deploy a Groc is quite big. Or was there any architecture learning? I guess they are using a mixture of expert pretty standard sitting with a couple of treats that I knew about. Yeah, there's not a lot of things to learn from the recipe themselves by looking at the weights. You can try to infer things, but reverse engineering is not that easy. It's basically compression information and it compresses information sufficiently highly so that you can't really find out what's going on. The cube is coming. It's okay. Yeah, I'm just curious about what are you guys going to focus on the model sizes? The oping is in that. Is you guys going to still go on a small or going to go to the larger ones? So model size are kind of set by scaling loads. It depends on the compute you have based on the learning infrastructure you want to go to, you make some choices. And so you optimize for training cost and foreign friends cost. And then there's obviously there's the weight in between, like for depends on the weight that you put on the training cost and the more you amortize it, the more you can compress models. But basically our goal is to be low latency and to be relevant from the reasoning front. So that means having a family of model that goes from the small ones to the very large ones.
这实际上对我们来说太大了,部署一个Groc相当大。或者有没有架构学习?我想他们正在使用一种专家标准的混合物,带有一些我知道的技巧。是的,从食谱中看重量并不能学到很多东西。你可以尝试推断一些事情,但逆向工程并不那么容易。它基本上是压缩信息,并且将信息压缩得足够高,以至于你无法真正找出发生了什么。方块即将来临。没事。是的,我只是好奇你们将会专注于模型大小吗?操作在于此。你们是继续使用小型模型还是会使用更大的模型?模型大小基本上是由扩展负载来设置的。它取决于你拥有的计算资源,基于你想要使用的学习基础设施,你做出一些选择。因此,你要优化训练成本和推理成本。然后在这之间显然有权衡,也就是根据你对训练成本的权衡,并愈合的越多,你就能压缩模型。但基本上我们的目标是低延迟并且在推理方面是相关的。这意味着拥有一系列从小型模型到非常大型模型的模型家族。
Hi, are there any plans for Mistral to expand into the application stack? So for example, when I released the custom GPTs and the assistance API, is that the direction that you think that Mistral will take in the future? Yeah, so I think as I've said, we are really focusing on the developer first. But there's many, like the frontier is pretty thin in between developers and users for this technology. And so that's the reason why we released like an assistant, the multi-talk called LOSHA, which is the cat in English. And it's the point here is to expose it to enterprises as well and make them able to connect their data, connect their context. I think that answers some need from our customers that many of the people we've been talking to are willing to adopt the technology, but they need an entry point. And if you just give them APIs, they're going to say, okay, but I need an integrator. And then if you don't have an integrator at hand, and oftentimes this is the case, it's good if you have like an official solution, at least you get them into the technology and show them what they could build for their core business. So that's the reason why we now have like two products offering. And the first one, which is the platform, and then we have the Shah, which should evolve into an enterprise of the shelf solution. Or over there.
嗨,对于Mistral是否有扩展到应用程序堆栈的计划?例如,当我发布了定制的GPT和辅助API时,您认为Mistral未来的发展方向是这样的吗?是的,正如我所说,我们确实将开发人员放在首位。但是在这项技术中,开发人员和用户之间的界限非常薄弱。因此,这就是为什么我们发布了一个助手,一个名为LOSHA的多对话助手,它在英语中是一只猫。关键在于向企业暴露它,并使它们能够连接其数据、连接其上下文。我认为这满足了我们的客户的一些需求,我们与很多客户交流后发现,他们愿意采用这项技术,但他们需要一个入口点。如果你只给他们API,他们会说,好吧,但是我需要一个集成商。而且,如果你手头上没有集成商,这种情况经常发生,如果你有一个官方解决方案,至少你可以让他们进入技术,并展示他们可以为他们的核心业务构建什么。这就是我们现在有两个产品供应的原因。第一个是平台,第二个是Shah,它将发展成一个企业级现成解决方案。
Just wondering like where would you be drawing the line between like stop doing prompt engineering and start doing like point tuning? Because like a lot of my friends and our customers are suffering from like where they stop doing more prompt engineering. I think that's the number one pain point that is hard to solve from a product standpoint. The question is, normally your workflow should be what should you evaluate on? And based on that, have your model kind of find out a way of solving your task. And so right now this is still a bit manual. You go and you have like several versions of prompting. But this is something that actually AI can help solving. And I expect that this is going to grow more and more automatically across time. And this is something that yeah, we'd love to try and enable.
我在想,在停止进行即时工程和开始进行调优之间应该划定哪条界线呢?因为很多我的朋友和客户都在痛苦地停止进行更多的即时工程。我认为这是一个产品角度很难解决的头号痛点。问题是,通常你的工作流应该是评估什么?基于此,让你的模型找到解决任务的方法。目前这还是有点手动的。你去了解并尝试几个版本的提示。但这实际上是AI可以帮助解决的事情。我期望这将在未来自动增长。这是我们乐意尝试和支持的事情。
I wanted to ask a bit more of a personal question. So like as a founder in the cutting edge of AI, how do you balance your time between explore and exploit? Like how do you yourself stay on top of like a feel that's rapidly evolving and becoming larger and deeper every day? How do you still talk? So I think this question is, I mean, we explore on the science part and the product part and on the business part. And the way you balance it is effectively hard for a startup. You do have to exploit a lot because you need to shift fast. But on the science part, for instance, we have like two or three people that are like working on the next generation of models. And sometimes they lose time. But if you don't do that, you are at risk of becoming irrelevant. And this is very true for the product side as well. Right now we have a very simple product. But being able to try out new features and see how they pick up is something that we need to do. And on the business part, you never know who is actually quite mature enough to use your technology. So yeah, the balance between exploitation and exploration is something that we master well at the science level because we've been doing it for years. And somehow it transcribes into the product and the business. But I guess we are currently still learning to do it properly.
作为人工智能领域的创始人,你如何平衡探索和利用之间的时间?如何能够保持在不断演化和日益扩大、深化的领域中保持领先地位?我认为这个问题包含着我们在科学、产品和商业方面的探索。在创业初期,平衡这些方面是非常困难的。你必须进行大量的利用,因为你需要快速转变。但就科学方面来说,我们有两三个人在致力于下一代模型的研究,有时候他们会花费大量时间。但如果不这样做,你就有可能变得不重要。对于产品方面也是如此。目前我们的产品非常简单。但尝试新功能并观察市场反应是必须的。在商业方面,你永远不知道谁是真正成熟能够使用你技术的人。因此,在利用和探索之间的平衡是我们在科学层面上做得很好的,因为我们这么做已经几年了。这种平衡方式也逐渐转移到了产品和商业层面。但我们仍在学习如何更好地进行平衡。
So one more question for me and then I think we will be done without a time. But in the scope of two years, models big, models small that have taken the world by storm, killer go to market partnerships, just tremendous momentum at the center of the AI ecosystem. What advice would you give to founders here? What you have achieved and the pace of which you have achieved is truly extraordinary. What advice would you give to people here who are at different levels of starting and running and building their own businesses and around the AI opportunity?
所以再问我一个问题,然后我想我们会很快结束。但在过去的两年里,一些大型的,一些小型的模型风靡全球,一些杀手级的市场合作,AI生态系统的中心拥有巨大的势头。您会给创始人们什么建议?您所取得的成就和您取得的速度真的非常了不起。您会给这里的人们什么建议?无论他们处于不同的起点、经营或建立自己的企业,还是在AI领域的机遇周围。
I would say it's always day one. So I guess we got some mind share but there's still many proof points that we need to establish. And so being a founder is basically working up every day and figuring out that you need to build everything from scratch every time, all the time. So it's a bit exhausting but it's also exhilarating. And so I would recommend to be quite ambitious, usually being more ambitious. Ambition can get you very far. And so you should dream big. That would be my advice.
我会说,一直都是开始的阶段。我想我们已经占据了一些思想市场,但还有很多证据需要我们建立。所以作为创始人,基本上每天都在努力工作,发现每次都需要从零开始建立所有东西。这有点令人筋疲力尽,但也让人兴奋。所以我建议要相当雄心勃勃,通常要更有雄心。雄心可以让你走得很远。所以你应该有大梦想。这就是我的建议。
Awesome. Thank you Artur. Thanks for being with us today. Thank you. Thank you.
太棒了。谢谢你,阿图尔。感谢你今天和我们在一起。谢谢你。谢谢你。