Trust, reliability, and safety in AI ft. Daniela Amodei of Anthropic and Sonya Huang - YouTube
发布时间 2024-03-26 06:18:19 来源
中英文字稿 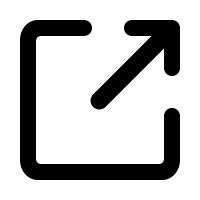
We are thrilled to have our next speaker with us. Daniela is the president and co-founder of Anthropic, which recently just launched the really impressive quad 3 model. Please welcome Daniela in conversation. Thank you so much for being here, Daniela. You're welcome. Yes, you do. Take this. Oh, that's so nice of you. Thank you. I think everybody in the audience is familiar with Anthropic as probably a customer of yours. But can you just do a quick refresher for everyone in the audience about Anthropic, the company, what is your mission, what's the future you imagine, and how are you building towards that future? Sure thing. So first of all, thanks so much for having me. Great to be with all of you today. So I'm Daniela. I am a co-founder and president at Anthropic. We are a generative AI company that is really working to build powerful transformative generative AI tools that really have humans at the center of them. So we have a huge focus on building this tech in a way that is trustworthy and reliable. And we've been around for just about three years, a little over three years. And in that time, have been able to advance the state of the art across generative AI on a number of dimensions. Wonderful.
我们很高兴可以和下一个演讲嘉宾一起。达妮拉是Anthropic的总裁兼联合创始人,该公司最近刚刚推出了令人印象深刻的Quad 3模型。请欢迎达妮拉加入我们的对话。非常感谢你能在这里,达妮拉。不客气。是的,请拿这个。哦,你太好了。谢谢。我想观众中的每个人都对Anthropic很熟悉,可能都是你们的客户。但你能为现场的每个人简要介绍一下Anthropic这家公司吗?我们的使命是什么,你所想象的未来是什么,以及你正在如何朝着未来发展?当然。首先非常感谢邀请我来这里。很高兴能与大家在一起。我是达妮拉,Anthropic的联合创始人和总裁。我们是一家生成式人工智能公司,真正努力构建强大的变革性生成式人工智能工具,以人类为中心。我们非常重视以可信赖和可靠的方式构建这项技术。我们成立大约三年多了,在这段时间里,我们已经在多个领域推动了生成式人工智能的最新技术。太棒了。
And what are the unique approaches that you're taking now that the foundation model space is getting very crowded? What are the things that make you uniquely anthropic? I love that question. So first of all, I would say there's a few different ways that I kind of like think about or interpret that question. One is really how do we kind of differentiate ourselves at the model level, right? What do we do when we're training the models or how do we want the models to sort of have people feel when they use them? And here what I would say is we really, again, thinking about this kind of commitment to trustworthiness, reliability of our models, we implement a number of different sort of technical safety approaches to help make the models really more aligned with what humans want them to be doing. So we pioneered a technique called constitutional AI, which really enables the models to incorporate documents like the UN Declaration of Human Rights, the Apple Terms of Service to really make it more aligned with values of the sort of human race. From a sort of business perspective, we really have tried to make a quad as approachable as possible in particular for enterprise businesses. So large businesses in particular, I think, have really resonated with our approach because they also value models that are helpful and honest and harmless, right? In general, very large enterprise businesses tend to be concerned about models that will hallucinate or say something very, very offensive.
在基础模型空间变得非常拥挤的现在,你们正在采取什么独特的方法?是什么让你们与众不同?我喜欢这个问题。首先,我想说有几种不同的方式来思考或解释这个问题。一种是我们如何在模型层面上区分自己,对吧?在我们训练模型或者当人们使用模型时,我们希望模型让人们有什么感觉?在这里,我想说的是,我们真的是再次考虑信任和可靠性这一承诺,我们实施了许多不同的技术安全方法,以帮助使模型更符合人类希望它们做的事情。因此,我们开创了一种叫做宪法AI的技术,它真的能让模型纳入诸如《联合国人权宣言》、苹果服务条款等文件,从而使其更符合人类价值观。从商业角度来看,我们真的尽可能地让四向贸易(quad as)方法对企业尤其是大企业尽可能容易接受。尤其是大企业,我认为他们对我们的方法产生了共鸣,因为他们也看重有用、诚实、无害的模型。一般来说,非常大的企业常常担心模型会产生幻觉或说出令人非常不悦的话。
Wonderful. Let's talk about use cases. I think one of the major questions people in the audience have today is where companies are finding the most product market fit. And I think you have a unique vantage point on that from anthropic. What are the use cases that you see that are already reaching real product market fit? And what are the use cases that you think are on the come that they're about to reach product market fit? So I think it varies a little bit, first of all, just kind of depending on industry. So there's kind of some industries that I think are kind of quite advanced in generative AI. Unsurprisingly, the technology industry has been an early adopter. That's often how it goes. But I think something that has sort of been interesting for us to see is we just released this new sort of suite of models, the Claude 3, we call it the model family. And so the kind of biggest model, Claude 3 Opus is the kind of state of the art. We sort of joke it's like the Rolls Royce of the models. It's incredibly capable and powerful. And really what we've seen is not everybody needs the kind of top tier state of the art model for all of their use cases. But the times when you do need it is when you need a model that is just incredibly intelligent, capable and powerful. So things like if you're doing scientific research or you're trying to have a model write very complex code for you at a fast pace or do complex macroeconomic policy analysis, Claude 3 Opus is a great fit for that. Claude 3 Haiku, which is the smallest model, this is the Ducati, it's sort of the racing motorcycle is amazing for things like customer support. So really what we've seen in the industry is that speed and cost are very important for anything that kind of requires real time response rates. And then Claude 3 Sonnet, which is sort of that middle model, a lot of enterprise businesses are using for things like day to day retrieval, summary of information. If they have unstructured data that they need to pull together and analyze. And so I would say it varies by industry, but it also sort of varies by use case and just how much ability customers have to kind of choose between what's available for them. Wonderful.
太棒了。让我们谈谈使用案例。我认为今天观众们最关心的一个重要问题是公司在哪些领域找到了最符合市场需求的产品。我认为您从Anthropic的独特视角对此有着独特见解。您认为哪些用例已经实现了真正的产品市场适配?您认为哪些用例即将实现产品市场适配?我认为首先,这在一定程度上取决于行业。有一些行业,我认为在生成AI方面已经相当先进。不出所料,科技行业是早期采用者。这通常是这样的。但我认为我们发现有趣的一点是我们刚刚发布了这一新的模型系列,我们称之为Claude 3模型系列。最大的模型,Claude 3 Opus是目前的领先者。我们有点开玩笑地说它就像是模型中的劳斯莱斯。它非常聪明、强大。而我们看到的是并非所有人都需要顶级的最先进模型来满足他们所有的用例。但当你需要一个极端聪明、强大的模型时,比如做科学研究,或者快速写复杂代码,或者进行复杂的宏观经济政策分析时,Claude 3 Opus就非常适合。Claude 3 Haiku,这是最小的模型,就像杜卡迪,是极速摩托车,非常适合客户支持等用途。在行业中我们看到速度和成本对于需要实时响应的任务非常重要。而Claude 3 Sonnet,这是中间模型,很多企业使用它来进行日常检索,信息摘要。如果他们有需要汇聚和分析非结构化数据的需求。我会说这取决于行业,取决于用例,也取决于客户在选择可用解决方案时的能力。太棒了。
Sure. One or two of your favorite use cases that people have built on anthropic? Yeah, for sure. I would say I'm like a do-go-to-red heart. So one of my favorite use cases is the Dana-Farber Cancer Institute uses Claude to help with genetic analysis. So looking for sort of cancer markers. I think there's also like much more kind of a sort of boring application, but there's a lot of kind of financial services firms like Bridgewater and Jane Street that are really using Claude to help them analyze financial information in real time. I think I like both of those because they really just sort of represent such a wide spectrum. I think it illustrates how truly general purpose these models are. It's a model that can help you to literally try and cure cancer faster, but also to do sort of the day to day bread and butter of illegal services or financial services firms work.
当然。有人在anthropic上构建的一个或两个你最喜欢的用例是什么?是的,当然。我会说我是个喜欢说做就做的人。所以我最喜欢的用例之一是达纳-法伯癌症研究所使用Claude来帮助进行基因分析,寻找癌症标记物。我认为还有一些更加沉闷的应用,像那些金融服务公司,比如布里奇沃特和简街,他们真的在使用Claude来帮助他们实时分析金融信息。我喜欢这两个用例,因为它们展示了如此广泛的应用范围。这说明了这些模型真正的通用性。这种模型可以帮助你加快治愈癌症的速度,同时也可以做金融服务公司日常的法律服务或金融服务工作。
Wonderful. Are you seeing more success in your customer's finding product market fit from startups or from enterprises right now? So I would say for anthropic in particular, we have really focused on kind of the enterprise use case. And again, this is really because we have felt such a resonance in approach for businesses that are interested in building in ways that are trustworthy and reliable, right? All of the things we've sort of been talking about. That being said, I think there's a ton of innovation that is always happening in the startup space. And so something that I think is really interesting to watch is sometimes we'll have kind of a startup sort of prototype something and we'll see like, wow, that's, you know, that's a really fascinating use case. Like we wouldn't have thought that you could use Claude that way. And then that will become something that like enterprise businesses sort of like later learn about because they know someone who works at that startup or they've kind of seen it in production.
太棒了。您现在在初创公司或企业中,您的客户在找到产品市场契合度方面看到了更多成功吗?所以我会说对于Anthropic来说,我们真的非常专注于企业的使用案例。而且,这主要是因为我们认为这种建设方式对于那些希望以可信赖和可靠的方式建设的企业非常有共鸣,对吧?我们之前讨论的所有事情都是如此。
话虽如此,我认为在初创公司领域总是有很多创新。因此,我认为非常有趣的一点是有时我们会看到一个初创公司原型设计某种产品,然后我们会看到,哇,那是一个非常有趣的使用案例。像我们之前没想过可以这样使用克劳德。然后,这将成为企业公司稍后会了解的东西,因为他们认识在那个初创公司工作的人,或者他们已经在生产中看到过。
So my sense is for us personally, we're much more sort of building for and pivoted towards the enterprise. But I think there's really a wide, wide ecosystem of development that that's happening in the business space. On the spectrum from prototyping to, you know, experimentation all the way to production, where do you think most of your customers are today on the journey? Yeah. I think on the kind of, I think for this, I'll like talk about enterprise and then startups because they're a little bit different. I think for enterprises, it actually ranges like pretty, pretty widely. There's some businesses that I would even say have multiple kind of production use cases, right? Where they might be using Claude internally to, you know, analyze health records or help doctors or nurses, you know, analyze notes and save themselves administrative time so they can be with patients more. But if they're a big company, they might also be using it for a chat interface, right? So depending on the business use case, sometimes they have, you know, multiple use cases in production. But it's a little spiky, right? There might be times where one of those, one of those use cases is like quite far along. They've already been in production for like a year. They really like know the question, right? They come to us and they're like, we really, really want to optimize like this metric or we really care about price or we really care about latency. And then there's businesses all the way on the other end of the spectrum who come to us and are like, I've been hearing about generative AI like from my board. You help us understand, is there a solution here, right? And so I think it does vary a lot, but I will say industries, I have personally been surprised that some industries that are not necessarily historically known for being early adopters, like insurance companies or financial services or healthcare, I think are actually great candidates for incorporating this technology and many of them have.
我个人的感觉是,我们更多地在为企业构建和调整。但我认为在商业领域真的有一个非常广泛的生态系统在发展。从原型设计到实验,一直到生产,你认为大多数客户在这个旅程中哪个阶段?
是的。我认为,在这方面,我会谈到企业和初创公司,因为它们有一些不同。我认为对于企业来说,实际上范围很广。有一些企业我甚至会说有多个生产用例,对吧?他们可能在内部使用克劳德来分析健康记录或帮助医生或护士分析笔记,节省自己的时间,这样就可以更多地与患者在一起。但如果他们是一家大公司,他们可能也在使用它作为聊天界面,对吧?所以,根据业务用例,有时候他们有多个生产用例。但它有点突兀,对吧?有时候其中一个用例已经走得很远。他们已经生产了一年,他们真的知道问题了,对吧?他们来找我们,说我们真的,真的想要优化这个指标,或者我们真的很在乎价格,或者我们真的很在乎延迟。然后还有另一端完全不同的企业来找我们,说我的董事会一直在谈论生成式人工智能。你能帮助我们理解,这里是否有解决方案?所以我认为它确实有很大的变化,但我想说,一些历史上不被认为是早期采用者的行业,像保险公司、金融服务或医疗保健,我认为实际上是整合这项技术的绝佳候选者,而其中许多已经这样做了。
Wonderful. Let's move on to cloud three and research. Maybe you just launched cloud three. Maybe tell us a little bit about what went into it and how the reception has been so far.
太棒了。让我们继续谈谈云三和研究。也许你刚刚推出了云三。能否稍微介绍一下它的特点以及迄今为止的反响如何呢?
So yes, we just, just a couple of weeks ago launched cloud three. As I mentioned, it's this sort of model family, right? So there's different models kind of available for different use cases, again, for businesses. And really, I think what has been so interesting is we've gotten great, you know, positive feedback about cloud. Of course, there's always things that we're improving and wanting to do better. But some, something that I have found, you know, really just interesting is customers have sort of simultaneously commented on how kind of capable and powerful the models are, right? They're the most intelligent state of the art models available on the market today.
所以是的,就在几周前我们推出了云三。就像我提到的,这是一种模型系列,对吧?所以针对不同的用例,为不同的企业提供不同的模型。事实上,我认为最有趣的是,我们收到了很多关于云的积极反馈。当然,我们总是在改进和希望做得更好。但有一点让我觉得很有趣,客户同时评论了这些模型的能力和强大性。它们是市场上目前最智能、最先进的模型。
But people have also commented, hey, it's way harder to jailbreak these or the hallucination rates have kind of gone down a lot. And so there has been this kind of dual language around both capability and safety. And then the last piece, which I always find really interesting is many customers have told us part of the appeal of cloud is that cloud feels more human. And so when people kind of interact with or talk to cloud, we've sometimes heard folks say it really feels like talking to, you know, a trusted person versus talking to a robot that was kind of trained to sound like a human.
但是人们也评论说,嘿,越来越难越破解这些设备了,或者幻觉率的下降幅度很大。因此,关于功能和安全性都存在着这种双重语言。最后一个方面,我总觉得很有趣的是,许多客户告诉我们,云服务的吸引力之一在于云服务更具人性化。因此,当人们与云服务进行互动或交流时,有时会听到一些人说,这感觉就像在与一个值得信赖的人交谈,而不是与一个被训练成听起来像人类的机器人交谈。
I love that. And I think everyone here has seen all the eval charts. I think cloud really, one of the areas where it really spikes is in coding, where I think the performance is just off the charts right now. Maybe can you tell us a little bit about how you made the model so good at coding in particular and then how you see the role, how you see AI software engineering playing out and anthropics role in it?
我喜欢这一点。我认为在座的每个人都看过所有的评估图表。我认为云计算确实是一个领域,在编码方面确实是一个亮点,我认为其性能现在简直是超乎想象的。也许你可以告诉我们一点关于如何使模型在编码方面如此出色,然后你如何看待人工智能软件工程的发展以及它在其中的作用?
So I think something that is interesting that I've like learned from my research colleagues so I don't sort of pretend to be an expert on this is as the models just become generally more performative, they kind of like get better at everything. And so I think much of the same training techniques that we used to improve the models, you know, accuracy and reading comprehension and general reasoning were also used to improve its ability to code. And I think that's something that, again, is kind of a fundamental interesting sort of research thing, which is like rising boat sort of lifts all tides.
因此,我认为有趣的是,我从我的研究同事那里学到的一点,我并不是假装自己是一个专家,就是随着模型变得更具表现力,它们在各方面都变得更加优秀。我认为我们用来提高模型准确性、阅读理解和一般推理能力的训练技巧,也被用来提高它编码的能力。我认为这是一个基本有趣的研究,就像是涨潮将提起所有船只一样。
That being said, there's a lot of variety in these models. And something I've always found interesting is certain models, like people are like, I always use this model for like task X right at the consumer level. And other times folks will say this model like you absolutely have to use for task Y. So I think there is a little bit of almost pull through personality that happens with these kind of regardless of the improvements, kind of a useful caveat.
话虽如此,这些模型有很多不同之处。我一直觉得有趣的是,有些模型被人们视为适合某种任务X,例如,在消费者层面上总是使用这个模型。而有时人们会说,对于任务Y,绝对必须使用另一个模型。因此我认为,这些模型可能会带有一些个性特征,无论改进如何,这都是一个有用的警告。
In terms of what are people doing in the sort of software engineering space and kind of what is the role of these models, I'm not a programmer. So I feel like I probably can't opine on this as well as others. But much of what we have heard from our customers is that Claude is a great tool in helping people who write code. Claude cannot replace a human engineer yet, but it can be a great kind of co-pilot in helping. Love that.
就人们在软件工程领域所做的事情以及这些模型的作用而言,我不是程序员。所以我觉得我可能不能像其他人那样发表看法。但是我们从客户那里听到的很多意见是,克劳德是一个很好的工具,可以帮助编写代码的人。克劳德还不能取代人类工程师,但它可以成为一个很好的辅助工具。很喜欢这个想法。
So we have a philosophical research question. How do you think about the role of transparency in AI research, especially as it seems like the AI field has become more and more closed? And Thorpek has always felt very strongly about publishing a large portion of our research. So we don't publish everything, but we have published something like two dozen papers. The vast majority of them are actually technical safety or policy research papers.
所以我们有一个哲学研究问题。你认为透明度在人工智能研究中的作用如何,特别是因为人工智能领域似乎变得越来越封闭?而索普克一直非常坚定地支持发布我们研究的很大一部分。所以我们并不是所有内容都发布,但我们已经发布了大约两打论文。其中绝大部分实际上是技术安全或政策研究论文。
The reason that we choose to publish those are as a public benefit corporation, we really view our job as helping to raise the watermark really across the industry in areas like safety. So we have a team that focuses on something called mechanistic interpretability, which is essentially the art of trying to figure out what is happening inside the black box that is these neural networks. And it's a very kind of emerging field of research. There's like two or three teams in the entire world that work on it.
我们选择发布这些信息的原因是作为一家公益性公司,我们真的认为我们的工作是帮助提高行业的水平,尤其是在安全等方面。因此,我们有一个团队专注于所谓的机械可解释性,这本质上是一种尝试弄清楚这些神经网络内部发生了什么的艺术。这是一种非常新兴的研究领域,全世界可能只有两三个团队在进行这方面的研究。
And we really feel like there's a lot of opportunity when kind of sharing that more broadly with the scientific community to just increase understanding around topics like that, particularly in sort of the elements of safety. So we've shared all of these research papers. And then additionally, we do a lot of work in kind of the policy sphere and try and publish research results. Papers are red teaming results as well. Thank you.
而且我们真的觉得,如果我们可以将这些更广泛地与科学界分享,就会有很多机会来增进对诸如这类主题的理解,特别是在安全方面。所以我们分享了所有这些研究论文。另外,我们还在政策领域做了很多工作,努力发表研究结果。也包括对结果进行了红队测试。谢谢。
One of the big themes of today's event is trying to think about what's next. So I was hoping to ask from your vantage point, what are the biggest challenges that you see your customers facing or your researchers thinking about when they're trying to build with LLMs? Like where are they hitting a wall? And how is anthropic working to address some of those problems?
今天活动的一个重要主题是尝试思考下一步。所以我希望从您的角度来问一下,您认为您的客户面临的最大挑战是什么,或者您的研究人员在尝试使用LLMs构建时正在思考什么?他们在哪些方面遇到了障碍?Anthropic是如何努力解决这些问题的?
So I think there's a few kind of classes of ways that these models are still sort of, they're still not perfect, right? I think one big one is there are just fundamental kind of challenges to how these models are developed and trained and used.
我认为这些模型仍然存在一些分类方式,它们仍然不完美,对吧?我认为一个重要的问题是这些模型的开发、训练和使用存在基本性的挑战。
So the kind of prototypical one that's talked about is this hallucination problem, right? I'm sure everyone in the room knows this, but models are just trained to predict the next word. And so sometimes they don't know the right answer. And so they just make something up.
人们经常谈论的是一种经典的问题,就是幻觉问题,对吧?我相信房间里的每个人都知道这一点,但模型只是被训练来预测下一个词。因此有时候它们不知道正确答案。所以它们只好凭空捏造。
And we have made a huge amount of progress as an industry in reducing hallucination rates from like the GPT-2 era. But they're still not perfect. I'm not entirely sure what this sort of decrease curve will look like for hallucination rate, right? We keep getting better at it. I'm not sure if we'll ever be able to get models to zero. That is a fundamental challenge for businesses, right?
作为一个行业,我们在降低幻觉率方面取得了巨大进展,从类似GPT-2时代开始。但它们还不完美。我不确定幻觉率会呈现怎样的下降曲线,对吧?我们在这方面不断取得进步。我不确定我们是否能够让模型完全消除幻觉。这对企业来说是一个根本性的挑战,对吧?
If your model is going to even very occasionally hallucinate for some of the highest stakes decisions, you probably wouldn't choose to use a model alone, right? You would say, hey, we need a human in the loop.
如果你的模型在一些最关键的决定时甚至偶尔出现幻觉,你可能不会选择仅仅使用一个模型,对吗?你会说,嘿,我们需要一个人参与进来。
And I do think something that's kind of very interesting is there's a really small set of cases today where LLMs alone can do the majority of the task, right? Like their best, again, I think in tandem with a human for the majority of kind of use cases.
我觉得有一件非常有趣的事情是,今天有一小部分案例,LLM独自就可以完成大部分任务,对吧?就像最好的情况下,我认为与人类一起合作,对于大部分使用案例来说,是最好的。
I also think there's just sort of this interesting. It almost feels a little more philosophical, which is just what are humans actually comfortable with giving to models, right? I think part of the sort of human in the loop story is also about helping, you know, businesses and industries and individuals feel more comfortable with an AI tool making fundamental decisions.
我认为这是有趣的一点。这个问题几乎带有一种更加哲学化的感觉,那就是人类实际上愿意向模型提供什么。我认为人在决策过程中的一部分也是在帮助企业、产业和个人感觉更加舒服,让人工智能工具做出基本决策。
Thank you for sharing that. A few of the folks here spoke about planning and reasoning. Is that something you all are thinking about at Enthropic? And could you share a few words on that? Yeah, definitely.
谢谢你分享这个。这里有一些人谈到了规划和推理。在Enthropic,你们是否正在考虑这些问题?你能分享一些想法吗?是的,当然。
So that can obviously mean a few things. So I think on the kind of dimension of like, how do you get these models to sort of like execute sort of multi-step instructions, right? I'm assuming that's kind of what planning means.
这可能有几种意思。我认为在这种维度上,如何让这些模型执行多步指令,是一种规划的意思。
You know, it's really interesting. There's a lot of research and kind of work that has gone into this sort of concept of like agents, right? Like how do you give the models the ability to like take control of something and like, you know, execute multiple actions in a row and like, can they plan, right? Can they sort of think through like a set of steps?
你知道,这真的很有趣。许多研究和工作已经投入到这种代理概念中。比如,如何让模型有能力控制某物并执行多个行动,他们能规划吗?他们能够思考一系列步骤吗?
I do think that Claude 3 sort of represented for us elite between kind of the last generation of models in its sort of ability to do that. But I actually think that level of kind of agentic behavior is still really hard. Like I think the models cannot quite do that reliably yet.
我认为Claude 3在某种程度上为我们展示了上一代模型之间的精英地位,因为它具备了这种能力。但事实上,我认为这种主动行为水平仍然非常困难。我认为模型们还不能够可靠地做到这一点。
Again, this feels like such a sort of fundamental research question that I don't know how long it will be until that's not the case. But I don't think it's the sort of, you know, the dream of like, can I just ask Claude to book my flight for me, like, please go book my reservation hotel, just plan my vacation.
再次,这个问题感觉像是非常基础的研究问题,我不知道要多久才能不再是这种情况。但我不认为这是那种可以让克劳德帮我订机票,订酒店,安排度假活动的梦想。
I don't actually think that that's like immediately around the corner. I think there's still some research work and engineering work that needs to go into making that possible. Yep. Yep. Okay.
我并不认为这个就在眼前。我认为仍然需要进行一些研究和工程工作才能使这成为可能。是的。是的。好的。
So the future is coming. The future is coming quickly. It's also coming, Choppily. It's a little unclear exactly which parts of it are going to come where. Okay. Very cool.
所以未来就要来了。未来来临得很快。它的到来也有点不稳定。具体哪些部分会在哪里出现还有点不清楚。好的,非常酷。
Can we talk about AI safety for a moment and Thropic really made a name for itself on AI safety. And I think you were the first major research institution to publish your responsible scaling policies.
我们可以稍微谈一下AI安全性吗?Thropic在AI安全领域确实声名鹊起。我认为你们是第一个主要研究机构公布负责任的规模政策的。
How do you balance innovation and accountability and how would you encourage other companies and make a system to do that as well? So something that we kind of get asked a lot is, you know, how do you all plan to compete if you're, you know, so committed to safety?
你如何平衡创新和责任,并如何鼓励其他公司建立一个类似的系统呢?所以我们经常被问到的一个问题是,如果你们如此注重安全,你们计划如何竞争呢?
And something that I think has been, you know, really interesting is many fundamental safety challenges are actually business challenges. And rather than sort of thinking of these two as something that, you know, two sides that are kind of opposed to each other, I actually think the past kind of mainline success in generative AI development runs through many of the safety topics we've been talking about, right?
我认为非常有趣的是许多基本的安全挑战实际上是商业挑战。与其将这两个方面看作是彼此对立的两面,我认为过去在生成式人工智能发展方面取得主要成功的路线实际上经过了我们正在讨论的许多安全主题,是吧?
Most businesses don't want models that are going to like spout harmful garbage, right? Like that's just not a useful product. The same thing is through like if the model refuses to answer your questions, if it's, if it's dishonest, right? If it makes things up, those are sort of fundamental business challenges in addition to kind of technical safety challenges.
大多数企业不希望模型会输出有害的内容,对吧?这对他们来说并没有任何帮助。同样,如果模型拒绝回答问题,或者不诚实,编造虚假信息,这些问题都是基本的商业挑战,除了技术安全方面的挑战之外。
I also think something we have really aimed to do as a business is sort of take the responsibility of developing this very powerful technology quite seriously, right? We sort of have the benefit of being able to look back on several decades of social media and say like, wow, much of what social media did for the world was incredibly positive. And there were these externalities that nobody predicted that it created, which I think are sort of now widely believed to be quite negative for people. So I think anthropic has always aimed to say, what if we could try and sort of build this technology in a way that better anticipates what some of those risks are and helps to prevent them? And the responsible scaling policy is basically our first attempt to do that, right? It might not be perfect. There could be things about it that are sort of laughably wrong later. But really what we've said are, what are the dimensions on which something can go wrong here, right?
我也认为作为一家企业,我们真正努力做的事情之一就是认真对待发展这种非常强大技术的责任,对吧?我们有幸能够回顾几十年的社交媒体发展,可以说,社交媒体为世界做出了非常积极的贡献。但也有一些外部因素是没有人能预测到的,这些因素创造了很多现在被广泛认为对人们有负面影响的情况。因此,我认为 Anthropia 一直致力于尝试构建这种技术的方式,可以更好地预测到一些风险,并有助于预防它们。而负责任的扩展政策基本上是我们第一次尝试这样做,对吧?它可能并不完美,迟早会发现其中有些笑话般错误的地方。但我们真正关注的是,这里可能出现哪些错误的方面呢?
And RCO, my brother, Dario testified to Congress about the potential risks for gender of AI to develop things like chemical and biological weapons. And what we've said is we actually have to do proactive work to ensure that these models are not able to do that. And the responsible scaling pact is really just a way of sort of saying, hey, we're committing to doing that work. Thank you for sharing that. Let's see. Any questions from the audience? Yes. Thanks so much. One of the things that I think was really awesome about the Claude Opus release was that it was really strong, specific performance in a few domains of interest. And so I was wondering if you could talk more about kind of like technically how you view the importance of research versus compute versus data for specific domain outperformance and what the roadmap looks like for where Claude will continue to get better. Yeah. That's a great question.
RCO,我的兄弟达里奥向国会作证,警告了人工智能发展化学和生物武器等潜在风险对性别的影响。我们认为确实需要积极的工作来确保这些模型无法做到这一点。而负责任的扩展协议实际上只是一种表明我们承诺做这项工作的方式。谢谢你分享这些信息。让我们看看,有没有观众提问?是的。非常感谢。我认为克劳德作品发布中的一个非常棒的地方在于它在一些领域的特定表现非常强。因此,我想知道您是否可以更详细地谈谈从技术上来看,您如何看待研究、计算和数据对特定领域表现的重要性,以及克劳德将如何继续变得更好的路线图。是的,这是一个很棒的问题。
I think my real answer is that I think you're probably giving the industry more credit than it deserves for having some like perfectly sort of planned structure between like, we'll sort of research area X and like increased compute will improve Y. I think there's a way in which training these large models is more a process of discovery by our researchers than kind of intentional deliberate decisions to like improve particular areas to kind of go back to that like rising tide lifts all boats sort of analogy. Making the models just generally more performative tends to just make everything better sort of across the board.
我认为我的真正答案是,我认为你可能给了这个行业比它应得的更多的赞誉,认为它在研究领域X和增加计算能力会改善Y之间有一种完美计划的结构。我觉得培训这些大型模型更像是我们研究人员的一种发现过程,而不是一种刻意的决策来改进特定领域,回到那个“水涨船高”的类比。通常情况下,使模型更加高效往往会在各个方面都变得更好。
That being said, there is sort of particular targeted work that we did do in some sub areas with constitutional AI and reinforcement learning from human feedback where we just saw that performance wasn't quite as good. But it's actually a smaller fraction than you might think compared to just generally improving the models and making them better. It's a great question. Yes, Sam. I've been loving playing with Claude III. Claude Opus, it's fantastic. And I totally agree it feels way more human to talk to you. One thing I've noticed that it almost feels like a specific human like it has a personality.
说到这一点,我们确实在某些细分领域开展了针对性的工作,其中包括了基于宪法的人工智能和从人类反馈中强化学习的工作,我们发现性能并不如预期那样好。但实际上,与仅仅改进模型并提高性能相比,这只是一个较小的部分。这是一个很好的问题。是的,萨姆。我一直很喜欢和Claude III一起玩。克劳德奥普斯,太棒了。我完全同意,与你交谈感觉更加像在和一个真人说话。我注意到一个事情,它几乎感觉像一个特定的人类,就像它拥有自己的个性。
And I'm kind of curious as you guys continue to work in this domain and make other models, how you see the boundary of kind of like personality development. If people are kind of trying to create specific characters, is there kind of a stance you guys are taking from the constitutional perspective of the boundaries of how Claude can actually play a character other than itself? So something that is really, I think unusual about kind of Claude is just how like seriously Claude will take feedback about its tone. If you're like Claude, you are, this is too wordy. Like please just be very factual and talk to me like I have a financial analyst, try it out.
我有点好奇,当你们继续在这个领域努力并制作其他模型时,你们如何看待个性发展的边界。如果人们试图创造特定角色,你们是否从宪法的角度来看,克劳德实际上可以扮演一个角色,而不仅限于克劳德本身?关于克劳德的一点真的很不同寻常,那就是克劳德会认真对待对其音调的反馈意见。如果你像克劳德一样,你会觉得这太啰嗦了。请简明扼要地跟我说,就像我是一名财务分析师,试试看。
Claude will absolutely sort of adjust its style to be more kind of in that sort of mil you or hey, I'm writing a creative writing story, like please use very flowery language or talk to me like you're angry at me or talk to me like you're sort of you know friendly or whatever. I think there's sort of an interesting other thing you're asking though, which is like what is the default mode that we should be setting these models kind of personalities to be. And I don't think we've sort of landed on kind of the perfect spot. But really what we were aiming for was like what is a slightly wiser, better version of us kind of how would they react to questions, right? Like some humility, I'm sorry I missed that. Thanks so much for the feedback, like I'll try to do that better.
Claude会完全调整其风格,让它更像是优雅的,或者像是“嘿,我在创作一个故事”,就像是请使用非常华丽的语言,或者像是你在对我生气,或者像是你对我友好之类的。不过,我觉得你还在询问另一件有趣的事,那就是我们应该将这些模型的个性设置为什么样的默认模式。我认为我们还没有找到完美的位置。但我们的目标其实是,如果有一个略微更明智、更好的版本的我们,他们会如何应对问题呢?像是有些谦卑,我很抱歉我错过了那个。非常感谢您的反馈,我会努力改进的。
I think there's kind of an interesting fundamental question though, which is as the kind of marketplace evolves, do people want like particular types of kind of chat bots or chat interfaces to sort of treat them differently, right? Like you might want to sort of coax a particular form of customer service bot to be like particularly obsequious or I don't know, there are just kind of other potential use cases. My guess is that's probably going to end up being the province of like startups that are built on top of tools like Claude. And I think our stance might vary a little bit there, but in general we've tried to start from a like friendly humble base and then let people tweak them as they go within boundaries of course.
我认为有一个有趣的基本问题,随着市场的发展,人们是否想要特定类型的聊天机器人或聊天界面来对待他们不同,对吧?比如你可能想要诱使一个特定形式的客服机器人特别谄媚或其他潜在用例。我猜这可能最终会成为像克劳德这样的工具上构建的初创企业的领域。我认为我们的立场可能会有所不同,但总体上,我们试图从友好谦卑的基础出发,然后让人们根据自己的需求进行调整,当然在一定范围内。
Hey, so the developer experience on Claude and the new generation of Claude 3 models is markedly different than other LLM providers, especially the use of XML as like a prompt template format. How are you thinking about introducing switching costs here and especially in the long term, do you want it to be an open ecosystem where it's very easy to switch between anthropic and your various competitors or are you thinking about making more of a closed ecosystem where I'm working directly with anthropic for all of my model needs?
嘿,Claude和新一代Claude 3型号的开发者体验与其他LLM提供商有明显区别,特别是在使用XML作为提示模板格式方面。您如何考虑引入转换成本,特别是从长远来看,您希望它成为一个开放的生态系统,让在Anthropic和其他竞争对手之间轻松切换,还是您考虑更多地建立一个封闭的生态系统,直接与Anthropic合作满足我的所有模型需求?
So I think maybe the best way to answer this is what we've seen kind of in the market today, which is that most like big businesses are interested in at some point, you know, some of them just use one model, but they like to try them out. And my guess is that likely developers will have that same instinct, right? So I think the more kind of open, hey, like it's whatever, it's easy to download your data, move it over. I think that's the sort of goal that we're trying to eventually aim towards.
因此,我认为也许最好的回答方式是我们今天在市场上所看到的情况,即大多数大型企业都对某种程度上感兴趣,有些企业可能只使用一个模型,但他们喜欢尝试不同的模型。我猜想开发人员可能会有相同的本能,对吗?因此,我认为更开放、更容易下载数据、迁移数据的方式可能是我们最终要努力实现的目标。
The one sort of difference I would say is that often developers, particularly when they're just getting started, are like the switching costs are just more laborious for them, right? They're like, hey, I'm building on this tool. It's annoying to switch. Like it's complicated to switch. You have to sort of redo your prompts because all of the models like react a little bit differently just depending on them. And like we have great prompt engineering resources, like please check them out. Also, it just takes some time and effort to like understand the kind of new personality of the model that you're using.
我想说的一种区别是,通常开发人员,特别是刚开始的时候,会觉得切换成本对他们来说更加繁琐,对吧?他们会觉得,嘿,我正在使用这个工具,要更换很麻烦。就像是很复杂。你必须重新设置提示,因为所有的模型反应有点不同,取决于它们。我们有很棒的提示工程资源,请去查看。另外,需要一些时间和精力来了解你所使用的模型的新性格。
I think my kind of short answer is yes, we're aiming for sort of that more open ecosystem, but also it's sort of tactically hard to do in kind of a perfect way. With interpretability research, I'm curious what you think is coming first to the product? What is looking most optimistic where I could say like turn on a switch and have it only output Arabic or something like that? What do you think is like closest working?
我认为我的回答比较简短,答案是肯定的,我们的目标是构建更加开放的生态系统,但在实践中很难以完美的方式去做到。就可解释性研究而言,我想知道你认为对产品而言最重要的是什么?哪个看起来最令人乐观,我可以打开开关,让它只输出阿拉伯语或其他什么?你认为哪个是最接近实现的?
So interpretability is a team that is deeply close to my heart despite me, like not being able to contribute anything of value to them other than telling them how great they are. I think interpretability is to me like the coolest and most exciting area that I researched today because it's fundamentally trying to figure out like what what are these models actually doing, right? It's like the neuroscience of like large models. I actually think we're like not impossibly far, but like not that close from being able to sort of productionize something and interpretability today, right?
因此,可解释性是一个让我非常关心的团队,尽管我无法为他们贡献任何有价值的东西,除了告诉他们他们有多么伟大。我认为可解释性对我来说是我今天研究过的最酷最令人兴奋的领域,因为它基本上是在试图弄清楚这些模型实际上在做什么,对吧?这就像是大型模型的神经科学。我实际上认为我们离实现某种程度的可解释性今天并不是不可能的,但也并不是那么远,对吧?
The kind of neuroscience analogy is a little bit strained, but I actually think it's it's relevant in one particular way, which is that like we can have a neuroscientist like look at your brain and be like, well, we know that these two things light up when you think about dogs, but it can't sort of like change you thinking about dogs, right? It's like you can sort of diagnose and understand and see things, but you can't actually like go in and change them yet. And I think that's about where we are at sort of the interpretability level.
这种神经科学的类比有点勉强,但我实际上认为在一个特定方面是相关的,那就是就像我们可以让神经科学家查看你的大脑并说,嗯,当你想到狗时,这两个区域会亮起,但不能改变你对狗的思考,对吧?就像你可以诊断和理解和看到事物,但还不能真正的去改变它们。我认为这大概是我们在可解释性水平上所处的位置。
Could we offer some insight like in the future? I think almost certainly yes, probably not even on a crazy long time scale, right? We could say, hey, if you're playing with sort of, you know, this type of model and it's it's, you know, it's activating strangely. I think that's the type of thing we could like show a sort of visualization to a customer of. I don't actually know how actionable it is, if that makes sense, right? And sort of the same way you're like, well, these these sort of two parts of the model are lighting up or this set of neurons is activating. But I think it's it's it's an interesting area of like very basic science or basic research that I think could have incredible potential applications like a couple of years from now.
我们可以像在未来一样提供一些见解吗?我认为几乎可以肯定是可以的,可能甚至不需要在很长时间尺度上,对吧?我们可以说,嘿,如果你在玩这种模型,并且它,你知道,激活得很奇怪。我认为我们可以向客户展示这种类型的可视化。我其实不知道这有多具体可行,如果你明白我的意思,对吧?就像你说的,这个模型的这两部分会亮起,或者这组神经元在激活。但我认为这是一个非常基础科学或基础研究领域,我认为未来几年可能会有令人震惊的潜在应用。
I'll ask a question. What maybe give the folks here a taste of what's going to come on the product roadmap? Let's assume that Claude gets smarter and smarter. But what are you all going to add on the developer facing product? And then what should we expect in terms of first party products from you? So first of all, we we are just sort of scrambling day in and day out to try and keep up with the incredible demand that we have. So we are incredibly grateful for everybody's patience. But I think really on the kind of, you know, developer side, we really want to just up level the tools that are available for developers to be able to kind of make make the most use of Claude sort of broadly. I think something that's really interesting, just sort of speaking to the kind of ecosystem point is there's so much opportunity for like knowledge sharing and sort of learning between developers and between people that are kind of using these models and tools. So we're also very interested in just sort of figuring out how to host more information sharing about how to get the most out of these models as well. Oh, you have the mic. Yes, go for it. Given your focus on safety, I was hoping you could comment on how you see the regulatory landscape evolving. Maybe not so much for you specifically, but for the companies that are using your models and others. So something that I think is just always an unknown is like, what's going to happen in the regulatory landscape? And how is it going to impact like how we build and do our work kind of in this space? I think, I mean, first of all, I don't have any amazing questions to say like this set of regulations I expect will happen. But I imagine what we'll see is kind of, it will probably start from the place of the consumer because that's really what kind of government and regulators are sort of most well positioned to try and defend or protect.
我将提出一个问题。也许可以给在座的人们一点产品路线图即将推出的味道?假设克劳德会变得越来越聪明。但你们将在面向开发者的产品上添加什么?然后我们应该期待从你们那里的第一方产品有什么呢?首先,我们每天都在挣扎,试图跟上我们不可思议的需求。所以我们非常感激大家的耐心等待。但我认为真正的问题在于,你知道,开发方面,我们真的想要提升为开发者提供的工具,使他们能够更广泛地充分利用克劳德。我认为非常有趣的一点,只是从生态系统的角度来看,有很多机会进行知识共享和学习,开发者之间以及使用这些模型和工具的人之间之间。因此,我们也非常感兴趣了解如何举办更多关于如何最大限度利用这些模型的信息分享。哦,你有话筒。是的,说吧。鉴于你们对安全的关注,我希望你能评论一下你认为监管格局如何发展。可能不是针对你们特别,而是针对使用你们模型和其他公司的公司。我认为一个永远是未知的是,监管格局会发生什么,以及它将如何影响我们在这个领域建设和工作的方式。我想,首先,我没有什么惊人的话要说,比如预计会发生这一系列法规。但我想我们将看到的可能会从消费者方面开始,因为这确实是政府和监管机构所最擅长尝试保护或维护的。
And I think a lot of the kind of narrative around data privacy is one that I expect will sort of see emerge, right? Around just, hey, what are you doing with my data? Right? People put personal things into these into sort of these interfaces and they want to know like, are the companies being responsible with that information? Right? What are they doing to protect it? Are they deanonymizing it? We don't train on people's data. But if other companies do, like what does that mean for that person's information? Completely speculative, but that sort of is my guess of where things will start. I also think there is a lot of interest and activation in sort of the policy space right now around like how to develop these models in a way that is safe from a sort of bigger picture, like capital S perspective, right? Some of the sort of scary things I talked about. But again, like regulation is a sort of it's a long process. And I think something we have always aimed to do is work closely with policymakers to give them as much information as possible so that there is thoughtful regulation that will, you know, prevent some of the potentially bad outcomes without sort of stifling innovation. Thank you. Thank you. We have time for one more question. OK, one more. I'm getting, I'm getting looks from Emma. Sorry.
我认为围绕数据隐私的叙述很大程度上会逐渐出现,对吧?人们把个人信息输入到这些界面中,他们想知道,公司是否负责任地处理这些信息?他们在做什么来保护它?他们是否在去匿名化它?我们不使用个人数据进行训练。但如果其他公司这样做了,那对该人的信息意味着什么?纯属猜测,但我认为事情将从这里开始。我也认为,在政策领域,人们对如何以一种从更大的角度来看是安全的方式来开发这些模型很感兴趣并在积极行动。一些我提到的可怕的事情。但是,再一次,监管是一个长期的过程。我认为我们一直努力与政策制定者密切合作,以尽可能提供尽量多的信息,这样就会有深思熟虑的监管,防止一些潜在的不良后果而不会扼杀创新。谢谢。谢谢。我们还有时间再问一个问题。好的,再一个。艾玛看着我。对不起。
Hey, Daniel. Claude, please. Awesome. Thank you. When you think about the model family and the hierarchy of models, you've any thoughts on whether it is effective to use prompts or if you've done any work internally on giving the smaller models inside that larger models are available at kind of say, hey, this is beyond my knowledge, but this is a good time to use the larger model. That is such a good idea. Are you looking for a job? That is a good. That's a great idea. That has not been something we have currently trained the models to do.
嘿,丹尼尔。克劳德,请。太棒了。谢谢。当你想到模型家族和模型的层级时,你对使用提示是否有效,或者你是否在内部工作中进行了任何关于内部模型的较小模型可用的工作,比如说,“嘿,这超出了我的知识范围,但现在是使用较大模型的好时机”。这是一个很好的主意。你在找工作吗?这是一个好主意。这是一个伟大的主意。我们目前还没有训练模型来做这件事。
I actually think it's a great idea. Something we, something we have thought about is just how to kind of make the process of switching between models within a business, just much more seamless, right? You can imagine that over time, the model should know like, hey, you're, you're not actually like trying to look at like macroeconomic trends in like the 18th century right now. You're just like trying to answer a sort of frontline question. You don't need opus. You need haiku.
我其实觉得这是一个很好的主意。我们考虑过的一个问题就是如何让企业内部模型切换的过程更加流畅。随着时间的推移,模型应该知道,你现在并不是在研究18世纪的宏观经济趋势,而是在回答某个前线问题。你不需要一部长篇巨著,你需要一首俳句。
And I think some of that is sort of a research challenge and some of it is actually just a product and engineering challenge, right, which is how well can we kind of get the models to self-identify the level of difficulty and really sort of price optimize right for customers to say, you don't actually need opus to do this task. It's really, really simple. Pay, you know, a tiny fraction of the cost for haiku and we'll just switch you to sonnet if it's sort of somewhere in the middle. We're not like, we're not there yet, but I think that's definitely something we've been, we've been thinking about in a request we've been hearing from customers, but I love your idea of adding in the sort of, sort of like self-knowledge of the models. It's a cool idea.
我认为这部分可能是一个研究挑战,也有部分实际上是产品和工程挑战,对吧,就是我们能够如何让模型自我识别困难程度,真正为客户优化价格,告诉他们其实不需要奥普斯来完成这项任务。这是非常简单的。你只需要支付很少成本就可以用俳句,如果在某种程度上介于两者之间,我们会切换到十四行诗。虽然目前还没有完全实现,但我认为这绝对是一个我们一直在考虑的问题,也是客户一直在提出的需求,不过我很喜欢你提出的关于模型自我认知的想法,这是个很酷的想法。
The call of friends. Exactly. Yeah. Wonderful. Thank you so much, Danielle. Thank you for sharing with us today. Thanks for having me.
朋友的电话。没错。是的。太棒了。非常感谢你,丹妮尔。谢谢你今天与我们分享。谢谢你接待我。