The AI opportunity: Sequoia Capital's AI Ascent 2024 opening remarks - YouTube
发布时间 2024-03-26 14:18:10 来源
中英文字稿 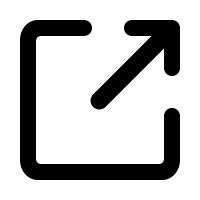
My name is Pack Radie. I'm one of the members of Team Sequoia. I'm here with my partners Sonia and Constantine, who will be your MCs for the day. And along with all of our partners at Sequoia, we would like to welcome you to AI Ascent. There's a lot going on in the world of AI. We have an objective to learn a few things while we're here today. We have an objective to meet a few people who can be helpful in our journey while we're here today. And hopefully we'll have a little bit of fun. So just to frame the opportunity. What is it?
我的名字是帕克·拉迪。我是Sequoia团队的成员之一。我与我的合作伙伴索尼娅和康斯坦丁一起在这里,他们将是今天的主持人。与Sequoia的所有合作伙伴一起,我们想欢迎您参加AI Ascent活动。人工智能的世界正在发生很多变化。我们今天的目标是学到一些东西。我们今天的目标是认识一些可以在我们的旅程中帮助我们的人。希望我们能有一点乐趣。因此,简单概括一下这个机会。这是什么?
Well, a year ago, it felt like this magic box that could do wonderful, amazing things. I think over the last 12 months, we've sort of been through this contracted form of the hype cycle. We had the peak of inflated expectations. We had the trough of disillusionment. We're crawling back out into plateau of productivity. And I think we've realized that what LLM's, what AI really brings to us today are three distinct capabilities that can be woven into a wide variety of magical applications. The first is the ability to create, hence the name, generative AI.
一年前,它感觉就像是一个能够做出奇妙和惊人事物的魔盒。我认为在过去的12个月里,我们经历了炒作周期的收缩形式。我们经历了期望过高的高峰,经历了幻灭的低谷。现在我们正在重新爬出生产力平台。我认为我们已经意识到LLM,AI今天真正给我们带来的是三种可以融入各种神奇应用的独特能力。第一是创造能力,因此有了“生成式AI”这个名称。
You can create images. You can create text. You can create video. You can create audio. You can create all sorts of things. Not something software has been able to do before. So that's pretty cool. The second is the ability to reason. Could be one shot. Could be multi step, a gintech type of reasoning. But again, not something software has been able to do before. Because it can create, because it can reason, we've sort of got the right brain in the left brain covered, which means that software can also, for the first time, interact in a human like capacity.
你可以创作图像。你可以创作文本。你可以创作视频。你可以创作音频。你可以创作各种各样的东西。这是软件以前无法做到的。所以这很酷。第二点是推理能力。可以是一次性的。也可以是多步骤的吉恩科技类型的推理。但同样,这也是软件以前无法做到的。因为它可以创作,因为它可以推理,我们可以说左脑右脑都有了,这意味着软件也可以首次以类似人类的能力进行互动。
And this is huge, because this has profound business model implications that we're going to mention on the next slide. So what? A lot of times we try to reason by analogy when we see something new. And in this case, the best analogy that we can come up with, which is imperfect for a million reasons, but still useful, is the cloud transition. Over the last 20 years or so, that was a major tectonic shift in the technology landscape that led to new business models, new applications, new ways for people to interact with technology. And if we go back to some of the early days of that cloud transition, this is circa about 2010.
这一点非常重要,因为这对商业模式有深远的影响,我们将在下一张幻灯片中提到。那又怎样?很多时候,当我们看到一些新事物时,我们会试图通过类比来推理。在这种情况下,我们能想到的最好的类比是云转换,尽管有许多缺陷,但仍然很有用。在过去的20年左右,这是技术领域的重大地壳运动,引发了新的商业模式、新的应用程序,以及人们与技术互动的新方式。如果我们回顾一下云转换的早期阶段,大约是在2010年左右。
The entire pie, the entire global tam for software is about 350 billion, of which this tiny slice just $6 billion is cloud software. Fast forward to last year, the tam has grown from about 350 to 650, but that slice has become 400 billion of revenue. That's a 40% Kager over 15 years. That's massive growth. Now, if we're going to reason by analogy, cloud was replacing software with software. Because of what I mentioned about the ability to interact in a human-like capability, one of the big opportunities for AI is to replace services with software.
整个软件全球市场的总额约为3500亿美元,其中云软件仅为60亿美元,只占很小一部分。快进到去年,市场规模从大约3500亿增长到6500亿,但云软件的收入却增长到了4000亿美元。这意味着在15年内,云软件增长了40%。这是巨大的增长。现在,如果我们进行类比推理,云软件正在以软件替代软件。鉴于我之前提到的人类化能力,人工智能的一个重要机会是将服务替代为软件。
And if that's the tam that we're going after, the starting point is not hundreds of billions, the starting point is possibly tens of trillions. And so you can really dream about what this has a chance to become. And we would posit, and this is a hypothesis, as everything we say today will be, we would posit that we were standing at the precipice of the single greatest value creation opportunity mankind has ever known. Why now?
如果这就是我们要追求的目标,起点不是数百亿,可能是数万亿。因此,你可以真正梦想这个机会会变成什么样子。我们将提出一个假设,正如今天我们说的一切都是假设一样,我们认为我们正站在人类有史以来最伟大的价值创造机会的悬崖边上。为什么是现在呢?
One of the benefits of being part of Sequoia is that we have this long history and we've gotten to sort of study the different waves of technology and understand how they interact and understand how they lead us to the present moment. We're going to take a quick trip down memory lane. So in the 1960s, our partner Don Valentine who founded Sequoia was actually the guy who ran the Goto market for Fairchild Semiconductor, which gave Silicon Valley its name with Silicon-based transistors. We got to see that happen.
作为Sequoia的一员,其中一个好处就是我们拥有悠久的历史,已经研究了不同技术浪潮,并理解它们是如何相互作用的,以及它们如何引导我们走向现在的时刻。让我们快速回顾一下历史。在1960年代,我们的合作伙伴唐·瓦伦丁(Don Valentine)创立了Sequoia,实际上是为Fairchild半导体公司运营了Goto市场的人,这就是用硅基晶体管为硅谷赢得了名声。我们见证了这一切发生的过程。
We got to see the 1970s. When systems were built on top of those chips, we got to see the 1980s. When they were connected up by networks with PCs as the endpoint and the advent of package software. We got to see the 1990s when those networks went public facing in the form of the internet changed the way we communicate changed the way we consume. We got to see the 2000s. When the internet matured to the point where it could support sophisticated applications, which became known as the cloud.
我们经历了1970年代。当系统建立在这些芯片之上时,我们看到了1980年代。当它们通过网络与个人电脑连接起来,并出现了软件包的时代。我们看到了1990年代,当这些网络公开面向互联网的形式改变了我们的沟通方式,改变了我们的消费方式。我们看到了2000年代,当互联网发展到可以支持复杂应用程序的程度,这被称为云。
We got to see the 2010s where all those apps showed up in our pocket in the form of mobile devices and changed the way we work. So why do we bother going through this little build? Well, the point here is that each one of these waves is additive with what came before. And the idea of AI is nothing new. It dates back to the 1940s. I think neural nets first became an idea in the 1940s. But the ingredients required to take AI from idea, from dream, into production, into reality, to actually solve real world problems in a unique and compelling way that you can build a durable business around. The ingredients required to do that did not exist until the past couple of years. We finally have compute that is cheap and plentiful. We have networks that are fast and efficient and reliable. Seven of the 8 billion people on the planet have a supercomputer in their pockets. And thanks in part to COVID, everything has been forced online. And the data required to fuel all of these experiences readily available. And so now is the moment for AI to become the theme of the next 10 probably 20 years. And so we have as strong conviction as you could possibly have in a hypothesis that has not yet proven that the next couple of decades are going to be going to be the time of a I.
我们能够见证到2010年代,所有这些应用程序以移动设备的形式出现在我们的口袋里,并改变了我们工作的方式。那么为什么我们要经历这么一番建设呢?嗯,重点在于每一波潮流都是在前一波的基础上叠加而来。人工智能的概念并非新鲜事物,可以追溯到1940年代。我认为神经网络最早是在1940年代提出的概念。但将人工智能从概念、梦想,转化为实际生产、现实解决真实世界问题的能力,在其周围构建一个稳定的业务所需的各种要素直到最近几年才齐备。我们现在拥有便宜和充足的计算力,快速高效可靠的网络,全球80亿人口中有7/8人口都拥有一部超级计算机在口袋里。并且在某种程度上要感谢COVID,一切都被迫转移到线上。提供所有这些体验所需的数据也是随手可得。因此,现在是人工智能成为未来10到20年的主题的时刻。我们对这个尚未被证明的假设具有极端的信念,即未来几十年将会是人工智能的时代。
What shape would that opportunity take? Again, we're going to analogize to the cloud transition and the mobile transition. These logos on the left side of the page. Those are most of the companies born as a result of those transitions that got to a billion dollars plus of revenue. The list is not exhaustive, but this is probably 80% or so of the companies formed in those transitions that got to a billion plus of revenue, not valuation revenue. The most interesting thing about this slide is the right side. And it's not what's there. It's what isn't there. The landscape is wide open. The opportunity set is massive. We think if we were standing here 10 or 15 years from today.
那个机会会呈现什么形状呢?再次,我们将类比云端转型和移动转型。页面左侧的这些标志。这些标志大多是作为这些转型的结果而诞生的公司,它们的营收超过十亿美元。这个列表并不详尽,但大概包括了这些转型时期中的80%左右的公司,它们的营收超过十亿美元,不是估值。这张幻灯片最有趣的地方在右侧。但是有趣的不是那里的内容,而是那里没有什么。这片区域是开放的。机会集合是巨大的。我们认为如果我们站在今天的十到十五年后这儿。
That right side is going to have 40 or 50 logos in it. Chances are it's going to be a bunch of the logos of companies that are in this room. This is the opportunity. This is why we're excited. And with that, I will hand it off to Sonia. Well, what a year. Chat GPT came out a year and a half ago. I think it's been a whirlwind for everybody here. It probably feels like just about all of us have been going nonstop with the ground shifting under our feet constantly. So let's take a pause, zoom out and take stock on what's happened so far. Last year we were talking about how AI was going to revolutionize all these different fields and provide amazing productivity gains. A year later, it's starting to come into focus. Who here is seeing this tweet from Sebastian at Clarina show of hands. It's pretty incredible. Clarina is now using open AI to handle two thirds of customer service inquiries. They've automated the equivalent of 700 full time agents jobs. We think there are tens of millions of call center agents globally. And one of the most exciting areas where we've already seen AI find product market fit is in this customer support market. Legal services. A year ago, the law was considered one of the least tech forward industries and the least likely to take risks. Now we have companies like Harvey that are automating away a lot of the work that lawyers do from day to day, grunt work and drudgery all the way to more advanced analysis. Or software engineering. I'm sure a bunch of people in this room have seen some of the demos floating around on Twitter recently. And it's remarkable that we've gone from a year ago, AI theoretically writing our code to entirely self contained AI software engineers. And I think it's really exciting. The future is going to have a lot more software. And AI isn't all about revolutionizing work. It's already increasing our quality of life.
右侧将有40或50个徽标。很可能会是这个房间里的公司的徽标。这是机会。这就是我们兴奋的原因。现在我将把话题交给索尼娅。哇,一年过去了。Chat GPT一年半前问世。我想对每个人来说都是一场旋风。大家都似乎一直在不停地前进,脚下的土地不断变化。让我们暂停一下,放大视野,回顾一下迄今发生的事情。去年,我们在谈论人工智能如何革命各个领域,带来令人惊人的生产力提升。一年后,情况开始清晰起来。这里有多少人看到Sebastian在Clarina的推文举手。这太不可思议了。Clarina现在正在使用open AI处理三分之二的客户服务查询。他们自动化了相当于700名全职代理的工作。我们认为全球有数千万名呼叫中心代理。我们已经看到AI在这个客户支持市场已经找到了产品市场契合点是最激动人心的领域之一。法律服务。一年前,法律被认为是最不向技术前进的行业之一,也是最不愿意冒险的行业之一。现在有像哈维这样的公司正在自动化律师日常工作的大部分工作,从日常琐事和苦差事到更高级的分析。或软件工程。我敢肯定这个房间里有很多人看过最近在推特上流传的一些演示。令人惊讶的是,我们从一年前,AI在理论上编写我们的代码,到完全自给自足的AI软件工程师。我想这真的很令人兴奋。未来将有更多的软件。而AI并不完全是革命性地改变工作,它已经增加了我们的生活质量。
Now the other day, I was in a zoom with Pat and I noticed that he looked a little bit suspicious. I didn't speak the entire time. And having reflected on it more, I'm pretty sure that he actually sent in his virtual AI avatar. And was actually hitting the gym, which would exploit a lot. Hi, this is Pat Grady. This is definitely me. I'm definitely here and not at the gym right now. And even gets the facial scrunch is right. This is courtesy of a gym. It's pretty amazing. This is how far technology's come in a year. It's just it's scary to think about. It's scary and exciting to think about how this all plays out in the coming decade.
前几天,我和帕特在Zoom会议上,我注意到他看起来有点可疑。我整个时间都没有说话。反思了一下,我很确定他实际上发送了他的虚拟AI化身。他其实在健身房锻炼,这点相当厉害。嗨,我是帕特·格雷迪。这绝对是我。我绝对在这里,现在不在健身房。甚至面部的皱眉也是正确的。这都归功于健身房。这真是太神奇了。科技在一年内取得了长足的进步。想到这点让人感到害怕。想想未来十年这一切会如何发展,既兴奋又害怕。
All getting aside, two years ago, when we thought that Jennifer AI might usher in the next great technology shift, we didn't know what to expect. Would real companies come out of it? Would real revenue materialize? I think the sheer scale of user poll and revenue momentum has surprised just about everybody. Generative AI, we think, is now clocking in around $3 billion of revenues in aggregate. And that's before you count all the incremental revenue generated by the FANG companies and the cloud providers in AI. So put $3 billion in context. It took the SAS market nearly a decade to reach that level of revenue. Generative AI got there its first year out the gate. So the rate and the magnitude of the C change make it very clear to us that generative AI is here to stay. And the customer poll in AI isn't restricted to one or two apps. It's everywhere.
抛开一切,两年前,当我们认为Jennifer AI可能会引领下一个重大技术转变时,我们不知道会发生什么。真正的公司会出现吗?真正的收入会实现吗?我认为用户调查和收入动力的规模之巨使几乎所有人都感到惊讶。我们认为,生成式人工智能现在总共达到了约30亿美元的收入。而且,在计算所有FANG公司和云服务提供商在人工智能领域产生的额外收入之前。所以将30亿美元放在适当的背景下。SAS市场花了将近十年的时间才达到这个收入水平。生成式人工智能在第一年就达到了这个水平。因此,变革的速度和规模非常清楚地表明,生成式人工智能将会持续存在下去。人工智能的客户调查并不局限于一两个应用程序,而是无处不在。
I'm sure everyone's aware of how many users chat GPT has. But when you look at the revenue and the usage numbers for a lot of AI apps, both consumer companies and enterprise companies, startups and incumbents. Many AI products are actually striking a chord with customers and starting to find product market fit across industries. And so we find the diversity of use cases that are starting to hit really exciting. The number one thing that has surprised me at least about the funding environment over the last year has been how uneven the share of funding has been. If you think of generative AI as a layer cake where you have foundation models on the bottom, you have developer tools and info above, and then you have applications on top. A year ago, we had expected that there would be a Cambrian explosion in the application layer due to the new enabling technology in the foundation layer. Instead, we've actually found that new company formation and capital has formed in an inverse pattern. More and more foundation models are popping up and raising very large funding rounds, while the application layer feels like it is just getting going. Our partner David is right here and posed a thought provoking question last year with his article, AI's $200 billion question.
我相信大家都意识到聊天GPT有多少用户。但是当你看到许多人工智能应用的收入和使用量时,无论是消费者公司还是企业公司,创业公司和老牌公司,许多人工智能产品实际上都在与客户建立联系,并开始在各个行业找到产品市场契合点。因此,我们发现了开始出现的各种用例的多样性非常令人兴奋。至少在过去一年里,让我感到惊讶的是资金环境的不均衡。如果您将生成式人工智能看作是一个分层蛋糕,底部是基础模型,中间是开发者工具和信息,顶部是应用程序。一年前,我们预计会有由基础层新的启用技术导致的应用层的堆积现象。但实际上,我们发现新的公司成立和资本形成的方式正好相反。越来越多的基础模型涌现出来,筹集了大量资金,而应用层则感觉刚刚开始。我们的合作伙伴大卫就在场,去年他在一篇文章中提出了发人深省的问题,《人工智能的2000亿美元问题》。
If you look at the amount that that the amount of money that companies are pouring into GPUs right now, we spent about $50 billion on NVIDIA GPUs just last year. And everybody's assuming if you build it, they will come. AI is a field of dreams. But so far, remember on the previous slide, we've identified about $3 billion or so of AI revenue, plus change from the cloud providers. We've put $50 billion into the ground, plus energy, plus data center costs and more. We've gotten $3 out. And to me, that means the math isn't mapping yet. The amount of money it takes to build this stuff has vastly exceeded the amount of money coming out so far. So we got some real problems to fix still. And even though the revenue and the user numbers in AI look incredible, the usage data says that we're still really early.
如果你看看公司目前投入到GPU的数量,我们光去年仅在NVIDIA的GPU上就花费了大约500亿美元。每个人都假设如果你建造它,他们就会来。人工智能是一个梦想之地。但到目前为止,我们只从云服务提供商身上识别出大约30亿美元左右的人工智能收入。我们投入了500亿美元,还包括能源、数据中心成本等等。但我们只得到了3亿美元。对我来说,这意味着数学还没有达到预期。建造这些东西所需的资金远远超过迄今为止产生的收入。所以我们仍然有一些严重问题要解决。尽管人工智能的收入和用户数量看起来令人难以置信,但使用数据表明我们依然处于早期阶段。
And so if you look at, for example, the ratio of daily to monthly active users, or if you look at one month retention, generative AI apps are still falling far short of their mobile peers. To me, that is both a problem and an opportunity. It's an opportunity because AI right now is a once a week, once a month kind of tinkery phenomenon for the most part for people, but we have the opportunity to use AI to create apps that people use every single day of their lives. When we interview users, one of the biggest reasons they don't stick on AI apps is the gap between expectations and reality. So that magical Twitter demo becomes a disappointment when you see that the model just isn't smart enough to reliably do the thing that you asked it to do. The good thing is with that $50 billion plus of GPU spend last year, we now have smarter and smarter base models to build on. And just in the last month, we've seen Sora, we've seen Cloud 3, we saw Grok over the weekend. And so as the level of intelligence of the baseline rises, we should expect AI's product market fit to accelerate.
因此,如果你看一下,例如,每日活跃用户与每月活跃用户之间的比率,或者看看一个月的用户保留率,生成式人工智能应用仍然远远落后于移动应用程序。对我来说,这既是一个问题,也是一个机会。这是一个机会,因为现在人工智能对大多数人来说仍然是一种每周一次、每月一次的玩乐现象,但我们有机会利用人工智能创造人们每天都会使用的应用程序。当我们采访用户时,他们不坚持使用人工智能应用的最大原因之一是期望与现实之间的差距。那些神奇的Twitter演示在你发现模型并不足够聪明以可靠地完成所要求的任务时,就会成为一种失望。好消息是,去年超过500亿美元的GPU支出为我们提供了越来越聪明的基本模型来构建。在过去一个月里,我们看到了Sora,我们看到了Cloud 3,周末我们看到了Grok。因此,随着基线智能水平的提高,我们应该期待人工智能的产品市场适应性加快发展。
So unlike in some markets where the future of the market is very unclear, the good thing about AI is you can draw a very clear line to how those apps will get predictably better and better. Let's remember that success takes time. We said this at last year's AI ascent and we'll say it again. If you look at the iPhone, some of the first apps in the V1 of the App Store were the beer drinking app or the lightsaber app or the flip cup app or the flashlight kind of the fun lightweight demonstrations of new technology.
所以,与一些市场未来不明朗的情况不同,人工智能的好处在于你可以清晰地看到这些应用将如何变得越来越好。让我们记住,成功需要时间。我们在去年的人工智能峰会上说过这句话,我们将再次重申。如果你看看iPhone,App Store V1版本中的一些最早的应用是喝啤酒的应用、光剑应用、翻杯应用或手电筒应用,这些都是有趣的轻量级展示新技术的应用。
Those eventually became either native apps, aka the flashlight etc, or utilities and gimmicks. The iPhone came out in 2007, the App Store came out in 2008. It wasn't until 2010 that you saw Instagram and DoorDash 2013. So it took time for companies to discover and harness the net new capabilities of the iPhone in creative ways that we couldn't just imagine yet. We think the same thing is playing out in AI. We think we're already seeing a peek into what some of those next legendary companies might be.
这些最终要么成为原生应用,比如手电筒等,要么成为实用程序和花哨小工具。iPhone于2007年推出,App Store于2008年推出。直到2010年才出现Instagram和DoorDash于2013年。因此,公司花费了时间去发现和利用iPhone的全新能力,以创造出我们无法想象的创意方式。我们认为AI领域也是如此。我们认为我们已经看到了一些可能成为下一个传奇公司的先行者。
Here are a few of the ones that I captured our attention recently, but I think it's much broader than the set of use cases on this page. As I mentioned, we think customer support is one of the first handful of use cases that's really hitting product market fit in the enterprise. As I mentioned with the clarinet story, I don't think that's an exception to the rule. I think that is the rule. AI friendship has been one of the most surprising applications for many of us.
这里是最近引起我们注意的几个案例,但我认为其实远不止这一页上列出的几种用例。正如我提到的,我们认为客户支持是企业中真正适应产品市场需求的第一批用例之一。就像我提到的单簧管故事一样,我不认为那是个例外。我认为那是规律。人工智能友谊对我们许多人来说是最令人惊讶的应用之一。
I think it took a few months of thinking for us to wrap our heads around, but I think the user and the usage metrics in this category imply very strong user love. And then horizontal enterprise knowledge. We'll hear more from Glean and Dust later today. We think that enterprise knowledge is finally starting to become unlocked. So here are some predictions for what we'll see over the coming year.
我认为我们花了几个月的时间来思考,但我认为在这个领域中的用户和使用数据表明用户的热爱程度非常高。接着是横向企业知识。今天稍后我们会听到更多关于Glean和Dust的信息。我们认为企业知识终于开始解锁。所以这里有一些关于未来一年我们将会看到的预测。
Prediction number one, 2024 is the year that we see real applications take us from co-pilots that are kind of helpers on the side and suggesting to you and help you to agents that can actually take the human out of the loop entirely. AI that feels more like a co-worker than a tool. We're seeing this start to work in domains like software engineering, customer service, and we'll hear more about this topic today. I think both Andrew Ng and Harrison Chase are applying the speak on it. Prediction number two, one of the biggest knocks against LLMs is that they seem to be parroting the statistical patterns in text and aren't actually taking the time to reason and plan through the task at hand.
第一个预测,2024年是我们看到真正的应用程序将把我们从辅助驾驶员转变为可以完全取代人类的代理人的一年。人工智能更像是一个同事而不是一个工具。我们正在看到这种开始在软件工程、客户服务等领域发挥作用,今天我们将会听到更多关于这个话题的讨论。我认为安德鲁·吴和哈里森·追击都在这方面取得了进展。
第二个预测,针对大规模语言模型的最大批评是它们似乎只是在重复文本中的统计模式,并没有真正花时间去推理和计划手头的任务。
That's starting to change with a lot of new research, like inference time, compute and gameplay style, value iteration. What happens when you give the model the time to actually think through what to do? We think that this is a major research thrust for many of the foundation model companies, and we expect it to result in AI that's more capable of higher level cognitive tasks like planning and reasoning over next year. And we'll hear more about this later today from Noam Brown of OpenAI.
随着大量新的研究出现,比如推理时间、计算和游戏风格、价值迭代等,情况开始发生变化。当你给模型真正思考应该怎么做的时间时会发生什么?我们认为这是许多基础模型公司的主要研究重点,我们预计这将导致AI在明年能够更能胜任更高级别的认知任务,比如规划和推理。今天稍后我们将从OpenAI的Noam Brown那里听到更多关于这方面的信息。
Prediction number three, we are seeing an evolution from fun consumer apps or prosumer apps where you don't really care if the AI says something wrong or crazy occasionally to real enterprise applications where the stakes are really high like hospitals and defense. The good thing is that there's different tools and techniques emerging to help bring these LLMs sometimes into the five nines reliability range from RLA Jeff to prompt training to vector databases.
第三项预测是,我们正在看到从有趣的消费者应用程序或专业用户应用程序发展出一种演变,在这些应用程序中,如果人工智能偶尔说错或发狂的话,你并不在意,到真正的企业应用程序,例如医院和国防,其中风险非常高。好消息是,现在出现了不同的工具和技术,帮助将这些LLMs有时提高到五个九的可靠性范围,从RLA Jeff到主动训练再到向量数据库。
And I'm sure that's something that you guys can compare notes on later today. I think a lot of folks in this room are doing really interesting things to make LLMs more reliable and production. And finally, 2024 is the year that we expect to see a lot of AI prototypes and experiments go into production. And what happens when you do that? That means latency matters. That means cost matters. That means you care about model ownership. You care about data ownership. And it means we expect the balance of compute to begin shifting from pre training over to inference. So 2024 is a big year. There's a lot of pressure and expectations built into some of these applications as they transition into production.
我相信这是你们之后可以交流心得的问题。我认为这个房间里有很多人正在做一些非常有趣的事情,让LLMs更加可靠和生产。最后,2024年是我们预计会有许多人工智能原型和实验投入生产的一年。当你这样做的时候会发生什么?这意味着延迟很重要。这意味着成本很重要。这意味着你会关心模型所有权。你会关心数据所有权。这也意味着我们预计计算的平衡将从预训练转向推断。所以2024年是一个重要的一年。有很多压力和期望融入到一些这些应用程序中,当它们过渡到生产阶段的时候。
And it's really important that we get it right. With that, I'll transition to Constantine who will help us dream about AI over it and even longer a time horizon. Thank you, Sonia. And thank you everyone for being here today. Pat, just set up the so what? Why is it so important? Why are we all in the room? And Sonia just walked us through the what now? Where are we in the state of AI? This section is going to be about what's next.
很重要的是,我们必须做对。因此,我将转向康斯坦丁,他将帮助我们在更长远的时间跨度内憧憬人工智能。谢谢,索尼亚。也感谢大家今天的到场。帕特,说说“所以呢”的重要性是什么?我们为什么都聚在这个房间?索尼亚刚才给我们讲解了现状,我们接下来讨论的内容是下一步该做什么。
We're going to take a step back and think through what this means in the broader concept of technology and society at large. So there are many types of technology revolution. There are communication revolutions like telephony. There are transportation revolutions like the locomotive. There are productivity revolutions like the mechanization of food harvest. We believe that AI is primarily a productivity revolution. And these revolutions follow a pattern.
我们将退后一步,思考这在科技和整个社会概念中意味着什么。所以有许多类型的科技革命。有通讯革命,比如电话。有交通革命,比如火车。还有生产力革命,比如农业机械化。我们认为人工智能主要是一场生产力革命。而这些革命都遵循一种模式。
It starts with a human with a tool that transitions into a human with a machine assistant. And eventually that moves into a human with a machine network. The two predictions that we're going to talk about in this section both relate to this concept of humans working with machine networks. Let's look at a historical example. The sickle has been around as a tool for the human for over 10,000 years. The mechanical reaper, which is a human and a machine assistant was invented in 1831, a single machine system being used by a human.
它始于一个使用工具的人逐渐转变为一个有机器助手的人。最终,发展为一个人与机器网络合作的状态。在本节中,我们将讨论两个关于人与机器网络合作的预测。让我们看一个历史例子。镰刀作为人类的工具已经存在了一万多年。 1831年发明了机械收割机,这是一个人和机器助手,是由人类使用的单一机械系统。
Today, we live in an era where we have a combine harvester. A combine harvester is tens of thousands of machine systems working together as a complex network. We're starting to use language in AI to describe this. Language like individual machine participants in the system might be called an agent. We're talking about this quite a bit today. The way that the apology and the way that the information is transferred between these agents, we're starting to talk about as reasoning, for example.
今天,我们生活在一个拥有联合收割机的时代。联合收割机是数万台机器系统作为一个复杂网络共同工作的产物。我们开始使用人工智能中的语言来描述这一过程。像系统中各个机器参与者可能被称为代理人这样的语言。我们今天正在大谈关于这一点。关于道歉的方式以及信息在这些代理人之间传递的方式,我们开始称为理性推理,例如。
In essence, we're creating very complicated layers of abstraction above the primitives of AI. I'll talk about two examples today, two examples that we're experiencing right in front of us in knowledge work. The first is software. So software started off as a very manual process. Here's Ada Lovelace who wrote logical programming with pen and paper and was able to do these computations, but without the assistant of a machine.
实质上,我们正在对人工智能的基本原理创建非常复杂的抽象层。我今天将谈论两个例子,这两个例子正发生在我们面前的知识工作中。第一个例子是软件。软件最初是一个非常手动的过程。这里有艾达·洛夫莱斯,她用纸和笔写了逻辑编程,并能够进行这些计算,但没有机器的帮助。
We've been living in an era where we have significant machine assistance for computation, not just the computer, but the integrated development environment and increasingly more and more technologies to accelerate development of software. We're entering a new era in which these systems are working together in a complex machine network. What you see is a series of processes that are working together in order to produce complex engineering systems. And what you would see here is agents working together to produce code, not one at a time, but actually in unison in harmony.
我们生活在一个时代,我们有重要的机器辅助进行计算,不仅仅是计算机,还有集成开发环境和越来越多的技术来加速软件开发。我们正在进入一个新的时代,在这个时代,这些系统正在联合工作在一个复杂的机器网络中。你所看到的是一系列的过程共同工作,以便产生复杂的工程系统。在这里,你会看到代理人共同合作编写代码,不是一个个地完成,而是实际上齐心协力共同完成。
The same pattern is being applied in writing very commonly. Writing was a human process, human in a tool. Over time, this is progressed to human in a machine assistant. And now we have a human that's actually leveraging not one, but a network of assistants. I'll tell you in my own personal workflow. Now, anytime I call an AI assistant, I'm not just calling GPD for calling me strong, large, I'm calling cloud three, I'm having them work together and also against each other to have better answers. This is the future that we're seeing right in front of us.
同样的模式在写作中被广泛应用。写作是一个人类的过程,在一个工具中展现人类。随着时间的推移,这进化为在机器助手中展现人类。现在,我们有一个人类实际上正在利用不止一个,而是一个助手网络。我会告诉你我的个人工作流程。现在,每当我呼叫一个人工智能助手时,我不只是呼叫GPD或称我强大、大型,我呼叫云三,我让它们一起合作,也相互对抗,以得到更好的答案。这就是我们眼前所看到的未来。
So what, what does this type of revolution mean for everyone in this room and frankly everyone outside of this room? In cold, hard economic terms, what this means is significant cost reduction. So this chart is the number of workers needed at an S&P 500 company to generate one million of revenue. It's going down rapidly. We're entering an era where this will continue to decline. What does that mean? Faster and fewer. The good news is it's not so that we can do less. It's so that we can do more and we'll get to that in the next set of predictions.
那么,对于在场的每个人以及事实上离开房间外的每个人来说,这种革命意味着什么?从冷酷的经济角度来看,这意味着显著降低成本。这个图表显示了需要多少员工才能在一个标准普尔500家公司创造100万美元的收入。这个数字正在迅速下降。我们正在进入一个这种趋势将继续下降的时代。这意味着什么?更快速,更少人。好消息是这并不意味着我们可以做得更少。而是让我们可以做更多事情,我们将在下一组预测中看到这一点。
Also fortunate is all the areas where we've had this type of progress in the past have been deflationary. I'll call out computer software and accessories. The process of computer software because we're constantly building on each other has actually gone down in cost over time. Uh, televisions are also here, but some of the most important things to our society. Education, college tuition, medical care, housing, they've gone up far faster than inflation.
我们在过去取得这种进步的所有领域都是幸运的,因为它们是通货紭的。我将举例计算机软件和配件。由于我们不断地进行技术升级,计算机软件的成本实际上是在不断降低的。嗯,电视也在这里,但对我们社会来说最重要的是教育、大学学费、医疗保健和住房,它们的涨幅远远超过了通货膨胀。
And it's perhaps a very happy coincidence that artificial intelligence is poised to help drive down costs in these and many other crucial areas. So that's the first conclusion about the long term future of artificial intelligence as a massive cost driver, a productivity revolution that's going to be able to help us do more with less in some of the most critical areas of our society. The second is related to what is it really doing?
也许非常幸运的是,人工智能有望帮助降低这些以及许多其他关键领域的成本。因此,这是关于人工智能长期未来作为巨大成本驱动因素的第一个结论,一场能够帮助我们在社会最关键领域用更少实现更多的生产力革命。第二个结论与它究竟真正做了什么有关。
One year ago on the stage, we had Jensen Hwang make a powerful prediction. He said that in the future pixels are not going to be rendered. They're going to be generated. Any given image, even information will be generated. What did he mean by this? Well, as everyone in this room knows, historically images have been stored as wrote memory. So let's think about the letter A ASCII character number 97. Okay, that is stored as a matrix of pixels, either the presence or absence if we use a very simple black and white presence or absence of those pixels. Well, we're entering a period in which we already are representing concepts, like the letter A, not as wrote storage, not as a presence or absence of pixels, but as a concept. A multidimensional point, I mean, the image to think about here is the concept of an A, which is generalizable to any given format for that letter A. So many different typefaces in this multidimensional space. We're sitting at the center. And where do we go from here? Well, the powerful thing is the computers are now starting to understand not just this multidimensional point, not just how to take it and render it and generate that image like Jensen was talking about. We are now at the point where we're going to be able to contextualize that understanding. The computer is going to understand the A, be able to render it, understand it's an alphabet, understand it's an English alphabet, and understand what that means in the broader context of this rendering. Computers going to look at the word multidimensional and not even think about the A, but rather understand the full context of why that's being bought up. And amazingly, this future is how we think, how humans think no longer are we going to be storing the rote pixels in a computer memory. That's not how we think.
一年前在舞台上,我们有黄正源(Jensen Hwang)做了一个强有力的预测。他说未来像素不会被渲染,而是会被生成。任何给定的图像,甚至信息都会被生成。他是什么意思呢?嗯,正如在座的每个人都知道的,历史上图像是以写入内存的形式存储的。那么,让我们思考一下ASCII字符编号为97的字母A。好的,它被存储为一个像素矩阵,要么存在,要么不存在,如果我们使用一个非常简单的黑白存在或不存在那些像素。嗯,我们正在进入一个时代,在这个时代中,我们已经开始将概念,比如字母A,不再作为简单的存储,不再以像素的存在或缺失来表示,而是作为一个概念。多维点,我是说,在这里要考虑的图像是字母A的概念,这个概念适用于任何给定的字母A的格式。在这个多维空间中有许多不同的字体。我们就坐在中心。那我们从这里该怎么办呢?嗯,强大的事情在于计算机现在开始理解不仅仅是这个多维点,不仅仅是如何将其渲染和生成像黄正源所说的那样的图像。我们现在正处在一个可以对这种理解进行情境化的阶段。计算机将理解这个A,能够渲染它,理解它是一个字母表,理解它是一个英文字母表,并理解这在更广泛的渲染背景中意味着什么。计算机将查看“多维度”这个词组,甚至不会考虑A,而是理解为什么这个词被提出的整个背景。令人惊奇的是,这个未来就是我们所思考的方式,就是人类所思考的方式,我们不再会在计算机内存中存储那些死记硬背的像素。这不是我们的思考方式。
I wasn't taught about the letter A as the presence or absence of a pixel on a page. Instead, we're going to be thinking about that as a concept powerfully. This is how we thought about it philosophically for thousands of years. Here's my fellow Greek Plato, 2500 years ago, who said this idea of a platonic form is what we all ascribe to are all striving for that you have this concept in this case of a letter A or this concept of software engineering that we actually are able to build a model around. So what now we've talked about the second pattern this idea that we're going to have generalization into inside computing itself. What does that mean for each of us? Well, it's going to mean a lot for company building.
我并没有把字母A教导为一页纸上像素的有无。相反,我们会将其看作一个强大的概念。这就是我们几千年来在哲学上思考它的方式。这里有我的希腊同伴柏拉图,他在2500年前说过,这个柏拉图形式的概念,我们都认同并且都在努力追求的,就像字母A的概念或者软件工程的概念,我们实际上能够围绕它建立一个模型。那么现在我们已经讨论了第二种模式,这个概念是我们将在计算机科学中推广的。这对我们每个人意味着什么呢?那将对公司的建设意味着很多。
Today, we're already integrating this into specific processes and KPIs. Sonia just mentioned how Clark is using this in order to accelerate their KPIs around customer support. They know that they have certain KPIs that they can drive towards and they can have a system that's actually retrieving information generating great customer experiences. Tomorrow, and this is already happening alongside new user interfaces. That might be a different interface for how the support is actually being communicated. And this is what I'm personally incredibly excited about is because of this future in which concepts are rendered because of this future in which everything is generated. Eventually, the entire company might start working like a neural network.
今天,我们已经将这一点整合到具体的流程和关键绩效指标中。索尼娅刚刚提到了克拉克如何利用这一点来加快客户支持方面的关键绩效指标。他们知道他们有一些关键绩效指标可以努力实现,他们可以有一个系统实际上检索信息,产生出色的客户体验。明天,这已经和新用户界面并行发生。可能会有一个不同的界面来实际传达支持信息。我个人非常激动的是,因为这个未来,概念的展现是因为这个未来,一切都是生成的。最终,整个公司可能会像一个神经网络一样运作。
Let me break that down in a specific example. This is a caricature. As with everything in this presentation, it's in reality, everything is continuous. These are all discrete. This is a caricature of the customer support process. You have customer service that has certain KPIs. These are driven by text-to-voice, language generation, customer personalization, and the like. This feeds into sub patterns, sub-trees that you're optimizing. And eventually, you're actually going to have a fully connected graph here. You're actually going to have feedback from the language generation to the end KPI for the servicing of the customers. This is going to be at some point a layer of abstraction where customer support is managed, optimized, and improved by the neural network.
让我用一个具体的例子来解释。这是一个漫画。就像这次演示中的一切一样,实际上,一切都是连续的。这些都是不连续的。这是关于客户支持流程的漫画。你有一些客户服务的关键绩效指标。这些指标受到文本转语音、语言生成、客户个性化等因素的驱动。这会反馈到你正在优化的子模式、子树中。最终,你实际上将在这里拥有一个完全连接的图。实际上,你会从语言生成得到反馈,用于客户服务的最终关键绩效指标。在某个时候,这将是一个抽象层,客户支持会被神经网络管理、优化和改进。
Now, let's think about unique customers, another part of the important job of building a business. Well, again, you have primitives of artificial intelligence from language generation to a growth engine to add customization and optimization. This will all feed into each other once again. The powerful conclusion here is eventually these layers of abstraction will become interoperable to the point where the entire company is able to function like a neural network. Here comes the rise of the one-person company. The one-person company is going to enable us not to do less, but to do more. More problems can be tackled by more people to create a better society.
现在,让我们考虑独特客户,这是建立业务的重要工作的另一个部分。同样,您可以从语言生成到增长引擎再到添加定制和优化的人工智能基本功能。所有这些将再次相互衔接。这里的强大结论是,最终这些抽象层将变得互操作,使整个公司能够像神经网络一样运作。一人公司的崛起就在眼前。一人公司将使我们能够做更多而不是更少。更多的人可以解决更多的问题,创造一个更美好的社会。
So, what's next? The reality is the people in the room here are going to decide what's next. You are the ones who are building this future. We personally are very excited about the future because we think that AI is positioned to help drive down costs and increase productivity in some of the most crucial things that we're doing. We think that we're going to be able to do more of the most crucial areas in our society, better education, healthier populations, more productive populations. And that's the purpose of convening this group today. You all are going to be able to talk about how are we able to take our technologies, abstract away complexity, mundane details, and actually build something that's much more powerful for the future.
那么,接下来呢?实际上,这里的人们将决定接下来的事情。你们是正在打造未来的人。我们个人对未来感到非常兴奋,因为我们认为人工智能可以帮助降低成本,提高生产效率,从而推动我们正在做的一些最关键的事情。我们认为我们将能够在社会中更好地实现教育、健康人口和更具生产力的人口等最关键领域。这就是今天召开这个小组会议的目的。你们将能够讨论如何利用技术,解决复杂性和枯燥细节,并实际上为未来建立更强大的东西。
I'll hand it off to Sonia to introduce our first speaker. Thank you.
我将把话筒交给索尼娅来介绍我们的第一位发言人。谢谢。