Keynote by NVIDIA CEO Jensen Huang at 2024 SIEPR Economic Summit - YouTube
发布时间 2024-03-07 07:32:51 来源
中英文字稿 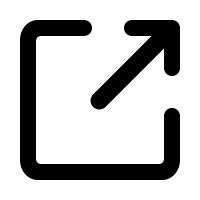
Welcome back everyone after the short break. I know that many of you are looking forward to hearing from our next speaker, Jensen Wong. Jensen is at the cutting edge of artificial intelligence and all of the innovation, technology, and human capital that is needed to support it. My good friend and Seeper colleague, John Chauvin, is going to introduce Jensen, and I hope he's here somewhere, so I'm just going to keep talking. And then the two of them will have a conversation before taking some of your questions. John Chauvin certainly requires very little introduction to many most in this crowd. As my predecessor, as the Trioni Director of Seeper, John is the one who started the Seeper Economic Summit 20 years ago.
大家在短暂休息后欢迎回来。我知道你们很多人都期待听到我们的下一个演讲者Jensen Wong。Jensen 处于人工智能的最前沿,需要创新、技术和人力资本的支持。我的好朋友兼Seeper同事John Chauvin将介绍Jensen,希望他还在现场,所以我继续说下去。然后两人将展开对话,接着回答一些你们的问题。对于在座的大多数人来说,John Chauvin几乎无需介绍。作为我在Seeper的前任,John是20年前发起Seeper经济峰会的人。
So I would just like right now for all of us to give John Chauvin a huge round of applause and appreciate the community. APPLAUSE That he had the foresight to build. For those of you who haven't been touched by John's research, his mentorship, or his friendship, here's what, here's just a snippet of what you might like to know about him. Along with being the former Seeper Director and a Seeper Senior Fellow Emeritus, John is the Charles R. Schwab Professor of Economics. He is also a Senior Fellow at the Hoover Institution and a Research Associate of the National Bureau of Economic Research.
所以我想现在让我们大家都为约翰·肖万鼓掌并感谢这个社群。掌声!他有先见之明去建立这个社群。如果你们还没有受到约翰的研究、指导或友谊的影响,这里只是你们可能想要了解的一点。作为前Seeper主任和Emeritus高级研究员,约翰是查尔斯·R·施瓦布经济学教授。他还是胡佛研究所的高级研究员和国家经济研究局的研究合作人。
He specializes in public finance and corporate finance and has published many articles over the years on social security, health economics, corporate and personal taxation, mutual funds, pension plans, economic demography, applied general equilibrium economics, and much more. John isn't one for long introductions, but I just will say that if I can be one-tenth as helpful to my successor as John has been to me, I'll feel like I've succeeded. So I will let you read more about his publications and accomplishments in the programs you've received today.
他专攻公共财政和公司财务,在多年来已发表了许多关于社保、健康经济、公司和个人税收、共同基金、养老金计划、经济人口统计学、应用一般均衡经济学等方面的文章。约翰不善长篇大论,但我只想说,如果我能对我的继任者有十分之一像约翰对我那样有帮助,我会感到成功。所以,我会让你们在今天收到的节目单里更多地了解他的文章和成就。
And so please join me in welcoming our good friend, John Chauvin, and I'm really looking forward to this. APPLAUSE Wow, thank you. So I have always thought that the more famous the speaker, the shorter the appropriate introduction. And if I were to follow that rule, I would stop right now and say Jensen Wong, but I'm not going to do that. So the Oxford English Dictionary defines the American dream. Believe it or not, it does that. And it says that it's a situation where everybody has an equal opportunity for success through hard work, dedication and initiative. And I would like to say that Jensen Wong is an example of the American dream.
请大家一起欢迎我们的好朋友约翰·肖文,我真的很期待这次。掌声响起,谢谢。所以我一直认为,演讲者越有名,适当的介绍就应该越短。如果按照这个规则,我现在就可以说“詹森·王”,但我不会这么做。牛津英语词典定义了美国梦。信不信由你,它确实这么做了。它说,这是一种每个人都有通过努力、奉献和主动性实现成功的机会的情况。我想说,詹森·王就是美国梦的一个例子。
Jensen was born in Taiwan, came to the U.S. at age nine with his brother, not with his parents, went to a rough, tough school in Kentucky, survived that. His parents came two years later, removed to Oregon, skipped two grades and graduated from high school, and went to Oregon State, electrical engineering major, 150 men and two women. He said he was 16, he looked like he was 12, he had no chance with the women. LAUGHTER Well, he sort of liked one of them and said, why don't we work on homework together? And did that over and over and over again? Six months later, he asked for a date. Well, he's still married to her. So another American dream. APPLAUSE Now to skip to age 30, he co-founds NVIDIA. He's the only CEO there's ever been, of NVIDIA. It's had its ups and it's down, more ups than downs. It's now the fourth largest company in the world.
Jensen 是在台湾出生的,在九岁时和他的兄弟来到美国,而不是和父母一起。他在肯塔基州上了一所粗放、艰难的学校,成功地度过了那段时期。他的父母两年后也来到了美国,搬到了俄勒冈州,跳了两个年级,高中毕业后进入了俄勒冈州立大学主修电气工程,班上有150名男生和两名女生。他说他16岁,看起来像12岁,和女生交往的机会几乎为零。大家笑了。不过,他对其中一位女生有好感,就说为什么不一起做作业?然后一遍又一遍地一起做作业,六个月后,约了一次会。现在他们依然相亲相爱。又是一个美国梦。掌声。再跳到30岁,他共同创办了 NVIDIA。他是NVIDIA迄今为止唯一的CEO。公司起起伏伏,但升势大于衰势,现在是世界第四大公司。
Third largest American company. So that sounds to me like the American dream. I should add that he also got a degree from Stanford, master's degree. I think he did it mostly at night. And he was always good with homework, it worked with his wife at work with Stanford too. Now, of course, we were here last week. NVIDIA announced its earnings. In the finance crowd, this got more attention than the Super Bowl. It occurred a couple of weeks earlier. It was pretty amazing. His company is at the absolute center of the most exciting development, I'd say, of the 21st century, technology development. And so he's to be congratulated on that. Let me just say he's received a lot of awards, a lot of recognition, and NVIDIA's received a lot of awards, a lot of recognition. But I should have a short introduction.
美国第三大公司。这听起来对我来说就像是美国梦。我还应该补充一点,他还在斯坦福获得了硕士学位。我想他大部分是在晚上读书。他总是能很好地完成作业,跟他的妻子在斯坦福工作也很顺利。现在,当然,我们上周在这里。英伟达宣布了其收益。在金融圈,这比超级碗更受关注。这发生在几周之前。这真的很不可思议。他的公司处于21世纪最令人兴奋的发展的绝对中心,我会这么说,技术发展。因此,他值得被祝贺。我只想说他获得了很多奖项,得到了很多认可,英伟达也获得了很多奖项,得到了很多认可。但是我应该简短介绍一下。
So I'm about to quit. I'm just going to talk about one award. Last month, he was elected as a member of the National Academy of Engineering. This is a pretty prestigious award. There are only three that I know of. I asked the S-Chat GPT, I didn't get an absolute clear answer. How many CEOs of S&P 500 companies are members of the National Academy of Engineering? But I think it's three. And two are in this room. Andrew Devgen of Cadence Design Systems was awarded it last year. So the two of them have that in common. But let me now just conclude and congratulate Jensen, not only on this award, but on the amazing success of your company. And thank you for speaking to us today at Seaport. Jensen. Thank you. You're here, I'm here. I guess so. Okay.
所以我快要离开了。我只想谈论一个奖项。上个月,他被选为美国国家工程院的院士。这是一个非常有声望的奖项。据我所知,只有三个人获得了这个奖项。我问了 S-Chat GPT,没有得到一个绝对清晰的答案。S&P 500 公司的CEO中有多少人是美国国家工程院的院士?我想是三个。而这个房间里就有两个。Cadence Design Systems 的 Andrew Devgen 去年获得了这个奖项。所以两人有共同之处。不过现在让我来总结并祝贺 Jensen,不仅是获得这个奖项,而且是因为你的公司取得了惊人的成功。感谢你今天在 Seaport 与我们分享。Jensen,谢谢你。你在这儿,我在这儿。我想是这样。好的。
So why don't you start off with maybe some opening remarks, and then I'll ask you a few questions, and then you get the tough questions. Well, I think that after your opening remarks, it is smartest for me not to make any opening remarks to avoid risking damaging all the good things you set. But let's see. It's always good to have a pickup line. And mine was, do you want to see my homework? And you're right, we're married still. We have two beautiful kids. We have a perfect life, two great puppies, and I love my job. And she still enjoys my homework. Well, if you want, I can ask you a few questions then. Yes, please. So in my lifetime, I thought the biggest technical development technology breakthrough was the transistor. Now, I'm older than you. And it was pretty fundamental. Should I rethink his AI now, the biggest change of technology that has occurred in the last 76 years to hint at my age? Yeah. Well, first of all, the transistor was obviously a great invention. But what was the greatest capability that enabled was software. The ability for humans to express our ideas, algorithms, in a repeatable way, computationally repeatable way, is the breakthrough.
那么你为什么不先说一些开场白,然后我再问你几个问题,接着你再回答一些困难的问题。我觉得在你说完开场白后,最明智的做法就是让我不说任何开场白,避免破坏你已经说的所有好话。但让我们看看。总是好有一句搭讪的话。我的是,“你想看我的作业吗?”你说得对,我们仍然是夫妻。我们有两个可爱的孩子,完美的生活,两只很棒的小狗,我热爱我的工作。她仍然喜欢看我的作业。如果你愿意,我可以问你一些问题。是的,请问吧。在我的一生中,我认为最大的技术发展突破是晶体管。现在,我比你年长。这是相当基础的。现在,我应该重新思考人工智能吗?在过去的76年里发生了最大的技术变革,来暗示我的年龄?是的。首先,晶体管显然是一个伟大的发明。但它最大的能力是启用了软件。人类能够以可重复计算的方式表达我们的想法、算法,这是突破所在。
What have we done? We dedicated our company, in the last 31 years, to a new form of computing called accelerated computing. The idea is that general purpose computing is not ideal for every field of work. We said why don't we invent a new way of doing computation such that we can solve problems that general purpose computing is ill-equipped at solving. What we have effectively done in a particular area of domain of computation that is algorithmic in nature that can be paralyzed, we've taken the computational cost of computers to approximately zero. What happens when you are able to take the marginal cost of something to approximately zero? We enabled a new way of doing software where it used to be written by humans. We now can use computers to write the software because the computational cost is approximately zero.
在过去的31年里,我们致力于一种叫做加速计算的新型计算方式。这个理念是通用计算并不适用于所有领域的工作。我们想为什么不发明一种新的计算方式,这样我们就能解决通用计算无法解决的问题。我们在某个特定的算法性质的计算领域中取得了实质性的进展,将计算机的成本降低到了接近零。当你能将某物的边际成本降低到接近零时会发生什么呢?我们实现了一种全新的软件编写方式,不再需要人类来编写软件,因为计算成本已经接近零。
You might as well let the computer go off and grind on just a massive amount of experience, we call data, digital experience, human digital experience, and grind on it to find the relationships and patterns that as a result represents human knowledge. That miracle happened about a decade and a half ago. We saw it coming and we took the whole company and we shaped our computer which was already driving the marginal cost of computing down to zero. We pushed it into this whole domain and as a result in the last ten years we reduced the cost of computing by one million times. The cost of deep learning by one million times. A lot of people said to me, but Jensen, if you reduced the cost of computing your cost by a million times and people buy less of it, it's exactly the opposite. We saw that if we could reduce the marginal cost of computing down to approximately zero, we might use it to do something insanely amazing.
你可以让计算机处理大量经验,我们称之为数据、数字经验、人类数字经验,然后分析这些数据,寻找其中的关联和模式,从而代表人类知识。这个奇迹大约发生在十五年前。我们看到了未来的发展趋势,就把整个公司的方向转向了这个领域,将计算成本降低到零。在过去的十年里,我们成功地将计算成本降低了一百万倍,也将深度学习成本降低了一百万倍。很多人问我,但是Jensen,如果你将计算成本降低了一百万倍,人们购买的计算机数量会减少吧?其实恰恰相反。我们发现,如果能将计算成本降低到接近零,我们可以做一些非常惊人的事情。
Large language models. To literally extract all of digital human knowledge from the internet and put it into a computer and let it go figure out what the knowledge is, that idea of scraping the entire internet and putting it in one computer and let the computer figure out what the program is is an insane concept. But you wouldn't ever consider doing it unless the marginal cost of computing was zero. So we made that breakthrough and now we've enabled this new way of doing software. Imagine for all the people that are still new to artificial intelligence, we figured out how to use a computer to understand the meaning, not the pattern, but the meaning of almost all digital knowledge and everything you can digitize, we can understand the meaning. So let me give you an example.
大型语言模型。从字面上说,从互联网中提取全部的数字人类知识,放入一台计算机中,让它自己去理解知识,这种将整个互联网数据抓取到一台计算机中,让计算机自己去理解的想法是一个疯狂的概念。但除非计算的边际成本为零,否则你绝对不会考虑这样做。因此,我们取得了这一突破,现在我们已经开启了这种新的软件方式。想象一下,对于所有还在初学人工智能的人们,我们找到了一种方法,通过计算机理解几乎所有数字知识的意义,而不只是模式,而是意义。一切你能够数字化的东西,我们都可以理解其意义。让我举个例子。
Gene sequencing is digitizing genes. But now with large language models, we can go learn the meaning of that gene. Amino acids, we digitized through mass spec, we digitized amino acids. Now we can understand from the amino acid sequence without a whole lot of work with cryo-e-m's and things like that, we can go figure out what is the structure of the protein and what it does. What does this mean? We can also do that on a fairly large scale pretty soon. We can understand what's the meaning of a cell. A whole bunch of genes that are connected together. And this is from a computer's perspective, no different than there's a whole page of words and you asked it to what is the meaning of it. Summarize, what did it say? Summarize it for me. What's the meaning? This is no different than a big, huge, long page of genes. What's the meaning of that? Big, long page of proteins, what's the meaning of that? And so we're on the cusp of all this. This is the miracle of what happened.
基因测序是数字化基因。但现在有了大型语言模型,我们可以去学习那个基因的含义。氨基酸,我们通过质谱仪数字化了,我们数字化了氨基酸。现在我们可以从氨基酸序列中理解,而不需要大量使用低温电子显微镜等设备,我们可以弄清楚蛋白质的结构和功能。这意味着什么?我们也可以很快在相当大的范围内做到这一点。我们可以理解一个细胞的含义。一大堆连接在一起的基因。从计算机的角度来看,这与一页满满的字母没有什么区别,你问它意思是什么。总结一下,它说了什么?为我总结一下。意思是什么?这与一大页基因有什么不同?一大页蛋白质,意思是什么?所以我们正处于所有这一切的前沿。这就是所发生的奇迹。
And so I would, as long as the answer to St. John, you're absolutely right, that AI, which was enabled by this new form of computing, we call it accelerated computing, that took three decades to do, is probably the single greatest invention of the technology industry. This will likely be the most important thing in the 21st century. I agree with that. 21st century. But maybe not the 20th century, which was the transistor, which it's got to be closed. We'll let history decide. That's right. We'll let history decide. Could you look ahead, you, I take it that the GPU chip that is behind artificial intelligence right now is your H100, and I know you're introducing an H200. And I think I read that you plan to upgrade that each year. And so could you think ahead five years, March 2029, you're introducing the H700? What will it allow us to do that we can't do now?
我认为,正如圣约翰所说,您是绝对正确的,人工智能是由这种新型计算形式推动的,我们称之为加速计算,这花了三十年的时间发展出来,可能是技术行业中最伟大的发明。这很可能是21世纪最重要的事情。我同意这一点。但也许不是20世纪,那时是晶体管,必须结束的时候。让历史来决定。这一点是正确的。我们让历史来决定。你可以展望未来吗?您认为目前推动人工智能的GPU芯片是您的H100,我知道您正在推出H200。我想我读到您计划每年升级一次。那么,您可否向五年后的2029年3月展望,您将推出H700?它将让我们做什么事情,现在我们做不到的?
I'll go backwards, but let me first say something about the chip that John just described. As we say a chip, all of you in the audience probably, because you've seen the chip before, you imagine there's a chip kind of like this. The chip that John just described weighs 70 pounds. It consists of 35,000 parts. Eight of those parts came from TSMC. It, that one chip replaces a data center of old CPUs like this into one computer. The savings, because we compute so fast, the savings of that one computer is incredible, and yet it's the most expensive computer the world's ever seen. It's a quarter of a million dollars per chip. We sell the world's first quarter million dollar chip. But the system that it replaced, the cables alone cost more than the chip, this H100. The cables of connecting all those old computers. That's the incredible thing that we did. We reinvented computing, and as a result, computing, marginal cost of computing went to zero. That's what you just explained. We took this entire data center, we shrunk it into this one chip.
我要倒车,但是让我先说一些关于约翰刚刚描述的芯片的事情。当我们说到芯片时,你们在观众席的所有人可能会想象到这样的芯片,因为你们以前见过这种芯片。约翰刚刚描述的芯片重量为70磅,由35000个零件组成。其中8个零件来自台积电。这个芯片可以将一个旧的CPU数据中心转变为一台电脑。由于我们计算速度很快,这台电脑的节约是令人难以置信的,然而它是世界上最昂贵的电脑。每个芯片的价格是25万美元。我们销售了世界上第一款25万美元的芯片。但它所取代的系统,单单连接所有那些旧电脑的电缆就比芯片还要贵,这就是H100。连接所有那些旧电脑的电缆费用更高。这就是我们所做的令人难以置信的事情。我们重新定义了计算,结果计算的边际成本变为零。这就是你刚刚解释的事情。我们将整个数据中心缩小至这一芯片中。
This one chip is really, really great at trying to figure out this form of computation that without getting weird on you guys called deep learning. It's really good at this thing called AI. The way that this chip works, it works not just at the chip level, but at the chip level and the algorithm level and the data center level. It works together. It doesn't do all of its work by itself. It works as a team. You connect a whole bunch of these things together, and networking is part of it. When you look at one of our computers, it's a magnificent thing. Only computer engineers would think it's magnificent, but it's magnificent. It weighs a lot, miles and miles and cables, hundreds of miles and cables. The next one is soon coming. It's beautiful in a lot of ways. It computes at data center scales. Together, what's going to happen in the next ten years, say John, will increase the computational capability for deep learning by another million times. What happens when you do that? What happens when you do that? We learn and then we apply it. We go train inference. We learn and we apply it. In the future, we'll have continuous learning. We could decide whether that continuous learning result will be deployed into the world's applications or not, but the computer will watch videos and new text and from all the interactions that it's continuously improving itself. The learning process and the training process and the inference process, the training process and the deployment process, application process will just become one. That's exactly what we do. We don't have between now and seven o'clock in the morning, I'm going to be doing my learning, and then after that I'll just be doing inference. You're learning and inferencing all the time.
这个芯片在尝试研究一种被称为深度学习的计算形式时真的非常出色。它在人工智能方面表现特别优秀。这个芯片的工作方式,不仅仅是在芯片级别,还涉及到算法级别和数据中心级别。它们共同协作,而不是独自完成所有工作。将大量这些设备连接在一起,网络也是其中的一部分。当你看到我们的电脑时,它是一件壮丽的事物。只有计算机工程师会认为它是壮丽的,但它确实很美。它重量很大,有成千上万英里的电缆,数百英里的电缆。下一个即将推出。从许多方面看,它非常精美。它在数据中心规模上进行计算。约翰说,在未来十年内,计算机的深度学习能力将再次增加百万倍。当这样做的时候会发生什么?会发生什么?我们学习然后应用。我们进行训练,推理。我们学习和应用。将来,我们将拥有持续学习。我们可以决定这种持续学习的结果是否要部署到世界的应用中,但计算机将观看视频和新文本,从所有这些互动中不断提高自己。学习过程、训练过程和推理过程,是一个训练过程和部署过程、应用过程将融为一体。这正是我们所做的。我们不是从现在到早上七点,我要学习一会儿,然后再推理。你一直在学习和推理。
That reinforcement learning loop will be continuous. That reinforcement learning will be grounded with real world data that is being through interaction as well as synthetically generated data that we're creating in real time. This computer will be imagining all the time. Does that make sense? Just as when you're learning, you take a pieces of information and you go from first principles that should work like this, and then we do the simulation, the imagination in our brain, and that future imagined state in a lot of ways manifests itself to us as reality. Your AI computer in the future will do the same. It'll do synthetic data generation, it'll do reinforcement learning, it'll continue to be grounded by real world experiences, it'll imagine some things, it'll test it with real world experience, it'll be grounded by that, and that entire loop is just one giant loop. That's what happens when you can compute for a million times cheaper than today.
强化学习循环将是连续的。这种强化学习将以实时交互产生的真实世界数据和我们实时创建的合成数据为基础。这台计算机将一直在进行想象。这有意义吗?就像当你学习时,你会获取一些信息,然后从第一原理开始,认为应该是这样,然后我们在大脑中进行模拟、想象,未来想象出的状态在很多方面会显现为我们的现实。未来的AI计算机将做同样的事情。它将进行合成数据生成,进行强化学习,将继续以真实世界经验为基础,它会想象一些东西,然后用真实世界经验进行测试,然后以此为依据,整个循环就是一个巨大的循环。当你能以比今天便宜一百万倍进行计算时,就会发生这种情况。
As I'm saying this, notice what's at the core of it. When you can drive the marginal cost of computing down to zero, then there are many new ways of doing something you're willing to do. This is no different than I'm willing to go further places because the marginal cost of transportation has gone to zero. I can fly from here to New York relatively cheaply. If it were to take in a month, you know, it'll probably never go. It's exactly the same in transportation and just about everything that we do. We're going to take the marginal cost of computing down to approximately zero as a result. We'll do a lot more computation. That causes me, as you probably know, there have been some recent stories that Nvidia will face more competition in the inference market than it has in the training market.
当我说这些话的时候,请注意其中的核心。当你能将计算的边际成本降至零时,那么你将有许多新的方式来做一些你愿意做的事情。这与我愿意前往更远的地方并没有什么不同,因为交通运输的边际成本已经降至零。我可以比较便宜地从这里飞往纽约。如果需要一个月的时间,你知道,这很可能永远不会发生。在交通运输以及我们所做的几乎所有事情中,情况完全相同。作为结果,我们将将计算的边际成本降至接近零。我们将进行更多的计算。正如你可能知道的那样,最近有一些报道称,Nvidia在推理市场上将面临比训练市场更多的竞争。
What you're saying is it's actually going to be one market, I think. Can you comment about, you know, is there going to be a separate training chip market and inference chip market, or it sounds like you're going to be continuously training and switching to inference maybe within one chip? I don't know. Why don't you explain to me? Today, whenever you prompt an AI, it could be CHAT or it could be copilot or it could be if you're using a service now platform, you're using mid-journey, using firefly from Adobe, whenever you're prompting, it's doing inference. You know, inference is a right, so it's generating information for you. Whenever you do that, what's behind it, 100% of them, is Nvidia's GPUs.
你的意思是实际上会是一个市场,我认为。你能谈谈,你知道,会有一个独立的培训芯片市场和推断芯片市场吗,还是听起来你们会不断进行培训并在一个芯片内切换到推断?我不知道。为什么你不解释给我听呢?今天,无论你启动一个AI,可能是CHAT,可能是copilot,可能是如果你正在使用服务平台,使用Adobe的firefly,无论你启动时,它都在进行推断。你知道,推断是正确的,所以它正在为你生成信息。每当你这样做时,背后使用的,其中100%是英伟达的GPU。
And so Nvidia's most of the time you engage our platforms are when you're inferencing. And so we're 100% of the world's inferencing today's Nvidia. Now, is inferencing hard or easy? A lot of people, the reason why people are picking on inferencing is when you look at training and you look at an Nvidia system doing training, when you just look at it, you go, that looks too hard. I'm not going to go do that. I'm a chip company. That doesn't look like a chip. And so there's a natural, and you have to, in order for you to even prove that something works or not, you're $2 billion into it. And you turn it on to realize it's not very effective. You're $2 billion in two years into it. The risk of exploring something new is too high for the customers. And so a lot of competitors tend to say, you know, we're not into training, we're into inference. Inference is incredibly hard. Let's think about it for a second.
因此,Nvidia大部分时间在你使用我们的平台时进行推理。因此,如今Nvidia占据世界推理的100%。那么,推理是困难还是容易的?许多人选择挑战推理的原因是当你看到训练和Nvidia系统在训练时,你就会觉得看起来太难了。我不想去做那个。我是个芯片公司,这看起来并不像是一个芯片。而且,你必须要投入20亿美元才能证明某件事是否有效。结果,你发现它并不那么有效。你已经投入20亿美元且两年的时间了。对于客户来说,探索新事物的风险太高了。因此,许多竞争对手倾向于说,你知道,我们不擅长训练,我们专门从事推理。推理是极其困难的。让我们思考一下。
The response time of inference has to be really high, but this is the easy part. That's the computer science part. The hard part of inference is the goal of somebody who's doing inference is to engage a lot more users, to apply that software to a large installed base. Inference is an installed base problem. This is no different than somebody who's writing an application on an iPhone. The reason why they do so is because iPhone has such an large installed base. Almost everyone has one. And so if you wrote an application for that phone, it's going to have the benefit of it's going to be able to benefit everybody. Well, in the case of NVIDIA, our accelerated computing platform is the only accelerated computing platform that's literally everywhere. And because we've been working on it for so long, if you wrote an application for inference and you take that model and you deploy it on NVIDIA's architecture, it literally runs everywhere. And so you can touch everybody, you can enable, have greater impact.
推断的响应时间必须非常快,但这是容易的部分。这是计算机科学的一部分。推断的困难之处在于,从事推断的人的目标是吸引更多用户,将该软件应用到庞大的安装基础上。推断是一个安装基础的问题。这与为iPhone编写应用程序的人没有什么不同。他们这样做的原因是因为iPhone拥有如此之大的安装基础。几乎每个人都有一部。因此,如果你为这款手机编写了一个应用程序,它将能够让每个人受益。嗯,在英伟达的情况下,我们的加速计算平台是唯一的加速计算平台,它几乎无处不在。由于我们已经在这方面努力了很长时间,如果你为推断编写一个应用程序,然后将该模型部署到英伟达的架构上,它几乎可以在任何地方运行。因此,你可以触及每个人,实现更大的影响。
And so the problem with inference is actually install base. And that takes enormous patience and years and years of success and dedication to architecture compatibility, you know, so on and so forth. You may completely stay to the art chips. Is it possible though that you'll face competition that is claims to be good enough? Not as good as NVIDIA, but good enough and much cheaper. Is that a threat? Well, first of all, competition, we have more competition than anyone on a planet has competition.
因此,推论的问题实际上在于基础安装。这需要巨大的耐心和多年多年的成功和对架构兼容性的投入,你知道的,诸如此类。你可能完全留在艺术芯片上。然而,你是否会面对声称足够好的竞争对手呢?虽然不如英伟达那么好,但足够好且价格更便宜。这是一种威胁吗?首先,竞争,我们拥有比地球上任何其他地方都更多的竞争。
Not only do we have competition from competitors, we have competition from our customers. And I'm the only competitor to a customer fully knowing they're about to design a chip to replace ours. And I show them not only what my current chip is, I show them what my next chip is, and I show them what my chip at Devattas. And the reason for that is because, look, if you don't make an attempt at explaining why you're good at something, they'll never get a chance to buy your products. So we're completely open-booked and working with just about everybody in the industry.
我们不仅面对竞争对手的竞争,还面对客户的竞争。我是唯一一个完全了解他们将要设计一款替代我们芯片的客户。我不仅展示了我的当前芯片,还展示了我的下一代芯片以及Devattas的芯片。这样做的原因是,如果你不尝试解释为什么你擅长某个领域,他们永远不会有机会购买你的产品。因此,我们完全开放合作,并与行业中的几乎所有人合作。
And the reason for that, our advantage is several. Our advantage, what we're about is several things. Whereas you could build a chip to be good at one particular algorithm. Remember, computing is more than even transformers. There's an idea called transformers. There's a whole bunch of species of transformers and they're new transformers being invented as we speak. And the number of different types of software is really quite rich. And the reason for that is because software engineers love to create new things, innovation. And we want that.
因此,我们的优势有几个方面。我们的优势在于几个方面。虽然您可以构建一款擅长特定算法的芯片。记住,计算不仅仅是变压器。有一个叫做变压器的概念。有各种各样的变压器品种,而且正在不断有新的变压器被发明。而不同类型的软件数量非常丰富。这是因为软件工程师喜欢创造新东西,创新。我们希望这样。
What NVIDIA is good at is that our architecture, not only does it accelerate algorithms, it's programmable, meaning that you can use it for the only accelerator for SQL. SQL came about in the 1960s, IBM, 1970s, in storage computing. I mean, SQL is a structured data as important as it gets. 300 z-bites of data being created every couple of years, most of it is in SQL's structured databases. And so we can accelerate that, we can accelerate quantum physics, we can accelerate Schonjers equations. Which is about fluids, particles, lots and lots of code.
NVIDIA擅长的是我们的架构,不仅可以加速算法,还是可编程的,这意味着你可以将其用作SQL的唯一加速器。 SQL诞生于20世纪60年代的IBM,70年代的存储计算。我的意思是,SQL是最重要的结构化数据之一。每隔几年就会产生300 z-字节的数据,其中大部分是在SQL的结构化数据库中。因此,我们可以加速这个过程,我们可以加速量子物理,我们可以加速Schonjers方程。这些方程涉及流体,粒子,以及大量的代码。
And so what NVIDIA is good at is the general field of accelerated computing. One of them is generative AI. And so for a data center that wants to have a lot of customers, some of it in financial services, some of it in manufacturing, and so on and so forth, in the world of computing we're a great standard. We're in every single cloud, we're in every single computer company. And so our company's architecture has become a standard, if you will, after some 30-some odd years. And so that's really our advantage. If a customer can do something specifically that's more cost effective, quite frankly, I'm even surprised by that.
因此,英伟达擅长的是加速计算的通用领域。其中之一是生成式人工智能。对于一个希望拥有很多客户的数据中心,有部分是金融服务领域,有部分在制造业等等,我们在计算领域是一个伟大的标准。我们在每一个云平台,每一个计算机公司都有所存在。因此,我们公司的架构在30多年的发展后已经成为了一个标准。这才是我们的优势。如果一个客户可以实现更加成本效益的特定操作,坦率地说,我甚至会感到惊讶。
And the reason for that is this. Remember, our chip is only part, think of when you see computers these days, it's not a computer like a laptop, it's a computer's a data center. And you have to operate it. And so people who buy and sell chips think about the price of chips. People who operate data centers think about the cost of operations. Our time to deployment, our performance, our utilization, our flexibility across all these different applications, in total, allows our operations cost, they call total cost of operations and our TCO.
而这其中的原因就在于这个。记住,我们的芯片只是部分,想象一下如今的电脑,它不是像笔记本电脑那样的电脑,而是一个数据中心。而且你必须操作它。所以购买和销售芯片的人会考虑芯片的价格。而操作数据中心的人会考虑运营成本。我们的部署时间、性能、利用率、在所有这些不同应用中的灵活性,总体上来说,可以降低我们的运营成本,他们称之为总运营成本和我们的TCO。
Our TCO is so good that even when the competitors' chips are free, it's not cheap enough. And that is our goal. To add so much value that the alternative is not about cost. And so of course that takes a lot of hard work and we have to keep innovating and things like that and we don't take anything for granted. But we have a lot of competitors. As you know, but maybe not everybody in the audience knows, there's this term artificial general intelligence which basically.
我们的总体成本控制(TCO)非常优秀,以至于即使竞争对手的芯片是免费的,也并不便宜。这就是我们的目标。为了增加如此多的价值,以至于选择另一种方式并不仅仅是为了成本。当然,这需要大量的辛勤工作,我们必须不断创新等等,我们绝不会掉以轻心。但我们有很多竞争对手。如你所知,但也许并非观众中的每个人都知道这个术语“人工通用智能”,基本上。
I was hoping not to sound competitive, but John asked a question that kind of triggered a competitive gene. And I want to say, I want to apologize, I came across, you know, if you will, a little competitive. I apologize for that. I could have probably done that more artfully. I will next time. He surprised me with that competitive. I thought I was in an economic forum. You know, just walking in here, I asked him, I sent some questions to his team and I said did you look at the questions, he says no, I didn't look at the questions, I wanted to be spontaneous, besides I might start thinking about it and then that would be bad.
我原本希望不要显得竞争心太重,但约翰提出了一个问题,引发了我的竞争天性。我想说,我要道歉,我显得有点竞争。对此我感到抱歉。我可能可以更巧妙地处理那个情况。下次我会注意的。他让我感到惊讶。我以为我在一个经济论坛。你知道,我刚走进来,问他,我发了一些问题给他的团队,我问他你看了那些问题吗,他说没有,我没看那些问题,我想要保持 spontaneity(自然而然),再说,我可能开始思考然后会不好。
And we're just kind of winging it here. Both of us. So I was asking when do you think. And of course, when do you think we will achieve artificial general intelligence, the sort of human level intelligence? Is that 50 years away? Is it five years away? What's your opinion? I'll give you a very specific answer. But first, let me just tell you a couple of things about what's happening, that's super exciting. First, of course, we're training these models to be multimodality, meaning that we will learn from sounds, we'll learn from words, we'll learn from vision, and we'll just watch TV and learn, so on and so forth. Okay, just like all of us. And the reason why that's so important is because we want AI to be grounded. Not just by human values, which is what chat GPT really innovated. Remember, we had large language models before, but it wasn't until reinforcement learning human feedback, that human feedback that grounds the AI to something that we feel good about, human values. And now, could you imagine, now you have to generate images and videos and things like that. Because the AI know that hands don't penetrate through podiums, that feet stand above the ground, that when you step on water, you all fall into it. So you have to ground it on physics. So now the AI has to learn by watching a lot of different examples, and ideally, mostly video, that certain properties are obeyed in the world. It has to create what is called a world model. So one, we have to understand multimodality. There's a whole bunch of other modalities, like as I mentioned before, genes and amino acids and proteins and cells, which leads to organs and so on and so forth. And so we would like to multimodality. Second is greater and greater reasoning capabilities. A lot of the things that we already do reasoning skills are encoded in common sense. Common sense is reasoning that we all kind of take for granted. So there are a lot of things in our knowledge in the internet that already encodes reasoning and models can learn that. But there's higher level reasoning capabilities.
我们在这里只是随意地尝试。我们两个人都这样。所以我在问你认为什么时候。当然,你认为我们何时会实现人类水平的人工智能,也就是通用人工智能?是50年后吗?是5年后吗?你的看法是什么?我会给你一个非常明确的答案。但首先,让我告诉你一些令人兴奋的事情。首先,当然,我们正在训练这些模型能够多模态,意味着我们会从声音中学习,从文字中学习,从视觉中学习,我们会观看电视学习等等。就像我们所有人一样。而这之所以如此重要,是因为我们希望人工智能能够接地气。不仅仅是受到人类的价值观的影响,这正是聊天GPT真正创新的地方。记住,以前我们有过大规模的语言模型,但直到强化学习和人类反馈,那才是将人工智能接地到我们感到满意的东西,也就是人类的价值观。现在,想象一下,现在你必须生成图像和视频等等。因为AI知道手不能穿过讲台,脚站在地面上,当你踩在水上时,你会掉进去。所以你必须让它接地到物理世界。现在,AI必须通过观看大量不同的例子,理想情况下主要是视频,学习到世界中存在一定的特性。它必须创建所谓的世界模型。因此,首先我们必须了解多模态。还有一大堆其他模态,比如我之前提到的基因、氨基酸、蛋白质和细胞,这些会导致器官等等。因此我们希望多模态。第二是越来越强大的推理能力。我们已经做的许多推理能力都是编码在常识中的。常识是我们都认为理所当然的推理。因此在互联网中的许多知识中已经编码了推理和模型可以学习这些。但也有更高级别的推理能力。
For example, there's some questions that you answer me right now when we're talking. I'm mostly doing generative AI. I'm not spending a whole lot of time reasoning about the question. However, there are certain problems, like for example, planning problems, where I'm going to, that's interesting. Let me think about that. And I'm cycling in the back and I'm coming up with multiple plans. I've got, I'm traversing a tree. Maybe I'm going through my graph and, you know, I'm pruning my tree and saying this doesn't make sense. But this, I'm going to put, and I simulated in my head and maybe I do some calculations and so on and so forth. That long thinking, that long thinking, AI is not good at today. Everything that you prompted to chat GPT responds instantaneously. We would like to prompt something into chat GPT, give it a mission statement, give it a problem, and for it to think a while, isn't that right? So that kind of system, what computer science calls system two thinking or long thinking or planning, those kind of things reasoning and planning, those kind of problems, I think we're working on those things. And I think that you're going to see some breakthroughs. And so in the future, the way you interact with AI will be very different. Some of it will be, just give me a question and answer. Some of it is that here's a problem. Go work on it for a while. Tell me tomorrow. And it does the largest amount of computation it can do by tomorrow. You can also say, I'm going to give you this problem. You know, spend $1,000 on it, but don't spend more than that. And it comes back with the best answer within the thousand. You know, so on and so forth. So that's, now, AGI. The question on AGI is, what's the definition? In fact, that's kind of the supreme question. Now, if you ask me, if you say Jensen, AGI is a list of tests, and remember, an engineer can only, an engineer knows that we've, you know, anybody in that prestigious organization that I'm now part of, knows for sure about engineers is that you need to have a specification and you need to know what the definition of success is. You need to have a test.
例如,在我们交谈时,有一些问题您现在回答我。我主要在做生成式人工智能。我没有花太多时间来推敲这个问题。然而,有一些问题,比如规划问题,我会觉得,这个有意思。让我想一想。我在脑海中循环思考,提出多个计划。我在遍历树。也许我在遍历我的图,并且修剪我的树,说这没有意义,但这个是我会采纳的,并在脑海中模拟,也许我做一些计算等等。那种长时间的思考,今天AI做得不好。你提出的一切问题,GPT都会立即回应。我们希望在GPT中输入一些内容,给它一个任务说明,给它一个问题,让它思考一会儿,对吧?因此,这种系统,计算机科学称之为系统二思维或长时间思考或规划,这些推理和规划问题,我认为我们正在研究这些问题。我认为你会看到一些突破。因此,未来与AI互动的方式将会有所不同。有些是,给我一个问题然后回答。有些是,这里有一个问题,去研究一下,明天告诉我。它会在明天尽可能多地计算。你也可以说,我会给你这个问题。花费1000美元,但不要超过这个数。它会给出在1000美元内的最佳答案。等等。那就是,现在,AGI。AGI的问题是,什么是定义?事实上,这是至高无上的问题。如果你问我,如果你说詹森,AGI是一系列测试,记住,工程师只能,工程师知道我们,你知道我们现在所属的那个负责任的组织中的任何人,就是你需要有一个规范,并且需要知道成功的定义是什么。你需要有一个测试。
If I gave an AI a lot of math tests and reasoning tests and history tests and biology tests and medical exams and bar exams, you name it. SATs and MCATs and every single test that you can possibly imagine, you make that list of tests and you put it in front of the computer science industry, I'm guessing in five years time, we'll do well on every single one of them. And so if your definition of AGI is that it passes human tests, then I will tell you five years. If you tell me, but if you asked it to me a little bit differently the way you asked it, that AGI is going to be, have human intelligence. Well, I'm not exactly sure how to specify all of your intelligence yet and nobody does, really, and therefore it's hard to achieve. Does an engineer, does that make sense? Okay. The answer is we're not sure, but we're all endeavoring to make it better and better. So I'm going to ask two more questions and I'm going to turn it over because I think there's lots of good questions out there. The first one I was going to ask about is could you just dive a little deeper into what you see as AI's role in drug discovery?
如果我给一个人工智能进行大量的数学测试、推理测试、历史测试、生物学测试、医学考试和律师考试,你可以列出所有你能想象的测试,然后把这份测试清单放在计算机科学行业面前,我猜想五年后,它们会在每一项测试上表现出色。
所以如果你对通用人工智能的定义是通过人类的测试,那么我会告诉你五年后。但是如果你稍微用另一种方式来问我,那通用人工智能会拥有人类的智能。嗯,我不太确定如何具体规定所有的智能,实际上也没有人知道,因此很难实现。工程师们,这样说通不通畅?好的。答案是我们不确定,但我们都在努力让它变得越来越好。我还打算问两个问题,然后把话题交给其他人,因为我觉得会有很多好问题。
第一个问题是,您能深入探讨一下您认为人工智能在药物发现中扮演的角色吗?
The first role is to understand the meaning of the digital information that we have. Right now we have all, as you know, we have a whole lot of amino acids. We can now, because of alpha fold, understand the protein structure and many of them. But the question is now what is the meaning of that protein? What does the meaning of this protein? What does this function? It would be great just as you can chat with GPT. As you guys know, you can chat with a PDF. You take a PDF file, doesn't matter what it is. My favorites are you take a PDF file of a research paper and you load it into chat GPT and just start just talking to it. It's like talking to the researchers. What inspired this research? What problem does it solve? What was the breakthrough? What was the state of art before then? What were the novel ideas? Just talk to it like a human.
第一个角色是理解我们所拥有的数字信息的含义。现在我们拥有所有的氨基酸,因为alpha fold的存在,我们现在可以理解很多蛋白质的结构。但现在问题是,这种蛋白质的含义是什么?这种蛋白质的功能是什么?就像你可以与GPT聊天一样,这将是很棒的。正如大家所知,你可以与PDF进行交流。你可以拿一篇研究论文的PDF文件,加载到聊天GPT中,然后开始与它交流。就像在与研究人员交谈一样。是什么启发了这项研究?解决了什么问题?有什么突破?在那之前技术的发展水平如何?有哪些新颖的想法?用人类的方式与它交流。
In the future, we're going to take a protein, put it into chat GPT just like PDF. What are you for? What enzymes activate you? What makes you happy? For example, there'll be a whole sequence of genes and you're going to take and it represents a cell. You're going to put that cell in. What are you for? What do you do? What are you good for? What are your hopes and dreams? That's one of the most profound things we can do is to understand the meaning of biology. Does it make sense? If we can understand the meaning of biology, as you guys know, once we understand the meaning of almost any information that it's in the world of computer science, in the world of computing, amazing engineers and amazing scientists know exactly what to do with it. That's the breakthrough. The multi-omnic understanding of biology. That's if I could deep and shallow answer to you. I think that's probably the single most profound thing that we can do. Boy, Oregon State and Stanford are really proud of you.
在未来,我们将把一种蛋白质放入像PDF一样的聊天GPT中。你是什么?哪种酶会激活你?什么会让你开心?例如,会有一系列基因,你会拿出来并代表一个细胞。你是为了什么?你会做什么?你有什么用处?你有什么希望和梦想?理解生物学的意义是我们可以做的最深刻的事情之一。这有意义吗?如果我们能理解生物学的意义,就像你们知道的,一旦我们理解了几乎世界上任何信息的意义,在计算机科学领域,在计算领域,了不起的工程师和了不起的科学家都会准确知道该怎么做。这就是突破。对生物学的多元理解。这是我可以对你提供的深浅答案。我认为这可能是我们可以做的最深刻的事情。奥勒冈州立大学和斯坦福大学真为你感到骄傲。
If I could switch gears just a little bit and just say Stanford has a lot of aspiring entrepreneurs, students, they're entrepreneurs and maybe they're computer science majors or engineering majors of some sort. Please, don't build GPUs. What advice would you give them to improve their chances of success? One of my great advantages is that I have very low expectations. And I mean that. Most of the Stanford graduates have very high expectations. You deserve to have high expectations because you came from a great school. You were very successful. You're top of your class. Obviously, you were able to pay for tuition. And then you're graduating from one of the finest institutions on the planet. You're surrounded by other kids that are just incredible. You naturally have very high expectations. People with very high expectations have very low resilience. And unfortunately, resilience matters in success. I don't know how to teach it to you except for I hope suffering happens to you. And I was fortunate that I grew up with it with my parents providing a condition for us to be successful. On the one hand, but there were plenty of opportunities for setbacks and suffering. To this day, I use the word the phrase pain and suffering inside our company with great glee. And I mean that. Boy, this is going to cause a lot of pain and suffering. And I mean that in a happy way. Because you want to refine the character of your company. You want greatness out of them. And greatness is not intelligence as you know. Greatness comes from character. And character is informed out of smart people. It's formed out of people who suffered. And so that's kind of the.
如果我能稍微换个角度,我想说斯坦福有很多有雄心壮志的创业者,学生们是创业者,也许他们是计算机科学或工程专业的学生。请不要建立GPU。您会给他们什么建议,以提高他们成功的机会?我的一大优势是我对成功的期望很低。我是认真的。大多数斯坦福毕业生对成功的期望很高。您理应有高期望,因为您来自一所很好的学校。您非常成功。您是班里的佼佼者。显然,您能支付学费。然后您从地球上最顶尖的机构毕业。您周围都是其他令人难以置信的孩子。您自然有很高的期望。期望很高的人很少具备应对挫折的韧性。不幸的是,韧性在成功中非常重要。我不知道如何教给您,除了希望您经历一些磨难。我很幸运,因为我在父母的环境下长大,他们为我们成功创造了条件,但也有很多机会让我们遭受挫折和痛苦。直到今天,我在公司里用“痛苦和痛苦”这个词组非常开心。我是认真的。哇,这将会带来很多痛苦和痛苦。我是开心的,因为您希望磨练公司的品质。您希望他们取得伟大成就。而伟大不是智慧,您知道的。伟大来源于品格。品格是由聪明人塑造出来的。它是由曾经遭受痛苦的人形成的。这就是这样。
And so if I could wish upon you, I don't know how to do it. But for all of you Stanford students, I wish upon you ample doses of pain and suffering. I'm going to back out on my promise and ask you one more question. How do you, you seem incredibly motivated and energetic. But how do you keep your employees motivated and energetic when they probably become richer than they ever expected to be?
所以如果我能许个愿望给你们,我不知道该怎么做。但对于所有斯坦福的学生,我希望你们能获得充足的痛苦和苦难。我要收回我之前的承诺,再问你们一个问题。你们似乎非常有动力和活力,但是当你们的员工可能变得比他们预期的更富有时,你们如何保持他们的动力和活力?
Yeah, I'm surrounded by 55 people. My management team, so you know, my management team, my direct reports is 55 people. I write no reviews for any of them. I give them constant reviews. And they provide the same to me. My compensation for them is the bottom right corner of Excel. I just drag it down. Literally many of our executives are paid the same. Exactly the dollar. I know it's weird. It works. And I don't do one on ones with any of them. Unless they need me, then I'll drop everything for them. I never have meetings with them just alone.
是的,我周围有55个人。我的管理团队,你知道的,我的管理团队,我的直接下属有55人。我不为他们写评语。我不断地给他们反馈。他们也给我同样的反馈。他们的薪酬是Excel表格右下角。我只需要往下拉。实际上,我们许多高管的薪酬都是一样的。确切到美元。我知道这很奇怪。但这样做有效。我不会和他们中的任何人做一对一的会议。除非他们需要我,那么我会为他们放下一切。我从不和他们独处开会。
And they never hear me say something to them that is only for them to know. There's not one piece of information that I somehow secretly tell East Ave. That I don't tell the rest of the company. And so in that way, our company was designed for agility, for information to be, to flow as quickly as possible, for people to be empowered by what they are able to do, not what they know. And so that's the architecture of our company. I don't remember your question. But oh, oh, oh, oh, oh, oh, oh, oh, oh, I got it. I got it. I got it. And the answer for that is my behavior. How do I celebrate success? How do I celebrate failure? How do I talk about success? How do I talk about setbacks? Every single thing that I'm looking for opportunities to instill every single day.
他们从来没有听过我对他们说的只有他们需要知道的事情。我没有偷偷告诉东大街的任何一条信息,而我却没有告诉公司其他人。因此,我们公司的设计目的是为了灵活性,信息能够尽可能迅速地流动,人们可以通过他们所能做的事情而不是他们所知道的事情得到赋权。这就是我们公司的架构。我记不起你的问题了。但噢,噢,噢,噢,噢,我明白了。我知道了。我明白了。问题的答案就是我的行为。我如何庆祝成功?我如何庆祝失败?我如何谈论成功?我如何谈论挫折?我都在每一天都在寻找机会来灌输。
I'm looking for opportunities to keep on instilling the culture of the company. And what is important? What's not important? What's the definition of good? How do you compare yourself to good? How do you think about good? How do you think about a journey? How do you think about results? All of that, all day long. Mark, Duggan, can you help us? Okay, good. So let's open it up for some questions. Let me start with Winston, and I'll come to you. We need a microphone. Can you just bend? You got this? Yeah. Board member Winston. I have a couple questions. What's the story about your leather jacket? And the second is according to your projection and calculation, in five to ten years, how much more semiconductor manufacturing capacity is needed to support the growth of AI?
我正在寻找机会继续灌输公司文化。什么重要?什么不重要?什么是好的定义?你如何与好相比?你如何看待好?你如何看待旅程?你如何看待结果?这些问题,整天都在考虑。Mark, Duggan,你们能帮助我们吗?好的,那么让我们开放一些问题。我先从 Winston 开始,然后轮到你。我们需要一个麦克风。你能弯腰拿吗?你能做到吗?是的。董事会成员 Winston。我有几个问题。你的皮夹克有什么故事?根据你的预测和计算,五到十年内,需要多少更多的半导体制造能力来支持人工智能的增长?
Okay, I appreciate two questions. The first question is, this is what my wife bought for me, and this is what I'm wearing. And because I do zero percent of my own shopping, as soon as she finds something that doesn't make me itch, because she knows she's known me since I was 17 years old, and she thinks that everything makes me itch. And the way I say I don't like something is it makes me itch. And so as soon as she finds me something that doesn't make me itch, if you look at my closet, the whole closet is a shirt, because she doesn't want to shop for me again.
好的,我欣赏两个问题。第一个问题是,这是我妻子给我买的,这是我穿的衣服。因为我自己零百分之零的购物,只要她找到一件不让我痒的东西,因为她认识我自从我17岁起,她觉得所有东西都会让我痒。我说不喜欢某件东西的方式就是让我痒。所以只要她找到了一件不让我痒的东西,如果你看看我的衣柜,整个衣柜里都是衬衫,因为她不想再为我购物了。
And so that's why this is all she bought me, and this is all I'm wearing. And if I don't like the answer, I can go shopping. Otherwise I can wear it. And it's good enough for me. Okay, we've got the second question on this. The forecast is actually very, this is very, I'm horrible at forecasting, but I'm very good at first principle reasoning of the size of the opportunity. And so let me first reason for you. I have no idea how many fabs, but here's the thing that I do know. The way that we do computing today, the information was written by someone, created by someone. It's basically prerecorded. All the words, all the videos, all the sound, everything that we do is retrieval based. It was prerecorded. Does that make sense?
所以这就是为什么她只买了这些给我,这也是我穿的。如果我不喜欢这个答案,我可以去购物。否则我就穿这个。这对我来说已经足够了。好的,我们来谈一下第二个问题。预测实际上非常困难,我对预测很差,但是我在机会规模的首要原理推理方面非常擅长。所以让我来为你推理一下。我不知道有多少个制造工厂,但是我知道的一件事是,我们今天进行计算的方式是,信息是由某人编写或创建的。它基本上是预先录制的。我们做的所有事情,所有的文字、视频、声音,都是检索系统基础。它们是预先录制的。这样理解可以吗?
As I say that, every time you touch on a phone, remember, somebody wrote that and stored it somewhere. It was prerecorded. Okay? Every modality that you know. In the future, because we're going to have AIs, it understands the current circumstance, and because it's tapped into all of the world's latest news and things like it's called retrieval based, okay, and it understands your context, meaning it understood why you asked, what you're asking about. When you and I ask about the economy, we probably are meeting very different things, and for very different context. And based on that, it can generate exactly the right information for you. So in the future, it already understands context, and most of computing will be generative. In the, today, 100% of content is prerecorded.
当我说这些话的时候,请记住,每次触摸电话时,都有人编写并存储了信息。这些信息是预先录制的。好吗?每一种模式你都知道。在未来,因为我们将会有人工智能,它能理解当前情况,并且因为它与世界上所有最新的新闻和其他信息联系在一起,这被称为检索系统,它能理解你的上下文,也就是理解你提问的原因及内容。当你和我谈论经济时,我们可能指的是完全不同的事情,有着完全不同的背景。基于这一点,它会为你生成出完全正确的信息。所以在未来,它已经理解了上下文,并且大部分的计算会是生成性的。而今天,100%的内容都是预先录制的。
If in the future 100% of content will be generative, the question is how many, how does that change the shape of computing? And so without torturing you anymore, that's how I reason through things. How much more networking do we need, more or less of that, do we need memory of this? And the answer is we're going to need more fabs. However, remember that we're also improving the algorithms and the processing of it tremendously over time. It's not as if the efficiency of computing is what it is today, and therefore the demand is this much. In the meantime, I'm improving computing by a million times every 10 years, while demand is going up by a trillion times. And that has to offset each other. Does that make sense? And then there's technology diffusion and so on and so forth. That's just a matter of time.
如果将来100%的内容都是生成的,问题是,这将如何改变计算的形态?所以,不再折磨你了,这就是我通过事物的方式推理。我们需要多少更多的网络,更多或更少的记忆?答案是我们将需要更多的fabs。然而,请记住,随着时间的推移,我们也在极大地改进算法和处理。计算的效率并不是今天的样子,需求也不是现在的这么多。同时,我每10年就将计算提高一百万倍,而需求却增长了一万亿倍。这两者必须互相抵消。这有道理吗?然后还有技术扩散等等,这只是个时间问题。
It doesn't change the fact that one day all of the computers in the world will be changed 100%. Every single data center will be, all of those generative computing data centers, 100% of the trillion dollars worth of infrastructure will be completely changed. And then there'll be new infrastructure built on, even on top of that. Okay, next question right here, Ben. And then over here to Randy. So, yeah. Thanks for coming today. So recently you said that you encourage students not to learn how to code.
这并不改变一个事实,那就是世界上所有的计算机总有一天会完全变化。每一个数据中心都将改变,所有那些生成计算数据中心,价值数万亿美元的基础设施都将完全改变。然后会建立新的基础设施,甚至在其之上建立一切。好的,下一个问题给本。然后再到兰迪这里。所以,是的。感谢今天的光临。最近你说鼓励学生不要学习编程。
Yeah. And if that's the case, it means one of maybe a few things. But do you think the world starts to look like from a company formation and entrepreneurship perspective that it goes towards many, many more companies that are created? Or do you think it's consolidation to just a number of the big players? Yeah. So first of all, I said it so poorly that you repeated back poorly. I didn't, if you would like to code, for God's sakes, code. Okay. If you want to make omelets, make omelets. I'm not, you know, coding has, coding is a reasoning process. It's good. Does, is it going to guarantee you a job? No, not even a little bit. The number of coders in the world surely will continue to be important. And we, NVIDIA needs coders. However, in the future, the way you interact with the computer is not going to be C++ mostly. For some of us, that's true.
是的。如果是这种情况,意味着可能有几种情况。但你认为世界从公司形成和创业的角度来看,是朝着创建更多更多公司的方向发展呢?还是你认为这是大公司的整合?是的。首先,我表达得太糟糕了,你说得也不好。如果你想编码,拜托,就去编码。如果你想做煎蛋卷,就去做煎蛋卷。我并不认为编码是一种过程。编码是一种推理过程。很好。它会保证你有工作吗?不,一点也不会。世界上编码人员的数量肯定会继续重要。而我们NVIDIA需要编码人员。然而,在未来,你与计算机互动的方式不会主要是C++。对一些人来说,这是真的。
For some of us, that's, but for you, you know, why, why programming Python? So weird. In the future, you'll tell the computer what you want. And the computer will, will, you say hi, I would like you to come up with a, a build plan with all of the suppliers and build the material for a forecast that we have for you. And based on all the equipment, all the necessary components necessary, come up with a build plan. Okay. And then if you, if you don't like that, you write me a Python program that I can modify of that build plan. And so remember, the first time I talked to the computer, I'm just speaking in plain English. The second time, so English, by the way, human, it's the best programming language of the future.
对于我们中的一些人来说,这可能很晦涩难懂,但对于你来说,你知道为什么选择编程Python吗?这多么奇怪。将来,你会告诉计算机你想要什么。然后计算机会根据你的要求制定一个供应商清单和建材预测的构建计划。基于所有必要的设备和组件,制定一个构建计划。好的。如果你不喜欢这个计划,那就给我写一个Python程序,让我可以修改它。记住,第一次我和计算机交流时,只是用简单的英语交流。第二次,顺便说一句,英语是未来最好的编程语言。
How you talk to a computer, how do you prompt it? It's called prompt engineering. How you interact with people. How do you interact with computers? How do you make a computer do what you want it to do? How do you fine tune the instructions with that computer? That's called prompt engineering. There's an artistry to that. Okay. So for example, most people are surprised by this, but it's not surprising to me, but it's surprising. For example, you asked me a journey to generate a picture, an image of a puppy on a, on a surfboard in Hawaii at sunset. Okay. And then, and then, and then a generous one. Go and you say, oh, more cute. Make it more cute. And it comes back, it's more cute. And you go, no, no, cuter than that. And it comes back. Why is it that software would do that? There's a, there's a structural reason why it does that. But for example, you need to know that that, that capability exists in the computer in the future. Isn't that right? If you don't like the answer first time, you could, you could fine tune in and get it to within the context that you, you know, you can make it, give you better, better results. And, and once you, you can even ask it to write the program altogether to generate that result in the future. And so my point is that programming has, has changed in a way that is probably less valuable.
你如何与计算机交流,如何提示它?这就是所谓的提示工程。你如何与人交互?你如何让计算机做你想让它做的事情?你如何调整计算机的指令?这就叫做提示工程。其中有一种艺术性。比如,大多数人会对此感到惊讶,但对我来说并不奇怪,但确实令人惊讶。比如,你让我生成一幅图片,一个小狗站在夏威夷的日落冲浪板上。然后,你说,再可爱一点。结果变得更可爱了。接着你说,不,再更可爱点。计算机会回应。软件为什么会这样做呢?这其中有一个结构性的原因。但是,比如,你需要知道,这种能力在计算机中存在,以后可以使用。对吧?如果第一次不喜欢答案,你可以微调,以在你知道的背景下让它给出更好的结果。甚至可以让它写程序,以便在将来产生这种结果。我的观点是,编程方式已经改变,可能不那么有价值。
On the other hand, let me, I will tell you this, that because of artificial intelligence, we have closed the technology divide of humanity. Today, about a, about 10 million people are gainfully employed because we know how to program computers, which leaves the other 8 billion behind. That's not true in the future. We all can program computers. Does that make sense? You all know how to prompt the computer to make it do things. And look at, all you do is look at YouTube and look at all the people who are using prompt engineering, all the kids and, you know, who are making a do amazing things. They don't know how to program. They're just talking to chat, GPT. They just know that if I tell it to do this, they'll do that, you know. And so it's, it's no different than interacting with people in the future. That's, that's the great contribution we've, the computer science industry has made to the world. We've closed the technology divide. That's it. That's inspiring. Okay, over here, we've got that. It sounds right. We've got Randy with a question right over here. So, thank you very much. I'm just wondering about, do you think very much about geopolitical risk and how do you see it impacting your industry if you do? A geopolitical risk, you know, we are almost a poster child of geopolitical risk. And the reason for that is because we make a very important instrument for artificial intelligence and artificial intelligence. John and I were talking about earlier is the defining technology of this, of this, of this time. And, and, and so the United States has every right to determine that this instrument should be limited to, to countries that, that it determines that it should be limited, limited with. And so, so the United States has that right and they, they exercise that right. And your question has to do with what is the implication to us?
另一方面,让我告诉你,由于人工智能的出现,我们已经消除了人类之间的技术鸿沟。如今,大约有大约1千万人获得了有利可图的就业机会,因为我们知道如何编程电脑,这给其他80亿人留下了差距。但未来将不再如此。我们都能够编程电脑。这是有意义的吗?你们都知道如何提示电脑让它执行任务。看看,你们只需要看看YouTube,看看所有使用提示工程的人,所有的孩子们,你知道,他们正创造出神奇的事物。他们并不知道如何进行编程。他们只是在与聊天机器人GPT互动,他们只知道如果告诉它做这个动作,它就会执行那个动作。因此,在未来与人们互动并没有什么不同。这是计算机科学行业对世界所做出的巨大贡献。我们已经消除了技术鸿沟。这是令人鼓舞的。好的,在这里,我们有这个。听起来很对。我们有兰迪提出了一个问题。所以,非常感谢。我只是想知道,你是否非常关注地缘政治风险,如果是的话,你认为它如何影响你的行业?地缘政治风险,你知道,我们几乎是地缘政治风险的缩影。原因在于我们为人工智能制造了一种非常重要的工具,而人工智能正是这个时代的决定性技术。因此,美国有权决定该工具是否应该被限制在其认为合适的国家。因此,美国有这个权利,他们行使了这个权利。你的问题涉及这对我们有什么影响?
I, we, first of all, we, we just have to understand these policies and we have to stay agile so that we can comply with the policies. Number one, on the one hand, it limits our opportunity in some places and it opens up opportunities in others. One of the things that has happened in the last, I would say, maybe even six to nine months is the awakening of every single country, every single society, the awakening that they have to control their own digital intelligence. That India can't outsource its data so that some country transforms that digital data into India's intelligence and imports that intelligence back to India. That awakening, that sovereign AI that you have to, you have to dedicate yourself to control your sovereign AI, your sovereign intelligence, protect your language, protect your culture for your own industries. That awakening, I think, happened in the last six, nine months. The first part was we have to be, we have to be mindful about safety. And the second part was, hold on a second, we, we all have to do this. And so every single country from, from India, Canada is doing this. The UK, France, Japan, Singapore, Malaysia, the list goes on. But every single country now realize that they have to invest in their own sovereign AI. So geopolitics, in the one hand, limited opportunities, but it created just enormous opportunities elsewhere. And so, hard to say.
首先,我们必须理解这些政策,保持灵活以便遵守这些政策。首先,一方面,它限制了我们在某些地方的机会,但在其他地方创造了机会。在过去的六到九个月里,每个国家、每个社会都觉醒了,意识到他们必须控制自己的数字智能。印度不能把数据外包给某个国家,让那个国家把数字数据转化为印度的智能,并把智能重新引入印度。这种觉醒,你必须专注于控制你的主权人工智能,你的主权智能,保护你的语言,保护你的文化,为你自己的产业。我认为,在过去的六到九个月里,这种觉醒发生了。首先是我们必须谨慎对待安全。其次是,等等,我们都必须这样做。因此,从印度、加拿大、英国、法国、日本、新加坡、马来西亚等每个国家现在都意识到他们必须投资于自己的主权人工智能。因此,一方面地缓和了机会,但在其他方面创造了巨大的机会。所以,很难说。
Okay, so I think we, I have multiple hands, but I have time for one more question. I am going to go right here. You had to, you were further on the question. Now remember, the last question has all, big pressure. You guys agree with that? Big pressure right here. The person who asked the last question, don't, don't leave us all depressed. I'm going to. Don't trigger me, please. I'm, I'm, that's all I'm saying. I'm just kidding. I'm going to invoke your commandment to have low expectations at this juncture. You, you mentioned you're competing with your customers and I'm wondering, you know, given the advantages that you have, why they're doing that. And I'm wondering if in the future you see yourself building more customized solutions for customers of a certain scale, as opposed to, you know, the solutions that you have now, which are more horizontal. So are we willing to customize the answer to yes?
好的,我认为我们,我手头有多个问题,但我还有时间再回答一个问题。我就从这个问题开始吧。你必须明白,最后一个问题有很大的压力。你们同意吗?这里有很大的压力。问最后一个问题的人,不要让我们都感到沮丧。我会的。请不要刺激我,我只是说说而已。开玩笑的。在这个时刻,我会遵循你的建议,降低期望值。你提到你正在与你的客户竞争,我想知道,鉴于你们已经拥有的优势,为什么他们会这样做。我想知道未来你是否会为一定规模的客户构建更多定制化的解决方案,而不是现在更为横向的解决方案。我们愿意定制化回答这个问题吗?
Now why is it that the, the bars relatively high? The reason why the bar is high is because each generation of our, our platform, first of all, there's a GPU. There's a CPU. There's a networking processor. There's a, there are two types of switches. I just build five chips for one generation. People thinks it's one chip, but it's five different chips. Each one of those chips are hundreds and hundreds of millions of dollars to do. Just hitting launch, which is tape out for us, launching a rocket is several hundred million dollars each time. Okay. I just, I got five of them per generation. Then you've got to put them into a system and then you've got to put, you know, if you got networking stuff, you got K transceiver stuff, you got optic stuff, you got a mountain of software to do, it takes a lot of software to run the computer as big as this room. And so, so all of that is complicated. If the customization is so different, then, then you have to repeat the entire R&D. However, if the customization leverages everything and adds something to it, then it makes, it makes a great deal of sense. Maybe it's a proprietary security system. Maybe it's a confidential computing system. Maybe it's a new way of doing numerical processing that could be extended. We're very open-minded to that. And our customers know that I'm willing to do all that and recognizes that if you change it too far, you've basically reset. And you've squandered, you know, the nearly $100 billion that's taken us to get here to redo it from scratch. And so they want to leverage our ecosystem to the extent that that will be done very open to it. And they know that. Yeah.
那么为什么这里的门槛相对较高呢?之所以门槛高是因为每一代我们的平台,首先有一个 GPU。有一个 CPU。有一个网络处理器。有两种类型的开关。我只为一代构建了五个芯片。人们认为这是一个芯片,但实际上是五个不同的芯片。每一个芯片都要耗费数亿美元。对我们来说,只是启动,也就是推出,每次推出一枚火箭都要耗费数亿美元。好吧。每代我们有五个。然后你必须把它们放入一个系统,然后你必须加入网络的东西,有 K 收发器的东西,有光学的东西,你有一堆软件要做,要运行像这个房间这么大的计算机需要大量的软件。所以所有这些都很复杂。 如果定制不同得这么多,那么你必须重复整个研发。然而,如果定制利用了一切并添加了一些东西,那么这就是有道理的。也许这是一个专有的安全系统。也许这是一个机密计算系统。也许这是一个新的数值处理方式,可以被扩展。我们对此非常开放。我们的客户知道我愿意去做所有这些,并认识到如果你改变太远,你就基本上重置了。你已经挥霍了我们花了近 1000 亿美元的成果,重新从头开始。所以他们希望利用我们的生态系统,尽可能开放地进行。他们知道这一点。是的。
Okay. So with that, I think we need to wrap up. Thank you so much to John and Jensen.
好的,那么我认为我们需要结束了。非常感谢约翰和詹森。