How “Digital Twins” Could Help Us Predict the Future | Karen Willcox | TED
发布时间 2023-09-05 11:00:39 来源
摘要
From health-tracking wearables to smartphones and beyond, data collection and computer modeling have become a ubiquitous part of everyday life. Advancements in these areas have given birth to "digital twins," or virtual models that evolve alongside real-world data. Aerospace engineer Karen Willcox explores the incredible possibilities these systems offer across engineering, climate studies and medicine, sharing how they could lead to personalized medicine, better decision-making and more.
If you love watching TED Talks like this one, become a TED Member to support our mission of spreading ideas: https://ted.com/membership
Follow TED!
Twitter: https://twitter.com/TEDTalks
Instagram: https://www.instagram.com/ted
Facebook: https://facebook.com/TED
LinkedIn: https://www.linkedin.com/company/ted-conferences
TikTok: https://www.tiktok.com/@tedtoks
The TED Talks channel features talks, performances and original series from the world's leading thinkers and doers. Subscribe to our channel for videos on Technology, Entertainment and Design — plus science, business, global issues, the arts and more. Visit https://TED.com to get our entire library of TED Talks, transcripts, translations, personalized talk recommendations and more.
Watch more: https://go.ted.com/karenwillcox
https://youtu.be/r2_VWdjxchY
TED's videos may be used for non-commercial purposes under a Creative Commons License, Attribution–Non Commercial–No Derivatives (or the CC BY – NC – ND 4.0 International) and in accordance with our TED Talks Usage Policy: https://www.ted.com/about/our-organization/our-policies-terms/ted-talks-usage-policy. For more information on using TED for commercial purposes (e.g. employee learning, in a film or online course), please submit a Media Request at https://media-requests.ted.com
#TED #TEDTalks
GPT-4正在为你翻译摘要中......
中英文字稿 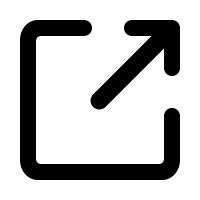
All right, well, let's start with an easy question. How many of you are wearing a Fitbird or an Apple Watch or some other kind of health tracking device? And how many of you have got a smartphone with you here today? Maybe I should say how many of you have not. The fact that so many of us have these technological marvels in our pockets or on our body is a short sign of the revolution that's taken place in computing over the last decade. And I want you to think with me for a second about the elements of that revolution.
好的,嗯,我们先从一个简单的问题开始。你们穿着Fitbird、苹果手表或其他健康追踪设备的有多少人?今天有多少人带着智能手机呢?也许我应该说,有多少人没有带。我们如此之多的人口带着这些技术奇迹,放在口袋里或身上,这是过去十年计算机领域革命的明显标志。我想请你们和我一起思考一下这场革命的要素。
So first off, the data. These devices are collecting data about our health, our movements, our habits and more. And what's really important is that those data are not generic population data, but they're data that are personalized to us, each as an individual.
首先,让我们关注数据。这些设备正在收集关于我们的健康状况、动作、习惯等各方面的数据。而且真正重要的是,这些数据不是泛泛而谈的群体数据,而是针对我们每个人个体化的数据。
Second, and just as important are the models. Inside these devices are very powerful mathematical and statistical models. Some of these models are learned entirely from data, perhaps a machine learning model that is learned to classify whether I'm running or walking or biking or sleeping. Some of these models are based in physics, such as a physiological model that describes the equations that represent cardiac function or circadian rhythm.
其次,同样重要的是模型。这些设备内部包含着非常强大的数学和统计模型。其中一些模型完全是通过数据学习的,例如一个用于分类我正在跑步、散步、骑车还是睡觉的机器学习模型。还有一些模型基于物理学,例如描述心脏功能或生物钟的方程式的生理模型。
And now when things get really interesting is when we start to put the data and the models together. Mathematically this is known as data assimilation. So we have data and we have models. With data assimilation, we start updating the models as new data are collected from the system. And we don't do this update just once, but we do it continually. So as the system changes, as I get older, and my circadian rhythm or as my cardiac function as not what it once was, the new data is collected and the models are evolving and following along with me.
现在当事情变得真正有趣的时候,我们开始将数据和模型结合起来。在数学上,这被称为数据同化。所以我们有数据和模型。通过数据同化,我们开始根据系统收集到的新数据来更新模型。我们不仅仅进行这一次更新,而是持续不断地更新。所以随着系统的变化,随着我逐渐变老,我的昼夜节律或心脏功能不再是过去的样子,新的数据会被收集起来,模型会随之演变并跟随着我。
Now that data assimilation is really important because it's what personalizes the models to me. And that then gets us to the fourth element, which is the element of prediction. Now that I have these personalized models, it's so powerful because I can now get predictions or recommendations that are tailored to me as an individual and that are tailored to my dynamically evolving state over my life.
现在数据同化非常重要,因为它可以个人化模型。这就引出了第四个要素,即预测。现在我拥有了这些个人化模型,它们非常强大,因为我可以得到针对我个人以及在我生活中动态变化的状态的预测或建议。
So that's what I'm describing. This working together of data and models is likely very familiar to all of you because it's been driving your personal choices in retail and entertainment and wellness for many years. But what you might not know is that a similar revolution has been taken place in engineering systems.
所以这就是我正在描述的内容。数据和模型之间的这种协同工作对于你们所有人来说可能非常熟悉,因为它多年来一直在推动你们在零售、娱乐和健康领域的个人选择。但你们可能不知道的是,在工程系统领域正在发生类似的革命。
And in engineering systems, the story is much the same. We have data and we have increasing amounts of data as sensors have become smaller, lighter, cheaper and more powerful. In engineering, we also have models. Our models are usually grounded in physics. These models represent the governing laws of nature. They're powerful models that let us predict how an engineering system will respond.
在工程系统中,情况也是如此。我们有数据,随着传感器变得越来越小、轻便、便宜和功能更强大,我们拥有的数据也越来越多。在工程中,我们还有模型。我们的模型通常根植于物理学。这些模型代表了自然的控制规律。它们是强大的模型,让我们能够预测工程系统的响应。
What you see up here on this slide is a picture of the unmanned aircraft that I have in my research group that we use for a great deal of our research. And for this aircraft, we have powerful finite element models that let us predict how the aircraft structure will respond under different conditions. So these models let us answer questions like will the structure of the aircraft hold together on takeoff if I design it in this way? Or what happens if the aircraft wing gets damaged and I continue to fly it aggressively? Will the aircraft hold together?
这张幻灯片上展示的是我的研究团队使用的无人机的图片,我们在大量的研究中使用它。针对这架飞机,我们拥有强大的有限元模型,可以预测飞机结构在不同条件下的响应。因此,这些模型使我们能够回答诸如:如果我以这种方式设计飞机,那么在起飞时它的结构是否会保持完好?如果飞机的机翼受损,我是否可以继续进行激烈飞行而不会发生事故?这些模型能够帮助我们解答这些问题。
And again, just like the Fitbit and the smartphone example, we can put the data and the models together to build a personalized model of the engineering system, a personalized model of the aircraft. And we call this personalized model a digital twin.
再次,就像Fitbit和智能手机的例子一样,我们可以将数据和模型结合起来构建一个个性化的工程系统模型,一个个性化的飞机模型。我们将这个个性化模型称为数字孪生。
So what is a digital twin? It is a personalized, dynamically evolving model of a physical system. And I want you to think about the digital twin of my aircraft. So as I create that digital twin, I'm going to be collecting data from the sensors on board the aircraft. I'm going to be collecting data from inspections I might make of the aircraft. And I'm going to be assimilating that data into the models.
那么什么是数字孪生?它是一个个性化的、动态演变的物理系统模型。我希望你们能想像一下我的飞机的数字孪生。当我创建这个数字孪生时,我会从飞机上的传感器收集数据。我还会从对飞机的检查中收集数据。然后,我会将这些数据融入模型中。
And what's really important is that I'm not building a generic model of just any old telemaster aircraft. I am building a personalized model of the very aircraft that is right now sitting in my garage down the road in South Austin. And so that digital twin will capture the differences, the variability from my aircraft to say my neighbor's aircraft.
而真正重要的是,我并不是在建造一架普通的泰勒马斯特飞机模型,而是在建造一架个性化的模型,就是现在就停在我位于奥斯汀南部的车库里的那架飞机。因此,这个数字孪生将捕捉到我飞机与我的邻居飞机之间的差异和变异。
And what's more that digital twin will not be static. It's going to change as my aircraft ages and degrades and gets damaged and gets repaired. We will be assimilating data all the time and the digital twin will follow the aircraft through its life.
而且更重要的是,数字孪生不会是静态的。随着飞机的老化、损坏和修复,它将会发生变化。我们将会一直收集数据,并将数字孪生与飞机的生命周期保持同步。
So this is incredibly powerful. I want you to imagine now that you're an airline or maybe in a few years time you're an operator of a fleet of unmanned cargo delivery drones. And imagine that you would have a digital twin like this for every vehicle in your fleet. And think about what that would mean for your decision making. You could make decisions about when to maintain any one aircraft depending on the particular evolving state of that aircraft. You could make decisions about how to optimally fly an aircraft on any given day, given the health of the aircraft, given the mission needs, given the environmental conditions. It would really let you optimally manage that fleet of aircraft.
这是非常强大的。现在我想你想象一下,你是一家航空公司,或者也许在几年后,你将成为一支无人货运无人机舰队的运营者。想象一下,你的舰队中的每一辆交通工具都有一个类似这样的数字孪生。想一想这对于你的决策意味着什么。你可以根据特定交通工具的演变状态决定何时进行维护。你可以根据交通工具的健康状况、任务需求和环境条件在任何一天选择最佳飞行方式。这将真正让你对飞机舰队进行最佳管理。
This idea of a digital twin is pretty neat. The term digital twin was coined in 2010 in a NASA report. But the idea, this idea of a personalized model, combining models and data is much older. And many people point to the Apollo program as being one of the places where digital twins were first put into practice.
这个数字孪生的概念非常好。数字孪生这个词在2010年的一份NASA报告中首次被提出。但这个概念,即个性化模型和数据相结合的想法,要追溯得久远得多。许多人认为阿波罗计划是数字孪生首次被应用的地方之一。
So in the Apollo program back in the 60s and the 70s, NASA would launch Apollo spacecraft up into space. And they would also deploy a simulator, a virtual model on the ground in Houston to follow along on the mission. And now this became very important and it became very useful in the Apollo 13 mission. And again, perhaps you all know the story because we've seen the movie. In the Apollo 13 mission, the spacecraft suffered a malfunction. It was very badly damaged. It became stranded up in space. And so the story goes that NASA were able to take the data from the real aircraft, the physical twin, stuck up in space, feed it into the simulator and to the virtual models on the ground in Houston, do the data assimilation, dynamically evolve the simulator so now that it represented the conditions of the damaged spacecraft, and then use that simulator to run predictions and ultimately guide the decisions that brought the astronauts back home safely.
在60年代和70年代的阿波罗计划中,NASA会将阿波罗飞船发射到太空。同时,他们会在休斯顿地面部署一个模拟器,用来跟随任务。这在阿波罗13任务中变得非常重要,也非常有用。也许你们都知道这个故事,因为我们看过电影。在阿波罗13任务中,太空船发生了故障,严重损坏,被困在太空中。据说NASA能够将真实飞船的数据,输入到模拟器和休斯敦地面的虚拟模型中,进行数据同化,动态演化模拟器,使其代表受损太空船的情况,然后使用该模拟器进行预测,并最终指导决策,安全地将宇航员带回家。
So more than 50 years later, this idea now has a really great name, the name of Digital Twins. And what's really exciting is that it's moving well beyond just aerospace engineering. So in our engineered world, we're starting to see digital twins of bridges and other civil infrastructure for structural health monitoring and predictive maintenance. We're starting to see digital twins of buildings for energy efficiency, digital twins of wind farms to increase efficiency and to reduce downtime. In the natural world, there's a lot of interest in creating digital twins of forests, farms, ice sheets, coastal regions, oil reservoirs, and even talk of trying to create a digital twin of planet Earth. And in the medical world, there's a great deal of interest in creating digital twins to help guide medical assessment, diagnosis, personalized treatment, and in silico drug testing. So many, many exciting potential applications of digital twins.
50多年后,这个想法现在有了一个非常好听的名字,就是数字孪生。令人兴奋的是,它已经超越了航空航天工程领域。在我们工程化的世界里,我们开始看到桥梁和其他土木基础设施的数字孪生,用于结构健康监测和预测性维护。我们开始看到建筑物的数字孪生,用于提高能源效率;风力发电场的数字孪生,以增加效率并减少停机时间。在自然界中,人们对创建森林、农田、冰原、沿海地区、油田甚至地球的数字孪生非常感兴趣。在医学界,人们极有兴趣地创建数字孪生,以帮助指导医疗评估、诊断、个性化治疗和体外药物测试。数字孪生有许多令人兴奋的潜在应用。
But now, I would not like you to leave my talk today thinking that all of this is a reality, that we can create digital twins today of all those complex systems. It's still beyond reach to create a digital twin of an entire aircraft. It's still beyond reach to create a digital twin of a cancer patient or of planet Earth. Creating digital twins of these very, very complex systems is very, very challenging. And let's think for a minute why it's so challenging.
但是现在,我不希望你离开我今天的演讲,认为所有这些都是现实的,我们可以创造出所有这些复杂系统的数字化双胞胎。目前为止,创造一个完整飞机的数字化双胞胎仍然是不可企及的。创造一个癌症患者或者地球的数字化双胞胎仍然是不可企及的。创建这些非常非常复杂系统的数字化双胞胎是非常具有挑战性的。让我们思考一分钟为什么这么具有挑战性。
So one reason it's very difficult is because of the scales that these systems cross. If you think about my aircraft, damage at the microscopic level on the material on the wing of the aircraft translates across scale to impact the way the vehicle flies at the vehicle level. In medicine, we all know that again, changes at the very fine level at the molecular or the cellular level in our bodies translate across scales to have impacts on us at the system level, at the human level. And computational models that resolve all of these scales from the micro scale all the way up to the system level are computationally intractable. We can't solve them even with today's super computing power.
因此,非常困难的一个原因是因为这些系统所跨越的尺度。如果你考虑一下飞机上微观层面上飞机翼材料的损伤,这些损伤会在不同尺度上影响飞机的飞行方式。在医学领域,我们都知道,我们体内分子或细胞层面上的微小变化会跨越尺度对我们的身体系统产生影响,甚至影响到人体整体水平。解析所有这些不同尺度的计算模型,从微观尺度一直到系统层面,这在计算上是不可行的。即使是现在的超级计算能力,我们也无法解决这些问题。
But then you might say, okay, well, what about the data? You said we had a lot of data. Can we not just learn digital twins from data? So yes, we live in an era of big data and we have a lot of data often for our systems. But when it comes to these very challenging, complex systems in engineering, in science and in medicine, the data by themselves are almost never enough. The data are almost always very sparse in both space and in time. The data are almost always noisy and they're indirect. As an engineer, I can almost never measure what it is I want to know. If I want to know about the health on the structure inside my aircraft wing, I can't just break it open and take a look. I am limited to those few sensors that are on the surface of the wing taking those measurements and then trying to guess more than guess, trying to infer what's happening inside the wing. The same is true in medicine. A medical practitioner can't open somebody up to take a look at an organ. Again, we are limited to sparse, noisy and indirect observations taken from the outside to try to infer what's going on.
然后你可能会说,好吧,那数据呢?你说我们有很多数据。我们不能仅仅从数据中学习数字孪生吗?是的,我们生活在大数据时代,我们通常有很多系统的数据。但当涉及到这些极具挑战性、复杂的工程、科学和医学系统时,单凭数据往往是不够的。数据在空间和时间上几乎总是非常稀疏的。数据几乎总是噪声干扰且具有间接性。作为一名工程师,我几乎无法测量我想知道的东西。如果我想知道飞机机翼内部的结构健康状况,我不能只是把它打开看看。我只能依靠机翼表面上的几个传感器来进行测量,然后试图猜测,不,是试图推断出机翼内部的情况。医学也是一样。医生不能打开某人来查看某个器官。同样,我们只能依靠从外部获取的稀疏、噪声干扰和间接的观察来尝试推断发生了什么。
So then you might say, well, we just have to wait a few years because sensing technology will get better and better and better and that's true. Maybe then we'll have enough data to really be able to characterize what is going on inside these very complex systems. But even that's not enough because all that would tell us is what's happening now. And remember, we have to do more than that. We have to be able to predict what might happen in the future if we take different actions. So we're always going to need the models.
那么,你可能会说,我们只需要再等几年,因为感应技术会越来越好,这是正确的。也许到那个时候,我们将有足够的数据来真正描述这些非常复杂系统内部发生的情况。但即使如此,这还是不够,因为所有这些只能告诉我们现在正在发生的事情。请记住,我们需要更多。我们必须能够预测如果我们采取不同的行动,未来可能会发生什么。所以我们总是需要模型。
So this sounds like a huge challenge and indeed it is. But the good news is that we have a lot of hope for addressing this challenge and a big part of this hope rests on this notion of predictive physics based models. These are the models that encode the governing laws of nature that let us make predictions, predict how a cancer tumor might grow or how a cancer tumor might respond to radiotherapy treatment or predict how an Antarctic ice sheet might flow under different future temperature scenarios. And bringing these predictive physics based models together with powerful machine learning, with scalable methods in data simulation and optimization and decision making and with high performance computing, that's the realm of the interdisciplinary field of computational science. And that's the focus of the Odin Institute here at UT Austin where we bring together faculty from 24 different departments across campus to tackle these kinds of challenging problems.
所以这听起来像是一个巨大的挑战,实际上确实是。但好消息是,我们对应对这个挑战有很大的希望,其中很大一部分希望寄托在基于预测物理的模型上。这些模型包含了自然界的控制规律,使我们能够做出预测,预测癌症肿瘤的生长方式、癌症肿瘤对放射疗法的反应方式,或者预测南极冰盖在不同未来温度情景下的流动方式。将这些基于预测物理的模型与强大的机器学习、可扩展的数据模拟、优化和决策方法以及高性能计算结合起来,这是跨学科领域计算科学的范畴。这也是奥丁大学在奥斯汀分校的重点所在,我们汇聚了来自校园内24个不同学科的教师,来解决这类具有挑战性的问题。
So I'm going to close by provoking your imagination and I hope you're excited, like I am, about the idea of a digital twin. And maybe as you go home you can look around and think, what if we had a digital twin of that. But let's look at some examples of some of the really exciting areas where digital twins could make a difference in tackling some of the biggest problems facing society. And as I go through this, you'll also see some of the really exciting research that we have going on here at UT Austin.
所以我要用激发你的想象力的方式结束演讲,希望你能像我一样对数字孪生的想法感到兴奋。当你回家的时候,或许你可以四处看看,想象一下我们如果有这个物体的数字孪生会是怎样的。让我们来看一些令人兴奋的领域的例子,数字孪生在这些领域可能会对解决社会面临的一些最大问题起到重要作用。而且,当我介绍这些领域时,你也将看到我们在德克萨斯大学奥斯汀分校进行的一些非常令人兴奋的研究。
So the first area is space systems. You probably all know we are at the dawn of a new space era. It is so exciting and it's so exciting for our students. And what's even more exciting is that central Texas is right in the midst of that new era. So digital twins clearly have a role to play in managing the health and the operations of space systems, of launch vehicles, of satellites. You can see here this is some of the work that I'm doing together with my colleagues from the Cockrell School, Renata Zanetti and Schunovac Berpier. Digital twins also have a big role to play in tracking and managing space objects and space debris. And here at UT Austin we have one of the world's leading experts in this area. That's Mariba Jar. Mariba is building digital twins for space domain awareness.
所以第一个领域是空间系统。你们可能都知道,我们正处在一个全新太空时代的黎明。这非常激动人心,对我们的学生来说也是如此。更令人兴奋的是,中德州正处在这个新时代的中心。因此,数字孪生在管理太空系统、发射器和卫星的健康和运营方面显然有着重要作用。你们可以看到,这是我和Cockrell学院的同事Renata Zanetti和Schunovac Berpier一起进行的一些工作。数字孪生在跟踪和管理太空物体和太空碎片方面也有着重要作用。而在奥斯汀大学,我们拥有世界领先的专家之一,就是Mariba Jar。Mariba正在为太空领域的意识构建数字孪生。
So we think about the environment in geo-sciences. Again, digital twins could play such a role here.
所以我们在地球科学中考虑环境问题。再次强调,数字孪生可以在这方面扮演重要的角色。
This picture you see omega tarsis, high resolution physics based model of the Antarctic ice sheet, which is put together with observational data of all different kinds to understand what might be going on to help guide decisions about where to drill ice cores, where to take observations and ultimately to inform the decision making around our future climate.
这张图片展示的是欧米茄塔尔西斯,一个高分辨率物理模型,用于研究南极冰盖。该模型结合了各种观测数据,旨在理解可能发生的情况,以帮助决策者确定取样冰芯的位置、观测数据的采集点,并最终为我们未来的气候决策提供信息。
We see also here the work of Clint Dawson in building a digital twin of a coastal area, here the Gulf Coast. Again, combining powerful physics models with all the different kinds of data and here focused on making storm surge modeling for hurricanes even more accurate, again in support of critical decision making.
我们在这里还可以看到克林特·道森在建立海岸地区(这里是墨西哥湾海岸)的数字双生态方面的工作。再次结合强大的物理模型与各种不同类型的数据,并且这次的重点是使飓风引起的风暴潮模拟更加准确,以支持关键决策的制定。
And then in medicine, I think it's pretty clear that digital twins have such a role to play in realizing the promise of personalized medicine. Here we see some of the work of Michael Sachs from our Odin Institute, Willison Centre, in moving towards patient specific personalized heart care and the work of Tom Yankolov and David Hormuth also in the Odin Institute, also working with Dow Medical School and part of biomedical engineering in building digital twins for cancer patients.
然后在医学领域,我认为很明显数字孪生在实现个性化医学的承诺方面发挥了重要作用。在这里,我们可以看到来自我们的奥丁研究所(Odin Institute)威利森中心(Willison Centre)的迈克尔·萨克斯(Michael Sachs)的工作,他在朝着针对特定患者的个性化心脏护理方面取得了进展,还有奥丁研究所的汤姆·扬科洛夫(Tom Yankolov)和大卫·霍默斯(David Hormuth)的工作,他们与道医学院(Dow Medical School)和生物医学工程学部门合作为癌症患者构建数字孪生技术。
So I hope that helps to, as I said, provoke your imaginations to think about what might be possible.
所以我希望这能够帮助你激发想象力,思考可能的事情。
I personally could not be more excited about a future world where digital twins are enabling safer, more efficient engineering systems. They're enabling a better understanding of the natural water around us and they're enabling better medical outcomes for all of us as an individual.
就个人而言,我对未来的世界感到非常兴奋,那个世界中数字孪生将能够实现更安全、更高效的工程系统。它们将帮助我们更好地了解身边的自然水源,并为每个人实现更好的医疗效果。
Thank you.
谢谢你。