Andrew Ng: Opportunities in AI - 2023 - YouTube
发布时间 2023-08-29 00:00:00 来源
中英文字稿 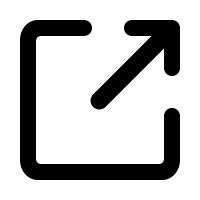
It is my pleasure to welcome Dr. Andrew Moon tonight. Andrew is the managing general partner of AI Fund, founder of Deep Learning AI and Lending AI, chairman and co-founder of COSERA and an adjunct professor of computer science here at Stanford. Previously he had started and led the Google Brain team, which had helped Google adopt modern AI and he was also director of the Stanford AI Lab. About 8 million people, one in 1,000 persons on the planet, have taken an AI class from him and through both his education and his AI work. He has changed numerous lives.
今晚非常荣幸邀请到Andrew博士来到这里。Andrew是AI基金的管理总合伙人,Deep Learning AI和Lending AI的创始人,COSERA的董事长和联合创始人,同时还是斯坦福大学的计算机科学兼职教授。他曾创办并领导谷歌脑团队,帮助谷歌引入现代人工智能,并且还担任过斯坦福人工智能实验室的主任。大约有800万人,全球每1000人中就有一人,曾经从他那里学习过人工智能课程,并且通过他的教育和人工智能工作,他改变了许多人的生活。
Please welcome Dr. Andrew Moon.
请欢迎安德鲁·蒙博士。
Thank you Lisa, it's good to see everyone. So what I want to do today is chat to you about some opportunities in AI. So I've been saying AI is the new electricity. One of the difficult things to understand about AI is that it is a general purpose technology, meaning that it's not useful only for one thing but it's useful for lots of different applications. Kind of like electricity. If I were to ask you what is electricity good for, you know, it's not any one thing, it's a lot of things. So what I like to do is start off sharing with you how I view the technology landscape and this will lead into the set of opportunities.
谢谢Lisa,很高兴见到大家。今天我想与大家聊一聊人工智能方面的一些机会。我一直说AI是新的电力。但要理解AI的一件困难的事情是,它是一种通用技术,意思是它并不只用于一件事,而是可以用于许多不同的应用。就像电力一样。如果我问你电力有什么用,你知道,它不是仅仅用于一件事,而是可以用于很多事情。所以我想开始与大家分享我对技术景观的看法,从而引出一系列机会。
So a lot of excitement about AI and I think a good way to think about AI is as a collection of tools. So this includes a technique called supervised learning which is very good at recognizing things or labeling things and generative AI which is relatively new exciting development. If you're familiar with AI, you may have heard of other tools but I'm going to talk less about these additional tools and I'll focus today on what I think are currently the two most important tools which are supervised learning and generative AI.
关于人工智能(AI)有很多兴奋的讨论,我认为将AI视为一组工具是一种很好的思考方式。其中包括一种被称为监督学习的技术,非常擅长识别事物或标记事物,以及相对较新且令人兴奋的生成式人工智能。如果您熟悉AI,可能已经听说过其他工具,但我今天将更少谈论这些附加工具,而是专注于我认为目前最重要的两种工具,即监督学习和生成式人工智能。
So supervised learning is very good at labeling things or very good at computing inputs, outputs or A to B mappings given input A, give me an output B. For example, given an email, we can use supervised learning to label it as spam or not spam. The most lucrative application of this that I've ever worked on is probably online advertising where given an ad, we can label if a user is likely to click on it and therefore show more relevant ads. For self-driving cars, given the sense of readings of a car, we can label it with where the other cars. One project that my team AI found worked on was ship route optimization where given a route to the ship is taking or considering taking, we can label that with how much fuel we think those are consumed and use this ship's more fuel efficient. It's still a lot of work in automated visual inspection in factories. So you can take a picture of a smartphone that was just manufactured and label is there a stretch or any other defect in it. Or if you want to build a restaurant review reputation monitoring system, you can have a little piece of software that looks at online restaurant reviews and labels that as positive or negative sentiment.
监督学习非常擅长标记事物,或者说在给定输入A的情况下,非常擅长计算输入输出或A到B的映射。例如,给定一封电子邮件,我们可以使用监督学习将其标记为垃圾邮件或非垃圾邮件。我曾经参与的最有利可图的应用可能是在线广告,通过给定广告,我们可以标记用户是否有可能点击它,从而展示更相关的广告。对于自动驾驶汽车,通过获取车辆的感知读数,我们可以标记与其他车辆的位置。我团队AI曾经参与的一个项目是船舶航线优化,根据船舶采取或考虑采取的航线,我们可以标记出我们认为需要消耗多少燃料,并使用更节能的方案来操作该船。在工厂的自动化视觉检测领域还有很多工作要做。例如,您可以对刚刚制造的智能手机进行拍照,并标记出是否存在拉伸或其他缺陷。又或者,如果您想要构建一个餐厅评论声誉监控系统,可以使用一小段软件来查看在线餐厅评论并标记其为积极或消极情感。
So one nice thing, one cool thing about supervised learning is that it's not useful for one thing. It's useful for all of these different applications and many more besides.
监督学习的一个好处是它不仅仅适用于一个领域,而是适用于各种不同的应用以及其他许多领域。
Let me just walk through concretely the workflow of one example of a supervised learning labeling things kind of project. If you want to build a system to label restaurant reviews, you then collect a few data points or collect the data set where say the bestrami sandwich grade, you say that it's positive, server is slow, that's negative, my favorite ship can curry, that's positive. And here I've shown three data points but you're building this, you may get thousands of data points like this or thousands of training examples, we call it. And the workflow of a machine learning project when the AI project is you get labeled data, maybe thousands of data points, then you have an AI entry team train an AI model to learn from this data. And then finally, you would find maybe a cloud service to run the trained AI model and then you can feed it, you know, less quality I've ever had and that's positive sentiment.
让我具体地解释一下一个监督学习的标记项目的工作流程。如果你想要建立一个用于标记餐厅评论的系统,那么你需要收集一些数据点或者收集包含某个比如说犹太熏鱼三明治得分的数据集,你可以说这是正面评价;服务员服务慢的话,那就是负面评价;我最喜欢的船饭有咖喱,那就是正面评价。这里我展示了三个数据点,但是在构建这个系统的时候,你可能会有上千个类似的数据点或者训练样本。当进行AI项目的工作流程时,你需要获得带有标签的数据,可能是上千个数据点,然后你的AI团队会通过这些数据对AI模型进行训练。最后,你可能会找一个云服务来运行已经训练好的AI模型,然后你可以输入类似"这是我吃过的最好的品质"这样的评论,这表示正面情感。
And so I think the last decade was maybe the decade of large scale supervised learning. What we found starting about 10, 15 years ago was if you were to train a small AI model, to train a small neural network or small deep learning algorithm basically, a small AI model may be not on a very powerful computer. Then as you fed more data, its performance would get better for a little bit but then it would flatten out, it would plateau and it would start being able to use the data to get better and better.
因此,我认为过去十年可能是大规模监督学习的十年。大约十五年前,我们发现如果训练一个小型的AI模型,如小型神经网络或小型深度学习算法,可能不需要一台非常强大的计算机。随着输入更多的数据,其性能会稍微提高,但后来会趋于平稳,达到一个优化的平台,然后能够利用数据变得越来越好。
But if you were to train a very large AI model, lots of compute on maybe powerful GPUs, then as we scaled up the amount of data we gave the machine learning model, its performance would kind of keep on getting better and better. So this is why when I started and let the Google brain team, the primary mission that I directed the team to solve at the time was let's just build really, really large neural networks that we then fed a lot of data to and that recipe fortunately worked. And I think the idea of driving large compute and large scale data that recipes really helped us, driven a lot of AI progress over the last decade.
但是,如果你要训练一个非常庞大的人工智能模型,使用大量强大的图形处理器进行计算,那么随着我们给予机器学习模型更多的数据,它的性能会不断提高。所以,当我开始领导谷歌脑团队时,我主要指导团队解决的首要任务就是构建非常庞大的神经网络,并向其提供大量的数据,而这个方法幸运地取得了成功。我认为推动大规模计算和大规模数据的理念,对过去十年来人工智能的进展产生了很大帮助。
So if that was the last decade of AI, I think this decade is turning out to be also doing everything we had in supervisor learning but adding to it the exciting two of generous of AI. So many of you, maybe all of you, have played with chat GPT and bard and so on but just given a piece of text which you call prompt, like I love eating, if you run this multiple times, maybe you get bigger screen keys or my mother's meat level, outer friends and the AI system can generate output like that.
如果说上一个十年是AI的最后一个阶段,我认为这个十年将继续在监督学习的基础上增加了令人兴奋的AI技术。很多人,也可能是所有人,都玩过Chat GPT、Bard等等,只需要输入一个叫做prompt的文本,比如“我喜欢吃”,如果你运行多次,可能会得到不同的输出,比如更大的屏幕按键或者我妈妈的肉汁水平,以及一些其他的输出。
Given the amounts of buzz and excitement about generous of AI, I thought I'd take just half a slide to say a little bit about how this works. So it turns out that generous of AI, at least this type of text generation, the core of it is using supervised learning that inputs output mappings to repeatedly predict the next word. And so if your system reads on the internet, the sentence like, my favorite food is a bagel with cream cheese and locks, then this is translated into a few data points where if it sees my favorite food is A, in this case, try to guess that the right next word was bagel or my favorite food is a bagel, try to guess next word is worth. And similarly, if it sees that, you know, in this case, the right guess for the next word would have been cream. So by taking text that you find on the internet or other sources, and by using this input output, supervised learning to try to repeatedly predict the next word, if you train a very large AI system on hundreds of billions of words, or in the case of the largest models, now more than a trillion words, then you get a large language model like chat GPT.
鉴于AI的热门和兴奋程度,我想花半张幻灯片简要介绍一下它的工作原理。原来,AI的慷慨(generous)特征,至少在文本生成方面,其核心是使用监督学习,通过输入输出映射反复预测下一个单词。所以,如果您的系统在互联网上读到这样一个句子:“我最喜欢的食物是配奶酪和鳟鱼的百吉饼”,那么它会转化为几个数据点,即如果它看到“我最喜欢的食物是”,在这种情况下,尝试猜测正确的下一个词是“百吉饼”;或者"我最喜欢的食物是配奶酪",尝试猜测下一个词是“奶油”。类似地,如果它看到,在这种情况下,正确的猜测下一个词应该是“奶油”。通过利用从互联网或其他来源找到的文本,并使用这种输入输出的监督学习来反复预测下一个单词,如果您使用数千亿个词进行大型AI系统的训练,或者在最大模型的情况下,现在超过一万亿个词,那么您会得到一个像Chat GPT这样的大型语言模型。
And you know, there are additional other important technical details, I thought of predicting the next word. Technically, these systems predict the next sub word, a part of what called a token. And then there are other techniques like our HF for further tuning the AI output to be more hopeful, honest and harmless. But at the heart of it is this using supervised learning to repeatedly predict the next word that that's really was enabling the exciting, you know, really fantastic progress on large language models.
你知道的,还有其他一些重要的技术细节,我考虑到了预测下一个单词。从技术上讲,这些系统预测下一个子词,也就是所谓的令牌的一部分。然后还有其他技术,比如我们的HF,用于进一步调整人工智能的输出,使其更有希望、诚实和无害。但其核心是使用监督学习来反复预测下一个单词,这真的为大型语言模型的令人激动、真正的奇妙进展提供了支持。
So while many people have seen large language models as a fantastic consumer to you can go to a website like chat GPT's website or bots or other large language models and use it as a fantastic to this one of the trend I think is still underappreciated, which is the power of large language models, not just as consumer to, but as a developer to. So it turns out that there are applications that used to take me months to build that a lot of people can now build much faster by using a large language model.
所以虽然很多人认为大型语言模型是一种很棒的消费工具,你可以去像Chat GPT网站或者其他大型语言模型的网站上使用它作为一个很棒的工具。但我认为还有一种趋势仍然被低估,那就是大型语言模型作为开发者的力量。结果证明,以前需要我几个月才能建立的应用程序,现在很多人可以通过使用大型语言模型更快地建立起来。
So specifically, the workflow for supervised learning, building the restaurant review system, say, would be that you need to get a bunch of label data and you know, maybe that takes a month to get a few thousand data points. And then have an AI team train and tune and really get, you know, optimized performance on your AI model. Maybe that will take three months. Then find a cloud service to run it, make sure it's running robustly, make sure it's recognized, maybe that will take another three months. So pretty realistic timeline for building a commercial great machine learning system is like six to 12 months, right?
具体来说,建立餐厅评论系统这种监督学习的工作流程是这样的:首先,你需要获得一堆标记数据,可能需要一个月的时间来获得几千个数据点。然后,你需要让一个人工智能团队来训练和调优你的人工智能模型,以获得最佳表现,可能需要三个月的时间。接下来,你需要找一个云服务运行它,确保它能稳定运行并被认可,可能会再花费三个月的时间。所以,建立一个商业级的优秀机器学习系统的实际时间范围是六到十二个月,对吗?
So teams have led will often took roughly six to 12 months to build and deploy these systems and some of them turned out to be really valuable. But this is a realistic timeline for building and deploying a commercial great AI system. In contrast with prompt based AI, where you write a prompt, this is what the workflow looks like. You can specify a prompt that takes maybe minutes or hours and then you can deploy it to the cloud and that takes maybe hours or days.
所以,团队在构建和部署这些系统时通常需要6到12个月的时间,其中一些系统被证明非常有价值。但是,对于构建和部署商业级的优秀人工智能系统来说,这是一个现实的时间表。相比之下,基于提示的人工智能系统的工作流程如下:您可以指定一个可能需要几分钟或几小时的提示,然后将其部署到云端,这可能需要几个小时或几天的时间。
So there are now certain AI applications that used to take me, you know, literally six months, maybe a year to build that many teams around the world can now build in maybe a week. And I think this is already starting, but the best is still yet to come. This is starting to open up a flood of a lot more AI applications that can be built by a lot of people. So I think many people still underestimate the magnitude of the flood of custom AI applications that I think is going to come down the pipe.
因此,现在有一些人工智能应用,以前我可能需要花费真正的六个月,甚至一年的时间来构建,但现在全球的许多团队可能只需要一周的时间就能完成。我认为这已经开始了,但最好的时代仍在后面。这开始引发了更多人可以构建的人工智能应用的洪流。因此,我认为很多人仍然低估了即将到来的大规模定制人工智能应用的影响。
Now, I know you probably were not expecting me to write code in this presentation, but that's what I'm going to do. So it turns out this is all the code I need in order to write a sentiment classifier. So I'm going to, you know, some of you will know Python, I guess, import some tools from open AI. And then I have this prompt that says, classify the text of the load delimited by three dashes as having either a positive or negative sentiment. Oh, no. I had a fantastic time at Stanford, GSB, and also made great new friends. All right. So that's my prompt. And now I'm just going to run it. And I've never run it before. So I really hope, thank goodness we got the right answer. And this is literally all the code that takes the builder sentiment classifier. And so today, you know, developers around the world can take literally maybe like 10 minutes to build a system like this. And that's a very exciting development.
现在,我知道你可能没想到我在这个演讲中会写代码,但这就是我要做的事情。事实证明,这就是我写一个情感分类器所需要的全部代码。所以,你们中的一些人可能知道Python,我猜,我要导入一些来自Open AI的工具。然后我有一个提示,它说,将被三个破折号括起来的文本分类为积极或消极情感。哦,不。我在斯坦福大学GSB度过了美好的时光,还交了很多好朋友。好了,这就是我的提示。现在我就要运行它了。这是我第一次运行它,所以我真的希望,谢天谢地我们得到了正确的答案。这是构建情感分类器所需的全部代码。所以今天,世界各地的开发者可能只需要十分钟就可以构建出像这样的系统,这是非常令人兴奋的进展。
So one of the things I've been working on was trying to teach, you know, online classes about how they use prompting, not just as a consumer tool, but as a developer tool. So start about the technology landscape. Let me now share my thoughts on what are some of the AI opportunities I see. This shows what I think is the value of different AI technologies today. And I'll talk about three years from now. But the vast majority of financial value from AI today is I think supervised learning, where for a single company like Google can be worth more than 100 billion US dollars a year. And also, there are millions of developers building supervised learning applications.
所以我一直在努力教授在线课程,关于如何使用提示作为开发者工具,而不仅仅是消费工具。让我们首先谈谈技术领域。现在让我分享一下我对人工智能机遇的一些看法。这显示了我认为今天不同AI技术的价值。我还将讨论三年后的情况。但我认为,如今AI带来的绝大部分财务价值是监督学习,像谷歌这样的单一公司每年可以价值超过1000亿美元。此外,还有数百万开发者在构建监督学习应用程序。
So it's already massively valuable and also with tremendous momentum behind it, just because of the sheer effort in, you know, finding applications and building applications. And in generous of AI is the really exciting new entrance, which is much smaller right now. And then there are the other tools I'm including for completeness, we can, you know, the sizes that these circles represent the value today. This is what I think I might grow to in three years. So supervised learning already really massive may double say in the next three years from truly massive to even more massive. And generous of AI, which is much smaller today, I think will much more than double in the next three years because of the number of amount of developer interest, the amount of venture capital investments, number of large corporates exploring applications.
所以它已经非常有价值,也因为将来有巨大的势头支持它,这仅仅是因为在寻找应用和构建应用方面付出了巨大努力。而慷慨的AI是令人兴奋的新进入市场,虽然目前规模较小。还有其他我为了完整性而包含的工具,我们可以看到这些圆圈代表了今天的价值。我认为在三年内,这可能会增长到多少。因此,监督学习已经非常庞大,可能在未来三年内翻倍,从非常庞大变得更加庞大。而慷慨的AI,虽然在今天规模较小,但我认为在未来三年内会翻倍以上,因为开发者的兴趣、风险投资的数量,以及大型企业探索应用的数量都在增加。
And I also just point out three years is a very short time horizon. If it continues to compound anything near this rate, then in six years, you know, it'll be even vastly larger. But this light shaded region in green or orange, that light shaded region is where the opportunities for either new startups or for large companies incumbents to create and to enjoy value capture. But one thing I hope you take away from this slide is that all of these technologies are general purpose technologies. So in the case of supervised learning, a lot of the work that had to be done over the last decade, but it's continuing for the next decade is to identify and to execute on the concrete use cases. And that process is also kicking off for generous of AI.
我还要指出三年是一个非常短的时间范围。如果它以接近这个速度继续复合,那么在六年内,你知道,它将会更加庞大。但是这个绿色或橙色的浅色阴影区域,这个浅色阴影区域是新创企业或大型公司有机会创造和享受价值捕获的机会所在。但我希望你从这张幻灯片上获得一点,那就是所有这些技术都是通用技术。所以在监督学习的案例中,过去十年需要做的大部分工作,但在未来十年仍将继续进行的是确定和执行具体的用例。这个过程也已经开始适用于AI的广泛应用。
So for this part of the presentation, I hope you take away from it that general purpose technologies are useful for many different tasks. A lot of value remains be created using supervised learning. And even though we're nowhere near finishing figure out the exciting use cases of supervised learning, we have this other fantastic tool of generous of AI, which further expands the set of things we can now do using AI. But one caveat, which is that there will be short term facts along the way.
希望在这部分的演示中,你能明白通用技术对于许多不同任务的有用性。使用监督学习可以创造出很多价值。尽管我们还远远没有完全找到监督学习的令人兴奋的应用案例,但我们还有这个出色的AI工具,使我们能够进一步扩展现在可以使用AI进行的任务范围。但是有一个注意事项,就是在这个过程中会发生一些短期的困难。
So I don't know if some of you might remember the app called Lenza. This is the app that will let you upload pictures of yourself and they'll render a cool picture of you as an astronaut or scientist or something. And it was a good idea and people liked it. And it's roughly just took off like crazy like that through last December. And then it did that. And that's because Lenza was, it was a good idea. People liked it. But it was a relatively thin software layer on top of someone else's really powerful APIs. And so even though it was a useful product, it was in a defensible business.
所以我不知道你们是否还记得一个叫做Lenza的应用。这是一个能够让你上传自己的照片,并将你渲染成宇航员、科学家或其他形象的应用。这个想法很好,人们也很喜欢它。去年的十二月份,它突然迅速爆火起来。然后就出现了一个问题。因为Lenza只是一个相对薄弱的软件层,建立在别人非常强大的API之上。所以尽管它是一个有用的产品,但却没有可持续竞争力的商业模式。
And when I think about, you know, absolute Lenza, I'm actually reminded of when Steve Jobs gave us the iPhone. Shortly after, someone wrote an app that I paid $1.94 to do this, to turn on the LED, to turn the phone into a flashlight. And that was also a good idea to write an app to turn on the LED light. But it also wasn't a defensible long term. It also didn't create very long term value because it was a easy, replicated and underprised and eventually incorporated into iOS. But with the rise of iOS, with the rise of iPhone, someone also figured out how to build things like Uber and Airbnb and Tinder. The very long term, very defensible businesses that created sustaining value. And I think with the rise of Gen.2.ai or the rise of new.ai tools, I think what really excites me is the opportunity to create those really deep, really hard applications that hopefully can create very long term value.
当我想到绝对的 Lenza 时,实际上让我想起了史蒂夫·乔布斯给我们带来 iPhone 的时候。很快之后,有人开发了一个应用程序,我支付了1.94美元来使用它,用来打开LED灯,将手机变成一个手电筒。这也是一个很好的点子,写一个应用程序来打开LED灯。但它也不能在长期内进行有效竞争。它并没有创造出长期价值,因为它太容易被复制,并且价格过低,最终被整合到了iOS中。但是随着iOS的崛起,随着iPhone的崛起,有人也找到了如何构建像Uber、Airbnb和Tinder这样的东西。这些非常长期,有竞争力的企业创造了持续的价值。我认为随着Gen.2.ai或新型ai工具的兴起,让我真正兴奋的是有机会创造那些非常深入,非常难的应用程序,希望能够创造出非常长期的价值。
So the first trend I want to share is AI as a general purpose technology. And a lot of the work that lies ahead of us is to find the very diverse use cases and to build them.
我想分享的第一个趋势是人工智能作为一项通用技术。我们面临的工作很大一部分是找到非常多样化的应用场景,并去构建它们。
There's a second trend I want to share with you, which relates to why AI isn't more widely adopted yet. It feels like a bunch of us have been talking about AI for like 15 years or something. But if you look at where the value of AI is today, a lot of it is still very concentrated in consumer software internet. Once you go outside, you go tech or consumer software internet, there's some air adoption, but the law feels very early. So why is that?
我想和大家分享第二个趋势,它解释了为什么人工智能尚未被广泛采用。感觉我们好像已经谈论人工智能15年了之类的。但是如果你看看人工智能的价值所在,很多还是集中在消费软件互联网领域。一旦超出了这个范围,比如技术或者消费软件互联网之外,采用程度就会有所减少,似乎仍处于早期阶段。那么为什么会这样呢?
It turns out if you were to take all current and potential AI projects and sort them in decreasing all their value, then to the left of this curve, the head of this curve, are the multi-billion dollar projects like advertising or web search or for e-commerce, product recommendations or company Amazon. And it turns out that about 10, 15 years ago, you know, various of my friends and I, we figured out a recipe for how to hire, say, 100 engineers to write one piece of software to serve more relevant ads and apply that one piece of software to billion users and generate massive financial value. So that works. But once you go outside consumer software internet, hardly anyone has 100 million or a billion users, they can write and apply one piece of software to you. So once you go to other industries, as we go from the head of this curve on the left over to the long tail, these are some of the projects I see and I'm excited about.
原来,如果将所有当前和潜在的人工智能项目按价值递减排序,那么在这条曲线的左侧,也就是曲线的头部,有着像广告、网络搜索、电子商务产品推荐或亚马逊等价值数十亿美元的项目。事实证明,在大约10到15年前,我和一些朋友们找到了一种方法,可以雇用100名工程师编写一款软件,为数十亿用户提供更相关的广告,并创造巨大的财务价值。这个方法是行之有效的。但是,一旦涉及到消费者以外的软件和互联网行业,很少有人有一亿或十亿的用户可以应用这个软件。因此,当我们从曲线左侧的头部转向曲线的长尾,即其他行业时,以下是我所看到并感到兴奋的一些项目。
I was working with a pizza maker that was taking pictures of the pizza they were making because they needed to do things like make sure that the cheese is spread evenly. So this is about a $5 million project, but that recipe of hiring 100 engineers or dozens of engineers to work on a $5 million project, that doesn't make sense. Or another example, working with an agricultural company that we figured out that we used cameras to find out how tall is the wheat and wheat is often bent over because of wind or rain or something. And we can chop off the wheat to the right height, then that results in more food for the farmer to sell and is also better for the environment. But this is another $5 million project that owed recipe of having a large group of high school engineers to work on this one project. That doesn't make sense. And similarly materials grading, cloth grading, sheet metal grading, many projects like this.
我曾经与一位制作披萨的师傅合作过,他们拍照记录制作过程,因为他们需要确保芝士均匀分布。所以这是一个价值500万美元的项目,但是招聘100名或几十名工程师来完成这个项目,这是没有意义的。另一个例子是与一家农业公司合作,我们发现可以使用摄像头来测量小麦的高度,因为小麦经常因风雨而弯曲。我们可以将小麦剪成适当的高度,这样就可以为农民增加更多的食品销售,并且对环境也更有好处。但这又是一个价值500万美元的项目,使用大批量的高中工程师来完成这个项目,这是没有意义的。类似的项目还有材料分级、布料分级、钢板分级等等。
So whereas to the left in the head of this curve, there's a small number of, let's say, multi-billion dollar projects and we know how to execute those delivering value.
因此,在这个曲线的头部向左,有一小部分,比如说价值数十亿美元的项目,我们知道如何执行并交付价值。
In other industries, I'm seeing a very long tail of tens of thousands of, let's call them, $5 million projects that until now have been very difficult to execute on because of the high cost of customization. The trend that I think is exciting is that the AI community has been building better tools that lets us advocate these use cases and make it easy for the end user to do the customization.
在其他行业中,我发现有数以万计的长尾项目,让我们称之为500万美元项目,直到现在由于定制成本高而很难实施。我认为令人兴奋的趋势是,人工智能社区一直在开发更好的工具,让我们能够倡导这些应用案例,并使最终用户可以轻松进行定制。
So specifically, I'm seeing a lot of exciting low-code and no-code tools that enable the user to customize the AI system. But this means this, instead of me needing to worry that much about pictures of pizza, we have tools, we can start into C2s that can enable the IT department of the pizza making factory to train an AI system on their own pictures of pizza to realize this $5 million worth of value. And by the way, the pictures of pizza, they don't exist on the internet. So Google and Bing don't have access to these pictures. We need tools that can be used by really the pizza factory themselves to build and deploy and maintain their own custom AI system that works on their own pictures of pizza.
具体来说,我看到了许多令人兴奋的低代码和无代码工具,使用户能够定制AI系统。但是这意味着什么呢?不再需要我过多关注披萨的图片,我们有了工具可以启动C2s(可能指的是Cognitive Automation Solutions,企业级自动化解决方案),使披萨制造工厂的IT部门能够根据他们自己的披萨图片来训练AI系统,以实现价值500万美元。顺便说一下,这些披萨的图片并不存在于互联网上,因此Google和Bing无法访问这些图片。我们需要的是真正适用于披萨工厂自己的工具,可以构建、部署和维护他们自己定制的AI系统,该系统能够处理他们自己的披萨图片。
And broadly, the technology for enabling this, some of it is prompting, text prompting, visual prompting, but really large language models and similar tools like that, or a technology called Data Century AI whereby instead of asking the pizza factory to write a lot of code, which is challenging, we can ask them to provide data which turns out to be more feasible. And I think the second trend is important because I think this is a key part of the recipe for taking the value of AI, which so far still feels very concentrated in the tech world and consumer software into their world and pushing this out to all industries really to the rest of the economy, which sometimes is easy to forget. The rest of the economy is much bigger in the tech world.
而广义上,实现这一点的技术,其中一部分是通过提示,文字提示,视觉提示等实现的,但真正起到作用的是大规模的语言模型和类似的工具,比如数据世纪人工智能技术。通过这种技术,我们不再需要让比萨工厂编写大量代码,这是一项具有挑战性的任务,相反我们可以要求他们提供数据,这样更加可行。我认为第二个趋势很重要,因为我认为这是实现AI价值的关键要素,到目前为止,AI的价值仍然在科技界和消费软件领域集中,而将其推广至所有行业乃至整个经济中则变得极为重要,这一点有时候很容易被忽视。整个经济体量远远超过科技界。
So, the two trends I shared, AI is a general purpose technology, lots of concrete use cases to be realized, as well as local, no-code, easily used tools, enabling AI to be deployed in more industries.
因此,我分享了两个趋势,第一个是人工智能是一种通用技术,有许多具体的用例可以实现,同时还有本地化、无代码、易于使用的工具,使得人工智能能够在更多行业中得到应用。
How do we go after these opportunities? So about five years ago, there was a puzzle I wanted to solve, which is I felt that many valuable AI projects are now possible. I was thinking, how do we get them done? And having led AI teams in Google and Baidu in big tech companies, I had a hard time figuring out how I could operate a team in a big tech company to go off there a very diverse set of opportunities and everything from maritime shipping to education to financial services and health care and all and all. It's just very diverse use cases, very diverse, go to marketers and very diverse really, you know, customer bases and applications.
我们如何抓住这些机会呢?大约五年前,我想解决一个难题,即我觉得现在有很多有价值的AI项目是可能实现的。我在思考,我们该如何将它们完成呢?作为谷歌和百度等大型科技公司的AI团队领导者,我一直很难弄清楚如何在这些公司中组建一个团队来开展非常多样化的机会,其中涉及到从海事运输到教育、金融服务和医疗保健等各个方面。这些使用案例非常多样化,市场也非常多元化,客户群体和应用程序也非常多样化。
And I felt that the most efficient way to do this would be if we can start a lot of different companies to pursue these very diverse opportunities. So that's why I ended up starting AI Fund, which is a venture studio that builds startups to pursue a diverse set of AI opportunities. And of course, in addition to lots of startups in company companies also have a lot of opportunities to integrate AI into existing businesses. In fact, one pattern I'm seeing for incumbent businesses is distribution is often one of the cyclical advantages of incumbent companies that they play the cards right can allow them to integrate AI into their products quite efficiently.
我感到最有效的方法是,我们可以成立许多不同的公司来追求这些非常多样化的机会。所以这就是为什么我最终开始了AI基金,它是一个建立初创企业以追求多元化AI机会的风险投资工作室。当然,除了大量的初创企业,公司还有很多机会将AI整合到现有业务中。实际上,我看到的一个模式是,对于现有企业来说,分销常常是一种周期性优势,如果他们能正确把握机会,就能够高效地将AI整合到他们的产品中去。
But just to be concrete, where are the opportunities? So I think of this as a, this is why I think of as the AI stack. At the bottom level is the hardware, semiconductor layer. Fantastic opportunities there, but very capital intensive, very concentrated. So these are a lot of resources relatively few winners. So some people can and should play there. I personally don't like to play them myself.
但是为了具体化,机会在哪里呢?所以我认为这是一个,我认为这就是人工智能领域的“堆栈”。在底层是硬件、半导体层。那里有很多机会,但需要大量的资本投入,且竞争非常集中。因此相对少数人能够获胜。有些人可以选择并且应该在那里参与。但我个人不喜欢这样做。
There's also the infrastructure layer also fantastic opportunities, but very capital intensive, very concentrated. So I tend not to play them myself either. And then that's a developer to layer. What I showed you just now was I was actually using OpenAI's API as a developer tool. And then I think the developer to sector is a hyper competitive. Look at all the startups chasing OpenAI right now, but there will be some mega winners. And so I sometimes play here, but primarily when I think of a meaningful technology advantage, because I think that earns you the right or earns you a better shot at being one of the mega winners.
还有基础设施层也有很棒的机会,但是需要大量资本投入,非常集中。所以我也不会亲自参与其中。然后还有开发者层面。我刚才展示给你的是我实际上在使用OpenAI的API作为开发工具。然后我认为开发者行业具有超级竞争力。看看现在所有追逐OpenAI的初创公司,但肯定会有一些巨大的赢家。所以我有时会在这里进行投资,但主要是在我认为有着有意义的技术优势时,因为我认为这能使你有更好的机会成为其中之一的巨大赢家。
And then lastly, even though a lot of the media attention in the buzz is in the infrastructure and developer tooling layer, it turns out that that layer can be successful only if the application layer is even more successful. And we saw this as the rise of SaaS as well. All of the buzz excitement is on the technology, the tooling layer, which is fine. Nothing wrong with that. But the only way for that to be successful is that the application layer is even more successful so that frankly, they can generate enough revenue to pay the infrastructure and the tooling layer.
最后,尽管许多媒体关注点都集中在基础设施和开发工具层面,但事实证明,只有应用层面更成功,那些层面才能成功。我们在软件即服务的兴起中也看到了这一点。所有的噪音和兴奋都集中在技术和工具层面上,这很好,没有错。但唯一使之成功的方式是应用层面更加成功,这样说实话,他们可以创造足够的收入来支付基础设施和工具层面的费用。
So actually, let me mention one example. Amor Rai, it's actually just texting the CEO yesterday. But Amor Rai is a company that we built that uses AI for romantic relationship coaching. And just to point out, I'm an AI guy and I feel like I know nothing really about romance. And if you don't believe me, you can ask my wife, she will confirm that I know nothing about romance. But we want to build this. We want to get together with the former CEO of Tinder, Renata Niebog, and with my team's expertise in AI and her expertise in relationships. Yes, she ran Tinder. She knows more about relationships than anyone I know. We're able to build something pretty unique using AI for romantic relationship mentoring.
所以,实际上,让我举个例子。Amor Rai,就是昨天给CEO发短信。Amor Rai是我们建立的一个使用人工智能来进行恋爱关系辅导的公司。我想指出的是,我是一个人工智能专家,但在浪漫方面,我觉得自己一无所知。如果你不相信我,你可以问问我的妻子,她会证实我对浪漫一窍不通。但是,我们想要建立这个项目。我们希望与Tinder的前CEO Renata Nieboj以及我团队在人工智能方面的专长和她在恋爱关系方面的专长共同合作。是的,她曾经管理Tinder,她在恋爱关系方面比我认识的任何人都更有经验。我们利用人工智能为浪漫恋爱关系提供指导,创造出了一些非常独特的东西。
And the interesting thing about applications like these is when we look around, how many teams in the world are simultaneously expert in AI and in relationships? And so at the application layer, I'm seeing a lot of exciting opportunities that seem to have a very large market, but where the competition set is very light relative to the magnitude of the opportunity. It's not that they're no competitors, but it's just much less intense compared to the developer tool or the infrastructure layer.
而且,这类应用的有趣之处在于当我们环顾四周时,全球有多少团队在人工智能和人际关系方面同时拥有专业知识呢?因此,在应用层面上,我看到了许多令人兴奋的机会,这些机会似乎有非常庞大的市场,但相对于机会的规模来说,竞争对手很少。并不是说没有竞争对手,而是相比于开发工具或基础设施层面,竞争程度要低得多。
And so because I've spent a lot of time iterating on a process of building startups, what I'm going to do is just very transparently tell you the recipe we've developed for building startups. And so after many years of iteration and improvement, this is how we now build startups. My team's always had access to a lot of different ideas, internally generated ideas from partners. And I want to walk through this with one example of something we did, which is a company bearing AI, which uses AI to make ships more fuel efficient.
因为我花了很多时间不断迭代建立创业企业的过程,所以现在我打算非常公开地告诉你我们开发出的建立创业企业的方法。经过多年的反复尝试和改进,这就是我们现在建立创业企业的方式。我的团队一直有很多不同的想法,包括来自合作伙伴内部产生的想法。我想举一个例子,说明我们做的事情,那就是一家利用人工智能提高船只燃油效率的公司 bearing AI。
So this idea came to me when a few years ago, a large Japanese conglomerate called Mitsui that is a major shareholder in sort of operates major shipping lines. They came to me and they said, hey, Andrew, you should build a business to use AI to make ships more fuel efficient. And the specific idea was, you know, think of it as a Google mask for ships. We can suggest their ship or tell a ship how to steer so that you still get to your destination on time by using, it turns out, about 10% less fuel.
这个想法是几年前一个叫做三井(Mitsui)的大型日本公司提出来的,他们是一家主要的股东,运营着几家大型航运公司。他们找到我,说:“嘿,安德鲁,你应该建立一个利用人工智能使船只更节能的业务。”具体的想法是,你可以把它看作是船只的谷歌导航,我们可以建议船只的航线或者告诉船只如何操纵,以便按时到达目的地,同时减少约10%的燃料消耗。
And so what we now do is we spend about a month validating the idea. So double check. This idea even technically feasible and in terms of prospective customers to make sure that this is marketing. So we spend up to about a month doing that. And if it passes this stage, then we will go and recruit a CO to work with us on the project.
所以现在我们要做的是花大约一个月的时间来验证这个想法。 进行再次检查,看看这个想法在技术上是否可行,以及潜在客户是否对其进行市场推广。 因此我们会花费大约一个月的时间来进行这方面的工作。如果通过了这个阶段,我们将招聘一位首席运营官与我们共同开展这个项目。
When I was starting out, I used to spend a long time working on the project myself before bringing on the CO. But after iterating, we realized that bringing on the leader at the very beginning to work with us, it reduces a lot of the burden of having to transfer knowledge or having a CO come in and revalidate whether we discover it. So the process is we've learned much more efficiently, just bringing the leader at the very start.
当我刚开始做事的时候,我会花很长时间自己独立完成项目,然后再引入首席运营官。但经过多次迭代之后,我们意识到,在最开始就与领导一起工作能够减轻很多知识传递的负担,也不需要首席运营官重新验证我们发现的东西。所以,我们发现在最开始就与领导合作,我们的学习过程更高效。
And so in the case of bearing AI, we found that fantastic CO Dylan Kyle, who's a repeat entrepreneur, one successful exit before. And then we spent three months, six, two weeks sprints to work with them to build a prototype, as well as do deep customer validation. If it survives this stage, and we have about a 2-thirds, 66% survival rate, we then write the first check in, which then gives the company resources to hire the executive team, build the key team, get the MVP working, minimum viable product working, and get some real customers.
在AI行业,我们找到了出色的CO Dylan Kyle,他是一位重复创业者,在此之前已经有一次成功的退出。然后,我们花了三个月的时间,进行了六个两周的高强度工作阶段,与他们一起构建原型,并进行了深入的客户验证。如果在这个阶段存活下来,我们有大约2/3,也就是66%的生存率,然后我们将投资第一笔款项,为公司提供资源来雇佣执行团队、组建核心团队、让最小可行产品运行起来,并吸引真实客户。
And then after that, hopefully then successfully raises additional external rounds of funding, and can keep on growing and scaling. So I'm really proud of the work that my team was able to do to support Mitsui's idea and Dylan Kyle as CO. And today, there are hundreds of ships on the high seas right now that are steering themselves differently because of bearing AI. And 10% fuel savings translates to rough order, maybe $450,000 in savings in fuel per year. And of course, it's also, frankly, quite a bit better for the environment.
然后在那之后,希望能够成功地进行额外的外部融资,并继续成长和扩张。所以我非常为我的团队所做的工作感到自豪,他们为三井的想法和首席运营官Dylan Kyle提供了支持。而今天,因为有了Bearing AI的存在,目前有数百艘船只在高海上以不同的方式自动导航。而10%的燃料节省大致意味着每年节省大约45万美元的燃料费用。当然,这对环境来说也更好一些。
And I think this is not a, I think would not have existed, if not for Dylan's fantastic work, and then also Mitsui brings this idea to me. And I like this example, because this is another one, it's like, this is a start of idea that, just a point out, I would never have come up with myself, right? Because, you know, I've been on a boat, but what do I know about maritime shipping? But it's the deep subject matter expertise of Mitsui that had to zen site together with Dylan and then my team's expertise in AI that made this possible.
我认为,如果不是因为Dylan的出色工作,这个想法可能根本不存在,还有Mitsui也向我介绍了这个想法。我喜欢这个例子,因为这是另一个启发点,我自己可能永远想不到。毕竟,我曾经乘船,但对于海运,我知道什么呢?正是Mitsui的深入专业知识与Dylan以及我的团队在人工智能方面的专业知识相结合,使这成为可能。
And so as I operate in AI, one thing I've learned is my swim lane is AI, and that's it, because I don't have time. It was very difficult for me to be expert in maritime shipping and romantic relationships and healthcare and financial services and on and on and on. And so I've learned that if I can just help get accurate technical validation and then use, you know, AI resources to make sure the AI tech is built quickly and well. And I think we've always managed to help the company's build a strong technical team, then partnering with subject matter experts often results in exciting new opportunities.
所以,当我在人工智能领域工作时,我学到的一件事就是我只专注于AI领域,其他的事情我没有时间去做。在海运、浪漫恋情、医疗保健、金融服务等领域要成为专家是非常困难的。因此,我学到的是,如果我能够帮助确保准确的技术验证,并利用人工智能资源来确保快速且良好地构建人工智能技术,我认为我们总是能帮助公司打造一个强大的技术团队,然后与专业领域的专家合作往往会带来令人兴奋的新机会。
And I want to share with you one other weird aspect of one other weird lesson I've learned about, you know, building startups, which is I like to engage only when there's a concrete idea. And this runs counter to a lot of the advice you hear from the design thinking methodology, which often says don't rush to solution, right? Explore a lot of alternatives for the solution. Honestly, we tried that. It was very slow.
我想与你分享另一个我在建立初创企业过程中学到的奇怪经验,这也是另一个奇怪的教训。我的做法是只在有具体想法的时候才参与其中。这与设计思维方法论中的很多建议相悖,后者经常呼吁不要着急解决问题,要尝试很多解决方案的替代方法。老实说,我们尝试过这样做,但进展非常缓慢。
But what we've learned is that at the ideation stage, if someone comes to me and says, hey, I'm not a financial manager, you should apply AI to financial services. Because I'm not a subject matter expert in financial services, it's very slow for me to go and learn enough about financial services to even figure out what to do. I mean, eventually you could get a good outcome, but it's a very labor intensive, very slow, very expensive process for me to try to learn industry after industry.
但是我们所学到的是,在构思阶段,如果有人来找我说:“嘿,我不是财务经理,但你应该将人工智能应用于金融服务。”因为我不是金融服务的专家,所以我需要花费很多时间去学习足够的金融知识,甚至无法确定该做什么。我的意思是,最终你可能会得到一个好的结果,但对我来说,尝试逐个学习行业是一个非常费力、非常缓慢、非常昂贵的过程。
In contrast, one of my partners wrote this idea as a tongue on the cheek, not really seriously. But, you know, let's say the concrete idea is by GPT, this eliminates commercials by automatically buying every product advertised and they change from not having seen yet. It's not a good idea, but it is a concrete idea. And it turns out concrete ideas can be validated or falsified efficiently. They also give a team a clear direction to execute.
相反地,我的其中一位伙伴以一种挖苦的方式写了这个想法,不是真正地认真对待。但是,你知道,假设具体的想法是由GPT提出的,它通过自动购买每个广告产品并改变他们尚未看到的方式来消除广告。这不是一个好主意,但它是一个具体的想法。并且事实证明,具体的想法可以有效地被验证或证伪。它们还为团队提供了明确的执行方向。
And I've learned that in today's world, especially with, you know, the excitement buzz and exposure to the AI of a lot of people, it turns out that there are a lot of subject matter experts in today's world that have deeply thought about a problem for months, sometimes even, you know, one or two years, but they've not yet had to build a partner. And when we get together with them and here, and they share the idea of us, it allows us to work with them to very quickly go into validation and building.
我发现在今天的世界中,特别是随着人们对人工智能的兴奋和暴露程度增加,实际上有很多学科专家在为一个问题深思熟虑,有时甚至持续了一两年,但他们还没有合作伙伴进行构建。当我们与他们聚在一起时,他们向我们分享了他们的想法,这使我们能够与他们合作,快速进行验证和构建。
And I find that this works because there are a lot of people that have already done the, you know, design thinking of exploring a lot of ideas and willing down to really good ideas. And I find that there's so many good ideas sitting out there that no one is working on, that finding those good ideas that someone has already had and wants to share it with us and wants to build part before that turns out to be a much more efficient engine.
我发现这种方法行之有效,因为已经有很多人进行过探索众多想法并筛选出最好的想法所需的设计思维。而且我发现有很多优秀的想法悬而未决,却没有人去开发,所以找到那些别人已经有过并愿意与我们分享的好点子,并在之前进行一些构建,这将成为一个更高效的引擎。
So before I wrap up, we'll go to the question in a second. Just a few slides to talk about risks and social impact. So it is very powerful technology to state something you probably guess. My team's and I, we only work on projects that move humanity forward. And, you know, we have multiple times, kill projects that we assess to be financially sound based on ethical grounds. It turns out I've been surprising, sometimes dismayed at the creativity of people to come up with good ideas, sorry, to come up with really bad ideas that seem profitable but really should not be built with kill a few projects on those grounds.
在我结束之前,我们会在一会儿来回答问题。现在只有几张投影片来讨论风险和社会影响。这是一项非常强大的技术,你可能已经猜到了。我的团队和我只致力于推动人类进步的项目。你知道的,我们已经多次出于伦理考虑而终止了那些在财务上看起来合适但实际上不应该建立的项目。结果让我有些惊讶,有时甚至感到沮丧,人们想出了一些看起来有利可图但实际上是真的不应该被建立的坏主意。因此我们就终止了一些项目。
And then I think has to make knowledge that AI today does have problems with bias, fairness, accuracy, but also, you know, the technology is improving quickly. So I see that AI systems today are less biased than six months ago and more fair than six months ago, which is not to dismiss the importance of these problems. They are problems that we should continue to work on them, but I'm also gratified at the number of AI teams working hard on these issues to make them much better.
然后我想要明确一点,那就是如今的人工智能确实存在偏见、公平性和准确性等问题,不过,你知道,技术正在迅速进步。因此,我看到当今的人工智能系统比六个月前更少偏见,更加公正,这并不意味着对这些问题的重要性不予理会。这些问题是我们应继续致力解决的问题,但我也对众多人工智能团队在努力解决这些问题以取得更好进展感到满意。
When I think of the biggest risk of AI, I think that the biggest risk, one of the biggest risks is the disruption to jobs. This is a diagram from a paper by our friend at the University of Pennsylvania and some folks that open AI analyzing the exposure of different jobs to AI automation.
当我考虑到人工智能最大的风险时,其中之一就是对工作的扰乱。这张图表来自一篇由我们宾夕法尼亚大学的朋友和开放人工智能组织的一些人员所撰写的论文,分析了各种工作面临人工智能自动化的暴露程度。
And it turns out that whereas the previous wave of automation, mainly the most exposed jobs were often the lower wage jobs, such as when, you know, we put robots into factories. With this current wave of automation is actually the higher wage jobs for the right of these axes that seems to have more of their tasks exposed to AI automation.
事实证明,尽管之前的自动化浪潮主要替代的是一些低收入工作,比如我们将机器人投入工厂,而当前的自动化浪潮实际上是涉及高薪工作中的那些,就是那些在右侧轴线上的工作,它们更多地面临着被人工智能自动化取代的任务。
So even as we create tremendous value using AI, I feel like as citizens and our corporations and the governments and really our society, I feel a strong obligation to make sure that people, especially people whose livelihoods are disrupted, are still well taken care of, are still treated well.
尽管我们利用人工智能创造了巨大的价值,作为公民、企业、政府和整个社会,我认为我们有责任确保人们,尤其是那些受到生计干扰的人们,仍能得到妥善照顾和良好对待。
And then lastly, there's also been, it feels like every time there's a big wave of progress in AI, there's a big wave of hype about artificial gender intelligence as well. When deep learning started to work really well 10 years ago, there was a lot of hype about AGI. Now that gender is working really well, there's another wave of hype about AGI.
最后,似乎每当人工智能取得重大进展时,关于人工智能性别智能也会引发一股热潮。10年前深度学习开始取得重大突破时,人工通用智能(AGI)引发了极大的炒作。而现在,当性别智能大放异彩时,又掀起了一波关于AGI的炒作。
But I think that artificial intelligence, AI didn't do anything that human can do. It still decades away. Maybe 30 to 50 years, maybe even longer. I hope we'll see it in our lifetimes. But I don't think there's any time soon. One of the challenges is that the biological path to intelligence, like humans and the digital path to intelligence, AI, they've taken very different paths. And the funny thing about the definition of AGI is your benchmarking this very different digital path to intelligence with really the biological path to intelligence.
但我认为人工智能(AI)没有做到任何人类能做到的事情。它仍然需要几十年的时间,也许是30到50年甚至更久。我希望我们能在有生之年见到它的问世,但我不认为这会很快实现。其中一个挑战是,生物智能(如人类)和数字智能(即AI)之间走过了截然不同的路径。有趣的是,关于强人工智能(AGI)的定义是将这两种截然不同的数字和生物智能路径进行比较。
So I think, you know, large language models are smarter than any of us in certain key dimensions, but much dumber than any of us in other dimensions. And so forcing it to do everything a human can do is a funny comparison. But I hope we'll get there. Maybe hopefully we'll in our lifetimes.
所以我认为,你知道,大型语言模型在某些关键维度上比我们任何人都聪明,但在其他维度上比我们都要愚蠢。因此,迫使它做人类能做的一切是一种有趣的比较。但我希望我们能达到那个程度。也许,希望我们能在我们的一生中实现这一点。
And then there's also a lot of, I think, overblown hype about AI creating extinction risk for humanity. Candidly, I don't see it. I just don't see how AI creates any meaningful extinction risk for humanity. I think that people worry, we can't control AI, but we have lots of AI will be more powerful than any person.
然后还有很多关于人工智能给人类造成灭绝风险的夸大宣传,我认为这太过夸张了。坦率地说,我看不到这种风险。我只是看不到人工智能如何给人类带来任何有意义的灭绝风险。我认为人们担心我们无法控制人工智能,但我们将拥有比任何人类更强大的人工智能。
But with lots of experience steering very powerful entities, such as corporations or nation states, they're far more powerful than any single person and making sure they for the most part benefit humanity. And also technology develops gradually the so-called hot take off scenario, whereas not really working today.
但是拥有丰富经验来引导公司或国家等非常强大的实体,它们比任何单个个体都更加强大,并确保它们在很大程度上造福于人类。而且,技术的发展是逐渐进行的,所谓的快速起飞情景实际上在今天并不适用。
意思是说,有丰富经验的人能够管理强大的组织或国家,这些实体比任何单个人都更有能量,并且他们会尽力让这些实体为人类带来最大的好处。此外,技术的发展是逐渐进行的,所以所谓的快速起飞情景在现实中并不适用。
And then suddenly one day overnight it works brilliantly and we achieve super intelligence takes over world. That's just not realistic. And I think the AI technology would develop slowly like all of that, you know, like, and then it gives us plenty of time to make sure that we provide oversight and can manage it to be safe.
然后有一天突然之间,它突然变得极其出色,超级智能掌控了世界。然而,这种情况并不现实。我认为AI技术的发展会慢慢进行,就像其他技术一样,这样我们就有足够的时间来确保我们提供监督和可以安全管理它。
And lastly, if you look at the real extinction risk of humanity, such as fingers crossed the next pandemic or climate change leading to a massive depopulation of some positive planets or much lower odds that maybe someday and as they're doing to us what it had done to the dinosaurs, I think we looked the actual real extinction risk to humanity. AI having more intelligence, even artificial intelligence in the world, would be a key part of the solution.
最后,如果你看看人类面临的真正灭绝风险,比如下一个大流行病或气候变化导致某些适宜居住的星球人口大规模减少,或者更低的可能性,也许总有一天它们对我们所做到与恐龙一样,我认为我们应该关注对人类真正的灭绝风险。更加智能的人工智能,包括世界上的人工智能,将是解决这个问题的重要组成部分。
So I feel like if you want humanity to survive and thrive for the next thousand years rather than slowing AI down, which some people propose, I would rather make AI go as fast as possible.
所以,我觉得如果你想让人类在未来的千年里生存和繁荣,而不是像一些人建议的那样减缓人工智能的发展速度,我宁愿让人工智能尽可能地发展得更快。
So with that, just to summarize, this is a lot of the last slide, I think that AI as a general purpose technology creates a lot of new opportunities for everyone. And a lot of the exciting and important work that lies ahead of us all is to go and build those concrete use cases and hopefully in the future, hopefully I have opportunities to maybe engage with more of you on those opportunities as well.
因此,总结一下,这张最后的幻灯片就是讲述了很多东西,我认为人工智能作为一种通用技术为每个人创造了许多新机会。我们所有人面临的激动人心且重要的工作之一就是构建那些具体的应用案例。希望未来会有机会与更多的人一起合作探索这些机会。
So that, let me just say thank you all very much.
所以,让我只是说非常感谢大家。