What Machine Learning Reveals About Forming a Healthy Habit
发布时间 2023-06-30 08:00:29 来源
摘要
Contrary to popular belief, behaviors don’t become habits after a “magic number” of days. Wharton’s Katy Milkman shares what machine learning is teaching scientists about habit formation. Hosted on Acast. See acast.com/privacy for more information.
GPT-4正在为你翻译摘要中......
中英文字稿 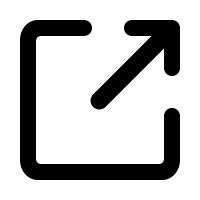
This podcast is brought to you by the Wharton School at the University of Pennsylvania. Can machine learning help us better understand how habits are formed? That was the goal of a group of researchers, including our next guest, Katie Milken, his professor of operations, information and decisions here at the Wharton School, also author of the recently released book, How to Change.
本播客由宾夕法尼亚大学沃顿商学院提供。机器学习能帮助我们更好地理解习惯形成的过程吗?这是一群研究人员的目标,其中包括我们的下一位嘉宾Katie Milken,他是沃顿商学院的运营、信息与决策教授,也是最近出版的书《如何改变》的作者。
Katie, great to have you back with us. Thanks for your time. Thanks for having me. I'm delighted to be here. Thank you. And I guess the interesting thing to start out with here is the term habit and habit forming is looked at in a variety of different ways, depending on kind of different sciences that are out there. And you note this in the paper right out of the gate. Take us through a little bit of how habit and habit forming is viewed in these areas.
凯蒂,很高兴你能回到我们这里。谢谢你抽时间。谢谢你邀请我。我很高兴能在这里。谢谢你。我想从这里开始探讨的有趣话题是习惯和习惯养成这个术语,在不同的科学领域中有不同的看法。你在论文中正好提到了这一点。能否向我们解释一下在这些领域中,习惯和习惯养成是如何看待的?
Yeah, well, habit formation has been studied really differently by psychologists and economists and neuroscientists. Some are trying to look at markers in the brain that predicts how habitual behaviors. Some have studied it in animals. In fact, we have a lot of great data on what looks like habit in animals and animal models. Economists like to study it experimentally, trying to see if they can design conditions that produce habit. And psychologists sometimes collect self-report data on how habitual behaviors have become. But to our knowledge, our work is really the first to look at habit formation happening in the wild.
是的,习惯形成的研究在心理学家、经济学家和神经科学家之间有不同的研究方法。一些人试图查看大脑中的标记,预测习惯性行为。有些人研究了动物方面的情况。实际上,我们对动物和动物模型中习惯行为的数据非常丰富。经济学家喜欢通过实验研究习惯形成问题,试图设计能产生习惯的条件。而心理学家有时会收集有关习惯行为程度的自我报告数据。但据我们所知,我们的研究是第一个观察到野外中习惯形成的研究。
So in a naturalistic way, people going about their daily lives and habits taking shape and to try to actually apply machine learning to explore, well, how do those habits take shape? What are the factors that underlie habit formation? How long does it take? And we do so with data sets from two different settings because we want to see how generalizable are the insights we can draw. One is looking at data on people's habit formation happening naturally when they join a gym. And the other is looking at caregivers and hospitals and how they form habits around hand sanitizing before entering patient rooms. So really different context.
在以自然主义方式中,人们按照日常生活的方式行动,习惯逐渐形成,并试图实际应用机器学习来探索,那么,这些习惯是如何形成的呢?底层因素是什么?需要多长时间?我们使用来自两个不同环境的数据集来进行研究,因为我们想要看到我们能得出的见解有多通用。一个是研究人们加入健身房时自然发生的习惯形成的数据,另一个是研究护理人员和医院在进入病房前如何形成手部消毒的习惯。所以,这是非常不同的背景。
Why those two areas? Is there something within the component of habit forming that is interesting about those two areas specifically? Well, gyms have been sort of the fruit fly of habit formation research. So an enormous amount of work in this literature focuses on gym attendance as the outcome and the reason is it's something that's quite easily to measure without requiring people to report to you and not lie. Their behavior rate. So when you enter gyms that's typically tracked, that's how they make money. They don't let people in who aren't members. And so this is very convenient. Unlike something like smoking or diet or nail biting, things that you might think of as natural candidates for habit research. The problem with those is somebody has to report to you, oh yeah, I've been my nail sayer. I didn't or I ate healthy today or I didn't. And people are often loath to do that truthfully. So gyms are really, that's like the workhorse. That's where a lot of habit research has done. And then we wanted to go beyond that and we were looking for a dataset that would similarly have objective measurement of behavior rather than self-report and realize there was a really interesting dataset that we could plumb that tracked these hospital caregivers and sanitizing decisions. And we thought that was really exciting to pair those two and see how different or similar are they.
为什么选择这两个领域?在习惯形成的组成部分中,这两个领域具有特别的有趣之处吗?好吧,健身房一直是习惯形成研究的“果蝇”。因此,这一领域的大量工作集中在健身房出勤率上,原因是出勤率是一项相对容易测量的指标,无需人们向你报告并保持真实性。他们的行为频率。通常在进入健身房时,成员的行为会被追踪,这也是他们赚钱的方式。他们不会让非会员进入。这非常方便。与吸烟、饮食或咬指甲等可能被视为习惯研究的自然候选对象不同,这些问题需要有人向你报告,比如,“是的,我咬指甲了”或“我今天吃得健康”等,人们通常不愿如实做出回答。所以健身房真的是固定的研究领域,习惯研究很多时候都在这里进行。然后我们想要超越这一点,寻找一个数据集,同样有客观行为测量而不是自我报告,并发现了一个非常有趣的数据集,可以追踪这些医院护理人员和消毒决策。我们觉得将这两者结合起来并观察它们的不同或相似之处非常令人兴奋。
So what were the results that you found out from looking at those two datasets? Well, I think the results are really surprising to many who have tried to what's a popular lay belief about a magic number of days that it takes to form a habit. So there's this widely spread rumor that it takes 21 days to form a habit. You may also have heard about 90 days to form a habit. There's popular books that count these numbers. By the way, don't have sound basis and research, but what we find is there is no such magic number and that the number of days it takes to form a habit, first of all, is quite different on average in these two samples, these two settings. So it's much faster. In general, to form a habit around hand sanitizing than gym going looks like it takes an order of magnitude sort of weeks on average in that context versus when we're looking at gym attendance, it takes months typically to form a habit. But there's also a huge variation across people. So it's not like this is a tight distribution. We can say, here's a magic number, even in a context. There's really widespread. So what that points to is this is really a false belief in the idea of a magic amount of time and probably we're better off focusing on things like how complex is the behavior, how often are you repeating what's the nature of the reward you're receiving as the main drivers of the speed as opposed to a gravitational pull towards a magic number.
那么你从查看这两个数据集中发现的结果是什么呢?嗯,我认为这些结果对于那些试图了解关于养成习惯需要多少天的流行观念的人来说确实是令人惊讶的。所以有一个广泛传播的传言说形成一个习惯需要21天。你可能也听说过需要90天养成一个习惯。有很多书都提到了这些数字。顺便说一下,并没有确凿的理论基础和研究,但我们发现并没有这样的神奇数字,而且在形成习惯所需的天数方面,在这两个样本,这两种情境中,平均而言是相当不同的。所以养成使用洗手液的习惯要快得多,总体来说,比去健身房的习惯要花掉大约一个数量级的时间,通常需要几个星期。但是不同的人之间也有很大的差异。所以并不是说这是一个严格的分布,我们可以说,这是一个神奇的数字,即使在一个特定的情境中也是如此。这实际上表明了这种关于时间的神奇观念是错误的,可能我们更应该关注的是行为的复杂性,你重复行为的频率以及你所获得的奖励的性质作为速度的主要驱动因素,而不是朝着一个神奇数字的引力。
How important then is this data in comparison to just thinking about habit formation in general in our culture, do you think? Well I think in general when we have data, it teaches us what's true as opposed to what we'd like to believe or what we think might be true based on observing ourselves.
在我们文化中,相比于一般的习惯形成,这个数据有多重要,你认为呢?我觉得一般来说,当我们拥有数据时,它能教会我们真相,而不是我们愿意相信或基于观察自己而认为可能是真相的东西。
So it was really exciting to bring 52 million observations to this question as opposed to question errors or people's intuitions who are writing self-help books. And we're really excited that we had an opportunity to do that here.
因此,将这5200万个观察结果带到这个问题中,而不是错误的问题或人们凭直觉写自助书,真是令人激动。我们非常高兴能有这个机会。
We're joined by Katie Milkman, Professor of Operations, Information and Decisions here at the Wharton School. Let me go back a second because you have done work in the past around gyms and those locations and the importance of that component to having a greater understanding of what is going on in this case around habit forming but just in general about how important you can develop and understand kind of moves that people make in those settings, correct? Yeah, absolutely, this is a really nice context.
我们这里有华顿商学院的运营、信息和决策教授Katie Milkman与我们一同参加。让我再回顾一下,因为你过去在健身房和这些场所方面做过研究,对于习惯形成的重要性以及了解人们在这些环境中做出的行为的重要性有更深刻的理解,是吗?是的,绝对没错,这是一个非常好的背景。
As I said, we think of it as like the fruit fly of habit research, being able to study the frequency at which people visit the gym, does it stick once people start going or does they fall off the wagon? It's a really nice setting and it's an important outcome from a policy perspective because actually about 9% of premature deaths are due to physical inactivity. It's an incredibly important factor in lifespan that many neglect but most are trying to habituate once they create a membership plan.
就像我之前所说的,我们将其视为习惯研究中的果蝇,可以研究人们去健身房的频率,一旦开始去了,是否会坚持下去,还是会放弃。这是一个非常好的环境,从政策的角度来看,这是一个重要的结果,因为实际上有大约9%的过早死亡是由于缺乏体育活动引起的。这是寿命中一个非常重要的因素,很多人忽视了它,但大多数人在创建会员计划后都在试图养成这个习惯。
What do you and your cohorts doing the research take from this research? Well, I think one thing that it shows us is the power of machine learning to explore patterns in human behavior in new ways that we couldn't do if we were just using standard old-fashioned methods. Survey data could be applied this way too. It's really exciting to see the objective data and to see that it can give us this kind of insight.
你和你的研究小组从这项研究中得出了什么结论?嗯,我认为其中一个结论就是机器学习在探索人类行为模式方面的能力。这种探索方式是我们使用传统老旧方法无法做到的。调查数据也可以以这种方式应用。看到客观数据并且能从中获得这种洞察力真的很令人兴奋。
We're fitting individualized models with this data and just learning a lot about what are the contextual factors that shape habit formation, how fast does it happen? So one, I'm really excited about machine learning.
我们正在使用这些数据进行个性化模型拟合,并且正在学习关于形成习惯的环境因素,以及它发生的速度有多快。因此,我对机器学习非常感兴趣。
A second thing that we take away that I haven't talked about besides this idea that there's no magic number is actually we also see that something happens with habits called reward devaluation which is that we had a point in time when lots of the people who we were studying who had been going to the gym started getting offers of programming to help them exercise more regularly.
除了我之前提到的没有魔法数字的概念,我们还可以得出另一个结论,即习惯也会发生奖励贬值的现象。在我们研究的人群中,曾经常去健身房的人开始接到一些有助于他们更有规律地进行锻炼的计划。
We see that the people who are models call quite habituated who are models say like they've reached a habit. Those people are actually much less sensitive to those kinds of offers and efforts to help them change their behavior than people who are not yet in this predictable habituated mode according to those models. So that's also I think really interesting and it's a big takeaway that we've seen this in animal models before but showing that once a person forms a habit it's really hard to change their direction we can predict with machine learning when they're in that state and then we know once they've reached that state they're going to be harder to perturb off of their course.
我们发现那些作为模型的人,可以说已经完全习惯于自己的模式,他们表达出已经养成了一种习惯。相较于那些还未进入这种可预测的习惯模式的人,他们对于改变自己行为的种种提议和努力都不太敏感。这也是我认为非常有趣并且很重要的一点,我们之前在动物模型中也观察到过这一现象,即一旦一个人养成了一种习惯,要改变他们的方向就变得非常困难,我们可以通过机器学习预测他们是否处于这种状态,一旦他们达到这种状态,就很难使他们偏离原来的轨道了。
So that's also important just for thinking about tailoring and personalizing the kind of offers we provide if you're a marketer, a health care plan, an employer. So I think that's really interesting too.
所以,这对于考虑定制和个性化我们提供的优惠对于市场营销人员、医疗保健计划和雇主也很重要。所以我觉得这点也非常有意思。
It sounds like this is also a little bit of a pivot moment as well from what machine learning can really give you as you move forward and you just kind of laid out of all the different potential impacts that are out there from having machine learning kind of going through a lot of this data.
听起来,这也是一个有点转折的时刻,随着你前进,机器学习真的可以给你带来什么,你列举了机器学习处理大量数据可能产生的各种潜在影响。
I think that's right. It's really exciting and you know when we think about what's the biggest contribution of this paper I think you know for most readers they'll be excited to learn there's not a magic number and that this personalization of you know sending offers to people differentially based on whether a model says they're habituated or not that adds value. Those are big practical takeaways but a really big and exciting takeaway for the scientific community and frankly for practitioners as well is the power of machine learning applied in this way to try to model habit and to tell us interesting things we might not have been able to learn otherwise about the nature of habit. So I think that's really promising.
我认为这是正确的。这真的令人兴奋,你要知道,当我们考虑这篇论文的最大贡献时,我认为对于大多数读者来说,他们会兴奋地了解到没有一个神奇数字,以及通过模型根据人们是否习惯于发送优惠的个性化方法增加了价值。这些都是实用的重要得出结论,但对于科学界和从业者来说,应用机器学习来建模习惯和告诉我们有趣的东西可能是我们在其他情况下无法学到的关于习惯的本质的力量,这是一个非常重要且令人兴奋的结论。因此,我认为这非常有前途。
Are there other areas that as you have now done this work that you are interested to find out to see how machine learning kind of parses other data sets in other areas as well? Oh my goodness I mean so many. There's so many opportunities you know obviously one of the big hot areas is medical decision making and I think that's so important I do a lot of research on medical decision making and the opportunity to combine human intelligence with machine intelligence the better model decisions and improve them is so exciting because so many lives can be saved.
现在您已经完成了这项工作,是否对其他领域也感兴趣,想看看机器学习如何对其他领域的数据集进行解析?哦天哪,我是说有很多领域可以研究。机会真的很多,你知道的,其中一个热门领域就是医疗决策,我认为这非常重要。我做了很多关于医疗决策的研究,结合人类智能和机器智能来进行决策,并对其进行改进,这样做的机会是如此令人兴奋,因为这可以挽救很多生命。
So that's where I feel most eager but I think there's enormous creative potential here and we're just starting to scratch the surface.
所以这就是我最渴望的地方,但我认为这里有巨大的创造潜力,而我们只是刚刚开始探索表面而已。
Katie always great to have you on the show thanks very much for your time enjoy the rest of your day.
Katie,很高兴你能来参加节目,非常感谢你花时间,祝你度过愉快的一天。
Thank you thanks for having me it was a pleasure.
谢谢你邀请我,我很高兴能够参与。
You got a Katie Milkman professor of operations information and decisions at the Wharton School and author of the recently released book How to Change.
你接受了凯蒂·米尔克曼的邀请,她是沃顿商学院运营信息与决策专业的教授,同时也是最近出版的《如何改变》一书的作者。
To keep engaged with Wharton Business Daily and other Wharton School shows visit business radio dot Wharton dot u pen dot edu.
保持与沃顿商业日报和其他沃顿商学院节目的联系,请访问business radio dot Wharton dot u pen dot edu。