NVidia 2023 Q1 Earnings Call
发布时间 2023-05-26 20:05:30 来源
中英文字稿 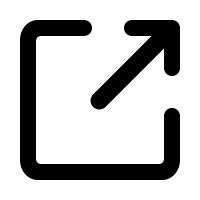
Good afternoon. My name is David and I'll be your conference operator today. At this time, I'd like to welcome everyone to Nvidia's first quarter earnings call. Today's conference is being recorded. All lines have been placed on mute to prevent any background noise. After the speakers are marked, there'll be a question and answer session. If you'd like to ask a question during this time, simply press C star key, follow by the number one on your telephone keypad. If you'd like to withdraw your question, press star one once again.
下午好,我叫David,我将是今天的会议主持人。现在,我想欢迎大家参加Nvidia公司的第一季度财报电话会议。本次会议正在录音。为了防止背景噪音,所有线路都已被静音。演讲者发言后,将进行问答环节。如果您想在此期间提问,请简单地按下电话键盘上的[C*]键,然后再按一次数字[1]键。如果您想撤回问题,请再次按下星号[1]键。
Thank you, Samona, Jen Kowski. You may begin your conference.
谢谢,Samona,Jen Kowski。你们可以开始你们的会议了。
意思是:谢谢 Samona 和 Jen Kowski,可以开始他们的会议了。
Thank you. Good afternoon, everyone. And welcome to Nvidia's conference call for the first quarter of fiscal 2024. With me today's human video, our gentleman, President and Chief Executive Officer, and collect CREF, Executive Vice President and Chief Financial Officer.
大家下午好,感谢各位参加英伟达2024财年第一季度电话会议。今天与我们一起的是亲切可爱的总裁兼首席执行官,以及聪明机智的执行副总裁兼首席财务官。
I'd like to remind you that our call is being webcast live on Nvidia's Investor Relations website. The webcast will be available for replay until the conference call to discuss our financial results to the second quarter of fiscal 2024. The content of today's call is in video's property. It can be reproduced or transprived without our prior written consent.
我想提醒您,我们的电话会议正在Nvidia投资者关系网站上进行现场网络直播。该网络直播可以重播,直到我们讨论2024财年第二季度财务成果的电话会议为止。今天的呼叫内容属于视频的财产。未经我们事先书面同意,不得复制或转录。
During this call, we may make four lives in statements, based on current expectations. These are subject to a number of significant risks and uncertainties, and our actual results may differ materially. For discussion of factors that could affect our future financial results in business, please refer to the disclosure in today's earnings release. Our most recent forms 10K and 10Q, and the report that we may file on form 8K with the Securities and Exchange Commission.
在本次通话中,我们可能会基于目前的预期做出四项业务陈述。然而这些陈述面临着许多重大风险和不确定性,实际结果可能发生重大差异。如果您想进一步讨论可能会影响我们未来财务结果的因素,请参考今天财报中的披露。您也可以查阅我们最近的10K和10Q报表以及我们可能向证券交易委员会提交的8K报告。
All our statements are made as of today, May 24, 2023, based on information currently available to us. Except it's required by law, we assume no obligation to update any such statements.
我们所有的声明均基于目前我们所掌握的信息,截止于2023年5月24日。除非法律要求,我们不承担更新任何此类声明的义务。
During this call, we will discuss non-guessful measure. You can find a reconciliation of these non-guessful measure to guessful measure in our CFO commentaries, which is posted on our website.
这次电话会议中,我们将讨论非猜测性指标。您可以在我们CFO的评论中找到这些非猜测性指标与猜测性指标的调和,该评论已发布在我们的网站上。
And with that, let me turn the call over to Collette.
同时,请允许我将电话转交给Collette。
Thanks, Simona.
谢谢,Simona。
Q1 revenue with 7.19 billion, up 19% sequentially, and down 13% year on year. Unsequential growth was driven by record data center revenue, with our gaming and professional visualization platforms emerging from channel inventory corrections.
Q1营收为71.9亿美元,环比增长19%,同比下降13%。非连续增长受到记录性数据中心营收的推动,我们的游戏和专业可视化平台从渠道库存纠正中脱颖而出。
Starting with data center, record revenue of 4.28 billion was up 18% sequentially and up 14% year on year. Unstrong growth were accelerated computing platforms worldwide. Creative AI is driving exponential growth in compute requirements and a fast transition to Nvidia accelerated computing, which is the most versatile, most energy efficient, and the lowest TCO approach to train and deploy AI. Generative AI draws significant upside in demand for our products, creating opportunities and broad-based global growth across our markets.
从数据中心开始,我们的营收记录为42.8亿美元,环比增长18%,同比增长14%。全球加速计算平台的强劲增长进一步加速了这一趋势。创意AI推动了计算需求的指数级增长,并加速了向最全能、最节能、最低TCO的Nvidia加速计算的转变,以用于训练和部署AI。生成式AI在我们的产品需求上有巨大的增长潜力,为我们带来机遇,并在我们的所有市场中创造了广泛的全球增长。
Let me give you some color across our three major customer categories, called service providers or CSPs, consumer internet companies, and enterprises. First, CSPs around the world are racing to deploy our flagship hopper and ampere architecture GPUs to meet the surge in interest from both enterprise and consumer AI applications for training and inference. Global CSPs announce the availability of H100 on their platforms, including private previews at Microsoft Azure, Google Cloud, and Oracle Cloud infrastructure, upcoming offerings at AWS and general availability at emerging GPU specialized cloud providers like Core Reef and Lambda.
让我向您介绍我们三个主要客户类别的情况,包括服务提供商(CSP)、消费互联网公司和企业。首先,世界各地的CSP正在竞相部署我们的旗舰产品——Hopper和Ampere架构的GPU,以满足企业和消费者在训练和推理方面越来越多的AI应用的需求。全球CSP宣布在其平台上提供H100,包括在Microsoft Azure、Google Cloud和Oracle Cloud基础设施上进行的私人预览,以及即将在AWS上提供和在新兴的GPU专业云提供商,如Core Reef和Lambda上进行的一般可用性。
In addition to enterprise AI adoption, these CSPs are serving strong demand for H100 from generative AI pioneers. Second, consumer internet companies are also at the forefront of adopting generative AI and deep learning-based recommendation systems, driving strong growth. For example, Meta has now deployed its H100 powered brand Teton AI supercomputer for its AI production and research teams.
除了企业人工智能采用外,这些通信服务提供商还为生成式人工智能先驱的H100需求提供了强有力的支持。其次,消费者互联网公司也处于采用生成式人工智能和基于深度学习的推荐系统的前沿,推动了强劲增长。例如,Meta现在已经为其人工智能生产和研究团队部署了H100强力驱动的品牌Teton AI超级计算机。
Third, enterprise demand for AI and accelerated computing is strong. We are seeing momentum in verticals such as automotive, financial services, healthcare, and telecom where AI and accelerated computing are quickly becoming integral to customers, innovation, road maps, and competitive positioning. For example, Bloomberg announced it has a 50 billion parameter model, Bloomberg GPT, to help with financial, natural language processing tasks, such as sentiment analysis, named entity recognition, news classification, and question answering. Auto Insurance Company, CCC intelligence solutions, is using AI for estimating repairs. And AT&T is working with us on AI to improve fleet dispatches so their field technicians can better serve customers. Among other enterprise customers using NVIDIA AI are devoid for logistics and customer service and AMGIM for drug discovery and protein engineering.
第三,企业对AI和加速计算的需求强烈。我们正在看到汽车、金融服务、医疗保健和电信等领域的势头,AI和加速计算正在迅速成为客户、创新、路线图和竞争定位的关键。例如,彭博社宣布拥有一个500亿个参数的模型,彭博GPT,帮助金融和自然语言处理任务,如情感分析、命名实体识别、新闻分类和问题回答。汽车保险公司CCC智能解决方案正在使用AI进行估算修复。而AT&T正在与我们合作,利用AI来改善车队派遣,使其现场技术人员能够更好地为客户服务。除了物流和客户服务方面的Devoid公司和药物发现和蛋白质工程方面的AMGIM公司,还有其他企业客户正在使用NVIDIA AI。
This quarter, we started shipping DGX H100, our Hopper Generation AI system, which customers can deploy on-prem. And with the launch of DGX Cloud through our partnership with Microsoft Azure, Google Cloud and Oracle Cloud infrastructure, we deliver the promise of NVIDIA DGX to customers from the cloud. Whether the customers deploy DGX on-prem or via DGX Cloud, they get access to NVIDIA AI software, including NVIDIA base command and AI frameworks and pre-trained models.
本季度,我们开始发货DGX H100,我们的Hopper Generation AI系统,客户可以在其本地部署。通过与Microsoft Azure,Google Cloud和Oracle Cloud基础架构的合作伙伴关系,我们推出了DGX Cloud,向客户提供了NVIDIA DGX的承诺。无论客户是在本地部署DGX还是通过DGX Cloud,他们都可以使用NVIDIA AI软件,包括NVIDIA base command和AI框架以及预训练模型。
We provide them with a blueprint for building and operating AI, spanning our expertise across systems, algorithms, data processing, and training methods. We also announced NVIDIA AI Foundations, which are model foundry services available on DGX Cloud that enable businesses to build, refine, and operate custom large language models and generative AI models trained with their own proprietary data, created for unique domain specific tasks.
我们向他们提供了一份建立和运营AI的蓝图,涵盖了我们在系统、算法、数据处理和训练方法方面的专业知识。此外,我们还宣布了NVIDIA AI Foundations,这是在DGX Cloud上提供的模型工厂服务,使企业能够构建、精制和操作具有自己专有数据的自定义大语言模型和生成式AI模型,以完成独特的特定领域任务。
They include NVIDIA Nemo for large language models, NVIDIA Picasso for images, NVIDIA and 3D, and NVIDIA BioNemo for life sciences. Each service has six elements, pre-trained models, frameworks for data processing and configuration, proprietary knowledge-based vector databases, systems for fine-tuning, aligning, and guard railing, optimized inference engines, and support from NVIDIA experts to help enterprises fine-tune models for their custom use cases.
这些服务包括 NVIDIA Nemo 用于大型语言模型、NVIDIA Picasso 用于图像、NVIDIA 和 3D、以及 NVIDIA BioNemo 用于生命科学。每项服务都有六个组成部分,包括预训练模型、数据处理和配置框架、基于专有知识的向量数据库、微调、调整和安全保障系统、优化后的推理引擎,以及 NVIDIA 专家的支持,以帮助企业优化模型以适应其自定义用例。
Service Nemo, a leading enterprise services platform is an early adopter of DGX Cloud and Nemo. They are developing custom large language models trained on data specifically for the service-nile platform. Our collaboration will let service-nile create new enterprise-grade generative AI offerings for the thousands of enterprises worldwide running on the service-nile platform, including for IT departments, customer service teams, employees, and developers.
Service Nemo是一家领先的企业服务平台,已成为DGX Cloud和Nemo的早期采用者。他们正在开发针对service-nile平台的特定数据进行训练的自定义大语言模型。我们的合作将使service-nile创建新的面向企业级生成式AI服务,应用于全球数千家运行在service-nile平台上的企业,包括IT部门、客户服务团队、员工和开发人员。
NVIDIA AI is also driving a step-function increase in inference work modes, because of their size and complexity, these workflows require acceleration. The latest ML Perk Industry benchmark released in April showed NVIDIA's inference platforms deliver performance that is orders of magnitudes ahead of the industry, with unmatched versatility across diverse work modes.
NVIDIA的AI技术还推动了推断工作模式的跨越式增长,由于其大小和复杂性,这些工作流需要加速。今年四月发布的最新ML Perk Industry基准测试显示,NVIDIA的推断平台在性能方面远远超过行业水平,具有在不同的工作模式下无与伦比的多样性。
To help customers deploy generative AI applications at scale, at GTC, we announced four major new inference platforms that leverage the NVIDIA AI software stack. These include L4, Tensor Core GPU for AI video, L40 for omniverse and graphics rendering, H100NBL for large language models, and the Grace Hopper Superchip for LLMs, and also recommendation systems and vector databases. Google Cloud is the first CSP to adopt our L4 inference platform with the launch of its G2 virtual machines for generative AI inference and other work modes, such as Google Cloud Data Proc, Google Alpha Fold, and Google Cloud Immersive Stream, which render 3D and AR experiences.
在GTC上,我们宣布了四个全新的推理平台,利用英伟达AI软件堆栈,为帮助客户在规模上部署生成式AI应用做出了贡献。其中包括L4、Tensor Core GPU(适用于AI视频)、L40(用于Omniverse和图形渲染)、H100NBL(适用于大型语言模型)和Grace Hopper超级芯片(适用于LLMs、推荐系统和向量数据库)。谷歌云是第一个采用我们的L4推理平台的云服务提供商,通过推出其G2虚拟机为生成式AI推理和其他工作模式,如Google Cloud Data Proc、Google Alpha Fold和Google Cloud Immersive Stream提供3D和AR体验。
意思是:英伟达公司为帮助客户在规模上部署生成式AI应用提供了四个新的推理平台。其中包括适用于AI视频、Omniverse和图形渲染、大型语言模型以及LLMs、推荐系统和向量数据库的Grace Hopper超级芯片。谷歌云是第一个采用L4推理平台的云服务提供商,提供生成式AI推理和其他工作模式,如Google Cloud Data Proc、Google Alpha Fold和Google Cloud Immersive Stream,为用户提供3D和AR体验。
In addition, Google is integrating our Triton inference server with Google Kubernetes engine and its cloud-based, MIRTEX AI platform. In networking, we saw strong demand at both CSPs and enterprise customers for generative AI and accelerated computing, which require high performance networking, like in videos and melanox networking platforms, demand relating to general purpose CPU infrastructure remains soft.
此外,Google正在将我们的Triton推理服务器与Google Kubernetes引擎和其云基础的MIRTEX AI平台集成。在网络方面,我们看到CSP和企业客户对生成AI和加速计算的强烈需求,需要高性能网络,例如视频和Mellanox网络平台,而与通用CPU基础架构相关的需求仍然较弱。
As generative AI applications grow in size and complexity, high performance networks become essential for delivering accelerated computing at data center scale to meet the enormous demands of both training and inferencing. Our 400GIG Quantum 2 Infinitban platform is the gold standard for AI dedicated infrastructure with broad adoption across major cloud and consumer internet platforms, such as Microsoft Azure.
随着生成AI应用程序规模和复杂度的增长,高性能网络成为数据中心规模的加速计算必不可少,以满足训练和推断的巨大需求。我们的400GIG Quantum 2 Infinitban平台是AI专用基础设施的黄金标准,受到了主要云和消费者互联网平台的广泛采用,例如Microsoft Azure。
With the combination of in-network computing technology and the industry's only end-to-end data center scale, optimized software stack, customers routinely enjoy a 20% increase in throughput for their sizeable infrastructure and mess line. Our multi-tenant cloud transitioning to support generative AI, our high speed Ethernet platform with Bluefield 3, DPUs, and Spectrum 4 Ethernet switching offers a highest available Ethernet network performance.
通过网络计算技术和行业中唯一的端到端数据中心规模优化软件堆栈的结合,客户通常可以享受到对于其庞大基础设施和混合线路的 20% 的吞吐量增加。我们的多租户云正在转型以支持生成式人工智能,我们的高速以太网平台配备 Bluefield 3、DPU和Spectrum 4 以太网交换机,提供了最高可用的以太网网络性能。
Bluefield 3 is in production and has been adopted by multiple hyperscale and CSP customers, including Microsoft, Azure, Oracle Cloud, PowerWeaf, BIDU, and others. We look forward to sharing more about our 400GIG Spectrum 4 accelerated AI networking platform next week at the Computex conference in Taiwan.
Bluefield 3已进入生产,并被多个超大规模和云服务提供商客户采用,包括微软、Azure、Oracle Cloud、PowerWeaf、BIDU等。我们期待下周在台湾的Computex会议上分享更多关于我们的400G Spectrum 4加速AI网络平台的信息。
Lastly, our Grace Data Center CPU is sampling with customers. At this week's International Supercomputing Conference in Germany, the University of Bristol announced a new supercomputer based on the NVIDIA Grace CPU Superchip, which is 6X more energy efficient than the previous supercomputer. This adds to the growing momentum for Grace with both CPU only and CPU GPU opportunities across AI and cloud and supercomputing applications.
最后,我们的Grace数据中心CPU正在与客户进行采样。在德国本周举行的国际超级计算大会上,布里斯托大学宣布了一款基于NVIDIA Grace CPU超级芯片的新超级计算机,比之前的超级计算机节能6倍。这增加了与CPU GPU机会相关的AI、云和超级计算应用中的Grace的增长动力。
The coming way of Bluefield 3, Grace, and Grace Hopper Superchips will enable a new generation of super energy efficient accelerated data centers.
Bluefield 3、Grace 和 Grace Hopper 超级芯片即将到来,将使得新一代超级高效的加速数据中心成为可能。
Now, let's move to gaming. Gaming revenue of $2.24 billion was up 22% sequentially and down 38% year on year. Strong sequence of growth was driven by sales of the 40 series GeForce RTX GPUs for both notebooks and dust tops. Overall, and demand was solid and consistent with seasonality demonstrating resilience against a challenging consumer spending backdrop.
现在我们来谈谈游戏。游戏收入为22.4亿美元,环比增长22%,同比下降38%。这种强劲的增长趋势是由于40系列GeForce RTX GPU在笔记本电脑和台式机上的销售推动的。总体而言,需求良好,并且符合季节性,表现出对挑战性消费支出环境的韧性。
The GeForce RTX 40 series GPU laptops were off to a great start, featuring four NVIDIA inventions, RTX Path Tracing, DLSS3 AI rendering, Reflex Ultra-Low Latency rendering, and MAX Q, energy efficient technologies. They deliver tremendous gains in industrial design, performance, and battery life for gamers and creators. And like our desktop offerings, 40 series laptops support the NVIDIA Studio platform of software technologies, including acceleration for creative data science and AI workflows, and omniverse, giving content creators unmatched tools and capabilities.
GeForce RTX 40系列GPU笔记本电脑开端良好,拥有四项NVIDIA发明,即RTX路径追踪、DLSS3人工智能渲染、Reflex Ultra-Low Latency渲染和MAX Q节能技术。它们为游戏玩家和创作者提供了极大的工业设计、性能和电池寿命的收益。与我们的桌面产品一样,40系列笔记本电脑支持NVIDIA Studio平台的软件技术,包括创造性数据科学和AI工作流程的加速,以及omniverse,为内容创作者提供无与伦比的工具和能力。
In desktop, we ramped the RTX 4070, which joined the previously launched RTX 4090, 4080, and the 4070 TI GPUs. The RTX 4070 is nearly 3x faster than the RTX 2070, and offers our large install base expect tax in our upgrade. Last week, we launched the 60 family, RTX 4060 and 4060 TI, bringing our newest architecture to the world's core gamers, starting at just $299. These GPUs, for the first time, provide two extra performances of the latest gaming console at mainstream price points. The 4060 TI is available starting today, while the 4060 will be available in July.
我们在台式机中推出了RTX 4070,它加入了之前推出的RTX 4090、4080和4070 TI GPU。RTX 4070比RTX 2070快近3倍,并且为我们庞大的安装基础提供了预期的税务升级。上周,我们推出了RTX 4060和4060 TI的60系列,将我们最新的架构带给了全球核心玩家,起价只有299美元。这些GPU首次在主流价格点提供了两个最新游戏机的额外性能。4060 TI从今天起就可用,而4060将在7月推出。
Generative AI will be transformative to gaming and content creation from development to runtime. At the Microsoft Build Developer Conference earlier this week, we showcased how Windows PCs and work stations within video RTX GPUs will be AI powered at their core. NVIDIA and Microsoft have collaborated on end-to-end software engineering, spanning from the Windows operating system to the NVIDIA graphics drivers, and Nemo, LLF Framework, to help make Windows on NVIDIA RTX 10-SERCOR GPUs a supercharged platform for generative AI.
生成式人工智能将对游戏和内容创作从开发到运行都带来革命性的影响。在本周早些时候的微软Build开发者大会上,我们展示了Windows计算机和视频RTX GPU将以人工智能为核心驱动的情景。NVIDIA和微软在从Windows操作系统到NVIDIA图形驱动和Nemo、LLF Framework等各方面进行了端到端软件工程方面的合作,帮助使Windows在NVIDIA RTX 10-SERCOR GPU上成为生成式人工智能的超强平台。
Last quarter, we announced a partnership with Microsoft to bring Xbox PC games to GeForce now. The first game from this partnership, Gears 5, is now available with more set to be released in the coming months. There are now over 1,600 games on GeForce now, the richest content available on any gaming service.
上个季度,我们宣布与微软合作,将 Xbox PC 游戏带到 GeForce now 平台。这个合作伙伴关系的第一个游戏《战争机器 5》现已上线,今后几个月还会有更多游戏推出。目前,GeForce now 平台上已有超过1,600款游戏,是所有游戏服务中最丰富的内容。
Moving to Pro Visualization. Revenue of $295 million was up 31% sequentially and down 53% year on year. Supprential growth was driven by stronger workstation demand across both mobile and desktop platform factors, with strength in key verticals such as public sector, healthcare, and automotive. We believe the channel inventory correction is behind us. The ramp of our ADA-lovelace GPU architecture in work stations kicks off a major product cycle. At GTC, we announced six new RTX GPUs for laptops and desktop workstations with further rollouts planned in the coming quarters.
转向专业可视化领域。 收入为2.95亿美元,较上一季度增长31%,同比下降53%。这是由于移动和桌面平台因素对更强的工作站需求的推动,尤其是公共部门,医疗保健和汽车等重要垂直行业的强劲表现。我们相信通道库存纠正已经结束。我们的ADA-Lovelace GPU架构在工作站上的推广引发了一次重大产品周期。在GTC上,我们宣布推出6款新的RTX笔记本电脑和桌面工作站GPU,计划在未来几个季度进行更进一步的推广。
Generative AI is a major new workload for Nvidia powered workstation. Our collaboration with Microsoft transforms Windows into the ideal platform for creators and designers, harnessing Generative AI to elevate their creativity and productivity. At GTC, we announced Nvidia Omniverse Cloud and Nvidia fully managed service running in Microsoft Azure that includes the full suite of Omniverse applications and Nvidia OVX infrastructure. Using this full stack cloud environment, customers can design, develop, deploy, and manage industrial metaverse applications. Nvidia Omniverse Cloud will be available starting in the second half of this year. Microsoft Nvidia will also connect Office 365 applications with Omniverse.
生成式人工智能是Nvidia驱动的工作站的重要新工作负载。我们与微软的合作将Windows转变成为创作者和设计师的理想平台,利用生成式人工智能来提升他们的创造力和生产力。在GTC上,我们宣布了Nvidia Omniverse Cloud和在Microsoft Azure上运行的Nvidia全管服务,其中包括Omniverse应用程序的全套和Nvidia OVX基础设施。使用这个全套云环境,客户可以设计、开发、部署和管理工业元宇宙应用程序。Nvidia Omniverse Cloud将于今年下半年开始推出。Microsoft Nvidia还将连接Office 365应用程序与Omniverse。
Omniverse Cloud is being used by companies to digitize their workflows from design and engineering to smart factories and 3D content generation from our team. The automotive industry has been a leading early adopter of Omniverse, including companies such as the MW Group, Yili Lotus, General Motors, and Jaguar Land Rover.
公司正在使用Omniverse Cloud将其从设计和工程到智能工厂和三维内容生成的工作流数字化。汽车行业一直是Omniverse的领先早期采用者,其中包括宝马集团、伊利莲花、通用汽车和捷豹路虎等公司。
Moving to automotive. Omniverse was 296 million up 1% sequentially and up 114% from a year ago. Our strong year on your growth was driven by the ramp of the Nvidia Drive, Orrin, across a number of new energy vehicles. As we announced in March, our automotive design went pipeline over the next six years, now it's down at 14 billion, up 11 billion a year ago, giving us visibility into continued growth over the coming years.
转向汽车行业。Omniverse的销售额为2.96亿美元,环比增长1%,同比增长114%。我们强劲的年度增长主要由Nvidia Drive Orrin在多款新能源车型中的推广所推动。正如我们在三月份宣布的那样,我们的汽车设计业务在未来六年内将获得14亿美元的订单,比一年前增长了110亿美元,这为我们未来的持续增长带来了可见性。
Sequentially, growth moderated as some of these customers in China are adjusting their production schedules to reflect slower than expected demand growth. We expect this dynamic to linger for the rest of the calendar year.
接下来,由于中国的一些客户正在调整他们的生产计划以反映比预期更慢的需求增长,增长逐渐放缓。我们预计这种形势在接下来的日历年中会持续存在。
During the quarter, we expanded our partnership with BID, the world's leading manufacturer of NIVs. Our new design win will extend BID's use of the Drive, Orrin, to its next generation, high volume, dynasty, and ocean series of vehicles set to start production in calendar 2024.
在本季度,我们与世界领先的NIV制造商BID扩展了我们的合作关系。我们的新设计突破将延伸BID对Drive、Orrin的使用,扩展至其即将于2024年开始投产的下一代、高产量、王朝和海洋系列车辆中。
Moving to the rest of the P&L, Gaprose margins were 64.6%, non-Gaprose margins were 66.8%, Gaprose margins have now largely recovered to prior peak levels, as we have absorbed higher costs and offset them by innovating and delivering higher valued products as well as products incorporating more and more software.
在P&L的其他部分中,Gaprose的利润率为64.6%,非Gaprose的利润率为66.8%,Gaprose的利润率现在已经大部分恢复到之前的峰值水平,我们通过创新和提供更高价值的产品以及融合越来越多的软件的产品来吸收更高的成本并抵消它们。
Sequentially, Gap operating expenses were down 3%, and non-Gap operating expenses were down 1%. We have held up X at roughly the same level over the last past four quarters, while working through the inventory corrections in gaming and professional visualization.
顺序地说,Gap的运营费用下降了3%,非Gap的运营费用下降了1%。在过去的四个季度中,我们一直将X维持在大致相同的水平,同时在游戏和专业可视化方面进行库存纠正。
简单易懂地说,Gap的运营成本降低了3%,而非Gap的运营成本降低了1%。在过去的四个季度中,我们一直维持着X的稳定水平,同时纠正了游戏和专业可视化方面的库存问题。
We now expect to increase investments in the business while also delivering operating leverage. We returned 99 million to shareholders in the form of cash dividends. At the end of the Q1, we have approximately 7 billion remaining under our share report of visualization through December 2023.
我们目前预计会增加对业务的投资,同时实现运营杠杆效应。我们通过现金股利向股东返还了9900万美元。在第一季度结束时,我们在2023年12月份之前的股票回购中还剩大约70亿美元。
Let me turn to the outlook for the second quarter of fiscal 2016. Total revenue is expected to be 11 billion, plus or minus 2%. We expect this sequential growth to largely be driven by data center, reflecting a steep increase in demand related to generative AI and large language models. This demand has extended our data center visibility out a few quarters, and we have procured substantially higher supply for the second half of the year.
让我谈一下2016财年第二季度的前景。总收入预计将达到110亿美元,误差范围在正负2%之间。我们预计这种连续增长主要是由数据中心驱动的,这是由于生成式人工智能和大型语言模型需求的急剧增加所致。这种需求扩展了我们对数据中心的看法,我们为下半年采购了大量的供应。
Gap and non-Gap gross margins are expected to be 68.6% and 70% respectively, plus or minus 50 basis points. Gap and non-Gap operating expenses are expected to be approximately 2.71 billion and 1.9 billion respectively. Gap and non-Gap other income and expenses are expected to be an income of approximately 90 million, excluding gains and losses from non-affiliated investments. Gap and non-Gap tax rates are expected to be 14%, plus or minus 1%, excluding any discrete items.
预计Gap和非Gap毛利率分别为68.6%和70%,误差在50个基点以内。预计Gap和非Gap运营费用分别为约27.1亿和19亿。预计Gap和非Gap其他收入和支出将约为9,000万美元的收入,不包括非附属投资的收益和损失。预计Gap和非Gap所得税税率将为14%左右,不包括任何离散项目。
Several expenditures are expected to be approximately 300 to 350 million. Further financial details are included in the CFO commentary and other information available on our IR website, including let me highlight some of the upcoming events.
预计有几笔支出将达到约3亿至3.5亿美元。财务细节将在首席财务官的评论和我们IR网站上提供的其他信息中详细说明,包括接下来的一些重要活动。
Jensen will give the compute tax keynote address in person in Taipei this coming Monday, May 29 local time, which will be Sunday evening in the U.S. In addition, we will be attending the BFA Global Technology Conference in San Francisco on June 6th and Rosenblatt Virtual Technology Summit on the age of AI on June 7th and the new Street Future of Transportation Virtual Conference on June 12th.
Jensen 将于本地时间5月29日星期一亲自在台北发表计算税演讲,美国当地时间将是当天晚上。此外,我们还将参加6月6日在旧金山举行的BFA全球科技会议,6月7日的罗森布拉特虚拟技术峰会,以及6月12日的新街道未来交通虚拟会议。
Our earnings call to discuss the revolts of our second quarter fiscal 24 is scheduled for Wednesday, August 23rd. Well, as covers our opening remarks, we're now going to open the calls for questions.
我们的二季度财政24年度收益电话会议定于8月23日星期三讨论。那么,接下来是我们的开场白,我们现在要开放提问环节。
Operator, would you please call for questions? Thank you.
操作员,请您发问。谢谢。
At this time, I'd like to remind everyone in order to ask a question, press star than the number one on your telephone keypad. We ask that you please limit yourself to one question.
此时,我想提醒大家,如果您想要提问,请按下电话键盘上的星号键和数字一键。我们要求您只提出一个问题。请您配合我们。
We'll pause for just a moment to compile the Q&A roster.
我们将暂停片刻来编制问答名单。这意味着我们会停止讨论一段时间,对话框中会出现一个名单,其中列出了问题和答案,以便更好地组织和梳理信息。
We'll take our first question from Tishia Hari with Goldman Sachs. Your line's open.
我们首先接受Goldman Sachs的Tishia Hari提出的问题。你可以开始提问了。
Hi, good afternoon. Thank you so much for taking the question and congrats on the strong results and incredible outlook.
您好,下午好。非常感谢您回答我们的问题,恭喜您取得了强劲的结果和令人难以置信的前景。
Just one question on data center. Collette, you mentioned the vast majority of the sequential increase in revenue this quarter will come from data center. I was curious what the construct is there. If you can speak to what the key drivers are from April to July. Perhaps more importantly, we talked about visibility into the second half of the year. I'm guessing it's more of a supply problem at this point. What kind of sequential growth beyond the July quarter can your supply chain support at this point?
只有一个问题与数据中心有关。Collette,你提到本季度绝大多数连续增长的收入将来自数据中心,我很好奇这是如何构建的。如果你能够谈谈4月到7月的关键驱动因素,那就更重要了。也许更重要的是,我们谈到了对今年下半年的可见性。我猜现在这更多是供应问题。你的供应链现在支持7月季度以后的连续增长是多少?
Thank you.
谢谢你。意思是表示感谢或感激之情。
Okay, so a lot of different questions there. So let me see if I can start and I'm sure Jensen will have some following up comments. So when we talk about our sequential growth that we're expected between Q1 and Q2, our generative AI, large language models are driving this surge into math. And it's a broad based across both our consumer internet companies, our CSPs, our enterprises and our AI startups.
好的,这里有很多不同的问题。让我看看我能否开始解答,我相信Jensen会有一些跟进的评论。所以当我们谈论我们在第一季度和第二季度之间期望的顺序增长时,我们的生成式人工智能、大型语言模型正在推动这个数学潮流。这是广泛基于我们的消费互联网公司、我们的CSP(云服务提供商)、我们的企业和我们的人工智能创业公司的。
It is also interest in both of our architectures, both of our hopper latest architecture as well as our ampere architecture. This is not surprising as we generally often sell both of our architectures at the same time. This is also a key area where deep recommendators are driving growth and we also expect to see growth both in our computing as well as in our networking business.
客户对我们的两种架构——最新的漏斗架构和安培架构——都表现出浓厚的兴趣并不令人惊讶,因为我们通常会同时销售这两种架构。深度推荐系统是推动我们的计算和网络业务增长的重要领域,因此我们也预计在这些领域会看到增长。
So those are some of the key things that we have baked in when we think about the guidance that we've provided to Q2.
这些都是我们在提供第二季度指导时考虑的关键因素之一。
We also surfaced in our opening remarks that we are working on both supply today for this quarter, but we have also procured a substantial amount of supply for the second half.
我们在开场白中也提到,我们正在为本季度的供应工作,但我们也已经采购了大量的供应品,以备下半年使用。
We have some significant supply chain flow to serve our significant customer demand that we see.
我们发现有大量的供应链流动来满足我们所见到的大量客户需求。
And this is demand that we see across a wide range of different customers. They are building platforms for some of the largest enterprises, but also setting things up at the CSPs and the large consumer internet companies.
这是我们在广泛的不同客户群中所看到的需求。他们正在为一些最大的企业构建平台,同时也在为云服务提供商和大型消费互联网公司搭建基础设施。
So we have visibility right now for our data center demand that has probably extended out a few quarters and this led us to working on quickly procuring that substantial supply for the second half.
目前我们已经能够预测数据中心需求的可见度,这个预测可能会持续数个季度,因此我们开始快速采购下半年需要的大量供应。这样做的原因是为了满足我们的需求并确保业务顺利运行。
I'm going to pause there and see if Jen's are going to add a little bit more.
我要暂停一下,看看Jen是否会增加一点内容。
I thought the little screen could live. Thank you.
我以为这个小屏幕可以复活。谢谢。
Next we'll go to CJ Muse with Evercore, ISI, your line's open.
接下来我们将与Evercore, ISI的CJ Muse联系,您可以发言了。
Yeah, good afternoon. Thank you for taking the question. I guess with data center, essentially double in quarter on quarter, two natural kind of questions that relate to one another come to mind.
嗨,下午好。感谢您回答我的问题。我想,随着数据中心在季度内基本上翻倍,会涉及到两个自然有关的问题,而这两个问题相互关联。
Number one, where are we in terms of driving acceleration into servers to support AI?
我们在推动加速发展服务器以支持人工智能方面的进展如何?
And as part of that, as you deal with longer cycle times with TSMC and your other partners, how are you thinking about managing the commitments there with where you want to manage your lead times in the coming years to best match that supply and demand?
作为此事的一部分,您在与台积电和其他合作伙伴处理漫长的周期时间时,如何考虑管理承诺以最好地匹配未来供求所需的提前期呢?
Thanks so much.
非常感谢你。
Yes, CJ. Thanks for the question. I'll still start backwards.
是的,CJ。谢谢你的问题。我会从后往前开始说。意思是要倒着说。
Remember, we were in full production of both Ampere and Hopper when the CHAT GPT moment came and it helped everybody crystallize how to transition from the technology of large language models to a product and service based on a chatbot.
请记得,当 CHAT GPT 时刻到来时,我们正全力以赴生产 Ampere 和 Hopper 两个产品,在这一刻,这个事件帮助大家明确了如何从大型语言模型的技术过渡到基于聊天机器人的产品和服务。
The integration of guard rails and alignment systems with reinforcement learning and human feedback, knowledge vector databases for proprietary knowledge, connection to search, all of that came together in a really wonderful way.
护栏和定位系统与强化学习和人类反馈的整合、专有知识向量数据库以及与搜索的连接,在一种非常出色的方式下共同实现了。
And the reason why I call it the iPhone moment, all the technology came together and helped everybody realize what an amazing product it can be and what capabilities it can have.
我称之为“iPhone时刻”的原因是所有技术都结合在一起,帮助人们意识到它有多么惊人的产品和潜力。
And so we were already in full production.
因此,我们已经处于全面生产状态。
Envious supply chain flow and our supply chain is very significant, as you know.
我们的供应链流和你们的供应链流一样都非常重要,这是毋庸置疑的。
And we built supercomputers in volume and these are giant systems and we built them in volume.
我们大量建造了超级计算机,这些是巨型系统,我们大量建造了这些系统。
It includes, it includes of course the GPUs but on our GPUs the system boards have 35,000 really components and the networking and the fiber optics and the incredible transceivers and the next, the smart nicks, the switches, all of that has to come together in order for us to stand up a data center.
这句话包括了许多内容,当然也包括GPU,但是我们的GPU系统板有35,000个组件,还有网络、光纤、令人惊叹的收发器、智能网卡、交换机等,所有这些都要协同运作,才能搭建一个数据中心。
And so we were already in full production and when the moment came, we had to really significantly increase our procurement substantial for the second half as Collette said.
因此,我们已经全面进入生产阶段,当时我们必须显著增加采购,就像Collette所说的那样,以应对下半年的需求。
Now let me talk about the bigger picture and why the entire world's data centers are moving towards exonerated computing.
现在让我谈一谈更大的背景以及为什么整个世界的数据中心都正在向免责计算靠拢。
It's been known for some time and you've heard me talk about it that exonerated computing is a full stack problem but it's a full stack challenge.
许多人已经知道,而且你也听我谈论过,免责计算是一个完整的技术堆栈问题,但它也是一个挑战性问题。
But if you could successfully do it in a large number of application domain has taken us 15 years.
但是,如果您能够在大量应用领域成功地做到这一点,这需要我们15年的时间。
意思是:如果能够在许多应用领域成功应用这个技术,那需要花费15年的时间。
And sufficiently that almost the entire data centers major applications could be exonerated, you could reduce the amount of energy consumed and the amount of cost for our data centers substantially by an order of magnitude.
通过充分的优化,几乎所有数据中心的主要应用都能够得到开解,这样就能够将数据中心的能耗和成本大幅降低一个数量级。
It takes the cost a lot of money to do it because you have to do all the software and everything and you have to build all the systems and so on and so forth.
做这件事情需要花费很多钱,因为你需要开发所有软件,构建所有系统等等。
But you know we've been at it for 15 years.
但是你知道我们已经坚持这个事情15年了。
意思是说,我们已经长时间地坚持做某事。
And what happened is when gender today I came along it triggered a killer app for this computing platform that's been in preparation for some time.
今天当性别意识到出现时,它激发了一款为该计算平台准备了一段时间的杀手应用程序。
And so now we see ourselves in two simultaneous transitions.
因此,我们现在正处于两个同时进行的转变中。
The world's $1 trillion data center is nearly populated entirely by CPUs today.
世界上总价值1万亿美元的数据中心目前几乎完全由中央处理器(CPU)组成。
这句话的意思是,当前数据中心中主要使用的设备是中央处理器,而这些中央处理器接近总价值1万亿美元。
And I, you know $1 trillion, $250 billion a year it's growing of course but over the last four years you know call it a trillion dollar so the infrastructure installed.
我说了,每年有1万2千5百亿美元,当然还在增长,但在过去四年里,我们安装的基础设施已经值得称作万亿美元的投资。
And it's all completely based on CPUs and dumb nicks.
这完全是基于CPU和愚蠢的功能的。
It's basically unaccelerated.
基本上是不加速的。
In the future it's fairly clear now with this with gender to the eye becoming the primary workload of most of the world's data centers generating information.
未来,很明显,性别分类将成为世界各大数据中心主要工作之一,不断生成信息。
It is very clear now that and the fact that accelerated computing is so energy efficient that the budget of a data center will shift very dramatically towards accelerated computing.
现在非常清楚的是,加速计算非常节能,数据中心的预算将出现非常明显的向加速计算的转变。
And you're seeing that now.
你现在正在看到这一点。这句话的含义可能是在某个过程或现象中,你现在已经开始观察到某些变化或趋势。
We're going through that moment right now as we speak.
我们正在说话的时候经历那个时刻。
Well, while the world's data center capex budget is limited at the same time we're seeing incredible orders to retool the world's data centers.
嗯,虽然世界数据中心的资本支出预算是有限的,但同时我们看到了令人难以置信的重新装备世界数据中心的订单。
意思是,尽管资金预算受到限制,但仍然有很多订单要求重新装备数据中心。
So I think you're starting you're seeing the beginning of you know call it a 10 year transition to basically recycle or reclaim the world's data centers and build it out as accelerated computing. You'll have you'll have this pretty dramatic shift in the spend of a data center from traditional computing and to accelerate computing with smart nicks smart switches. You know of course GPUs and and the workload is going to be predominantly gender to the eye.
所以,我认为你正在看到一个持续十年的转变的开始,可以称之为回收或恢复全球数据中心,并将其建立为加速计算。你会看到数据中心的花费从传统计算向智能网卡、智能交换机和GPU加速计算的转变。当然,大部分工作将会是面向AI的。
Okay, we'll move to our next question. Vivek, are you with B of A securities your lines open? Well, thanks for the question. So could I just wanted to clarify does visibility mean data center sales can continue to grow sequentially in Q3 and Q4 or do they sustain at Q2 levels? I just wanted to clarify that. And then, then my question is that you know given this very strong demand environment, what does it do to the competitive landscape? You know does it invite more competition in terms of custom asics? Or does it invite more competition in terms of other GPU solutions or other kinds of solutions? How do you see the competitive landscape change over the next two to three years?
好的,接下来我们进入下一个问题。Vivek,你是来自B of A证券的吗?你的线路已经开通了吗?谢谢你的问题。我想澄清一下,可见性是否意味着数据中心销售可以在Q3和Q4以连续增长的方式继续增长,还是会保持在Q2的水平?我只是想澄清一下。接着,我的问题是,考虑到这种非常强劲的需求环境,它对竞争格局会产生什么影响?它是否会在定制ASIC方面引入更多的竞争?还是它会在其他GPU解决方案或其他解决方案方面引入更多的竞争?在未来两到三年内,您如何看待竞争格局会发生什么变化?
Yeah, Vivek, thanks for the question. Let me see if I can add a little bit more color. We believe that the supply that we will have for the second half of the year is we should sustain a relatively larger than H1. So we are expecting not only the demand that we just saw in this last quarter, the demand that we have in Q2 for our forecast, but also planning on seeing something in the second half of the year. We just have to be careful here, but we're not here to guide the second half. But yes, we do plan essential increasingly in the second half compared to the first half.
嗯,Vivek,谢谢你的问题。让我看看能否再加一些细节。我们相信我们下半年的供应将比上半年要更大,我们预计不仅会有上个季度出现的需求,还会在Q2的预测中有所表现,并且在下半年也有一定的市场需求。当然,我们需要谨慎,因为我们不是在预测下半年的情况。但是,是的,我们计划在下半年的市场需求将较上半年有所上涨。
But regarding competition, we have competition from every direction. Startups really, really well funded and innovative startups, countless of them all over the world. We have competitions from existing, existing semiconductor companies. We have competition from CSPs with internal projects and many of you know about most of these. So we're mindful of competition all the time and we get competition all the time. And various value proposition at the core is we are the lowest cost solution.
关于竞争问题,我们从各个方向都面临着竞争压力。有那些资金充足且创新的初创公司,全世界都有无数这样的公司。我们还有已经存在的半导体企业和一些已经在推行的CSP项目带来的竞争。因此,我们一直都对竞争保持警觉,并且我们时刻都在面对竞争。而我们的核心竞争优势就是我们提供的是最低成本的解决方案。
Where the lowest TCO solution and the reason for that is because it's already computing is two things that I talk about often, which is it's a full stack problem. It's a full stack challenge. You have to engineer all of the software and all the libraries and all the algorithms integrate them into and optimize the frameworks and optimize it for the architecture of not just one ship at the architecture of an entire data center, all the way into the frameworks, all the way into the models. And the amount of engineering and distributed computing, fundamental computer science work is really quite extraordinary. It is the hardest computing as we know.
“最低TCO解决方案”的含义是,由于它已经是计算的两个重要元素,我经常谈论的是它是一个全栈的问题。这是一个全栈的挑战。您需要设计所有软件、库和算法,将它们整合并优化框架,并针对整个数据中心的架构进行优化,从框架一直到模型。所涉及的工程和分布式计算,基础计算机科学工作是非常不同寻常的。这是我们所知道的最难的计算。
And so number one, it's a full stack challenge and you have to optimize it across the whole thing and across just a mind-blowing number of stacks. We have 400 acceleration libraries. As you know, the amount of libraries and frameworks that we accelerate is pretty mind-blowing. And the second part is that generative AI is a large scale problem and it's a data center scale problem. It's another way of thinking that the computer is the data center or the data center is the computer. It's not the chip. It's the data center. And it's never happened like this before.
首先,它是一个全栈挑战,你必须在整个系统中进行优化,出现了惊人的大量技术栈。我们有400个加速库。正如你所知道的,我们加速的库和框架的数量相当惊人。第二部分是生成式人工智能是一个大规模问题,并且是一个数据中心规模的问题。这是另一种思考方式,计算机就是数据中心,或者说数据中心就是计算机。重要的不是芯片,而是数据中心。这之前从未发生过。
And in this particular environment, your networking operating system, your distributed computing engines, your understanding of the architecture of the networking gear, the switches, and the computing systems, the computing fabric, that entire system is your computer. And that's what you're trying to operate. And so in order to get the best performance, you have to understand full stack and understand data center scale. That's what accelerator computing is. The second thing is that utilization, which talks about the amount of the types of applications that you can accelerate and diversity of your architecture, keeps the utilization high.
在这种特殊的环境下,您的网络操作系统、分布式计算引擎、以及对网络设备、交换机和计算系统,计算布局等整体架构的理解,都是您的计算机。您需要了解完整的系统架构和数据中心规模,才能获得最佳性能。这就是加速计算的意义。其次,利用率越高,异构架构的多样性和加速的应用程序种类越广,就能最大限度地提高利用率。
If you can do one thing and do one thing only and incredibly fast, then your data center is largely underutilized and it's hard to scale that out. And if you do this universal GPU, in fact, that we accelerate some of these facts, makes our utilization incredibly high. And so number one is your pet and that software, that's software intensive problems, a data center architecture problem, the second is utilization, versatility problem. And the third is just data center expertise. We've built five data centers of our own and we've helped companies all up to world build data centers.
如果你只能做一件事情,并且能以非常快的速度做好,那么你的数据中心很可能被低效利用,难以扩展。而且,如果你使用通用GPU,事实上,我们加速了一些这些任务,使我们的利用率非常高。因此,第一个问题是你的宠物和那些软件密集型问题,一个数据中心架构问题,第二个问题是利用率,多功能性问题。第三个问题只是数据中心专业知识。我们已经建立了五个自己的数据中心,并帮助公司建立世界级的数据中心。
And we integrate our architecture into all the world's clouds. And the moment of delivery of the product to standing up and the deployment, the time to operations of a data center is measured. You know, if you're not good at it and you're not proficient at it, it could take months. Standing up a supercomputer, let's see, some of the largest supercomputers in the world were installed about a year and a half ago and now they're coming online. And so it's not, you know, it's unheard of to see a delivery to operations of about a year. Our delivery to operations measured in weeks. And that's, we've taken data centers and supercomputers and we've turned it into products and the expertise of the team in doing that is incredible.
我们的架构被整合到了全球所有的云端。我们测量交付产品到部署的时间和数据中心运营的时间。如果你不擅长它,那可能需要数月的时间。要组建一个超级计算机,最大的一些超级计算机大约一年半前安装,现在才开始启动。因此,交付到操作大约需要一年的情况是不可思议的。我们的交付到操作的时间为数周。我们已经将数据中心和超级计算机转化为产品,团队在这方面的专业知识是令人难以置信的。
And so our value proposition is in the final analysis, all of this technology translates in the infrastructure, the highest throughput and the lowest possible cost. And so I think our market is of course very, very competitive, very large, but the challenge is really, really great.
因此,我们的价值主张可以说是,在最终分析中,所有这些技术都转化为基础设施、最高吞吐量和尽可能低的成本。因此,我认为我们的市场当然非常竞争,非常庞大,但挑战确实非常大。
Next, we go to Aaron Eraker's with Wells Fargo. You're lying to open. Yeah, thank you for taking a question and congrats on the clutter.
接下来,我们要去见沃尔斯·法戈的亚伦·艾瑞克。你们准备好了。嗯,感谢您接受提问并祝贺您的成功。
As we kind of think about unpacking the various different growth drivers of the data center business going forward, I'm curious, Collette, just how we should think about the monetization effect of software, considering that the expansion of your cloud service agreements continues to grow. I'm curious of what, where do you think we're at in terms of that approach, in terms of the AI enterprise software suite and other, you know, drivers of software only revenue going forward?
当我们考虑分解不同数据中心业务增长的各种驱动因素时,我很好奇,考莱特,我们应该如何看待软件的货币化效应,特别是考虑到您的云服务协议持续增长。我很想知道,在AI企业软件套件和其他软件收入驱动因素方面,您认为我们目前处于什么位置?
Thanks for the question. Software is really important to our accelerated platforms. Not only do we have a substantial amount of software that we are including in our newest architecture and essentially all products that we have, we are now with many different models to help customers start their work in generative AI and accelerated computing. So anything that we have here from DGX Cloud on providing those services, helping them build models or as you've discussed the importance of Nvidia AI enterprise, essentially that operating system for AI. So all things should continue to grow as we go forward, both the architecture and the infrastructure as well as the both availability of the software and the digital monetization data as well, the turnover of the Genshin-Circumence.
谢谢您的提问。软件对我们的加速平台非常重要。我们不仅将大量软件包含在我们的最新架构和所有产品中,我们现在还有许多不同的模型来帮助客户开始进行生成式人工智能和加速计算的工作。所以我们在DGX Cloud上提供这些服务,帮助他们构建模型,或者正如您所讨论的,Nvidia AI企业的重要性,这实际上是AI操作系统。因此,所有这些事情都应该随着我们前进而不断增长,包括架构和基础设施、软件的可用性以及数字货币数据的周转。
Yeah, we can see in real time the growth of generative AI and CSPs. Both for training the models, refining the models as well as deploying the models. As Colette said earlier, inference is now a major driver of accelerated computing because generative AI is used so, so, so, capability in so many applications already.
是的,我们可以实时观察到生成式人工智能和CSP的增长。它们不仅用于训练模型、优化模型,还用于部署模型。正如科莱特之前所说,推理现在是加速计算的主要驱动力,因为生成式人工智能已经在很多应用程序中有如此强大的能力。
There are two segments that requires a new stack of software and the two segments are enterprise and industrial. Enterprise requires a new stack of software because many enterprises need to have all the capabilities that we've talked about, whether it's large language models, the ability to adapt them for your proprietary use case and your proprietary data in alignment to your own principles and your own operating domains. You want to have the ability to be able to do that in a high performance computing sandbox and we call that DJX Cloud and create that model. Then you want to deploy your chatbot or your AI in any cloud because you have services and you have agreements with multiple cloud vendors and depending on the applications, you might deploy it on various clouds.
有两个领域需要一个新的软件堆栈,这两个领域分别是企业和工业。企业需要一个新的软件堆栈是因为很多企业需要我们谈到的所有能力,无论是大语言模型,还是能够适应自己的专有用例和专有数据,以符合自己的原则和经营领域。您希望能够在高性能计算沙盒中实现这一点,我们将其称为DJX云,并创建该模型。然后,您希望在任何云中部署您的聊天机器人或人工智能,因为您与多个云供应商有服务和协议,并根据应用程序的情况部署到各种云中。
For the enterprise, we have Envidia AI Foundation for helping you create custom models and we have Envidia AI Enterprise. Envidia AI Enterprise is the only accelerated stack, GPO accelerated stack in the world that is enterprise safe and enterprise supported. You know, they're they're they're constant patching that you have to do. There are 4,000 different packages that build up Envidia AI Enterprise and represents the operating engine and and operating engine of the entire AI workflow. The only one of its kind from data ingestion, data processing, you know, obviously in words of training in an AI model, you have a lot of data, you have to process and package up and curate and in a line and there's just a whole bunch of stuff that you have to do to the data to prepare it for training.
对于企业而言,我们有Envidia AI Foundation来帮助您创建定制模型,同时我们还有Envidia AI Enterprise。Envidia AI Enterprise是全球唯一的、具备GPO加速的完整堆栈,同时也是企业可靠和有支持的。您知道,要不断进行补丁更新。Envidia AI Enterprise包含了4,000个不同的软件包,构建了整个AI工作流的操作引擎。它是从数据摄取、数据处理到训练AI模型的唯一一种。在训练AI模型时,您需要处理很多数据,将其打包、整理和排列,以进行训练前的准备。
That amount of data could consume some 40, 50, 60 percent of your computing time. So data processing, data processing is a very big deal. And the second aspect of it is training the model, refining the model and third is deploying model for inferencing. And then in the AI Enterprise supports and patches and security patches continuously all of those 4,000 packages of software and and for an enterprise that wants to deploy their engines, it's like they want to deploy red hat Linux.
这么多数据可能会耗去你大约40、50、60%的计算时间。因此,数据处理非常重要。其次,重要的是训练模型、改进模型以及为推理部署模型。此外,在AI企业中,支持、补丁和安全补丁一直都在不断更新这4,000个软件包。对于想部署引擎的企业来说,这就像部署红帽Linux一样。
This is, you know, incredibly complicated software in order to deploy that in every cloud and as well as on-prem it has to be secure, it hasn't be supported. And so in VDA AI Enterprise is the second part. The third is on-prem.
这是非常复杂的软件,为了在每个云和本地网络上部署,必须保证其安全性和可支持性。因此,在VDA AI Enterprise中有第二部分,第三部分是本地部署。
Just as people are starting to realize that you need to align an AI to ethics, the same for robotics, you need to align the AI for physics. And aligning an AI for ethics includes a technology called reinforcement learning human feedback. In the case of industrial applications and robotics, it's reinforcement learning omniverse feedback. And omniverse is a vital engine for software defined in robotic applications and industries.
正如人们开始意识到需要让人工智能符合道德规范一样,对于机器人学科也是同样如此,需要让人工智能符合物理原则。而让人工智能符合道德规范包括一项名为强化学习人类反馈的技术。在工业应用和机器人学科中,它被称为强化学习万物反馈。而万物反馈是机器人学科和工业应用中软件定义的一个重要引擎。
And so omniverse also needs to be a cloud service platform. And so our software stack, the three software stacks, AI foundation, AI Enterprise and omniverse runs in all of the worlds, all of the worlds clouds that we have partnerships, DJX, cloud partnerships with.
因此,全宇宙也需要一个云服务平台。因此,我们的软件堆栈,包括AI基础、AI企业和全宇宙,在我们与DJX云合作伙伴关系中覆盖的所有世界及其云中运行。
Azure, we have partnerships on both AI as well as omniverse with GCP and Oracle. We have great partnerships in DJX Cloud for AI and AI Enterprise is integrated into all three of them. So I think the in order for us to extend the reach of AI beyond the cloud and into the worlds enterprise and into the worlds industries, you need two new software stacks in order to make that happen.
在 Azure 中,我们与 GCP 和 Oracle 有关于 AI 和 omniverse 的合作伙伴关系。我们与 DJX Cloud 有着良好的 AI 合作伙伴关系,并且 AI Enterprise 已经集成到它们的所有三个平台中。因此,为了将 AI 的覆盖范围扩展到企业和行业外的世界,我们需要两个新的软件堆栈来实现。
And by putting it in the cloud, integrated into the worlds CSP clouds, it's a great way for us to partner with the sales and the marketing team and leadership team of all the cloud vendors.
将它放在云端,并将其与世界各大云服务商的云集成,这是我们与所有云供应商的销售、市场团队和领导团队进行合作的好方法。
Next we'll go to Timothy Arquiri with UBS, your line haven. Thanks a lot. I had a question and then I had a clarification as well.
接下来我们会和瑞银的Timothy Arquiri联系,您那边接通了吗?谢谢。我想问一个问题,还有一点需要澄清。
So the question first is Jensen on the Infiniband versus Ethernet argument. Can you sort of speak to that debate and maybe how you see it playing out? I know you need the low latency of Infiniband for AI, but can you sort of talk about the attach rate of your Infiniband solutions to what you're shipping on the core compute side? And maybe whether that's similarly crowding out Ethernet like you are on the compute side.
首先,问题是关于Infiniband与以太网论战的。您可以谈谈这场辩论,以及您如何看待它的发展趋势?我知道,人工智能需要Infiniband的低延迟,但能否谈谈您的Infiniband解决方案与您核心计算方面发货的附加率?还可能像在计算方面一样,是否也在压缩以太网的市场份额?
And then the clarification collette is that there wasn't a share buyback despite you still having about $7 billion on the share repo authorization. Was that just timing?
科莱特的澄清是,尽管您仍有约70亿美元的股票回购授权,但并没有进行股票回购。那只是时间问题吗?意思是,尽管还有股票回购的授权,但却没有进行股票回购,问是否只是因为时间上的问题。
Thanks. How about you go first? Let me take this question. That is correct. We have a seven billion available in recurrent authorization for repurchasing. We did not repurchase anything in this last quarter, but we do repurchase upper to stick with and we'll consider that as we go forward as well.
谢谢。你可以先回答吗?让我回答这个问题。那是正确的。我们有70亿的可用回购授权。上个季度我们没有回购任何股票,但我们会考虑回购一些股票来保持股价稳定。随着时间的推移,我们会再考虑。
Thank you. Infiniband and Ethernet are target different applications in a data center. They both have their place. Infiniband had a record quarter. We're going to have a giant record year. And Infiniband has a really amazing quantum Infiniband. It has an exceptional roadmap. It's going to be really incredible.
谢谢。Infiniband和Ethernet针对数据中心中不同的应用,它们各有其优势。Infiniband曾经创造了记录季度,并将创造一个巨大的记录年。此外,Infiniband还拥有真正惊人的量子Infiniband,具有优秀的路线图,相信未来将会非常令人难以置信。
The two networks are very different. Infiniband is designed for an AI factory, if you will. If that data center is running a few applications for a few people for a specific use case and it's doing it continuously, and that infrastructure costs you pick a number $500 million. The difference between Infiniband and Ethernet could be 15-20% in overall throughput.
这两种网络非常不同。Infiniband是为人工智能工厂设计的。如果数据中心不断地运行几个特定用例的应用程序,支撑几个人使用,并且基础设施成本为5亿美元,那么Infiniband和以太网在总体吞吐量上的差异可能为15-20%。
And if you spent $500 million dollars in an infrastructure and the difference is 10-20% and $100 million, Infiniband is basically free. That's the reason why people use it. Infiniband is effectively free. The difference in a data center throughput is too great to ignore. And you're using it for that one application.
如果你在基础设施上花费了5亿美元,差距只有10-20%和1亿美元,Infiniband基本上是免费的。这就是人们使用它的原因。Infiniband实际上是免费的。数据中心吞吐量的差异太大,不能忽视。而你正在用它来做一个应用。
And so, however, if your data center is a cloud data center and it's multi-tenant, it's a bunch of little jobs. A bunch of little jobs and it's shipped by millions of people. And Ethernet's really the right answer. There's a new segment in the middle where the cloud is becoming a generative AI cloud. It's not an AI factory, per se. But it's still a multi-tenant cloud.
因此,如果你的数据中心是一个云数据中心且具有多租户功能,那么它就是由许多小任务组成的。这些小任务由数百万人同时完成,并且以太网是最适合的解决方案。现在,在云技术中存在一个新的领域,即生成性人工智能云。尽管不是一个完整的人工智能工厂,但仍然是一个多租户云。
But it wants to run generative AI workloads. This new segment is a wonderful opportunity. And at Computex, I refer to it at the last GTC. At Computex, we're going to announce a major product line for this segment, which is for Ethernet, focused, generative AI application type of clouds.
它希望运行生成式人工智能工作负载。这个新领域是一个绝佳的机会。在台湾国际电脑展上,我提到过它。在这个展会上,我们将为这个领域宣布一个重大的产品线,该产品线专注于以太网、集中式、生成式人工智能应用类型的云计算。
But Infiniband is doing fantastically. And we're doing record numbers quarter on quarter a year and a year. Next, we'll go to Stacey Radgund with Bernstein Research Shareline-Dovon.
但因芬巴因很出色。我们连续几个季度创下了记录数字,今年和去年都是如此。接下来,我们将转向Bernstein Research Shareline-Dovon的Stacey Radgund。
Hi guys. Thanks for taking my question. I had a question on inference versus training for generative AI. So you're talking about inferences being a very large opportunity.
大家好,感谢回答我的问题。我关于生成式人工智能中的推理和训练有一个问题。您说推理是一个非常大的机会。
I guess two sub parts of that. Is that because inference basically scales with the usage versus training is more of a one in done? And can you give us some sort of, even if it's just qualitatively, if you think inference is bigger than training or vice versa, if it's bigger, how much bigger is it? Because the opportunity is at 5x, as it 10x. Is anything going to give us on those two workloads within generative AI, the helpful?
我想这个问题有两个子问题。是因为推理基本上与使用规模成比例,而训练更多是一次性任务吗?你能否就一个定性的回答告诉我们,你认为推理比训练更重要,或者反过来,如果推理更重要,它比训练更重要多少?机会是5倍,还是10倍?在生成型人工智能的这两个工作负载中,有什么有用的信息可以提供给我们吗?
Yeah. I'll work backwards. You're never done with training. You're always, every time you deploy, you're collecting new data, when you collect new data, you train with the new data. And so you're never done training. You're never done producing and processing a vector database that augments the large language model. You're never done with vectorizing all of the collected, unstructured data that you have.
是的。我会往回看。培训从未结束。每当你部署时,你都会收集新数据,当你收集到新数据后,你就会用新数据进行培训。所以你永远不会停止培训。你永远不会停止生成和处理增强大型语言模型的向量数据库。你永远不会停止将收集到的非结构化数据向量化。
And so whether you're building a recommender system, a large language model of vector database, these are probably the three major applications of the three core engines of you well. Of the future of computing. It's all a bunch of other stuff, but obviously these are very three very important ones. They're always, always running. You're going to see that more and more companies realize they have a factory for intelligence and intelligence factory.
因此,无论你是在建造一个推荐系统,一个大型语言模型还是一个向量数据库,这些都很可能是你的核心引擎的三个主要应用。这是未来计算的全部内容。虽然还有其他许多东西,但显然这三个非常重要。它们总是在运行。你会看到越来越多的公司意识到他们拥有一个智能工厂和智能工厂的概念。
And in that particular case, it's largely dedicated to training and processing data and vectorizing data and learning representation of the data so on and so forth. The inference part of it are APIs that are either open APIs that can be connected to all kinds of applications. APIs are integrated into workflows, but APIs of all kinds are behind us of APIs in a company.
在这种情况下,主要是用于训练、处理、向量化数据和学习数据的表示等。推断部分是API,可以连接到各种应用程序的开放API。这些API被集成到工作流中,但所有种类的API都在公司的API背后。
Some of them they built themselves, some of them part that many of them could come from companies like ServiceNow and Adobe that we partner with in AI foundations. And they'll create a whole bunch of generative APIs that companies can then connect into their workflows or use as an application. And of course there'll be a whole bunch of internet service companies.
其中一些是我们自己建造的,还有一些是和我们在人工智能领域合作的公司,比如ServiceNow和Adobe提供的。它们将创建许多生成API,公司可以将其连接到他们的工作流程中或用作应用程序。当然,也会有许多互联网服务公司。
So I think you're seeing for the very first time simultaneously a very significant growth in the segment of AI factories as well as a market that a segment that really didn't exist before, but now it's growing exponentially practically by the week for AI inference with APIs.
因此,我认为您现在首次同时见证了人工智能工厂领域的显著增长以及一个之前几乎不存在、但现在每周以指数级增长的API人工智能推理市场的增长。
The simple way to think about it in the end is that the world has a trillion dollars of data center installed and it used to be 100% CPUs in the future. We know we've heard it in enough places and I think this year's ISC keynote was actually about the end of Moore's Law. We've seen it in a lot of places now that you can't reasonably scale out data centers with general purpose computing and that accelerated computing is the path forward.
最简单的思考方式是,现在全球拥有一万亿美元的数据中心,过去百分之百依靠 CPU 进行运行,但未来将不再如此。我们已经在多个场合听到过它,我认为今年的 ISC 主题演讲实际上就是关于摩尔定律的终结。现在我们已经看到,在大规模数据中心扩容上,通用计算已经无法满足需求,加速计算是未来的方向。
And now it's got a killer out. It's gone generative AI. So the easiest way to think about that is your trillion dollar infrastructure. Every quarter's capital catbex budget would lean very heavily into generative AI into accelerated computing infrastructure everywhere from the number of GPUs that would be used in the catbex budget to accelerate switches and accelerated networking chips that connect them all.
现在,这个系统已经拥有了致命的杀手。它实现了生成式人工智能。最简单的理解方法是,你的兆亿级基础设施。每个季度的资本支出预算都将大量倾斜于生成式人工智能,包括加速计算基础设施,从用于计算预算的大量GPU到连接它们的加速交换机和加速网络芯片。
The easiest way to think about that is over the next four or five or ten years. Most of that trillion dollars and then compensating just for all the growth in data center still will be largely generative AI. The easiest way to think about that is training as well as inference.
最简单的方法是考虑未来四五年或十年。这万亿美元大部分是为了弥补数据中心的增长而产生的人工智能。最简单的方法是考虑训练和推断。
Next we'll go to Joseph Moore with Morgan Stanley. Your line's open.
接下来我们将与摩根士丹利的约瑟夫·摩尔联系。你可以开口了。简单明了地表示我们要联系到某个人,并让对方开口发言。
Great thank you. I wanted to follow up on that. In terms of the focus on inference it's pretty clear that this is a really big opportunity around large language models. But the cloud customers are also talking about trying to reduce cost per query by very significant amounts. You can talk about the ramifications for you guys.
非常感谢。我想跟进一下这件事。就推理方面的重点而言,很明显,这对于大型语言模型来说是一个非常重要的机遇。但云客户也在谈论如何通过大大降低每个查询的成本。您可以谈一谈这对你们的影响。
Where are some of the specialty inference products that you launched at GTC? How are you going to help your customers get the cost per query down?
在 GTC 上,您推出了一些特别推理产品在哪里?您将如何帮助客户降低每次查询的成本?
意思是问在GTC上您推出了哪些特别的推理产品,您将如何帮助客户降低他们查询的成本。
That's a great question. Whether you start by building a large language model and you use that large language model very large version and you could distill them into medium, small and tiny size. The tiny size ones you could put in your phone and your PC and so on and so forth. They all have good, you know, they all have, it seems surprising but they all can do the same thing.
这是个很棒的问题。你是否可以从构建一个大型语言模型开始,并使用该大型语言模型的版本,将它们缩小到中等、小型和微型大小。其中的微型尺寸可以放置在你的手机、电脑等设备上。它们都有很好的表现,似乎令人惊讶的是它们都可以做同样的事情。
But obviously the zero shot or the generalizability of the large language model, the biggest one is much more versatile and it can do a lot more amazing things. And the large one would teach the smaller ones how to be good AI.
显然,零样本或大型语言模型的通用性更强,可以做更多令人惊叹的事情。而大型模型会教小型模型如何成为优秀的AI。
And so you use the large one to generate prompts to align the smaller ones and so on and so forth. And so you start by building very large ones and then you also have to train a whole bunch of smaller ones. Now that's exactly the reason why we have so many different sizes of our inference.
所以你使用大型的来生成提示,以便对齐较小的,依此类推。因此,您开始构建非常大型的,然后还必须训练一堆较小的。这正是我们推理中有这么多不同尺寸的原因。
You saw that I announced L4, L40, H100 NBL, which also has H100 and then we have H100 multi-node with NV link and so there's, there's a, you could, you could have model sizes of any kind that you like.
你看到了我宣布了L4、L40、H100 NBL,其中还有H100,然后我们有带有NV链接的H100多节点。因此,你可以选择任何你喜欢的模型大小。
The other thing that's important is these are models but they're connected ultimately to applications and the applications could have image in, video out, video in, text out, you know, image in, proteins out, text in, 3D out, video in in the future, 3D graphics out. You know, so the input in the output requires a lot of pre-imposed processing.
另一个重要的事情是,这些是模型,但它们最终与应用程序连接在一起。这些应用程序可以具有图像输入,视频输出,视频输入,文本输出,图像输入,蛋白质输出,文本输入,3D输出,未来可能还有视频输入和3D图形输出。因此,输入和输出需要大量的预处理和后处理。
The pre-imposed processing can be ignored and this is one of the things that most of, most of the specialized chip arguments fall apart and it's because the link, the model itself is only, you know, call it 25% of the data of the overall processing of inference. The rest of it is, is about pre-processing, post-processing, security, you know, decoding, all kinds of things like that. And so, so I think the multi-modality aspect of inference, the multi-diversity of inference that it's going to be done in the cloud on prem. It's going to be done in multi-cloud, that's the reason why we have an AI enterprise and all the clouds. It's going to be done on prem, that's the reason why we, you know, we have great partnership with Dell, which is the NALST the other day called Project Helix. That's going to be integrated into third party services, that's the reason why we have a great partnership with ServiceNow and Adobe because they're going to be creating a whole bunch of generative AI capabilities.
预设处理可以被忽略,这也是大多数专业芯片争论中的一个矛盾点之一,因为链接和模型本身只构成了整个推理处理的25%左右。其余的则与预处理、后处理、安全、解码等相关。因此,我认为推理的多模式方面,多样性将在云端、本地和多云上完成,这就是我们拥有一个AI企业和所有云的原因。它将在本地完成,这就是我们与戴尔合作的重要性,他们最近提出的“Helix项目”将被整合到第三方服务中,这也是我们与ServiceNow和Adobe建立伟大合作的原因,因为他们将创造一系列生成AI能力。
And so there's all the diversity and the reach of generative AI is so, so broad. You need to have some very fundamental capabilities like what I just described in order to really address the whole space of it.
因此,生成式人工智能的多样性和覆盖范围非常广泛。你需要拥有一些非常基本的能力,就像我描述的那样,才能真正涵盖整个空间。
Next we'll go to Harlan, sir, with JP Morgan, your lines open. Hi, good afternoon and congratulations on the strong result of the execution. I really appreciate more of the focus or some of the focus today on your networking products. I mean, it's really an integral part, it took sort of maximized the full performance of your compute platforms. I think that data center networking business is driving about a billion dollars of revenues per quarter plus or minus. You know, that's two and a half X growth from three years ago, right, when you guys acquired Mel and also very strong growth.
接下来我们将与摩根大通一起去哈兰,您可以开始发言了。下午好,恭喜您获得强劲的业绩。今天更加关注您的网络产品,我非常感激。网络产品是整个计算平台发挥最大性能的重要组成部分。我认为,数据中心网络业务每个季度提供大约10亿美元的收入,这比三年前收购Mel时增长了2.5倍,增长非常强劲。
But given the very high attach of your Infinity Band, you can add solutions to your accelerated compute platforms is the networking run rate stepping up in line with your compute shipments. And then what is the team doing to further unlock more networking bandwidth going forward? Just to keep pace with the significant increase in compute complexity, data sets, requirements for lower latency, better traffic predictability and so on.
但是考虑到您的无限带宽的非常高的连接性,您可以将解决方案添加到加速计算平台中,以便使网络运行速率与计算产品的交付保持一致。那么团队正在做什么来进一步释放更多的网络带宽?只是为了跟上计算复杂性、数据集、更低延迟要求、更好的流量可预测性等方面的显著增加的步伐。
Yeah, Harlan, I really appreciate that. Nearly everybody who thinks about AI, they think about that chip, that accelerator chip. And in fact, misses the whole point nearly completely. And I've mentioned before that that accelerator computing is about the stack, about the software. And networking, remember, we announced a very early on this networking stack called DOKA. And we have the acceleration library called MagnumIO. These two pieces of software are some of the crown jewels of our company. Nobody ever talks about it because it's hard to understand. But it makes it possible for us to connect tens of thousands of GPUs. How do you connect tens of thousands of GPUs if the operating system of the data center, which is the infrastructure, it is not insanely great.
哈兰,我非常感谢你。几乎所有思考AI的人都会想到那个芯片、加速器芯片。事实上,几乎完全忽视了整个关键点。我之前提到过,加速计算是关于堆栈、关于软件的。记得我们早期公布了一个叫DOKA的网络堆栈。还有一个叫MagnumIO的加速库。这两个软件是我们公司最重要的部分之一。很少有人谈论它,因为它难以理解。但正是它使我们能够连接数万个GPU。如果数据中心的操作系统基础设施不是极好的,那么怎么连接数万个GPU呢?
And so that's the reason why we're so obsessed about networking in the company. And one of the great things that we have, we have, you know, Melanox, as you know, quite well, was the world's highest performance and the unambiguous leader in high performance networking. That's the reason why our two companies are together. You also see that our network expands starting from NVLink, which is a computing fabric with really super low latency. And it communicates using memory references, not network packets. And then we take NVLink. We connect it inside multiple GPUs and I've described going beyond the GPU and I'll talk a lot more about that at Computex in a few days. And then that gets connected to Infiniband, which includes the NIC, the SmartNIC, Bluefield 3 that we're in full production with and the switches, all of the fiber optics that are optimized end to end, these things are running at incredible line rates.
这就是为什么我们公司如此痴迷于网络的原因。我们公司有一个很棒的东西,你也非常熟悉,那就是Melanox,它是世界上性能最高的高性能网络的无可争议的领导者。这也是我们两家公司联合的原因。你会发现我们的网络从NVLink开始扩展,它是一个计算网络,具有超低的延迟,并使用存储器引用而不是网络数据包进行通信。然后我们将NVLink连接到多个GPU内部,我也解释了超越GPU,并且在几天后的Computex上我会更详细地讲解。然后这个GPU连接到Infiniband,包括NIC、SmartNIC、Bluefield 3开关和优化端到端的所有光纤,这些东西以令人难以置信的线速运行着。
And then beyond that, if you want to connect the Smart AI factory, the SmartFact, this AI factory into your computing fabric, we have a brand new type of ethernet that we'll be announcing at Computex. So this whole area of the computing fabric, extending, connecting all of these GPUs and computing units together all the way through the networking, through the switches, the software stack is insanely complicated. And so we're delighted you understand it. But we don't break it out particularly because we think of the whole thing as a computing platform as it should be. We sell it to all of the world's data centers as components so that they can integrate it into whatever style or architecture that they would like and we can still run our software stack.
接着,如果你想将智能 AI 工厂 SmartFact 连接到你的计算网络中,我们将在 Computex 上推出一种全新类型的以太网。计算网络这一整个领域,通过扩展、连接所有的 GPU 和计算单元,一直延伸到网络、交换机以及软件堆栈,过于复杂。我们很高兴你能够理解这一点。但是我们没有特别地把它分离出来,因为我们认为整个计算平台应该被视为一个整体。作为组件,我们将它销售给全球数据中心,这样他们就可以将它集成到任何风格或架构中,而我们仍然可以运行我们的软件堆栈。
That's the reason why we break it up. It's way more complicated the way that we do it. But it makes it possible for Nvidia's computing architecture to be integrated into anybody's data center in the world from cloud of all different kinds to on-prem of all different kinds all the way out to the edge to 5G. And so this way of doing it is really complicated but it gives us incredible reach.
这就是我们分化的原因,虽然我们这样做变得更加复杂,但是这使得Nvidia的计算架构可以集成到世界上任何数据中心中,从各种各样的云到各种各样的本地机器,甚至延伸到边缘至5G。因此,这种做法确实很复杂,但是却使我们拥有极强的覆盖范围。
And our last question will come from Matt Ramsey with TD Cowan. Your line's over. Thank you very much. Congratulations, Jensen, and to the whole team. One of the things I wanted to dig into a little bit is the DGX cloud offering. You guys have been working on this for some time behind the scenes where you sell in the hardware to your hyperscale partners and then lease it back for your own business. And the rest of us kind of found out about it publicly a few months ago. And as we look forward over the next number of quarters as Collette discussed to high visibility and the data center of business, if you could talk a little bit about the mix you're seeing of hyperscale customers buying for their own first party internal workloads versus their own sort of third party their own customers versus what of that big upside in data center going forward is systems that you're selling in with potential to support your DGX cloud offerings and what you've learned since you've launched it about the potential of that business.
我们的最后一个问题来自TD Cowan的Matt Ramsey。你的话筒已经开了。非常感谢。祝贺Jensen和整个团队。我想深入探讨一下DGX云服务。你们在幕后已经有一段时间在销售硬件给超大规模合作伙伴,然后再通过租赁回租方式为自己的业务提供服务。而我们其他人几个月前才公开了解到。随着Collette所讲的向数个季度的高能见度和数据中心业务发展,在向前看的时候,你能否谈一下超大规模客户购买第一方内部工作负载与他们自己的第三方客户的混合比重,以及在数据中心去年商业的巨大增长中,你们销售的系统是否有潜力支持DGX云服务,以及自开展以来你们对这项业务的潜力学习?
Thanks. Yeah, thanks, Matt. It's without being too specific about numbers but the ideal scenario, the ideal makes is something like 10% Nvidia DGX cloud and 90% the CSP's clouds. And the reason and our DGX cloud is the Nvidia stack, it's the pure Nvidia stack. It is architected the way we like and it achieves the best possible performance. It gives us the ability to partner very deeply with the CSPs to create the highest performing infrastructure.
谢谢。是的,谢谢,Matt。不具体说明数字,理想情况下,理想的情况是约10%是Nvidia DGX云,90%是CSP的云。而我们DGX云的原因是它是纯Nvidia堆栈。它被构建的方式是我们所喜欢的,并达到了最佳性能。这使我们能够深度合作与CSPs一起创建最高性能的基础设施。
Number one, number two, it allows us to partner with the CSPs to create markets. Like for example, we're partnering with Azure to bring omniverse cloud to the world's industries. And the world's never had a system like that. The computing stack with all the generate today, I stopped and all the 3D stuff and the physics stuff, incredibly large database and really high speed networks and low latency networks. That kind of a virtual industrial virtual world has never existed before. And so we partnered with Microsoft to create omniverse cloud inside Azure cloud. And so it allows us number two to create new applications together and develop new markets together. And we go to market as one team and we benefit by getting customers on our computing platform and they benefit by having us in their cloud number one, but number two, the amount of data and services, security services and all of the amazing things that Azure and GCP and OCI have, they can instantly have access to that through omniverse cloud. And so it's a huge win win and for the customers, the way that Nvidia's cloud works for these early applications, they could do it anywhere.
第一、第二,它允许我们与CSP合作创建市场。例如,我们正在与Azure合作,将全能云带给世界的工业。世界上从未有过这样的系统。计算堆栈带有所有今天生成的内容,我停下来和所有的3D内容和物理内容,非常大的数据库和高速网络和低延迟网络。这样一种虚拟的工业虚拟世界以前从未存在过。因此,我们与微软合作,在Azure云内创建全能云。因此,它使我们能够共同创建新的应用程序和开发新的市场。我们作为一个团队走向市场,我们通过在我们的计算平台上获得客户而受益,并且他们通过我们在他们的云中的数量,数据和服务、安全服务以及Azure、GCP和OCI拥有的所有惊人的东西,他们可以通过全能云立即获得。因此,这是一个巨大的双赢,对于客户而言,Nvidia的云计算如何针对这些早期应用程序工作,他们可以在任何地方完成。
So one one standard stack runs in all the clouds. And if they would like to take their software and run it on the on the CSPs cloud themselves and manage it themselves, we're delighted by that because Nvidia enterprise and Nvidia AI foundations and long term, this is going to take a little longer, but Nvidia omniverse will run in the CSPs clouds. Okay, so our goal really is to drive architecture to partner deeply in creating new markets and the new applications that we're doing and provide our customers with the flexibilities to run Nvidia everywhere, including on-prem. And so those were the primary reasons for it and it's worked out incredibly. Our partnership with the three CSPs and we currently have DJX cloud in. And there are Salesforce and marketing teams, there are leadership teams, it's really quite spectacular, it works great.
一个标准的堆栈可以在所有云平台上运行。如果他们想要将他们的软件运行在CSP云端并自己管理,我们很高兴,因为Nvidia企业、Nvidia AI基础设施和长期的Nvidia Omniverse都将在CSP云端运行。我们的目标是深入合作,推动架构,创建新市场和新应用程序,并为客户提供在任何地方运行Nvidia的灵活性,包括在企业。这就是我们的主要原因,这个合作非常成功。目前我们与三个CSP的合作伙伴关系已经达到了极佳的表现,其中包括了DJX云构建,还有Salesforce和营销团队,领导团队,这真的非常壮观,运作得非常好。
Thank you, I'll now turn it back over to Jensen Wong for closing remarks. The computer industry is going through two simultaneous transitions, accelerated computing and generative AI. CPU scaling has slowed, yet computing demand is strong. And now with generative AI, supercharged. Data computing, a full stack and data center scale approach that Nvidia pioneered is the best path forward.
谢谢,现在我将把话题交还给 Jensen Wong 进行结束语。计算机行业正在经历两个同时进行的转变,即加速计算和生成式人工智能。CPU 的缩放速度减慢了,但计算需求强烈。现在,随着生成式人工智能的出现,这种需求更强劲了。据中的数据计算,一种全栈和数据中心规模的方法,是 Nvidia 开创的最好的前进道路。
There's a trillion dollars installed in the global data center infrastructure based on the general purpose computing method of the last era. Companies are now racing to deploy accelerated computing for the generative AI era. Over the next decade, most of the world's data centers will be accelerated. We are significantly increasing our supply to meet their surging demand.
全球数据中心基于上一时代的通用计算方法已安装了数万亿美元,而现在企业正在竞相推出用于生成人工智能时代的加速计算。在未来十年内,大部分世界的数据中心都将进行加速。我们正在显著增加我们的供应,以满足它们激增的需求。
Large language models can learn information encoded in many forms. Guided by large language models, generative AI models can generate amazing content. And with models to fine tune, guard rail, align to guiding principles and ground to ground the facts, generative AI is emerging from labs and is on its way to industrial applications.
大规模语言模型可以学习多种形式编码的信息。在大规模语言模型的指导下,生成型人工智能模型可以生成惊人的内容。而且通过针对模型进行微调、监管、对准指导原则和基于事实的根据,生成型人工智能正在从实验室中崛起,并朝着工业应用的方向发展。
As we scale with cloud and internet service providers, we are also building platforms for the world's largest enterprises. Whether within one of our CSP partners or on-prem with Dell Helix, whether on a leading enterprise platform like ServiceNow and Adobe or Bespoke with Nvidia AI foundations, we can help enterprises leverage their domain expertise and data to harness generative AI securely and safely.
随着云和互联网服务提供商的扩展,我们同时也在为世界最大型企业构建平台。无论是我们的CSP合作伙伴之一,还是在Dell Helix等地方,无论是在像ServiceNow和Adobe这样的领先企业平台上,还是在基于Nvidia AI的定制板上,我们都能帮助企业安全、安全地利用他们的领域专业知识和数据来利用生成AI。
We are ramping a wave of products in the coming quarters, including H100, our grace and grace hopper superchips and our Bluefield 3 and Spectrum 4 networking platform. They are all in production. They will help deliver data center scale computing that is also energy efficient and sustainable computing.
我们即将在未来几个季度推出一系列产品,包括我们的 H100、Grace 和 Grace Hopper 超级芯片和 Bluefield 3 和 Spectrum 4 网络平台。它们都正在生产中。它们将帮助提供数据中心规模的计算,同时也是能源高效和可持续的计算。
Join us next week at Computex and we'll show you once next.
下周加入我们参加Computex展会,我们会向你展示最新的技术。
Thank you. This concludes today's conference call. You may now. Thank you.
谢谢大家参加今天的电话会议。现在你们可以离开了。谢谢。