How Generative AI Changes Strategy
发布时间 2023-05-25 17:07:10 来源
摘要
Strategy is about making the future happen, not just reacting to it, according to author Gary Hamel. And with generative artificial intelligence, senior leaders suddenly wield an awesome new tool to change the fortunes of their organizations. The promise of generative AI is more than just a sweet hack to boost productivity and streamline operations. Its deeper potential lies in companies that rethink what they do and conjure brand-new, AI-first products and services. Simply put, generative AI is blasting open new strategic paths to create novel business opportunities, even as it brings serious risks and heightened competition.
In this episode, How Generative AI Changes Strategy, HBR editor in chief Adi Ignatius speaks to Microsoft’s head of strategy Chris Young and Harvard Business School professor Andy Wu. They lay out the technology, its emerging value chains, and its main providers. They also break down the key choices and tradeoffs that large and small companies alike will be making in this fast-changing market.
This is the fourth and final episode in the special series How Generative AI Changes Everything. Each week, HBR editor in chief Adi Ignatius and HBR editor Amy Bernstein have been hosting conversations with experts and business leaders about the impact of generative AI. Find those episodes on the impact on productivity, creativity, and organizational culture in the HBR IdeaCast feed.
And for more on ethics in the age of AI, check out HBR’s Big Idea on implementing the new technology responsibly.
GPT-4正在为你翻译摘要中......
中英文字稿 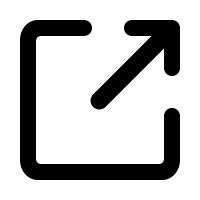
Do you want to go deeper on business strategy? I want to suggest HBR's new podcast feed, HBR on Strategy. HBR editors like me hand select the best strategy case studies and conversations from across HBR's podcasts, videos and beyond. Listen for free to HBR on Strategy wherever you get your podcasts. New episodes every Wednesday.
你想进一步了解商业策略吗?我想推荐《哈佛商业评论》的新播客节目HBR on Strategy。像我这样的HBR编辑会精选最佳的策略案例研究和讨论,来自HBR的播客、视频等多个方面。您可以在任何您获取播客的地方免费收听HBR on Strategy。每周三都会有新的节目发布。
Chris, you've been a tech company CEO, your strategy headed Microsoft. You've seen a lot of technologies over the years. There's some that land and we think, okay, this is truly transformative, disruptive. This is an inflection point. Your mind is that where we are now or do we need to wait and see what the impact of generative AI will be on business and on everything? We're at a place where we see this as truly transformative.
克里斯,作为一名科技公司CEO,你领导了微软的战略,这些年来经历了很多技术的变革。有些技术会落地实现,我们就会认为它是真正具有颠覆性的,这是一个拐点。你认为现在我们已经到达这个点了,还是需要等待并观察生成式人工智能对于商业和一切事物的影响?我们目前处于一个真正具有革命性变革的时刻。
I might actually draw a contrast to one of the trends that many people were very excited about in the tech landscape just a little bit over a year ago, which was Web 3 in the promise of blockchains and everything that would happen. There was definitely a segment of the world that was saying, well, interesting, but not sure what the practical applications are here. Not sure it's going to change everything. No one's saying that about AI. I think immediately many of us recognize that this is going to be completely game changing, not only for the tech landscape, but for humanity.
我想和大约一年前许多人非常兴奋的一种科技趋势进行对比,那就是Web3的承诺和区块链的一切。当时肯定有人说,这很有趣,但不确定它在实践中的应用是什么。不确定它是否会改变一切。但没有人会这样评价AI。我认为我们中的许多人都会立即认识到,这将完全改变游戏,不仅是科技格局,而且还会改变人类。
Welcome to how generative AI changes everything, a special series of the HPR IDA cast. There many shorthand definitions of strategy. One that I like is from the author Gary Hamill, who says that strategy is about making the future happen, not just reacting to it. Well, this sure feels like a time when it's possible to make the future happen. Generative AI has stormed into the consciousness of practically every business leader, and it's opening up new strategic paths and new ways to create new businesses. This week, how generative AI changes strategy.
欢迎来到HPR IDA Cast 的特别系列“How generative AI changes everything”。关于战略有许多简短的定义,我喜欢的一个来自作家Gary Hamill,他说战略是关于让未来发生,而不仅仅是应对它。嗯,现在似乎正是有可能让未来发生的时候。生成式AI已经风靡了几乎所有的商业领袖,它正在开辟全新的战略路径和创造新业务的新方法。本周,我们来探讨生成式AI如何改变战略。
I'm Adi Ignatius, Editor-in-Chief of Harvard Business Review and your host for this episode. In this special series, we're talking experts to find out how this new technology changes workforce productivity and innovation, and we're asking how leaders should adopt generative AI in their organizations and what it means for their strategy. Later on in this episode, you're going to hear from Andy Wu, a professor at Harvard Business School. I ask him to talk through the trade-offs that large and small companies alike will be making in this fast-changing market. But right now, I'm talking to Chris Young. He's the head of strategy at Microsoft, which is partnered with OpenAI and is rolling out generative AI in its search product, office productivity suite, and cloud computing services.
我是Adi Ignatius,哈佛商业评论杂志的主编,也是本期节目的主持人。在这个特别系列节目中,我们邀请了专家来谈论新技术如何改变生产率和创新力,并询问领导者应该如何在企业中采用生成式AI以及它对他们的战略意义。在本期节目的后半段,你将会听到哈佛商学院的教授Andy Wu的讲解。我请他分析大型和小型公司在这个快速变化的市场中所做出的权衡。但现在,我正在与Microsoft公司战略负责人Chris Young交谈。Microsoft与OpenAI合作,在搜索产品、办公生产力套件和云计算服务中推广生成式AI。
Chris, thanks for coming on the show. Great to be here, Adi. So, I want to jump right in. Do you feel that there's an urgency here as a provider that you need to be first mover to win in this market? You don't necessarily have to be the first mover, but there are significant advantages in being first mover. I do think that's one of the reasons why there's so much velocity in the startup community. Certainly, that's one of the things that we're sensitive to in our own business is making sure that we move quickly because the market is moving quickly.
克里斯,感谢你来参加节目。很高兴在这里见到你,阿迪。那么,我想直接开始谈。作为服务提供商,你觉得有紧迫性需要成为先行者来赢得这个市场吗?你不一定非要成为第一人,但是成为先行者具有显著优势。我认为这正是创业社区中有如此高速发展的原因之一。当然,这也是我们自己业务的敏感问题之一,我们要确保快速行动,因为市场正在快速变化。
This is, I will tell you, the cycles in and around AI are faster than we've ever seen in the tech landscape. Companies being built, changes every week. Much companies are moving faster more than we've ever seen. I think it's because of everybody sees the opportunity here and the promise for change.
我告诉你,人工智能领域和周边领域的发展循环速度比科技领域中我们曾经见过的都快。每周都有新的公司被创建,变化不断。许多公司都在发展得比以往更快。我想这是因为每个人都看到了这里的机会和改变的承诺。
That makes sense, but then, of course, you get the backlash, which is, let's slow down. Let's make sure that this technology, what it creates is safe, is not biased. You hear all the complaints. How do you balance the sense of wanting to innovate, but also not wanting to be reckless with a technology that's powerful and we're not even sure exactly everything can do?
这个观点很有道理,但随之而来的,当然会受到回击,也就是我们要减缓步伐。我们要确保这项技术创造的东西是安全和不带偏见的。你会听到许多抱怨。我们该如何平衡想要创新的愿望,但又不想对一项强大的技术不负责任,而且我们甚至不确定它能做到什么程度?
We're very committed to safe and responsible AI. That's a core pillar of everything we're doing. Certainly at Microsoft and that's something I'm personal believer in. I will tell you that in some ways, while the cycles are moving quickly today, they've been many years in the making. There's a lot of work that's gone in behind the scenes to build the right foundational principles around AI, how we deliver it, the systems around which we bring AI to the market. Microsoft, our partner open AI and others have been very grounded in those sets of principles and practices for a while now. That's allowed us to move quickly as the market really shifted after chat. TPPT came out in the fall.
我们非常致力于安全和负责任的人工智能,这是我们正在做的一切的核心支柱。在微软公司,这是一项非常重要的工作,而我个人也深信不疑。我可以告诉你,在某些方面,尽管现在的技术变得非常快速,但这些技术已经经历了多年的发展。在人工智能的基本原则、如何交付以及我们将人工智能引入市场的系统等方面,有很多工作在幕后完成。微软公司、我们的合作伙伴开放AI和其他公司一直致力于这些原则和实践。这使我们能够在市场在聊天TPPT出现后迅速转移方向。
One of the words in the air now is regulation. Is this a moment where regulation would be welcome in the industry or is that something you rather you brace yourself for? It's inevitable. We welcome it. It's going to be an important part of this. AI is such a powerful and these foundational models are really, really quite powerful. We at Microsoft are very, very committed to participating with government and shaping the future around this.
目前社会上流传着“监管”的一个词。您认为这个行业是否需要监管,或者您是否更愿意自我保护呢?监管是不可避免的,我们欢迎监管,因为它将成为这方面重要的一部分。人工智能是如此强大,并且这些基础模型非常强大。微软非常致力于与政府合作,共同塑造未来。
We've heard a lot of that in the media very recently. We think it's an important part of the evolution of AI in our society. How quickly is this market moving more generally in terms of just people out there developing products and services and doing incredible things? Well, I sit in San Francisco and in the startup world, I can tell you, in the fall, we were tracking about 80 companies that were working on AI. Today, we're tracking 8,000. It's literally a Cambrian explosion of organizations that are working on this.
我们最近在媒体上听到了很多这方面的消息。我们认为这是人工智能在我们社会中演化的重要部分。就开发产品和服务以及做一些令人惊叹的事情而言,在这个领域整体市场的发展速度是多快?嗯,我在旧金山的创业圈里,我可以告诉你,在秋季,我们跟踪了大约80家正在从事人工智能工作的公司。今天,我们正在跟踪8,000家公司。这简直就是寒武纪大爆发,大量机构都在这个领域进行研究。
Now, some of those 8,000, many of them were probably doing something else as a startup and now they're pivoting to making AI a bigger part of their offer. The way I think about it is any company that was a SaaS company before has now got to find a way to make AI a part of what they're doing because in many ways, in the fullness of time, AI is going to change the nature of how software and humans interact with one another. I think that'll be a very different way in which we expect services to happen.
现在,那些8000家企业中的一些可能最初从事其他创业项目,现在他们正在将人工智能作为他们服务的重要组成部分。我的理解是,任何一家以SaaS为主业的公司现在都必须找到一种方法来将人工智能融入到他们正在做的业务中,因为从长远来看,人工智能将改变软件和人类互动的方式,这将带来非常不同的服务方式。
Today, we use software to do things. We use software to bring experiences to us, to augment our capability. With AI, it's going to abstract a lot of that interface with the software and it's going to force us to think differently around what is in a software experience or a SaaS experience to look like for a customer. I think that's going to change a lot of businesses that exist today. It's also going to bring new businesses that we haven't yet thought about.
今天,我们使用软件来完成事情。我们使用软件为我们提供体验,增强我们的能力。随着人工智能的发展,它将抽象化很多软件界面,强迫我们以不同的方式考虑对客户而言软件体验或SaaS体验应该是什么样的。我认为这将改变许多现有的商业模式,并带来我们尚未想到的新的商业机会。
With any technology, we talk about how's it have nots and the gap between the two. With this technology, do you imagine this will widen the gap or narrow it again between the technological haves and have nots? One of the big productivity opportunities with AI is writing code. It's technology development. Traditionally, small companies or small organizations, they don't generally have the same access to great developers or lots of developers and big companies have been able to make those investments but with the capabilities that AI can bring to develop code, the promise of that is it can be really profound.
在任何技术方面,我们都会谈论它的优势和差距。对于这项技术,您是否想象它将扩大还是缩小科技领域中的差距?AI技术的一个重要生产力机会是编写代码。这是技术发展。传统上,小公司或小型组织通常没有同样伟大的开发人员,也没有像大公司那样投入大量的开发人员和资金,但是利用AI的能力来开发代码,它的潜在价值是非常深远的。
Think about bringing this capability to small community-based organizations where someone who's not technical now has the ability to create software, to create workflow, to build tooling that allows that organization to achieve its mission more efficiently and more effectively whereas before you would have had to hire consultants and spend money that you didn't have in order to technology enable that organization. If done well, this allows so many more organizations and people to be able to get the promise and the benefit of what technology can do for all of us. That argument is that it's really democratizing access to the highest level of technology. That's how I feel about it.
考虑将这种能力带给小型社区组织,让那些非技术人员也能够创建软件、创建工作流程、构建工具,使该组织能够更高效、更有效地实现其使命,而以前你不得不雇佣顾问、花费你没有的钱来为该组织技术赋能。如果做得好,这将使更多的组织和人能够获得技术为我们所有人所带来的承诺和好处。我的观点是,这真正实现了对最高水平技术的民主化获取。
Imagine for a second, somebody who's heading a relatively small firm is aware of all this chatter and early developments with this technology, not quite sure how to get in. What was the head of strategy for Microsoft tell the head of strategy for a smaller firm how to get into this realm? The first thing I would do is start using it and get familiar with it. Then think about what can I do to improve productivity across different functions in my organization? What can I do then to apply it to workflows in my organization because there will be value there and then what new experiences can I create?
试想一下,一个经营相对较小公司的人意识到这个技术的早期发展和所有的讨论,但不确定应该如何介入。如果微软的战略主管告诉一个较小公司的战略主管如何进入这个领域,他会说什么呢?首先,我会开始使用它并熟悉它。然后思考如何提高我的组织在不同功能上的生产力?然后我可以将其应用于我的组织工作流程,因为这里有价值,最后我可以创造出什么新的体验?
What new business models might be available to me? Again, I think that's a process that anyone can go through in a big company as well as a small company. To what extent does generative AI change the nature of strategy? How we approach it? The first thing I think it does to strategy is it speeds up the cycles. Anyone who's thinking, hey, I'm going to do an annual strategy process and that's going to be sufficient, it's no longer sufficient.
有哪些新的商业模式可以为我所用?我认为这是一个大公司和小公司都可以进行的过程。生成式人工智能在多大程度上改变了战略的性质?我们如何去应对?我认为它首先加速了战略的周期。任何人都不应该认为,我只需要进行年度战略流程就足够了,现在这已经不够了。
Certainly not in the tech landscape. You just got to be faster than that. You got to be more iterative than that. I also think that it's going to help us frame trade-offs and decisions in a more efficient way.
在科技领域绝对不能这样慢悠悠。你必须更快速、更具迭代性。我还觉得这会帮助我们更有效地权衡取舍和做出决策。
For example, one of the things that AI does for us is it brings access to information that you would normally have to send humans out to go gather a lot of information, maybe do a lot of interviewing, and finally you synthesize it down into, hey, how do I do that? How do we think about these trade-offs or these decisions that we want to make? This is just going to speed up all those cycles. You're going to be able to get at those conversations a lot more quickly than we've been able to as we've gone through these normal strategy processes.
例如,人工智能为我们做的一件事情就是让我们能够获得人类需要去收集大量信息,进行许多采访,并最终将其综合成“如何去做”“我们如何考虑这些取舍或决策”的信息。这将加速所有这些过程的速度。我们将能够更快地获得这些交流,比我们在经历这些常规战略过程时所能获得的要快得多。
We'll be able to ask ourselves some different questions because of what's possible. Then there's the whole, what are the new things we can go do? We can go create because AI is now available to us. That's a big strategy question for literally everyone. Whether you're a business, a nonprofit, or even a government agency.
由于技术的进步,我们将能够提出不同的问题。而且我们现在可以开展一些新事物,因为人工智能已经向我们开放。这对每个人都是一个重大的战略性问题,不管你是一个企业、非营利组织或政府机构。
So you've been talking today mostly about applications that are available right now. Throw this forward. What does the workplace look like? The AI-powered workplace look like in, say, five or ten years? I think the AI-powered workplace is going to change the nature of work for pretty much everyone because you're going to now have, at minimum, you're going to have a set of co-pilots that allow people who have certain job functions today to do their jobs more efficiently, more effectively, to produce better output.
今天你们谈论的主要是当前可以使用的应用程序。那么往前看,未来五到十年,AI驱动的工作场所会是什么样子?我认为AI驱动的工作场所将会改变几乎每个人的工作性质,因为你将会有至少一组副驾驶员,让今天某些工作职能的人更高效、更有效地完成工作,产出更好的成果。
I think the customer experience for everyone is going to be completely transformed. That's one of the things I'm honestly super excited about from a business perspective is the transformation of the customer experience. You call a company, you've got a problem, and it takes a lot of energy and effort to get the, particularly if it's a thorny problem, they get it fixed because people are searching through different systems. They might hand you off to three or four different reps, and then you've got to re-explain your issue. In a world where you have co-pilots that are AI enabled, I think you can make it far more efficient and pleasant for the user who's calling in, the customer who's calling in, who just wants to get whatever experience it is or product it is or issue it is handled, quickly, painlessly, so they can move on with their lives.
我认为每个人的客户体验将会完全变革。从商业角度来看,我最期待的事情之一就是客户体验的转型。当你打电话给一家公司并且遇到问题时,需要很多精力和努力来确保它被解决,尤其是对于复杂的问题,因为人们需要在不同的系统中进行搜索。他们可能会将你引荐给三四个不同的代表,然后你必须重新解释你的问题。在一个使用AI技术的共同领航世界里,我认为你可以使打电话的用户(也就是顾客)能够更有效、更愉悦地处理他们所遇到的任何经历、产品或问题,让他们可以快速、无痛苦地继续他们的生活。
I think that's going to be completely transformed. For the user, it's going to allow you to get on with your life, and that's going to reshape some things inside of organizations, but it's going to, I think also open up a lot of different opportunities. There's so much that we still have to go do in our society, for example, we've got to electrify vehicles, we've got to make supply chains more efficient. We need to educate our students in ways where we've got shortages of teachers. We need to provide healthcare in a much more broad-based fashion at a cheaper cost basis.
我认为这将会发生彻底的变革。对于用户来说,它将使你的生活更轻松,这将会重塑组织内部的某些事情,同时我认为它也会带来许多不同的机会。我们社会还有很多事情要做,例如,我们必须电气化车辆,使供应链更加高效。我们需要以更便宜的成本基础为广大人民普遍提供医疗保健服务。我们需要以更广泛的方式教育学生,我们缺乏教师。
I think AI's potential to change things in a way that's good for humanity is something I get very excited about when I think about the future. Chris Young, I really want to thank you. You're on the front lines and developing these technologies. I want to thank you for joining us today. Thanks for having me.
我认为人工智能在改变对人类有利的方面的潜力是让我对未来感到非常兴奋的事情。克里斯·扬,我非常感谢你。你在一线开发这些技术。感谢你今天加入我们。谢谢你接待我。
Coming up after the break, I ask HBS Professor Andy Wu about navigating this emerging landscape. Should you build your own generative AI? Buy it? Something in between? It depends. He breaks down these strategic choices. Stay with us.
广告过后,我将采访哈佛商学院教授Andy Wu,探讨在这个新兴的领域中如何导航。你应该自己建立生成性人工智能还是购买?或者在这两者之间做出选择?答案是取决于具体情况。他将解析这些战略选择。请继续关注。
Welcome back to how generative AI changes strategy. I'm Adi Igdacias. Joining me now to discuss how to navigate this new strategic landscape is Andy Wu, a professor at Harvard Business School who specializes in technology in business. Andy, thank you for joining me. Adi, thank you for having me.
欢迎回来,我们今天要谈论生成AI是如何改变商业策略的。我是Adi Igdacias。现在加入我们的讨论是哈佛商学院的技术专家Andy Wu。今天我们将探讨如何在这个新的战略环境中导航。Andy,感谢您的加入。Adi,谢谢你邀请我参加讨论。
So let's start by talking money. I think a lot of us have been enjoying playing with some of these generative AI tools for free or at a nominal price tag. I get the sense that the generative AI requests costs a lot more, maybe 10 times more, as a commercial web search. I guess my question for you is, is that true? And what is the basic cost structure here?
让我们先来谈谈钱。我觉得我们很多人都在免费或者极少花费的价格下享受使用一些生成AI工具。但是我感觉,生成AI请求的费用要比商业网络搜索贵很多,也许高出十倍。我想问你的问题是,这是真的吗?这里的基本成本结构是什么?
Right. I think thinking about the economics is really important now. And Adi, you're absolutely right about the high cost of inference in terms of how we input and output information from these large language models or any other generative AI model. It is more expensive on a variable cost basis than anything we've ever done in computing. A relative to a simple Google search, doing a generative AI type output like Chatchy PT could be anywhere from 10 to 100 times or more expensive than just a basic Google search. And that's something that companies are going to have to reckon with. And shall we assume that those costs will come down? The way costs sometimes come down?
没错,我认为现在思考经济是非常重要的。Adi,你关于在输入和输出信息方面使用这些大型语言模型或任何其他生成型AI模型时推断成本很高是完全正确的。相对于简单的Google搜索,做一个生成型AI输出像Chatty PT这样的成本可能是基础Google搜索的10到100倍甚至更贵。这是公司必须面对的问题。我们可以假设这些成本会降低吗?就像成本有时会下降一样?
Cost will almost certainly come down. But at the same time, we have to remember that we are in an arms race right now of increasingly complicated and large models. So even as costs come down, the models are going to get more complicated that will raise costs again. So this is always going to be more expensive on a variable cost basis to how we've done computing in the past.
成本几乎肯定会降低。但与此同时,我们必须记住,我们现在处于越来越复杂和大型模型的军备竞赛中。因此,即使成本下降,这些模型也将变得更加复杂,这将再次提高成本。因此,与过去的计算方式相比,这将始终具有更高的可变成本。
All right. So let's say you're a large corporation or a midsize company even. You see the potential for Gen AI in your company. You want to get in the game. But what do you need to know about what it is that you bring to the table to start thinking through these decisions and whether this technology would have value for you?
好的,假设你是一家大型公司或中型公司,你看到了Gen AI在你的公司中的潜力,你想加入这场游戏。但是你需要知道什么是你的优势,以开始思考这些决策,以及这项技术是否对你有价值?
So there's three levels of increasing sophistication of how a company can get involved and really bring AI into their organization or their product. The most simple way of doing it is basically looking for the right applications that you can use in the market. Of course, Chat GPT has made its way into a lot of companies already. And that's not going to require a lot of deep thinking.
因此,公司可以通过三个不同层次逐渐深入地使用人工智能来参与他们的组织或产品。最简单的方法就是寻找市场上可用的适当应用程序。 当然,聊天GPT已经进入了许多公司。 这并不需要过多深思熟虑。
But when we get to the next level of perhaps integrating a third party application programming interface into your workflow, into your product, that's where we really got to think more about what are the ways that a custom integrated AI tool for your organization might be valuable. And so for instance, consider the example of two retailers. So think about the department store, Macy's, or the supermarket croakers. And so they might consider the possibility of using an API and application programming interface from open AI or elsewhere.
但是,当我们达到了将第三方应用程序编程接口(API)融合到您的工作流程或产品中的下一个级别时,我们就需要更加思考一下,定制集成 AI 工具对您的组织可能有哪些有价值的方式。例如,考虑两个零售商的示例。想象一下百货商店 Macy's 或超市 Kroger,它们可能考虑使用来自 Open AI 或其他地方的 API 和应用程序编程接口的可能性。
And then integrating that into their applications so that their customers can have like a Chat interface to learn about new products. Now there's going to be a lot of potential for custom modifications at that level to tailor that AI for your particular customer-based organization. And so in the example I just gave, imagine if a customer typed in the word dressing into the Chatbot. For Macy's and for croakers, they want the word dressing to deliver completely different responses.
然后将这个引入到他们的应用程序中,使得他们的客户可以像聊天界面一样了解新产品。在这个层次上,会有很多潜在的自定义修改机会,以适应你特定的客户组织的AI。所以在我刚才举的例子中,想象一下如果一个客户在聊天机器人中输入了“调料”,对于梅西百货和克鲁克斯而言,他们想要的“调料”会提供完全不同的响应。
And so you'd need to modify the input, say, prompt engineering so that Macy's would want to append the word clothing in front of the word dressing. And croakers might want to append the word salad in front of the word dressing to make sure the Chat is giving the right kind of response.
因此,您需要修改输入,例如,提示Macy's希望在“dressing”一词前附加“clothing”一词。而Croakers可能希望在“dressing”一词前附加“salad”一词,以确保Chat提供正确的响应。
One level even deeper than the API is many companies I think are going to need to look at the serious possibility of putting together their own model. And that doesn't mean starting from scratch. It means taking an open source model that's out there that Facebook or others have already committed hundreds of millions of dollars to developing. And then using your own proprietary data to then fine tune that model for your use case.
比 API 更深层次的是,我认为许多公司需要认真考虑建立自己的模型。这并不意味着要从头开始。它意味着利用已经投入了数亿美元开发的 Facebook 或其他公司的开源模型,然后使用自己专有的数据来对这个模型进行调整以适应自己的使用情况。
So Bloomberg has trained a model already of their own. And what I want managers to know is that the buried entry to doing this is actually pretty attainable. The numbers I'm hearing from the marketplace right now, it could cost you a million dollars or less. I've seen some people fine to models for under a thousand dollars. And you can have a custom model that is based on your data for your organization. And that's going to depend on whether or not you have the proprietary data and be whether or not a custom model for your specific vertical is going to dominate a more general model.
彭博社已经训练了一个自己的模型。我想要让经理们知道的是,实际上,这个埋藏的入口是相当容易实现的。听到的市场数字是,它可能会花费你一百万美元或更少。我见过一些人为模型花费不到一千美元。你可以拥有一个基于你的数据定制的、适用于你的组织的模型。这取决于你是否有专有数据,以及你的特定垂直领域的定制模型是否会比更通用的模型更为主导。
And then are you suggesting that companies could build these themselves or how do you think through the Bivers' build decision here, whether you develop in house or partner with somebody else that has this know how?
你是在建议公司可以自己建造吗?你如何考虑Bivers在这里的建造决策,无论是在公司内部开发还是与拥有这种专业知识的其他合作伙伴合作?
Yeah. Well, fortunately, there's a lot of resources now that you can have your own model but have other people help you put together that model. So for example, Amazon has something called SageMaker where let's say you have your own data available. You can just give it to Amazon and they will fine tune the model for you. And then boom, you've got your nice little custom model already.
好的。现在有很多资源可以让你拥有自己的模型,但同时也能得到其他人的帮助来建立它。比如,亚马逊有一个叫做SageMaker的东西,如果你有可用数据,你可以直接把它交给亚马逊,他们将会为你微调这个模型。然后巴拉,你就已经拥有了自己的小定制化模型了。
At the same time, of course, the larger your organization and the more specific tweaks that you're going to need to the model, then you're going to want to consider the possibility bringing a lot more of this in house. Of course, right now the talent in AI is very expensive. And so that's something we'll have to run into.
同时,当然,如果你的组织规模越大,模型需要的特定优化越多,那么你可能要考虑将更多的工作内部处理。当然,现在AI领域的人才非常昂贵。因此,这是我们将不得不面对的问题。
How do you even know who to partner with? I mean, suddenly there is a whole generative AI industry that's springing up. So if you want to, if you want to partner, who do you partner with? How do you trust the expertise? Figure out who's who and who's a valuable partner.
你如何知道该与谁合作?我的意思是,突然间出现了整个生命创造人工智能产业。如果你想合作,你要与谁合作呢?你如何信任其专业知识?找出谁是谁,谁是有价值的合作伙伴。
So there's three factors I would consider here.
这里有三个因素需要考虑。
The first is your trust of that partner in terms of their ability to potentially handle your secure data. Whether or not you're going to give them all your data at once to build a model or you're going to have your employees type in potentially confidential stuff into an application. And I think the process for evaluating that trust and safety and security is the same process our enterprise CTO has already been doing for two decades.
首先,是你对合作伙伴的信任问题,包括他们有无能力处理你的安全数据。无论你是一次性交给他们所有的数据来建立模型,还是让你的员工输入可能涉及机密的内容到应用程序里面。我认为,评估信任、安全和安全性的过程与我们企业的首席技术官已经进行了20年的过程。
Second part of this is simply just price. In that, in the longer term, I think a lot of the more general technologies we're going to be using are going to be fairly competitive in the market. And so in the end, a lot of this is going to get driven down to just the variable cost of the cloud computing or computing technology. And so I think price is actually surprisingly important here.
第二部分就是价格。从长远来看,我认为我们将使用的许多更为通用的技术将在市场上竞争激烈。因此,最终,很多东西都将转化为云计算或计算技术的变量成本。所以我认为价格在这里实际上非常重要。
Even across the big models that we've seen like from Meadow or from OpenAI, actually for most purposes, the difference in quality isn't that significant. So in most cases, I would pick the one that was cheaper on a variable cost basis especially.
即使在我们所见过的大型模型中,例如Meadow或OpenAI,实际上对于大多数目的而言,质量的差异并不那么显著。因此,在大多数情况下,我会选择基于变量成本更便宜的一个。
And then the third thing, and this is particularly important in today's environment, is that you really got to think about the stability of your partner and their financial viability. And so a decent amount of the AI companies we're talking about, the most well-funded ones, they raised a lot of their money before the current downturn in the market. And many of them are going to be struggling with their cash situation this year. Certain number of them will not make it into next year. And so I would want to have a partner, a provider, who you think will make it into the coming years.
接着第三个问题,在当今环境下尤其重要,那就是你必须考虑你的伙伴的稳定性和财务实力。我们正在谈论的一些人工智能公司,大部分募集资金是在当前市场下滑之前。他们中的许多人将会在今年面临资金问题。其中一些甚至无法进入明年。因此,我希望我的合作伙伴或服务提供商是能够在未来几年中持续存在的。
When you talk about this, there could be a lot of, I guess, new job categories, new specializations. I mean, even when you say, train the AI on the content. What does that mean in sort of layman's terms? What is the job of training an AI bought on a company's exclusive content or data?
当你谈论这个话题时,可能会有很多新的工作类别和专业化,我猜测。我的意思是即使你要“训练AI学习内容”,这句话也需要用通俗易懂的语言来阐述。那么,训练一款AI来学习公司专有的内容或数据,这个工作具体是什么意思呢?
Well, at least in today's world, and especially in the next couple of years, there is an increasing amount of tools that assist developers in doing the fine-tuning process. So I think in general, like any sort of backend data engineer, software engineer would increasingly be comfortable doing this kind of thing, particularly as we are updating undergraduate and graduate education around this kind of technology.
好吧,至少在今天的世界中,尤其是未来的几年,越来越多的工具可协助开发者进行微调过程。因此,我认为一般来说,与任何后端数据工程师、软件工程师一样,这种工作将越来越容易,尤其是我们在本科和研究生教育中更新了这种技术。
But what it does in tail is, I would say, some hard factors and some soft factors. So in terms of hard factors, there are technical skills about how to run the model and to a particular run the model efficiently when you're training it. I've heard stories of companies in Silicon Valley where some engineer made a very human mistake of typing a bracket instead of a coltlin or something. And then in the process of running the training, they literally just lit $500,000 of electricity and hardware on fire. Like, because they just started the process with the typo and then now we've got a completely wasted training cycle.
但就其尾部的功能而言,我认为它包括一些硬性因素和一些软性因素。在硬性方面,涉及的是关于如何运行模型和如何在训练模型时有效地运行模型的技术技能。我听说过硅谷的一些公司发生过这样的事情:一些工程师由于输入了一个括号而不是一个冒号之类的符号,就在训练模型的过程中引起了500,000美元的电力和硬件浪费。因为他们只是因为一个小错误,就启动了整个训练周期,而这完全是无用的。
Now I think the soft factors here are also important when you're fine-tuning. And this is something that I was really surprised about to see engineers doing this. But a lot of this training process is based on fuel and intuition. And so what happens is that they will take data and then introduce it to the model to help re-weight the parameters of an existing, say, open source model. And there isn't an obvious point where you want to stop the apox or iteration process. And so what they're doing is they're feeling it out and eyeballing it and running some, there's some mathematical tests, but they're also just eyeballing the results that are coming out and then making a judgment call, okay, have we trained this model enough? Do we have enough data to do what we want?
现在我认为在微调时,软因素也很重要。这是我真的很惊讶地看到工程师们在做这个的时候会用到。很多训练过程都是基于直觉和经验的。他们会拿数据来给一个现有的开源模型加重参数,没有明显的点来结束近似或者迭代过程。所以他们在感觉和观察中进行,同时也会运用一些数学测试。他们会从结果中筛选出来进行判断,看看这个模型是否已经训练足够了,是否有足够的数据去完成我们想要的任务。
Interesting. So when you think about the org chart of the future, what does AI, what does generative AI do to middle managers? I think middle managers are going to be here to stay and let me tell you why. If we think about this new job category of prompt engineers or people who specialize in interacting with the AI, what are they doing? They're asking good questions, they're giving detailed instructions. What does a middle manager do? They're a prompter of humans. And so in that sense, like the skill set of a middle manager, I think is actually more and more important. And actually what I think is different here is as we contrast managers with, say, individual or independent contributors, I think it's actually the middle managers that are coming out ahead in that there are the ones that actually have the skill set that are to actually work with the AI as opposed to someone who is, say, writing a contract or writing code as an independent contributor.
很有趣。那么,当您考虑未来的组织结构图时,人工智能、生成式人工智能会对中层管理人员产生什么影响呢?我认为中层管理人员将继续存在,让我告诉您为什么。如果我们考虑到这个新的职业类别——提示工程师或是专门与人工智能互动的人,他们在做什么呢?他们在提出好问题,给予详细指示。而中层管理人员是人类的提示者。因此,在这个意义上,我认为中层管理人员的技能组合实际上越来越重要。实际上,我认为这里的不同之处在于,与独立贡献者相比,管理人员实际上处于领先地位,因为他们具有与人工智能一起工作的技能组合,而不是像独立贡献者那样撰写合同或编写代码。
Yeah, so it's sort of all about the prompt. I mean, is that a burgeoning industry now, chief prompt officer or something like that? I think in the short term, it's going to be quite important. And we're still early on how that's exactly going to be organized. But I do imagine that a significant amount of managers and companies are themselves in addition to managing humans going to have to be managing an AI. And in that case, those people, I think, will also have to develop the skill set of working with the AI and building that intuition over time. And I imagine the training for that would be a combination, like kind of how we teach business and management of humans today. It's a mix of a good HBR article plus like years of experience on the job, right?
是的,这个问题主要就是关于提示的。我的意思是,是不是现在出现了一种新兴的行业,像是最主要提示官之类的?在短期内,我认为这非常重要。至于如何组织这一切,我们仍处于早期阶段。但是我认为,很多经理和公司除了管理人类外,还要管理人工智能。在这种情况下,这些人也需要培养与人工智能合作并逐渐建立直觉的技能。我想这种培训会是一种组合,就像今天我们教授商业和人类管理的方式一样。这是一篇好的《哈佛商业评论》文章加上多年的工作经验的混合。
All right. So let's say you're a technology provider. How would you be thinking about these markets right now? This is a very tough one to look at right now as far as being a technology provider. But I think the upside is that there's a lot of places in the value chain here that you can compete in as a technology provider, you can compete in supplying data or you can compete in helping other people build models. There's also a burgeoning industry of going back to private data centers that I think will need more of. And of course, at the application layer, I think we will see a whole different category of applications that are going to be AI first. And then that's the area where I think we're going to have a whole new generation of entrepreneurs that are going to be quite disruptive to the older generation.
好的,假设您是一家科技提供商,您现在会如何考虑这些市场呢?作为一家科技提供商,现在看这些市场确实是非常困难的。但我认为好处在于,在这个价值链中有很多地方可以竞争。作为科技提供商,您可以在提供数据方面竞争,也可以在帮助其他人构建模型方面竞争。此外,现在有越来越多的企业开始重新关注私有数据中心,我认为这个市场还需要更多的供应商。当然,在应用层面上,我认为我们将会看到一整个全新的应用类别,逐渐成为AI的主要应用领域。这就是我认为将会涌现出一批全新的企业家,他们将会对老一代产生非常大的冲击的领域。
But what I do want to caution people here about is the open questions about the fundamental profitability of being just a model company. Isn't it interesting that Microsoft has essentially outsourced the model and that Google and Facebook has historically actually open sourced the model and Facebook today is very aggressive about open sourcing that model. And so what we think of is actually the core AI technology, these large companies are actually, it's not internal or they're handing it out for free. And what does that tell us about where the profitability is and may not actually be in that part of the technology? If we go back to the internet and look at what are the most important technologies for the rise of the internet, I would put the three technologies as telecommunications, like H&T Verizon, second would be TCPIP, third would be HTML. How much money did those people make on the internet? Approximately nothing.
但我要提醒大家的是,要注意对于仅仅成为一个模型公司的基本盈利能力存在的疑问。值得注意的是,微软基本上已经将模型外包了,而Google和Facebook历史上实际上已经将模型开源,而Facebook现在也非常积极地推动开源这个模型。因此,我们考虑的是核心的AI技术,这些大公司实际上并不是内部开发,或者是免费提供的。这对于盈利所在的地方告诉我们什么呢?也许真正的盈利点并不在这个技术的这个部分。如果我们回到互联网,看看互联网崛起的最重要的技术是什么,我会把三个技术归为一类:电信技术,比如H&T Verizon;第二是TCP / IP,第三是HTML。那些人在互联网上赚了多少钱?近乎无。
And that's what we have to be careful about here is that I think there are going to be portions of the value chain for AI that will not capture a lot of the profitability. So if you had to guess right now, who's going to make the big money in this? Who stands to be the big winner? So I think that the providers of the hardware technology used for this kind of technology are going to come out very strong.
在这里我们需要小心的是,我认为人工智能的价值链中可能会有一些部分无法捕获大量的利润。因此,如果你现在不得不猜测谁将在其中赚大钱,谁有可能成为大赢家呢?我认为为这种技术提供硬件技术的供应商将会走得非常强势。
There's nobody in the world that prefer to be right now than Jensen Huang and Nvidia. And on the other end of the stack, I do think that entrepreneurs building AI first applications will be in a great position, particularly as they're using and thinking about AI and reimagining how we traditionally think about applications. In terms of industry verticals where I think there is the biggest growth opportunity, the two areas I'm most excited about right now are video gaming and media or social media.
现在世界上没有人比Jensen Huang和Nvidia更想要现在这个时刻了。另一方面,我认为构建AI优先应用程序的企业家将处于一个很好的位置,特别是他们正在使用和思考AI以及重新想象我们传统地思考应用程序的方式。就我认为增长机会最大的行业领域而言,我现在最感兴趣的两个领域是视频游戏和媒体或社交媒体。
And I think a lot of the conversation we're having today is focused on AI as a productivity tool. I think the better way to look at it is actually AI as enhancing consumption. And so what we are talking about here with generative AI is we now have the ability to provide infinite personalized content to any person to entertain them until the end of time. And so I think that's an area that is going to be very, very exciting.
我认为今天我们所谈论的大部分是关于把人工智能看作一种提高生产力的工具,而更好的方式应该是把人工智能看作消费增强器。我们所称的生成式人工智能,意味着我们现在有能力为每个人提供无限的个性化内容,以供他们在未来的日子里进行娱乐消费。因此,我认为这是一个非常令人兴奋的领域。
So this is a super fast moving market, presumably the rules of classic strategy still apply. But I'd love to know, does this feel different from new technologies that you've studied before? Basically I like to think of this as a new type of computing platform. And so when I teach about technology, we teach students about the past in terms of the rise of operating systems and web browsers.
这是一个超级快速发展的市场,经典策略的规则可能仍然适用。但我很想知道,这与你之前研究过的新技术是否有所不同?基本上,我认为这是一种新型的计算平台。因此,当我教授关于技术的知识时,我们通常会通过操作系统和Web浏览器的兴起来讲述过去的故事。
And in terms of the current age, platform technologies like metaverse virtual reality, telecommunications enhancement and through 5G, as well as a variety of other computing interfaces like voice. These are all platforms. This is a new kind of platform that allows us to do a much more human-like form of computing. And I would say that many observers in Silicon Valley, I think, were surprised by this type of chat GPT model being the thing that really resonated with people. And that part, you know, I'm still surprised by, but I think it speaks to the broader mission of artificial generative intelligence in terms of reaching sort of the singularity and sort of replicating a human that really does speak to people quite literally.
在当前时代中,像元宇宙虚拟现实、电信增强以及通过5G等平台技术,以及其他各种计算接口(如语音)都属于平台。这种新型平台使我们能够进行更加人类化的计算。我认为,在硅谷的许多观察者中,他们对这种聊天GPT模型成为真正引起人们共鸣的事情感到惊讶。我仍然很惊讶,但我认为这表明了人工生成智能的更广泛的使命,即达到奇点并复制一个真正与人交流的人类。
All right, so what's your basic message to senior leaders who, you know, want to lead their organizations into the future with a generative AI solution? So for leaders that are trying to lead their organizations into the future, my core message would be you need to chill out and panic at the same time. And so when you're thinking about making investments in AI today, I want you to do it with a sense of urgency, but I don't want you to play for the next two years. You're playing for the next 10 years.
好的,那么对于那些想要用产生性人工智能解决方案引领他们的组织进入未来的高级领导者,你的基本信息是什么?所以,对于试图引领他们的组织进入未来的领导者而言,我的核心信息将是你需要保持冷静并同时感到紧张。当你考虑今天在人工智能方面进行投资时,我希望你抱有紧迫感,但我不希望你只考虑未来两年。你应该考虑未来十年。
Right now, it's a difficult time to make decisions around AI because at least my bed is we're almost certainly in the middle of a hype cycle around the technology. And so in that sense, it's not really about the current phase of the technology, but without a doubt in the next 10 years, advancements in computing and AI will continue to be transformative and they will have transformative effects around the same logic that you and I have talked about today. And that the same assumptions about the importance of data, the same assumptions about fixed costs and variable costs, all that will be the same. And the more you can start getting your organizations ready now, then you'll be ready for the next transformation.
现在,决定与人工智能有关的事情很难,因为我们几乎可以肯定,目前我们正处于对该技术的炒作周期中。因此,在这个意义上,它不是关于技术的当前阶段,但毫无疑问,在未来的10年中,计算和人工智能的进步将继续具有变革性,它们将在同样的逻辑上产生变革性影响,就像我们今天讨论的那样。所有涉及数据重要性的假设,以及固定成本和可变成本的假设,都将保持不变。如果您现在能让您的机构做好准备,那么您将为下一次变革做好准备。
Right now, there's a very specific set of technology to AI is based on that will almost certainly be different in five to 10 years. And I want you to be ready for the next one. The broader competitive part though, that's a little more frightening is that in fact, other companies, your competitors are almost certainly going through the same exercise. And so really we're on a treadmill here. And then if you're looking at AI right now, you've really got to take some action because your competitors are also going to be doing the same kind of thing.
现在,人工智能所基于的技术是非常特定的,而且在五到十年内几乎肯定会有所不同。我希望你们能为下一步做好准备。然而更广泛的竞争部分,会更加令人害怕,因为事实上,其他公司,也就是你的竞争对手,几乎肯定也在进行同样的练习。所以,我们真的是在走上一个不断前进的道路上。如果你现在正关注人工智能,那么你真的需要采取一些行动,因为你的竞争对手也会采取同样的做法。
Where can companies go wrong? You know, are you seeing misunderstanding or misconceptions that can push executives in the wrong direction as they try to figure out how to respond to this technological opportunity? There's two directions I would think about in terms of mistakes. And these are actually competing mistakes in that generally you make one or the other.
公司在哪里会出错?你知道,是否存在误解或误解可以将高管推向错误的方向,尝试弄清如何回应这种技术机遇?在错误方面,我会考虑两个方向。实际上,这些是相互竞争的错,通常您会犯其中之一。
And so the first mistake is being suspicious about the technology and suspicious about particularly privacy of data and restricting your employees from experimenting and using it. And in particular, over the next couple of years, we're going to see a large battery of new applications that are AI based coming up. And the only way you're going to be able to figure out which of those applications is good and that you should diffuse to the organization is by letting some frontline employees play around with that new technology. But that does entail the risk of letting your employees submit some data that's privacy to the company to those applications and into those models. So there's a little bit of intellectual property risk there, perhaps a lot.
因此,第一个错误是对技术和特别是数据隐私持怀疑态度,限制你的员工进行实验和使用它。特别是,在未来的几年里,我们将看到许多新的运用人工智能的应用程序出现。唯一的方式,你将能够弄清哪些应用程序是好的,并应该扩散到组织中,就是让一些一线员工尝试使用那种新技术。但这就涉及到允许你的员工向公司提交一些隐私数据以供应用程序和模型使用的风险。因此,这可能存在一定的知识产权风险,也许是很大的风险。
And the other risk here is the total opposite of that is that companies need to really think about how they're protecting intellectual property, particularly on the open internet. We're in kind of a pickle right now for intellectual property in that we've lived through an era where for, say, text-based information that there's a distinction between copyrighted and non-copy-righted text. And what is different now is that it's not just about copyrighted or not. It's also about whether or not that information is available publicly or privately.
另一个风险是完全相反的,那就是企业需要认真思考如何保护知识产权,特别是在开放互联网上。我们现在面临的是知识产权方面的困境:对于以文字为基础的信息,我们曾经生活在一个区别于受版权保护的和未受版权保护的文字之间的时代。不同之处在于,它不仅仅是关于版权是否存在的问题。它还与信息是否公开或私有有关。
So for example, a New York Times article would be copyrighted but public in that Google can index it through its search process and people can find it online. That is the data that is going to be used to train the next generation of models. And that's data that I think companies like the New York Times and elsewhere really need to think about actually blocking off from the open internet so that they can retain the ability to use it, sell that data or to train their own models with it as opposed to letting everybody train their models with it. So that's such a great point.
举个例子,纽约时报的文章会受版权保护,但是公开的,因为谷歌可以通过搜索把它编入索引,人们也可以在网上找到它。这就是用来训练下一代模型的数据。我认为,像纽约时报等公司真正需要考虑把这些数据从公开互联网中封锁起来,以便保留使用、销售该数据或训练自己的模型的能力,而不是让所有人都用它来训练模型。这是一个非常好的观点。
And I think for people who generate content, it feels like the generative AI bot have already scraped our information without our permission. And that's part of this great aggregation of language and data that's being used to generate these kind of amazing new things. And it seems like an IP question that at best is a gray area and at worst is actionable.
我认为,对于那些创造内容的人来说,似乎生成的AI机器人已经在未经授权的情况下获取了我们的信息。这是语言和数据的伟大聚合的一部分,它们被用来生成这些令人惊叹的新事物。这似乎是一个知识产权问题,在最好的情况下是一个灰色地带,在最坏的情况下是可诉的。
Right, it's definitely a gray area right now. There's a number of ongoing lawsuits and a lot of legal scholars have been really debating exactly what we should do here. Some have argued that we need to have more stringent protections to prevent people from training AI on some other person's copyrighted data. And if we don't make any change, then we are in the tough situation that companies will probably have to pull their data off or make it harder to access the general public, in which case our notion of an open internet is going to be much more challenged.
目前,这绝对是一个灰色地带。目前有很多正在进行的诉讼案件,许多法律学者一直在争论我们应该在这里采取什么措施。有人认为我们需要更严格的保护措施,以防止某人使用另一个人的受版权保护的数据来训练人工智能。如果我们不做出任何改变,那么公司可能不得不将其数据撤回或使其难以访问一般公众,这种情况下,我们对于开放式互联网的观念将面临着更大的挑战。
The internet could be much less open than we would imagine in the past. So with any of these big technological developments, there's sort of a split between techno optimists and techno pessimists. And they're usually the same arguments, you know, that well, this is going to improve efficiency and let us do new things and free up humans to do more creative things. The pessimists tend to be, well, yeah, one or two humans will be able to do creative things, but all the other humans will lose their jobs.
互联网可能比我们过去想像的要不那么开放。因此,在任何大型技术发展中,通常会出现技术乐观主义者和技术悲观主义者之间的分裂。他们通常提出的是同样的论点,即这项技术将提高效率,让我们做新的事情,并让人类获得更多创造性的事情。悲观主义者的态度是,确实有一两个人能够做创造性的事情,但其他所有人将失去工作。
Where do you come down in terms of optimism versus pessimism in terms of this technology? You know, Adi, that's a super tough question. But I guess I would probably come down on the pessimistic side right now in terms of the broader impacts. And again, I'm not of the opinion. There's any real way to stop the current process that we're undergoing in society.
在谈到这项技术的乐观和悲观的态度方面,你会倾向于哪一方呢?你知道,Adi,这是一个非常难回答的问题。但我想我现在可能会更倾向于悲观派,尤其是在谈到广泛的影响方面。而且,我并不认为有任何真正的方法可以阻止我们正在经历的社会进程。
But the pessimism from my perspective comes from what we've seen already with the digitization of society in the last 20 years. And on the productivity side, on the employment side, automation has already had a tremendous impact on at least the American workforce in terms of lost jobs in middle America and manufacturing in the retailing sector, the broad transformation of brick and mortar to e-commerce has also cost a lot of jobs as well in local communities.
从我的角度来看,悲观情绪来自我们已经看到的社会数字化的影响。在生产力和就业方面,自动化已经对至少美国劳动力市场造成了巨大的影响,失去了中美洲和制造业中的就业,同时,实体店转向电子商务的广泛转变也在地方社区造成了很多工作岗位的流失。
I'm actually more concerned about the transformation on the consumption side. Now we spend our time outside of work and I think that's actually where it's most dangerous. The analogy here would be if you look at the last 20 years, if you look at our young people, how much time they spend playing video games and on social media. And now we have the ability to at low cost generate unlimited video games and unlimited social media.
我实际上更关心消费方面的转变。现在我们在工作之外的时间花费,我认为这是最危险的。类比在这里就是,如果你看看过去20年,我们的年轻人花费多少时间玩视频游戏和社交媒体。现在,我们有能力以低成本生成无限的视频游戏和无限的社交媒体。
And in that world, the thing I would worry about is not necessarily whether or not your kids or my kids or anyone's kids can find a job. It's really whether or not they would want to ever work a job. Like if you can for very low cost just sit there and live in the digital world of unlimited perfectly personalized content, what's the point of doing anything else? And then we have to reckon with whether or not that's a world we want to live in.
在那个世界里,我担心的并不是你的孩子、我的孩子或者任何人的孩子能否找到工作,而是他们是否会想要去工作。例如,如果你只需以极低的成本坐在那里并享受数字世界中无限定制的完美内容,那么做任何其他事都没有什么意义了。然后,我们必须考虑一下这是否是我们想要生活的世界。
Andy Wu, I want to thank you for for joining us. Thank you for your insights. All right, thank you for having me. That's Andy Wu, a professor at Harvard Business School.
安迪·吴,谢谢您加入我们。感谢您的见解。好的,感谢您邀请我。安迪·吴是哈佛商学院的教授。
Before that I spoke with Chris Young, had his strategy at Microsoft. This is the last episode in our series, How Generative AI Changes Everything. To listen to the other episodes, on the impact on productivity, creativity, and organizational culture, you could find them in the HBR IDA cast feed. And for more on this topic, check out HBR's latest big idea on how to implement this new technology responsibly. That's at hbr.org slash tech ethics.
在此之前,我和Chris Young交谈,了解了他在微软的战略。这是我们系列节目《生成式人工智能如何彻底改变一切》的最后一集。想听关于生产力、创造力和组织文化影响的其他节目,可以在HBR IDA Cast平台上找到。还想了解更多相关话题,请查看HBR的最新大想法:如何负责任地实现这项新技术,网址是hbr.org/techethics。
This episode was produced by Kurt Nickish. We get technical help from Rob Eckhart, our audio product manager is Ian Fox, and Hannah Bates is our audio production assistant. Special thanks to Marine Hope. Thank you for listening to How Generative AI Changes Everything, a special series of the HBR IDA cast.
本集由 Kurt Nickish 制作。我们得到 Rob Eckhart 的技术支持,音频产品经理为 Ian Fox,Hannah Bates 是我们的音频制作助理。特别感谢 Marine Hope。感谢您收听 HBR IDA cast 的特别系列节目《生成式人工智能如何改变一切》。
I'm Adi Acations.
我是阿迪行动。
Hi, it's Allison. Before you go, I have a question. What do you love about HBR? I worked at newspapers before I came to HBR, and the thing that has impressed me most is the amount of attention and care that goes into each and every article. We have multiple editors working on each piece. They put their all into translating these ideas typically from academia or from companies in practice into advice that will really change people's lives in the workplace.
嗨,我是艾莉森。在你离开之前,我有一个问题。你喜欢HBR的什么?在来HBR之前,我曾在报纸工作过,而印象最深刻的是每篇文章都受到了大量的关注和关心。我们有多名编辑在每篇文章上工作。他们全力以赴,将学术或实践中的思想转化为真正能够改变人们在工作场所生活的建议。
If you love HBR's work, the best thing you can do to support us is to become a subscriber. You can do that at hbr.org slash subscribe IDA cast, all one word, no spaces. That's hbr.org slash subscribe IDA cast. Thanks.
如果你喜欢哈佛商业评论的作品,最好的支持方式就是成为我们的订阅者。你可以在hbr.org/subscribeidacast(一个词,无空格)上完成订阅。谢谢。