E1005 ScaleAI CEO Alexandr Wang: future of self-driving, China’s ML advantages, next major AI trends
发布时间 2019-11-29 22:17:13 来源
摘要
Scale AI CEO & Co-founder Alexandr Wang creates training data for all AI applications to improve machine learning, shares insights on the future of autonomous vehicles, China's AI advantages over US, importance of humans focusing on higher-value work & next major trends in AI
1:04 Jason intros Alexandr
2:19 Alexandr shares his personal startup history
5:17 How & why did Scale start?
8:26 What is the best example of Scale in practice? What problem are they solving?
10:44 Video demo of Scale's platform
15:34 Acquiring the scale.com domain name & insights on the unique spelling of Alexandr
17:31 How does Scale deal with data-sharing between customers?
21:34 LIDAR vs. non-LIDAR... or both?
32:29 When will we have capable self-driving vehicles from Palo Alto to San Francisco? Over/under 2030? How will gov't regulations affect self-driving?
36:03 China vs. US in the race of self-driving
41:22 Explainability in ML
47:26 Does it matter that we sometimes don't know the answer to ML systems?
51:39 Should explainability have to be proven in ML?
55:13 How should inherently biased data-sets (like US justice system) be handled via ML?
1:00:00 Importance of focusing on higher-value work
1:02:41 Are dangers of AI overblown?
1:08:50 Will "General AI" happen in our lifetime?
1:12:26 What's the next major AI trend after self-driving?
1:23:46 Does Alexandr remember a time before the Internet?
1:26:35 Jason plays "good tweet/bad tweet" with Alexandr
A HUGE Thanks to our generous Patreon supporters: Stefan Samne, Niall Dennehy, Tom Meagher, Abdel Bioud, Carlos Aguilar, Podcast Notes, Mike Jonas, Tasneem, Vin Dowling, Stefano Vettorazzi, Mikael Pawlo, Luke Mroz, Tim-Kress Spatz, Dith Na, Jeremy Davidson, Clayton Memele, Scott LaVictor, Kirstan Barnett, David Wolkin, Darius Vasefi, Liron Shapira, Hassan Albalawi, Dominik Faber, Anthony Holley, Blaine Tanner, David Bailey, Marc Cyr, Monika Norwid, Ron Berkes, Russell S Holmes, Nicholas Wickman, Ryan Morrison, Robera Geleta, Barrie Heptonstall, Eric Dewhirst, Tony Bova, Bob Wilkinson, Ashon, Victor Izmaylov, Igor Ostrovsky, Alistair Palmar, Brian Kearns, Straight Cash Homie, Gordon Gooch, Said Saad, Ron Theis, Florian Schwarz, Scott Gay, Andy Vinh, Almuhtada Smith, Dave Rimmer, Arnaud Devie, Tim Shnaider, Brian Kearns, Dave, Steve Bernat, Cynthia, Ayo Dele, Chris Swain, Jack Reichert, Zen Lenon, Pam, Nick, Miguel Solano JJ, David Daily, Mark John & Tolu!!!
https://www.patreon.com/twistartups
GPT-4正在为你翻译摘要中......
中英文字稿 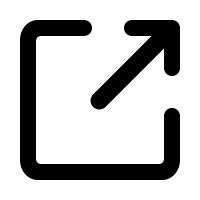
This week in Startups is brought to you by Calm. Seize the day and sleep the night with the help of Calm. The number one app for sleep. This week in Startups listeners get 25% off a calm premium subscription at calm.com slash twist. That's c-a-l-m dot com slash twist. LinkedIn. A business is only as strong as its people and every time. Go to LinkedIn.com slash twist and get a $50 credit towards your first job post. And cabbage. Get the money you need to run your small business today. Go to cabbage.com and use code twist to get a $100 credit on your first loan statement. That's c-a-b-b-a-g-e. .com and use promo code twist. Terms and conditions apply. Offer ends November 30th, 2019.
本周的初创企业板块由Calm赞助,借助Calm帮助您充分利用白天,安然入睡。Calm是睡眠领域的一款首屈一指的应用。本周的初创企业板块听众可在calm.com/twist上享受25%的高级订阅折扣。LinkedIn表示,企业的强度仅在于其员工,每一次机会都可以发挥员工的优势。请前往linkedin.com/twist并获得50美元的首个职位发布信用额度。与此同时,Cabbage则为财务资助初创企业提供便利,用twist 注册Cabbage,即可在首次贷款对账单中获得100美元的信用额度。详细条款和条件适用,优惠截止日期为2019年11月30日。
Apply for the next launch accelerator cohort. Applications are due December 23rd. Learn more and apply at launch accelerator dot com.
申请下一个创业加速器招生班。申请截止日期为12月23日。了解更多信息并在launchaccelerator.com上申请。
Hey everybody welcome to this weekend Startups. I'm your host Jason Calcanis and this is the podcast where we talk to founders about their vision for how they want to change the world and today. Kind of an interesting cat on the program. His name is Alexander Wang. He is a CEO and co founder of scale AI. You got the domain name scale dot com. That's like a million dollar domain name. We'll find out what he paid for it later. And I guess the thing that most people would think is remarkable is candidly that you've raised over a hundred million dollars in your last round of funding. That's a lot of money. It's quite a bit. It's quite a bit of money from founders fund. And that I think you're 22 years old. 22. Yeah. 22.
大家好,欢迎来到本周末初创企业节目。我是您的主持人Jason Calcanis,这是一个我们与创始人谈论他们如何改变世界愿景的播客节目。今天我们节目中有一个非常有趣的人,他的名字是Alexander Wang。他是scale AI的CEO和联合创始人。他们拥有一个价值数百万美元的域名scale.com。我们稍后会了解他支付了多少价格。而且,我想大多数人会认为最值得注意的事实是,在他的最后一轮融资中,他筹集了超过1亿美元的资金,这是一笔很大的资金。这笔投资来自创始人基金公司,而他只有22岁。22岁,是的。
So that's annoying to be young and successful because then every interview starts with your age. A little bit. It's annoying. I had it happen to me. It was like 23 year old publisher of cyber serve or 25 year old publisher of Silicon. And I was like, what is my age matter? Now I tell you when you hit about 3540, they don't mention it anymore. Because they're like, wow, you're 40. You should be doing interesting things or be successful in the world.
这就是年纪轻轻却非常成功的烦恼,因为每次采访都会以你的年龄开始。有点烦人。我也经历过这种情况。比如:“23岁的网络服务发布商”或“25岁的硅谷发布商”。我当时想,我的年龄有什么关系呢?但是当你到了35或40岁时,他们就不再提及年龄了。因为他们会想,“哇,你都40岁了,你应该在世界上做出有趣的事情,或者取得成功。”
But you've been running this company since you were how old? 19. 19. Now were you a teal fellow or something? How did you get into the game? No. I, so I have a fun little history. I grew up in Los Alamos, New Mexico. So we're my parents or physicists. And they worked at the National Lab in Los Alamos. Yeah. I tell people about that lab. It was the lab where the atomic bomb was originally built. So the Manhattan Project started in Los Alamos. It was very secretive at that time. Yeah. And where did they call that lab?
但是,你从多少岁开始经营这家公司?19岁。19岁。那你是一位天才吗?你是怎么进入这个游戏的?不。我有一个有趣的小历史。我在新墨西哥州洛斯阿拉莫斯长大。我的父母都是物理学家,他们在洛斯阿拉莫斯的国家实验室工作。是的。我告诉人们关于那个实验室。那是最初制造原子弹的地方。曼哈顿计划始于洛斯阿拉莫斯。那时非常神秘。是的。他们如何称呼那个实验室?
Los Alamos National Lab. Los Alamos National Lab. Pretty boring. Yeah. And then I, and it's a government sponsored lab. Exactly. Yeah. Totally government funded. And then growing up in high school, I did a bunch of programming. I did all these coding competitions. And I was getting recruiter in bounds in high school.
洛斯阿拉莫斯国家实验室。洛斯阿拉莫斯国家实验室其实挺无聊的。是的。它是一个政府资助的实验室。确切地说,它完全是由政府资助的。在我上高中的时候,我做了很多编程。我参加了所有这些编程竞赛。在高中时期我就有了招募的机会。
So after high school, I actually came out here to work. I worked at this company, Kora, for a couple of years. No, we know. They do a Q and A site. Yeah, Q and A site. How did you get that job? You just applied and they saw your code. And they were like, okay. They recruiter in bounds because I was on these, I was an anonymous person on these coding competitions. And then you could just go into a coding competition. Nobody knows your age. Yeah.
高中毕业后,我来到这里工作了。我在Kora这家公司工作了几年。我们知道,他们是一个问答网站。你是如何得到这份工作的?你只是申请并展示了你的代码,然后他们就决定雇用你了。这是因为我参加过编程比赛,当时我匿名参赛,所以任何人都不知道我的年龄。
And then you teach yourself how to code? Well, of course, the internet taught me how to code. So I guess the internet just looked it up. You found courses online on YouTube or. Yeah. I don't, it's hard to remember. I think I just googled around anyway.
然后你自学编码了?当然了,因特网教会了我如何编码。所以我想,应该是因特网搜索了一下。你在YouTube上找到了在线课程或者别的什么?是的。我不太记得了。我想我只是随便在谷歌上搜索了一下。
So I worked at Kora for a couple of years doing engineering, infrastructure, et cetera. And then no college. Well, then I went to college after that. Ha. I went to MIT and then got basically bored after a year and started a year and started scale. So you left? I left. Yeah. So your parents are heartbroken about you leaving MIT at that time?
我在Kora工作了几年,主要从事工程和基础设施等方面的工作。然后我没有上大学,之后才去上大学。哈,我去了麻省理工学院,然后在一年后就感到无聊了,便开始了自己的公司。所以你离开了麻省理工学院?是的,我离开了。那你的父母对你当时离开麻省理工学院感到心碎了吗?
For now, yeah, for now. They're still heartbroken. Even after you raised 100 million, my god, these parents are heart-standers. Yeah, yeah, I mean, it's a meme, but it's true. Yeah. All right. Listen, mom and dad, he's going to build a building at MIT with his name, with your name on it, the family name on it. So give, cut him a break. It'll be okay. He'll be a professor emeritus at some point. Yeah.
现在来看,是的,现在。他们仍然心碎。即使你筹集了1亿美元,我的天啊,这些父母仍然很难过。是的,是的,虽然这是一个梗,但它是事实。好了,听着,爸妈,他将在麻省理工学院建造一座以他自己的名字和你们家族的名字命名的大楼。所以给他一点时间吧。一切都会好起来的。他最终会成为荣誉教授的。是的。
Well, one can hope. One can hope. No, it wouldn't be a professor emeritus. That would be somebody who left. You'd be like an honorary professor. Yeah. That would probably, that would be the way to piece my parents. Yeah, because you went to work for a couple of years, then you went to MIT. That is not the way to do it, because you're going to be sitting there and everything's going to be going so slow and everything's theoretical. Yes. That's exactly what happened. Yeah, that's a. You went from running fast, you're driving race cars, and then they like, put you in the pit and the go-carts, and they're like, here's some go-carts.
希望如此。希望如此。不会是名誉教授,那样的话,表示你离职了,你会成为一位荣誉教授。嗯,这可能是让我的父母高兴的方式,因为你工作了几年,然后去了麻省理工学院。这不是一个好的方式,因为你将坐在那里,一切都会变得缓慢和理论化。是的,这正是发生的事情。是的,你从高速运行转变为开赛车,然后他们让你停在车道旁,开小轿车,告诉你:“这是一些小轿车。”。
Yeah. And if I'm being honest, I think the. I think the speed, the slower speed of school is sort of what got me agitated enough to eventually start the company. I think there's an alternate world where I continued working at companies after Cora for many, many years. So what was the vision for scale? How did you get the idea? When did you have the idea? So our mission is to accelerate the development of AI applications. I think we fundamentally view AI and machine learning as kind of a once-in-a-generation shift in technology. It might be a once-in-a-species, by the way. Yeah, I mean, it's depending on how this goes. Yeah, we'll see. It's obviously very hyped, but I think we hold that belief quite strongly.
嗯。如果我诚实地讲,我认为学校较慢的速度最终让我感到不安,从而开始公司。我认为如果在Cora之后继续在公司工作,可能会有一个另外的世界。那么规模的愿景是什么?你是如何得到这个想法的?你是什么时候有这个想法的?所以我们的使命是加速人工智能应用的发展。我认为我们从根本上将人工智能和机器学习视为一种技术上一次性的转变。这可能是一次物种间的转变,顺便说一下。是的,取决于这件事的进展方式。是的,我们会看到的。显然,它受到了很大的炒作,但我认为我们非常坚定地持有这样的信念。
And do you think it says big as the internet or bigger? The internet itself took billions of people and connected them for the first time? Yeah, I say bigger than the internet and bigger than the silicon chip being, you know, CPUs being created. I think it's more comfortable to the advent of computing than it is to the advent of the internet. Because it's an enabler of all these things that previously had to be done by humans and now can be done by machines. Got it. Okay. So if you had to rank them AI, computer, internet, or maybe computer AI, internet. We'll see what happens between AI and computer. We'll watch, we'll watch, intently over the coming decades. Computers did change our day-to-day lives pretty significantly. Yeah, well AI will as well, with autonomous vehicles and all these assistance on your phone. And I think it'll go on and on and on. There's a lot more applications.
你认为它比互联网还要大吗?互联网本身将数十亿人连接在一起,这是首次实现了吗?是的,我认为它比互联网还要大,比如创造CPU这种计算机的技术。我认为它跟计算机出现相比更符合引领计算时代的趋势。因为它可以使得以前需要人类完成的所有事情现在都可以由机器完成。好的。那么,如果你必须排名AI、计算机、互联网,或者可能是计算机AI、互联网?我们将看看AI和计算机之间会发生什么。在未来几十年里,我们将密切关注。计算机确实显著改变了我们的日常生活。是的,AI也将如此,例如自动驾驶汽车和在手机上提供的所有这些帮助。我认为这将会持续并有更多的应用。
All right, so that's the backdrop and then you have some insight on what was holding back AI or something? Yeah, so the big reason I went back to school actually was to. Was this study machine learning? So I was at Core. It was a very machine learning driven company. But I didn't have that strong and academic backing. And so I went to back to MIT to really study this more deeply. And then I had all these ideas of products I wanted to build. But there was sort of an elephant in the room problem, which was how are you supposed to get the data to build these machine learning models that you could integrate into a product?
好的,这就是背景,你有一些关于AI受阻的见解,对吧?是的,我回学校的主要原因就是为了学习机器学习。我之前在一个非常注重机器学习的公司工作,但我自己没有更深入的学术支持。所以我回到MIT更深入地学习。然后我有很多想要开发的产品的想法。但是有一个大问题,那就是你要如何获取数据以构建这些机器学习模型,然后将其集成到产品中呢?
So give me an example of that. And then define for the audience that's not super familiar with the term machine learning. What is the difference between saying that we're machine learning and AI for people who hear them together? Yeah. How would you explain what each one is? Yeah, so machine learning is a subset of AI. It's sort of a particular kind of AI where in particular we're training these. You sort of are writing programs that are able to do various things that humans normally do or various tasks that traditionally require human judgment. And they're able to do that by feeding them lots of data and sort of a particular brand of AI, if you will.
给我举个例子。然后对那些不是非常熟悉机器学习术语的观众进行定义。对于那些同时听到“机器学习”和“人工智能”的人,如何解释两者之间的区别?是的。您会如何解释每个是什么?是的,机器学习是人工智能的一个子集。这是一种特定类型的人工智能,其中特别是我们正在训练这些。您可以编写程序,使它们能够执行人类通常执行或传统上需要人类判断的各种任务,通过向它们提供大量的数据来实现。如果您愿意,这是一种特定的人工智能品牌。
So we pick a task that humans have done with their brains, which is some combination of logic, intuition, who knows what human brains are, how they're making decisions. Exactly. A lot of debate about that. So you feed a bunch of data to a machine learning algorithm. Yep. And then it gives you an answer that it thinks would approximate a human's answer or the best answer. It thinks it would approximate the best answer, but the only way it's going to know what the best answer is is through all this data that humans have created.
所以我们选择一个人类用他们的大脑完成的任务,这涉及到逻辑、直觉以及人类的大脑如何做出决策的混合。确切地说,对此存在着许多争论。因此,我们将一堆数据输入到机器学习算法中。是的。然后,它会给出一个它认为最接近人类答案或最佳答案的答案。它认为它能够近似最佳答案,但是要知道最佳答案的唯一方法是通过人类创建的所有这些数据。
Got it. Let's come up with the most illustrative example. Let's an example that when you gave this example to VCs, they threw money at you. Well, the one that has really captivated the world's attention is autonomous vehicles, right? Sure. And it's a compelling example because first, nobody likes driving, but also driving is very unsafe. And there's a lot of risk in driving. Sure. And so the- A lot of stake. Yeah, exactly. And so the captivating sort of machine learning model is one that can take in all of the camera data and other sensor data from the vehicle. Understand everything that's going on around it, something that's very easy for your eye, but currently, or at least before machine learning was very difficult for machines. And then can determine the best path to take and figure out how to drive on its own, basically.
明白了。让我们举出一个最具有说明性的例子。让我们举一个这样的例子,当你向风险投资家介绍时,他们会向你投钱。好吧,真正吸引全球注意力的一个例子是自主车辆,对吧?当然。这是一个很有说服力的例子,因为首先,没有人喜欢开车,而且开车非常不安全。而开车存在很多风险。当然。因此,迷人的机器学习模型是一个可以从车辆摄像头和其他传感器接收所有数据的模型。理解周围发生的所有事情,这对你的眼睛来说非常容易,但是对于机器来说,在机器学习之前至少非常困难。然后可以确定最佳路径并找出如何自主驾驶。
Got it. So we see the lane markers, double yellow markers, double white markers on the highway. We know, keep the car between those two lines. Exactly. As smoothly as possible. Yeah. We see somebody deviate from their lane into ours. We know to slow down, give them some room, maybe they're drunk. Machine doesn't know that inherently. We have to teach it that. Exactly.
明白了,我们在高速公路上看到车道标线、双黄标线、双白标线,我们明白要保持车辆在这两条线之间。是的,尽可能平稳地行驶。对,如果我们看到有人超出自己的车道进入我们的车道,我们知道要减速,给他们一些空间,也许他们喝醉了。但机器本身不知道这些。我们必须教会它这些。没错。
And what does scale.com do that? Tesla and Waymo don't already do. Yeah, exactly. Because they're solving that problem. Do they use your software and do they need to? Yeah, so-so. That's a great question.
那么,scale.com是做什么的呢?它解决的问题是特斯拉和Waymo已经解决了吗?是的,正是因为他们正在解决这个问题。他们是否使用你们的软件,他们需要吗?这是一个很好的问题。
So the core problem, as you just laid out, is that machines don't know what to do, unless they have data that actually tells them what they're supposed to be doing. And so what that means is one of the huge bottlenecks for machine learning is data. It ends up being like data that tells these algorithms, tell these models what they're supposed to be doing. And that's where scale comes in.
因此,正如您所说的那样,核心问题在于机器没有知道要做什么,除非它们具有实际告诉它们应该做什么的数据。这意味着机器学习的一个巨大瓶颈是数据。最终,就像数据告诉这些算法、模型它们应该做什么一样。而这就是规模的作用。
What we are is sort of this data refinery, if you will. We accept a bunch of raw data from our customers. We go through and process it, and we sort of, we tell the machine what it should be doing. For example, given an image taken by a self-driving car, we would outline these are where the people are. These are where the cars are. These are the lane markings, etc. So that over time, these algorithms can learn those things. You have a video of that. You can share it right here.
我们类似于一个数据精炼厂,我们从客户那里接收各种原始数据,然后对其进行处理和加工,告诉机器它应该怎么做。比如说,对于一辆无人驾驶汽车拍摄的图像,我们会标明哪里有人,哪里有车,哪里有车道标线等等。这样,随着时间的推移,这些算法就能够学习到这些东西。你还可以在这里分享这个视频。
So here's a video of Pacific Street. And you have a better eye than I. I'm going to take a guess. That's one of those streets. And I see you are highlighting cars, you're highlighting people. And the machine is figuring out, okay, that's the approximate shape of a Dodge pickup truck. That's a Toyota Prius and these look like the silhouettes of people. But that's a human telling the machine that's what it is for now. Yeah, exactly.
这是一段关于太平洋街的视频。你的眼力比我强,我猜测那是其中一条街道。你突出了汽车、人,那个机器则会计算出这是道奔驰皮卡车的近似形状,或是丰田普锐斯车,还有人的轮廓。但其实这是有人告诉机器现在这个是什么,没错,就是这样的。
So the core way that our whole pipeline works is that a lot of work is done behind the scenes by machines and our own AI models originally. And then humans basically give input and correct mistakes to make sure that the end data is extremely accurate. Because that ultimately is what's important for the safety of these systems and for low bias, etc.
因此,我们整个流程的核心是通过机器和我们自己的AI模型在幕后完成了大量的工作。然后人类基本上提供输入并纠正错误,以确保最终的数据非常准确。因为这最终对这些系统的安全和低偏见等非常重要。
All these things that are needed, imperative for machine learning to be formed. So you would go to a customer, is Waymo or Uber a customer? Yeah, exactly. They're both customers. They're both customers. Got them. And you could say that, it's public knowledge. Yes.
所有这些都是必需的,是机器学习形成不可或缺的。所以你会去找客户,像是 Waymo 或 Uber?对,没错。他们都是客户。他们都是客户。对,你可以这样说,这是公开的知识。是的。
Got it. Okay. So they're both customers. So they would give you videos of their cars driving and then you would annotate it for them and put that data into a database somehow. This is exactly right. So for example, if they gave us a video like this, you'll see originally the first step was a human drawing a box. Yep.
明白了。好的。所以他们都是顾客,他们会给你他们的车辆驾驶视频,然后你会为他们注释这些视频,并将数据以某种方式存入数据库。这完全正确。例如,如果他们给我们一个像这样的视频,你就会看到最初的第一步是一个人画了一个框。是的。
And then a machine learning model that's already pre-processed through all this data has determined the path of that vehicle over time. Right. And then we confirm that all this is correct and then send that data over to the customer and they train machine learning models on top of it.
然后,一台已经经过所有这些数据预处理的机器学习模型已经确定了该车辆随时间的行驶路径。是的。然后我们确认所有信息都是正确的,然后将这些数据发送给客户,并在其上进行机器学习模型的训练。
Got it. And this is how I guess one of the cars got fooled. Somebody drew on the ground like an arrow turning and a car followed the arrow, which our human would do too. But they basically drew a turning signal to see if like it would fool a self-driving car. And of course it did. I didn't see this news, but I would believe that that is how that's what would happen basically.
知道了。我猜这就是其中一辆车被欺骗的原因。有人在地上画了一个箭头转弯的路标,一辆车沿着箭头走了,就像我们人类也会这么做。但他们实际上是画了一个转弯信号,看看是否会欺骗自动驾驶汽车。当然,它确实欺骗了。我没有看到这个新闻,但我相信基本上就是这样发生的。
Yeah. And that's what would happen to a human by the way. So I thought that was the stupidest prank ever. They're like, look, we can fool a machine that's driving cars to make a wrong turn. It's like you would also fool a human to make the same right turn. It's like taking the do not enter sign off of the off ramp and putting on ramp on it. Like congratulations like fight club. You just did like some crazy stupid prank. Yeah, that's exactly right. I mean, a lot of ways they will have some of the same challenges that humans have in driving.
对啊。这也是人会发生的事情。所以我觉得那是最愚蠢的恶作剧。他们说:“看,我们可以愚弄开车的机器人,让它走错路。”这好像也会让人去走同样的路。就像把禁止驶入的标志拿掉,换上前进的标志。祝贺你,就像《搏击俱乐部》一样,你刚刚做了一些疯狂愚蠢的恶作剧。是的,完全正确。在很多方面,他们在驾驶方面会面临和人类相同的挑战。
All right. When we get back, I want to understand if you are storing all this data and annotating it for one company or is this some sort of grand plan to have it go across multiple companies. So everybody doesn't have to recreate the wheel when we get back on this week in startups. So to speak.
好的。回来后,我想了解一下你是将所有这些数据存储和加注批注只给一家公司,还是有某种宏伟计划使其可以用于多家公司。这样我们每次回到这周的创业公司时,就不必重新发明车轮,所谓的。
Are you struggling to sleep while you're not alone one in three US adults does not get the sleep that they need and not sleeping enough. That affects all your cognitive function. Think about it like learning and problem solving and decision making. All these things we do as founders every day.
你是否在入睡方面感到困难?你并不孤单,美国三分之一成年人都没有获得他们需要的睡眠。睡眠不足会影响所有认知功能。想象一下,学习、解决问题和做出决策等活动,这些都是我们作为创业者每天做的事情。
Sleeplessness causes people to also be prone to more accidents, weight gain and depression. But when we sleep and we get that great night sleep, you know what I'm talking about. Then you're more focused and relaxed and you're actually happier. So that's why we're partnering with com com com the number one app for sleep.
失眠会使人更容易遭受事故、发胖和抑郁。但当我们睡得好,得到美好的夜间睡眠时,你知道我在说什么。然后你会变得更加专注、放松,实际上更加快乐。因此,我们正在与睡眠应用程序com com com合作。它是排名第一的睡眠应用。
You're going to get a library of programs from com that are designed to help you get the sleep your brain and body needs. Like soundscapes and over 100 sleep stories and I do these with my kids and they love it and I do it with myself. And it is amazing and here's my associate, Presh, who has been having trouble sleep because his boss is too intense. And he goes through it and he finds some nonfiction and he's looking.
你将获得从com获取的程序库,这些程序旨在帮助你获得大脑和身体所需的睡眠。像音景和超过100个睡眠故事,我和我的孩子一起做这些,他们喜欢它,我自己也很喜欢。这太神奇了,这是我的同事Presh,他因老板过度紧张而一直无法入睡。他翻阅着非虚构类作品,正在寻找解决方法。
Ooh, a cruise on the Nile, some Matthew McConaughey watch Matthew McConaughey reading some sleep stories. He looks at the ASMR painting beauty in the beast and then he looks at sleep and he does lullaby to the star. So relaxing. So here's your call to action. This week and startup listeners will get 25% off a com premium subscription at com.com slash twist.
哦,尼罗河上的游轮,马修•麦康纳看马修•麦康纳读入眠故事。他看着“美女与野兽”中的ASMR画作,然后关注睡眠,并给星星唱催眠曲。太放松了。所以,这是你的行动呼吁。本周,创业听众可在com.com/twist享受com高级订阅25%的折扣。
That's right com.com slash twist. C-A-L-M dot com slash T-W-I-S-T. 40 million people have downloaded a com and it was apples 2017 after of the year. Find out why at com.com slash twist. Thanks again to com.com. I'm an investor in the company. I love the company and I'm so proud of the work the team over there is doing. It's just such an amazing app and such a great story.
没错,是com.com/twist。CALM.com/twist。已有4000万人下载了这个应用,它曾被苹果评选为2017年最佳应用。到com.com/twist了解详情吧。再次感谢com.com。作为该公司的投资者,我热爱这家公司,也非常为那里的团队工作感到自豪。这是一个非常优秀的应用,背后也有一个很好的故事。
Okay, let's get back to this amazing episode. Alright Alexander Wang is here blah blah blah 20 something year old who cares. He's young, he's smart. Don't worry. It's yeah, it's all get old eventually. You'll get old eventually. You'll get people will stop saying that you're 22 and whatever.
好的,让我们回到这个令人惊叹的情节上。好的,亚历山大·王在这里,呃呃呃,20多岁,谁在乎呢。他年轻,他聪明。不用担心,我们终究会变老。你最终也会变老,人们会停止说你是22岁之类的。
And he is building scale dot com. That's like a six or seven figure domain name. No comment no comment on the price of that domain. It's not cheap. It's in the dictionary and it's less than six letters. So it's not cheap. It was not it was not inexpensive. No, not inexpensive. But boy, a good domain name does help the branding. Does it not? Well, I guess I guess we'll see that'll happen in the out years. We'll see what happens.
他正在建立scale.com。这是一个价值六位或七位数字的域名。关于这个域名的价格没有评论。它不便宜,因为它在字典中并且拥有不到六个字母。但好的域名确实有助于品牌宣传。我们将在未来看到它是否发挥了作用。
It's baller. Everybody is like, oh, what's your email? I was like Alexander at scale dot com. Just leave out an e. Pick the right to leave out. So you wait, your name is Alexander, but you're missing the e between the D and the R. Yeah, this is actually right. It's my typo on your birth certificate. My given name intentional, very intentional. So like a joke by your programmer parents in some way?
这是很酷的。每个人都会问,你的电子邮件是什么?我会说是Alexander at scale dot com。只需删掉其中一个“e”字母,选对该删的字母即可。所以,你的名字是Alexander,但是在D和R之间没写“e”字母?是的,这是正确的。这是我的出生证明中的一个笔误。我的名字是有意为之的。似乎是你的程序员父母开了个玩笑?
My parents wanted eight letters in my first name, because they're Chinese. And that's good luck. Eight eight is very good luck. Yes, extremely good luck. Yeah. So literally did that for good luck. And it worked. Yeah, it worked. I mean, I have a friend I play poker with. He's extremely superstitious in his Chinese. Yeah, buys in when you play poker.
我父母想让我的名字有八个字母,因为他们是中国人。在中国文化中,八是非常幸运的数字。所以他们为了求好运而这样做了。而且,这真的有效。我曾经有一个玩扑克牌的朋友,他非常迷信并且也是中国人。每次玩扑克时,他都会买到好牌。
If he's not playing well, if he's losing, he buys in for $88,000. That's that's not right. Does not look at his cards and pushes the chips in blind in poker. Texas hold them. And then two or three of us will just call him if we have an A or whatever and then he wins every time. Oh, wow. For $88,000. I've seen him do it five times in a row. He's a legend in Los Angeles. Yeah, that's what I can say. He sounds really good.
如果他打得不好,输了,他会买入88000美元。这不对。他不看底牌,在德州扑克中盲注。然后我们两三个人如果有A或其他好牌就跟他下注,然后他每次都赢。哇,88000美元。我看过他做了五次。他是洛杉矶的传奇。是的,这就是我能说的。他听起来真的很厉害。
Yeah. I love to play poker. It's pretty amazing. Because we think about the worst-standing poker is like typically 80, 20 or something like that. So you even have a chance. But yeah, it's quite a thing to say. So before we left for the break, I wanted to know if you're doing all this data, are all these companies programming their algorithms and data sets in a silo over and over and over again?
是的,我喜欢玩扑克牌,这真的很有趣。因为我们认为最差的牌一般都是80:20之类的比例,所以你还有机会获胜。但是,这是一个相当说服力的陈述。所以,在我们休息之前,我想知道是否所有这些公司都在孤立地不断编程他们的算法和数据集?
There's no sharing across these companies. Yeah, that's exactly right. That's crazy. Well, because they all want to win in the end. So they're all not sharing their data. Well, I think this is what kind of comes down to is what is IP in the machine learning context. And I think it kind of-
这些公司之间没有进行数据共享。是的,完全正确。这很疯狂。因为它们最终都想获胜,所以它们都不会共享他们的数据。我觉得这归结于机器学习背景下的知识产权的定义。
Controelectural property. Exactly. And it would be equivalently crazy if every company in the valley were to just develop their code out in the open. I think it's like- Like open source. Exactly. There's a little bit of open source. But for the underlying technology people do open source. But Google's not giving their algorithm away. Yeah, exactly. Or open sourcing that. And so ultimately, it's not that crazy.
反向思考的财产权。确实。如果谷中的每个公司都在公开开发他们的代码,那同样会很疯狂。就像开源一样。对于底层技术,人们会有一点点的开源。但 Google 并没有公布他们的算法。所以,归根结底,这并不是很疯狂。
I think it means that it means that there's an incentive to do things that are novel and interesting and produce value. Okay, so you get the variability of 10 different people trying 10 different data sets. But you lose the efficiency of 10 different people working off a common data set. Yeah, I mean, the core- by the way, the core of your stance on this, it's like it's a very similar thing to whether or not you believe in just free market economics in general. Yeah.
我认为这意味着,做一些新奇有趣而且有价值的事情会获得激励。好的,你可以得到十个人尝试十个不同数据集的变异性。但你会失去十个人在一个共同的数据集上工作的效率。是的,我的意思是,你这个立场的核心,实际上就类似于你是否相信自由市场经济一般。
You have a lot of people running around, running in the same direction, running in different directions. And it's a like- And if you believe in that approach versus a sort of planned economy where there's high efficiencies with maybe low variance and low low chaos, then I think it's really fine. Yeah, but because you have 10 different people competing with 10 different data sets and there are big prizes at the end, like whoever solves stuff driving wins $100 billion or $1 trillion. Yeah, you've incentivized a large groups of influential pools of capital to pursue it. Yeah, exactly.
你周围的人很多,有些人朝同一个方向奔跑,有些人朝不同的方向奔跑。如果你相信这种方法,相比于计划经济,这种方法高效、方差小、混乱度低,那么我觉得没什么问题。因为你有10个不同的人竞争着使用不同的数据集,最终会有巨大的奖励,比如解决有驱动力的问题会获得1000亿美元或1万亿美元的奖金。你已经激励了一大群具有影响力的资本池去追求这个目标。
And there is an open source company, isn't there? There's somebody doing an open source company. Do you know about this company? There's- They're going to open source the data and do exactly what I'm talking about. I forgot the name of it. Uh, it is not a new idea. And in fact, in the research community, um, people open source the data quite commonly. Really? Yeah, so the- The- A lot of people say the- The start of all of the machine learning, uh, particularly the deep learning life cycle was this large A- So called ImageNet, which was- ImageNet. Yeah, which was published by this Stanford professor, Fei-Fei Li, um, who, uh, basically produced this large data set, and then it really kicked off this sort of, uh, machine learning, deep learning hype, uh, hype.
还有一个开源公司,是吗?有人在经营这个开源公司。你知道这家公司吗?他们将开源数据,并做我所说的事情。我忘了它的名字。这不是一个新的想法。实际上,在研究界,人们很常常开源数据。真的吗?是的,很多人说,所有机器学习特别是深度学习生命周期的开始是由这个斯坦福教授Fei-Fei Li发布的大型ImageNet数据集引起的。他基本上产生了这个大数据集,然后真正推动了这种机器学习、深度学习的热潮。
Wow. So because he got all of those open source creative comments, images up there, and then had everybody train them, you had a trained set? Yes, exactly. So she had a- She- She- She published this large- She and her lab published this large, uh, this extremely large data set of- Millions of images, uh, classified with what- What was in those images? So- This is an orange, this is an apple. Yeah, this is an orange apple, this is a cat. There- There's some rare ones, like this is a rare kind of fish, et cetera. This is an cat eating an orange. Uh, not quite that detailed, so this is, uh, this is- This was the beginning mind you. Yeah. Um, and, uh, and basically that- That created this open source data set that then sort of the whole world could work on top of.
哇,因为他把所有这些开源的创意评论和图片放在那里,然后让每个人都去训练它们,所以你就有了一个经过训练的集合?没错,就是这样。所以她和她的实验室发表了一个非常大的数据集,其中包含数百万张图片,并且按照图片中的内容进行了分类。比如这是一个橙子,这是一个苹果,这是一只猫等等。还有一些罕见的物种,比如一种罕见的鱼等等。并不是像一只猫吃着橙子这样的详细信息,这只是刚开始的阶段。基本上,这创建了一个开源的数据集,全世界的人都可以在上面进行工作。
So that would be the example of centralization, open source being perhaps something between, let's say, a socialist communist, singular government approach versus a democratic capitalist approach. There was open source, which kind of sits somewhere between the two, doesn't it? Yeah, ultimately the- The, um, actually maybe it's socialist. I'm trying to figure it out. Uh, yeah. I- I do think that, um, open source is- I'm not going to comment exactly how- How to line these- In these economic situations, but, um, But I think- I think very much so like in general, the trend of providing some like core underlying infrastructure, Yeah. For a large group of people, or a large community of people who are all iterating or building on top of that infrastructure is very valuable.
这就是集权化的例子,开源项目可能处于社会主义共产主义、单一政府方法和民主资本主义方法之间。开源项目位于这两者之间。嗯,最终这可能是社会主义。我正在思考。是的,我认为开源项目非常重要,可以为一个大群体或大社区提供一些核心基础设施,这些人都可以对这些基础设施进行迭代或进行改进。这一趋势非常有价值。至于具体的经济情况,我不会评论。
In self-driving, is it the video of what's on the road, or is it some other way of recording it that is the most effective? So we have LiDAR, Google Bet the Farm on LiDAR, Elon Bet the Farm with Tesla on video cameras. Everybody thought Elon was an idiot. Um, turns out, I'm hearing now that people are starting to think the cameras are getting so good, and the data set's getting so good, that cameras will win the day in LiDAR will be ridiculously unnecessary. So we actually- Which is true.
在自动驾驶技术中,记录路面情况最有效的方法是什么?是路面视频还是其他方式?我们有激光雷达,Google把赌注放在了激光雷达上,而埃隆-特斯拉用的是视频摄像头。当时大家都认为埃隆很傻。但现在听说人们开始觉得摄像头的质量越来越好,数据集也越来越好,所以摄像头会胜出,激光雷达将变得非常不必要。这是真的。
Uh, so my personal opinion, uh, is that I do think both sensors have different advantages, and fundamentally they're- they're both very good in different scenarios. So if you explain. So, uh, so we actually- we published this blog post about this, because we obviously see a lot of LiDAR data, a lot of image data. What's the name of the post? Uh, I think it's called LiDAR versus cameras, Elon versus Larry. I think it's called LiDAR versus cameras, or something like that. I will search for it at scale.com.
嗯,就我个人的观点而言,我认为两种传感器都有不同的优势,基本上它们在不同的场景中都非常好用。因此,如果您解释一下。所以,我们实际上发布了一篇关于此事的博客文章,因为我们显然看到了很多激光雷达数据和图像数据。这篇文章的名称是什么?嗯,我想它叫做“激光雷达对相机,以埃隆对拉里的比较”。我想它叫做“激光雷达对相机”,或者类似的东西。我会在scale.com上搜寻它。
But, uh, yeah, one of- I think there's- there's different scenarios where both are good, right? So, uh, LiDAR is very good. First of all, at giving you a 3D map of everything around you, that turns out to be very valuable, if you're planning very careful maneuvers, and it's very- it's very reliable in giving you that 3D map. Yeah. It makes a map that is incredibly well refined. Uh, Here it is. Is Elon wrong? How about LiDAR? Is Elon wrong about LiDAR? Exactly. There you go.
嗯,我认为有不同的情况下,两者都是很好的选择,对吧?那么,激光雷达非常棒。首先,它可以为你提供周围一切的三维地图,这非常有价值,如果你在计划非常小心谨慎的机动时,它非常可靠地提供给你那个三维地图。它制作的地图非常精细。Elon错了吗?激光雷达呢?Elon对激光雷达误判了吗?没错。就是这样。
It's also very good in, uh, in dark scenarios, because the LiDAR has created its own light, so you- you know exactly what's going on around you. Um, but it's- it's bad in other scenarios.
它在暗场景下也非常好,因为LiDAR可以产生自己的光,这样你就可以确切地知道周围发生的事情。但是在其他场景下,它就不太好用了。
It's bad when there's a lot of fog, etc. Why is it- Why is LiDAR bad in snow and fog? Uh, because it- it's shooting out these little lasers, and, uh, snow and fog are both very reflective, and basically screw with how these later- Got it. So it makes an imperfect model in the situation.
有大雾等天气条件时不利于人们的行动。为什么激光雷达在雪和雾中不好用呢?因为它会发射出小的激光,而雪和雾都具有很强的反射性,会对激光雷达的测量造成影响和干扰。所以,在这种情况下会得到一个不完美的模型。
In the case- Exactly. An imperfect 3D model, uh, or at least one that you'd care about. So, for example, if there's a plume of smoke, LiDAR will, uh, will catch the plume of smoke, but you're actually- it's fine if you drive through a plume of smoke. Right.
在这种情况下,就是这样。一个不完美的三维模型,或者至少是你关心的一个。例如,如果有一股烟雾,LiDAR会捕捉到烟雾的位置,但如果你开车穿过烟雾是没有问题的。
Yeah. Okay. Um, and so, can machine learning now, is it able to reconcile when both systems are on effectively, to know, hey, the LiDAR is built as perfect model, but the LiDAR is hitting something that could be smoke in the cameras like- uh, or it could be a brick wall that just suddenly appeared, and the cameras like, no, it's smoke. We can tell because cameras are better at detecting smoke and- snow.
是的。好的。那么,现在机器学习能够有效地协调两个系统吗?例如,LiDAR建立了完美的模型,但是LiDAR碰到的可能是烟雾或者是突然出现的砖墙,而相机会认为这是烟雾。我们能够告诉机器学习这个区别,因为相机比较擅长识别烟雾和雪。
Yeah, that's why- that's why fundamentally you want both, right? So, um, so both is the best system. Both is definitely the best system. Because, so, uh, the- the place where the camera stuff breaks down is that, right now, if you were to look at, uh, at sort of the state of the art, computer vision models that work on cameras, um, they're accurate, maybe like 99% of the time. Which sounds like a lot, uh, but not 99.99%, 99% of the time.
是的,这就是为什么,基本上你们都想要两者兼备,对吧?所以,两者都是最好的系统。两者肯定是最好的系统。因为目前相机技术存在的问题是,如果你现在看一下相机上运行的最先进的计算机视觉模型,它们的准确率可能只有99%。听起来好像很高,但并不是99.99%,而是只有99%。
99.99% of the time. 99.99% of the time. 99.99% of the time. 99.99% sounds like a lot, until you drive 100 hours. Yeah. 100 hours. Exactly. 100 hours is not. And that happens to be the second where a bolder rolls into the street. Exactly. Yeah. So, it's, um, it's pretty important that you get like these, these asymptotically difficult levels of quality. And you can do that. You can actually do that if you have multiple sensors that have different strengths and different weaknesses and can sort of play off of one another when you need it. Which is why you want both. You really want both.
99.99% 的时间。99.99% 的时间。99.99% 的时间听起来很多,但是如果你驾驶100个小时,那就不是很多了。恰恰是在这100个小时里,一块大石头滚到了街上。所以说,你需要达到接近渐近难度级别的质量水平,这是非常重要的。如果你有多个传感器,它们具有不同的优势和劣势,在需要时可以相互配合,你就可以做到这一点。这就是为什么你需要两者。你真的需要这两种。
It's a software doing that today currently, or are people just picking one system and going with it? Uh, a lot, no, no, they very much so work together. Like on a WaymoVieCool, or on a cruise vehicle or whatnot. They very much work together. So in particular scenarios, you'll pay more attention to what the camera tells you and others. You'll pay more attention to that. But the camera is the default now, right?
现在有一个软件在做这件事情,还是人们只是选择一个系统并加以实施?实际上,它们很多时候都是结合使用的,比如在WaymoVieCool或者自动驾驶车辆等场景中,它们会很好地协同工作。在某些特定情况下,你会更加关注摄像头的信息,而在其他情况下,你会更加关注其他设备。但是现在摄像头通常会作为默认设备,对吧?
Uh, I, that's not true. No, OK. I think, I think a lot of, a lot of these cars still drive very much influenced by the LiDAR. Really? Yeah. Ah, it's a really good sensor. If, if we had LiDARs on our phones, life would be great. Why? Uh, it's, uh, it gives you a lot of experience. Uh, it gives you, again, it gives you a very accurate 3D map of the world around you. So you can, you can basically do a lot more with your surroundings.
嗯,我觉得这不是真的。不是这样的。我认为很多汽车仍然受激光雷达的影响而行驶。真的吗?是的。嗯,它是一个非常好的传感器。如果我们的手机上也有激光雷达,生活会更美好。为什么?嗯,它可以给你很多体验。它可以为你提供非常准确的环境的三维地图。因此,你可以在你周围的环境中做更多的事情。
And that is, I thought Google was starting to put that kind of depth sensing in there. They're not doing it with LiDAR. They're doing that with some other sensor. Yeah, there's a, there's a structured light sensor on the front of your phone. Now most of these phones have, yeah, that does face ID or whatnot. Ah, structured light sensor. Yeah. And that can do depth. It knows the depth of my nose, my eyes, all that kind of stuff. So it knows it's me, not you. Exactly.
我认为谷歌开始在手机中加入深度感应功能了。他们并不是用 LiDAR 来实现这个,而是使用其他传感器。现在大多数手机的前面都有一个结构光传感器,可以用于进行面部识别等。这个结构光传感器可以进行深度感知,它知道我的鼻子、眼睛等深度信息,因此可以确保是我而不是其他人。
And that's why a flat photo doesn't work, because it would be pretty hard to fake. Uh, yeah, exactly, exactly. Well, though I heard Asian faces, the original versions didn't work for, or like, the people who looked similar who were Asian, when the white guys created the algorithm, had Google, or Apple, it didn't actually, Asian people could unlock each other's phones. I, I saw, I saw the articles. Is that true or not?
这就是为什么平面照片行不通的原因,因为很难伪造。嗯,是的,完全正确。虽然我听说亚洲面孔,在白人创建的算法中的原始版本无法使用,或者说,看起来类似的亚洲人,在谷歌或苹果使用时,实际上无法解锁对方的手机。我看过这些文章,这是真的吗?
The, uh, this is, this gets back to the core of the issue, which is machine learning is, uh, is really hard, because it's all about the data. So, uh, who knows what was going on in the underlying data that trained those algorithms? It was some white guys, camera roll. You said, here we go. Uh, well, either way, this is why it's really important, have really good data in algorithms, because otherwise they'll do weird things.
嗯,这个问题的核心在于机器学习非常困难,因为它完全依赖数据。因此谁又知道在那些算法的训练数据中究竟发生了什么?可能是一些白人男性的相册中的照片,你说这里怎么了。无论如何,这就是为什么在算法中有非常好的数据非常重要,否则它们会做出奇怪的事情。
But it would make sense, right? Like, as if the algorithms were built off of a database in China, let's say the flicker of China, and 99.99% of the photos were of Chinese descent, you would be optimizing for that data set. And if you did it in America, and whatever percent was white, and the percentage of Asian might be whatever, two, three, four percent, let's say, it's not going to be as refined.
这样做是有道理的,对吧?比如算法是基于中国的数据库构建的,比如中国的闪存,99.99%的照片都是中国人的,那么你就会针对这个数据集进行优化。如果你在美国这样做,而白人的比例可能是某个百分比,而亚洲人的比例可能是二三四个百分比,那么结果就不会那么精细。
That's exactly right. Yeah, this is why, I mean, this is why, when a lot of our customers, and a lot of, I think, companies doing machine learning today, think about it, it's really about how do we, how do we constantly improve with more and more data, that sort of fills in the gaps, and makes the whole system, holistically more robust over time.
这完全正确。是的,这就是为什么我们的许多客户和很多今天从事机器学习的公司在考虑的时候,真正要考虑的是如何通过更多的数据不断改进,填补空白,使整个系统随着时间的推移更加全面强大。
And you guys build which piece of this? The data storage, the algorithms, I'm still unclear, so which piece you build?
你们在这个项目中负责哪个部分?是数据存储还是算法?我还不是很清楚,你们具体负责哪个部分?
Yeah, so what we do is, uh, all this, so, all this data that comes in, let's say it's camera images, it's just images, it's simpler to think about. Talk about petabytes of data. Yeah, these petabytes of images come in, and, uh, pre-maphacia, you have no idea what's going on in these images. Right. Right. And so what you need is, you need to, uh, you need to figure out, where are the people, where are the cars, where are the, where are the stop signs, where are the cats, et cetera, and you need to figure out that out for every single one of these images, so that you can train a machine learning model on top of that.
嗯,我们所做的是,所有这些数据,比如摄像头图像,就是图像,更容易理解。我们谈论的是以拍字节为单位的数据。嗯,这些以拍字节为单位的图像被输入进来,而且在现有的情况下,你并不知道这些图像中发生了什么。对吧,对吧。所以你需要做的是找出人、汽车、停车标志、猫等的所在位置,并对每个图像进行分析以便能够在其上构建机器学习模型。
Got it. So what we do is, we build this pipeline, where, uh, most of the work is done by machines on our end. We have classical computer vision algorithms.
明白了。所以我们要做的就是建立一个流水线,在这个流水线中,大部分工作都由我们这边的机器完成。我们有经典的计算机视觉算法。
Uh, So it's like you do a first scrub of the data.
嗯,这就像你先对数据进行一次初步清洗。意思是先对数据进行一次基础处理。
Exactly. So somebody like Waymo could say, hey, here are, here's 10 million miles of driving. Have at it. You say, okay, here's what we think. These are all the minivans, these are all the pickup trucks, these are all the cats, these are the bouncing balls, et cetera.
没错。那么像Waymo这样的公司可以说,嘿,这里有1千万英里的驾驶数据,尽管拿去用。你可以这么说,好的,这是我们的分析:这些是所有的面包车,这些是所有的皮卡车,这些是所有的猫,这些是弹跳球等等。
Yep. And then we, we also do, we also have a, uh, a large team of smart, well trained humans who can basically go through and spot errors that these, that, that are made.
是的。此外,我们还拥有一支庞大的聪明、经过良好培训的人员团队,可以浏览并发现这些错误。
And then the, well, that's a second level of scrubbing, which is humans looking at things that computers have a low degree of certainty of.
然后,嗯,这是第二层洗涤,即人类查看计算机确认度较低的内容。
Yes. So if the computer is 99% certain, you just go with the computer. Uh, well, we have, we have more sophisticated filters than that, but more or less. Yeah.
是的。所以如果电脑确定度达到了99%,你就跟随电脑。嗯,我们有比那更为复杂的筛选器,但大致如此。是的。
And then, and then basically this, this highly accurate data, it goes back to the customer, and they feel great about it. They retrain their machine learning models. It, uh, it's a wonderful cycle. So they don't have to worry about building a team, or to do this basic level. It's almost like, you're just really good at getting that data set scrubbed and clean for them, and normalizing it in some way, so that they can work on the higher level stuff, like what to do with the minivan, or what to do with the minivan turned on that side. Oh, it rolled over, with something's going wrong here, right?
然后,基本上这个高度准确的数据会回馈给客户,他们对此感到非常满意。他们重新训练自己的机器学习模型。这是一个美妙的循环。因此,他们不必担心建立团队或处理基本层面的问题。这就像是你擅长清理、整理数据集,使其规范化,以便他们可以专注于更高层次的问题,比如如何处理一辆小型货车或者发生了意外情况,例如车翻了。
That's exactly right. And, and the way that we think about this in general, is we're really providing this sort of, this infrastructure layer for machine learning globally, or AI globally, where in AI in general, there's like, there's one big problem, which is getting all the data, and there's another big problem, which is like, how do you improve, how do you build these models, and how do you improve the models? Yeah.
这完全正确。而且,我们通常会这样考虑,我们真正为全球的机器学习或AI提供了这样的基础设施层,在AI中,有一个大问题,那就是获取所有数据,还有另一个大问题,那就是如何改进,如何构建这些模型以及如何提高模型的质量。
And we're trying to take the first problem off of people's plates. And we get back to this break, I want to know, when you believe, based on your seat, which is very close to the data, in fact, you're sitting on top of the data, you're soaking in it. I want to know when you, Alexander Wang, with no E, eight letter characters in that first name, I want to know when Alexander Wang thinks, we will not need a steering wheel on cars, in San Francisco, driving from Palo Alto, to San Francisco. When do you think that'll be legal, without a steering wheel, when we get back on the swing story.
我们正在努力解决人们的第一个问题,让他们少担心。回到这个话题,我想知道你在这个问题上的看法,因为你坐的位置非常接近数据,实际上你就坐在数据上。我想知道,没有“E”这个字母的Alexander Wang,当你认为我们在从帕洛阿尔托驾车到旧金山时,不需要方向盘时,你认为这将在什么时候合法化。我们在讨论这个话题时,请告诉我你的看法。
All right, listen, there's 600 million people on LinkedIn, including me, and you, and the person sitting next to you, and the three people you just email, and you have to hire people, but where are all those potential candidates? Well, they probably have a job right now, and so you got to get in front of them because they're passive job searchers. If they see something interesting, they might just click it.
好的,听我说,LinkedIn上有6亿人,包括你、我和坐在你旁边的人,还有你刚刚发邮件联系的三个人。你必须雇人,但是这些潜在候选人在哪里呢?他们很可能现在已经有一份工作,所以你必须进入他们的视野,因为他们是被动的求职者。如果他们看到有趣的职位,他们可能会直接点击。
Well, how do you do that? Well, here's how you do it. Watch my associate, he's going to go on LinkedIn Talent Solutions, and he's going to post a new job for our client's success manager in our Toronto office. He quickly selects the skills that are needed, writes a quick description, adds additional screening questions, which I love, and then he sets a daily budget that's, you know, reasonable, and he's on his way to finding the perfect candidate, whether they're looking for a job or not. LinkedIn is going to get you in front of hundreds of millions of job seekers who are not actively seeking a job.
那么,你该怎么做呢?好的,以下是如何操作。请看我同事的示范,他将前往LinkedIn Talent Solutions,为我们位于多伦多办公室的客户成功经理发布一份新职位。他迅速选择所需的技能,写下简短的描述,添加附加筛选问题,我非常喜欢,然后他设置了合理的每日预算,开始寻找完美的候选人,无论他们是否正在找工作。LinkedIn将让您接触到数亿正在不积极寻找工作的求职者。
So, with LinkedIn jobs, you can pay what you want, and the first $50 is on them. What? Yes, that's right. LinkedIn Talent Solutions is going to give a $5050 for you. Right now, all you have to do is go to LinkedIn.com slash twist, LinkedIn.com slash twist. I don't know how long the sooner or less work, but it's $50 for you right now, for free, from our friends at LinkedIn. And by the way, a hire is made every eight seconds at LinkedIn, and you know that that's true, because how many people do you know who found a job or a candidate on LinkedIn? It is the central repository of talent, and now with LinkedIn Talent Solutions, you can leverage that massive network.
因此,通过LinkedIn招聘,您可以自行决定支付多少费用,前50美元由他们支付。是的,没错。LinkedIn人才解决方案将为您提供5050美元。现在,您只需要访问LinkedIn.com slash twist,LinkedIn.com slash twist。我不知道这个优惠会持续多久,但您现在可以免费获得来自LinkedIn朋友的50美元。顺便说一下,在LinkedIn上每8秒就会有一次招聘,您知道这是真的,因为您认识多少人是在LinkedIn上找到工作或候选人的呢?它是人才的集中库,现在通过LinkedIn人才解决方案,您可以利用这个庞大的网络。
Okay, let's get back to this amazing episode. All right, Alexander Wang is here, scale.com, scale AI. You heard what they do, and how many people you got working on with, innit? I think now the team is about 150 folks, mostly here in San Francisco, Paira. Yeah, exactly.
好的,让我们回到这个惊人的事件。好的,亚历山大·王在这里,scale.com,scale AI。你听到了他们的业务以及他们有多少人员,对吧?我想现在团队大约有150人,主要在旧金山的Paira。是的,确切如此。
Hey, when I left our hero, that's you, Alexander. I was wondering, when do you think we'll have a self-driving car? In a major city like San Francisco driving, a major route, let's say, from Palo Alto onto the highway, off the highway, you know, into, you know, Cokari, the great Greek restaurant here. Have you been? I'm not. Cokari, you can write that down. Cokari is the best Greek restaurant. Some people can say the best restaurant in San Francisco. Get the octopus and the Sagonaki. So you get them there.
嘿,当我离开我们的主角,也就是你,亚历山大时,我在想,您认为我们什么时候能拥有自动驾驶汽车?在像旧金山这样的大城市,通过主要路线,比如从Palo Alto驶入高速公路,然后离开高速公路,到达像Cokari这样的伟大的希腊餐厅。你去过吗?如果没有,你可以把Cokari记下来,就是最好的希腊餐厅之一,有些人甚至说是旧金山最好的餐厅。在那里尝试章鱼和Sagonaki吧。
You leave your house in Palo Alto, you get out, you order the Sagonaki. What year would this be possible? 2030 and over or under 2030. When do you think we'd first see this? Ballpark. Would you pick under 2030 or above 2030? So this is over under. This is the million dollar question, which is when or. It's actually a trillion dollar question. A trillion dollar question. Let's be real. But we're at, like fundamentally, the technology is getting better and better every year.
当你离开你在Palo Alto的家,走出去并点一份Sagonaki时,这将在哪一年成为可能?在2030年及以后还是在2030年之前成为可能?你认为我们什么时候会首次看到这种技术?大概是什么时候?你会选2030年之前还是之后?所以这是一个上下限问题。这是一个百万美元的问题——实际上是一个万亿美元的问题。让我们保持现实一点。但从根本上讲,技术每年都在变得越来越好。
And how much better on a percentage? Do we double, triple? Well, it's hard based on what metric you're measuring, but the algorithms that perceive the environments, we're getting a lot better, like asymptotically better every year. And then the algorithms that figure out what the cars supposed to do, the planning, algorithms, etc. are also getting better. So it really is, it's sort of only a matter of time before we get to the point where this, these kinds of routes are possible and we'll live in a safer world. Got it.
在百分比上会提高多少呢?会翻倍还是三倍?这很难根据你所衡量的指标来衡量,但可以说我们每年都在以指数形式变得更好,尤其是感知环境的算法。然后规划、算法等决定汽车应该做什么的算法也越来越好。所以只是时间问题,我们最终可以实现这种类型的路线,生活在更安全的世界中。明白了。
So that seems to me that you're thinking less than 10 years from now, this will be happening with regulation and all that counted in. Yeah, I think, I don't think regulations necessarily going to be that tricky. Well, I think there is precedent for, like you think of when autopilot first came about. I think there's precedent for how to think about a lot of these things. You mean autopilot in the airplane sense or in the Elon sense? In the airplane sense, yeah. Got it. Sorry.
你的意思是,你认为不到10年后,这一切都会在法规和相关计算中发生。是的,我认为,我认为法规不一定会那么棘手。我认为有先例,就像当自动驾驶首次出现时一样。我认为有许多这些事情需要考虑。你是指飞机自动驾驶还是伊隆自动驾驶?我是指飞机自动驾驶,是的,明白了。抱歉。
So the FAA and whatever regulatory bodies were like, okay, we get it. Autopilot works better than a human. Yeah, exactly. It's pretty obvious. Yeah. Plenty of room up there to operate when you're up at 30,000 feet. A lot less room to operate when you're going through the tunnel and there's six people in the middle of the street, though. The challenges are a bit different, but I think there's precedent for how to think about these things.
因此,美国联邦航空局和其他监管机构都认识到自动驾驶比人类驾驶更加出色。这是非常显而易见的。当你在三万英尺高空时,有很多可操作的空间。但是,当你穿过隧道,街上有六个人时,可操作的空间就少了很多。虽然挑战有所不同,但我认为在思考这些问题时,我们还是有先例的。
I think the technology, once it gets good enough, will be clearly extremely good. And so I don't think it will be that big of a deal. Under, so you think 10 years may be less? Yeah, I'm excited for this software. I'm excited for this software in the future. Got it. Wow, look at that. You will make a commitment. I like it.
我认为一旦技术达到足够好的水平,它将变得极其优秀。所以我认为这并不是什么大问题。那么,您认为可能在10年内或更短的时间内实现吗?是的,我对这个软件很兴奋。我对未来的这个软件感到兴奋。知道了。哇,看看这个。你会作出承诺。我喜欢它。
Why is that? You want to be neutral because of all your partners, your customers, or all the driving. You don't want to speed on their behalf or something? Or you just don't like the idea of gambling? Well, I didn't put any money on this, but I'll put the Kokari lunch on it if you want. I have no problems with gambling. I'm a risk-risk-seeking guy. What do you like? You blackjack guy? You're a poker guy? What do you like? I play poker, yeah. Really? I would be a little bit above. I would be a little bit above. So you play a mix game? Yeah. I would love to play a regular game. Do you have a regular game? There are some games I play at in San Francisco. Yeah. Yeah.
为什么会这样呢?你想保持中立是因为你有很多合作伙伴、客户或者因为你是司机吗?你不想为他们冒险或者什么的吗?或者你只是不喜欢赌博的想法?好吧,我没有在这上面下赌注,但如果你愿意的话,我可以拿我的Kokari午餐与你打赌。我对赌博没有问题。我是一个喜欢冒险的人。你喜欢什么?你喜欢21点吗?还是你喜欢扑克牌?你喜欢哪一种?我玩扑克牌,是的。真的吗?那我就稍微高估你了。你玩混合游戏吗?是的。我很想玩一局正常的游戏。你有正常游戏吗?在旧金山有一些我经常玩的游戏。
You probably have bigger games in the game. Well, play the bigger game. I don't have to play the bigger games. You may watch a play. Yeah. One day. One day. Let's start. Post-ipo. Yeah. Well, I mean, there's always secondary shares, and you know, common shares play. That's where I always tell founders. Common shares play. I would settle up a 50K balance with some common shares. And scale. I'm not calm.
你可能在游戏中有更大的目标。那就去玩更大的游戏吧。我不必玩更大的游戏。你可以看一场演出。是啊。有一天。让我们开始吧。IPO后。是的。我是说,总有二级股份,你知道,普通股份玩法。这就是我经常告诉创始人的地方。普通股份玩法。我会用一些普通股份结清50K的余额。并扩大规模。我不平静。
So let me ask you about the race between China and America for this. China is really putting a lot of effort into this. Yeah. And do you have any customers in China currently? We don't have any customers in China. We work with some US arms of Chinese companies. God. I do, for example. God. I do. I do. I do. I do. I do. I do. I do.
那让我问你对于中美之间竞争这一点的看法。中国在这方面确实付出了很多努力。你们有当前在中国的客户吗?我们没有在中国的客户。我们与一些中国公司的美国分支合作。哦天啊,我知道其中一个。是的,就是我。
I don't have an employee of affairs in China because they seem to be doing a Manhattan project back to your parents. They're low-s, low-s, alamos. They're kinda doing a Manhattan project. Does that mean they're gonna get a lot further than us, you think? I think it's definitely true that China is innovating very much in machine learning and AI. And a lot of it is, it's very clear that it's very, it's a very concerted effort on the part on a lot of these large tech companies in China's part, like there's a lot of investment where I think if you were to look five years back, it would be kind of shocking at how much progress they've made today. So it's very, something is definitely happening.
我在中国没有雇用公事员,因为他们似乎正在进行一项回到你们父母的曼哈顿计划,他们很低调,阿拉莫斯,他们有点像进行曼哈顿计划。你认为这意味着他们会比我们取得更大的进展吗?我认为中国在机器学习和人工智能方面正在进行很大的创新。很多技术公司在这方面都在进行着非常有计划的投资,这一点非常明显,如果回顾五年前的情况,你可能会感到震惊,看到他们今天取得的进展。所以,肯定有一些事情正在发生。
And I think to be clear, I think machine learning, a lot of the machine learning is definitely very good in the US and for most things is much better, but there are certainly making a lot of progress. And there's a lot of data sets that they have access to that we don't necessarily have access to. And so like CCTV cameras, they have cameras everywhere. They can find somebody already on facial recognition. It was a 60 minutes piece. I guess they found people in like less than five minutes. Yeah. Pretty scary. Yeah.
我认为要说清楚,美国的机器学习很好,在大多数情况下要好得多,但它们正在取得很多进展。他们可以访问许多我们无法访问的数据集,比如电视监控摄像头,他们的摄像头无处不在。他们可以通过面部识别找到某个人。这是一个60分钟的节目。我想他们找到人的时间不到5分钟。非常可怕。
Yeah, it's very, oh, look at that. Sian. Sian, yeah. Oh, Kai Fully, I know Kai Fully. He likes to play Parker too. Yeah. He does actually. But yeah, it's basically, it's very, there's a lot of concerted effort. There's a lot less, there's a lot fewer questions as this technology gets productionized in China versus in the US.
是的,哦,看那个。厌倦。厌倦,没错。哦,Kai Fully,我认识Kai Fully。他也喜欢玩Parker。是的。事实上,随着这项技术在中国得到推广,与在美国相比,这里有更多协调的努力,也有更少的问题。
I think we're, you mean moral, ethical, regulatory issues? They're going to just go faster there. As Sian, the angel investor points out on Twitter, Kai Fully said that China would get further because they don't have the same issues around human casualties. That's interesting. Yeah. And they're more, they're more accepting of risks, et cetera. And I think, I mean, I do think move fast, break things, lets you move faster. Right. So I think there is, there is a real tension there. Yeah. And in the two approaches.
我认为你的意思是道德、伦理、监管等问题吧?在那方面,他们会更快。正如投资天使Sian在推特上指出的,Kai Fully表示中国会更进一步,因为他们没有关于人员伤亡的同样问题。这很有趣。是的,他们更接受风险等。我认为,“快速行动,打破桎梏”可以让你走得更快。所以我认为这里存在真正的紧张关系,以及两种方法之间的差异。
I've been pretty excited that in America, we haven't had a panic over self-driving. So in the horrible Uber accident in Arizona happened where the driver, the safety driver, wasn't paying attention. And I believe they were playing Candy Crush or on their SMS. Did you see the actual video of them in the, they were, yeah, they were, I, they were on their phone. Yeah, they were looking down at a video. Yes. They knew they were on camera. And they were told they're being videotaped the whole time. They still couldn't keep their eyes on the road. And they killed the poor homeless person who was walking across the street in the middle of a dark road. I, yeah, I believe it was, it was a man with a bike, but yeah. Oh, it was a man with a bike. Yeah. And why somebody said it was a homeless person at some point, but they, it's interesting that somebody said that it was almost like they're saying in the person by the nature of being homeless, they were doing something bad. But they were doing something that they were crossing like an eight lane highway in the middle of the road where they were absolutely no business being, which is what the algorithms job is.
我一直很高兴地看到在美国,我们对无人驾驶没有恐慌。然后,在亚利桑那州发生了可怕的Uber事故,那名安全驾驶员没有注意到情况。我相信他们正在玩糖果传奇或使用短信。你看过他们在实际视频中的表现吗?是的,他们在低头看手机,即使知道自己被摄像机记录下来了,他们仍然无法关注路面,最终导致一名可怜的无家可归者在黑暗中穿越路中被撞身亡。我相信这是一名骑自行车的男子。有人说是一名无家可归者,但有意思的是,这似乎是暗示着这个人因为无家可归而做了什么坏事。但其实这名男子正在穿越一条八车道的公路,完全不该去那里,这是算法应该照顾到的事情。
So how does an algorithm take that into account? A, who is blatantly going against all conception of what is normal, like walking across a six or eight lane boulevard avenue freeway? Well, I think in this case, this is actually a great example of two things. First where light are is great. And then second where, didn't they have light on that or no? So the thing is the, the model is actually detected that person person.
那么算法如何考虑这个问题呢?A正在公然违反一切正常概念,比如穿过一个六或者八车道的林荫大道高速公路?好的,我认为在这种情况下,这实际上是两个方面的很好的例子。首先,灯光位置的确很重要。然后其次,他们是否在那里有灯光?所以问题在于,模型实际上已经检测到了那个人。
So in this case, the sort of machine learning and the light are, by the way, the light are also detected the person who sort of it was doing its job. It was more of the sort of like higher level processing that sort of that made the unfortunate decision to not break. Yeah. And to break at whatever 45 or 60 miles an hour on one of those freeways, that comes with consequences too, because there are people behind you. Yes, definitely. How is the computer supposed to make that decision?
在这种情况下,机器学习和光线被检测到了正在执行工作的那个人,而更多的是那种较高级别的处理,导致不幸的决定不打破。是的,以45或60英里每小时的速度在高速公路上刹车也会带来后果,因为有人在你后面。计算机应该如何做出这个决定呢?
Let's put aside this issue where obviously the decision tree didn't make the right decision on the data, which was clearly presented. So it wasn't a sensor issue. It wasn't a processing of the data issue. It was a decision issue. It decided not to slam on the brakes. Is that right? That, I believe, if you read the N, the Nitzer report, that is what's happened. Yeah.
让我们把这个问题放在一边,显然决策树没有根据明确呈现的数据做出正确的决定。因此,这不是传感器问题,也不是数据处理问题,而是决策问题。它决定不刹车。没错吧?我相信,如果你读过尼策尔报告,就会知道这就是发生的事情。是的。
That's a developer who wrote code didn't write the code properly. Well, it's a complex code system that ultimately made the decision it did.
这是一个开发人员编写的代码存在问题。这是一个复杂的代码系统,最终做出了它所做的决定。
Do we even know what's going on with this machine learning? Because my understanding is a lot of times we put a bunch of data in. The answer comes out and you ask the person who set the whole system up. They don't know how the answer was come to. Just that it came to the answer.
我们是否确实了解这个机器学习正在做什么?因为我的理解是有很多时候我们把大量数据输入进去,答案就出来了,然后我们问整个系统的创建者,他们并不知道这个答案是怎么得出的,只知道答案是出来了。
Well, I do think, so you bring up an interesting topic, which is about explainability. How do you actually know what these algorithms are doing? And this, again, I don't know something like a broken record, but it does come down to the data. And when you actually dig in, usually when the algorithm makes a weird decision, usually you can trace that back to something weird in the data that it was.
我认为你提出了一个有趣的话题,就是可解释性。你怎样知道这些算法在做什么?而这,同样我就像唱 broken record 一样,又归结于数据。当你深入挖掘时,通常当算法做出奇怪的决定时,你可以追溯到它所处理的数据中有一些奇怪的地方。
Do you have any examples of that without mentioning specific customers, but in your testing less A or in your laboratory, or even in the real world without mentioning who, what, when, where? Do you have an example of the data was confusing and the output was then, you know?
你能给出任何不提及具体客户的例子吗?比如在你们的测试中减少了A,或者在实验室或现实世界中出现的情况,但不涉及到具体的人、事、时间和地点?你是否有数据混乱而输出结果不明确的例子呢?
Yeah, I think. Cool. Do you bear the responsibility of that? We don't. We do bear a quality responsibility with our customers. In fact, we sign up for the quality of data we get for our customers. They have the ability to look through all the data, look like audit, et cetera. It's kind of heavy, isn't it? It's a lot of responsibility.
是啊,我想是的。很酷。你负责这个吗?我们不负责。我们与客户承担质量责任。实际上,我们为客户获得的数据质量签约。他们有能力查看所有数据,进行审计等。这是一项相当重要的责任,是非常沉重的。
Well, it's one, it's why I think, I mean, ultimately, if you think about it, if you really believe in machine learning, a lot of, a lot of the things that you need to do machine learning, there needs to be stable infrastructure just like running water, right? So if you think about, for example, AWS has a pretty tough job.
嗯,我觉得这是一个原因。我是说,如果你真正相信机器学习,很多需要进行机器学习的事情,需要像自来水一样稳定的基础设施才能实现。例如,如果你想想,AWS有一个非常艰巨的任务。
They have to, like, they have to say that all these machines that they have up and running are going to be up 99.9 or 99.9. Yeah, yeah, like a crazy high percentage of the time and that they, like, all your quarries will take less than X amount of time, et cetera.
他们必须表示,所有这些正在运行的机器都将达到99.9%或99.9%的正常运行时间,而且他们的所有采石场将在X时间内完成等等。
But that infrastructure lets all these people build on top of it and build these machines, et cetera. So I do think as a general rule, as an infrastructure provider, you need to give infrastructure that's very reliable that people can depend on.
这个基础设施让所有这些人都能在其之上构建并构建这些机器等等。因此,作为基础设施提供者,我认为你需要提供非常可靠的基础设施,以供人们依赖。
And you know, it's really interesting. They do such a good job, AWS, that when AWS does go down, which seems to be like some portion of it goes down, you know, Northeast, whatever, it's sometimes as regional or some section of it goes down, it's almost like people have a funny joking, like it's a snow day response to it for three or four hours.
你知道,这真的很有趣。AWS做得非常好,以至于当AWS宕机时,似乎部分服务会中断,例如在东北部某个区域中断,人们有时会像对待雪天一样开玩笑,对此有一个有趣的反应,持续三到四个小时。
As opposed to five or 10 years ago, when this would happen, people would get really bent out of shape. They would go down for two days or a day or I don't know what the longest Twitter outage was, but it would be for hours. Like, it could be a half a day of the farewell. And then before that, it was like reason to like not trust the internet. So we went from like not trusting it to being extremely frustrated. To now it's kind of like a joke like, oh, it's down. We know it's coming back. Yeah, no big deal. They're going to reboot the servers and figure it out.
与五到十年前不同,当这种事情发生时,人们会非常生气。他们会将整个一两天的时间花在上面,或者是不知道Twitter最长的故障时间是多长,但那个时间会持续数小时,甚至半天。在此之前,我们对互联网的信任从不可信到极度沮丧。现在,我们已经把这个问题当作一个笑话,说它停了,我们知道它会回来,没什么大不了的。他们会重新启动服务器并解决问题。
We've really gotten used to it because it's, it's such a rarity. Yeah. Something is going to happen to machine learning and AI over time.
我们已经习惯了这种情况,因为这种情况非常罕见。是的,随着时间的推移,机器学习和人工智能将会发生一些变化。
Like right now, you framed it very well. Right now we're in this period of high distrust of these systems. We see them do weird things and we don't really know what's going on and we feel like it just feels foreign and weird.
像现在这样,你表达的非常好。现在我们正处于对这些系统高度不信任的时期。我们看到它们做一些奇怪的事情,我们并不真正知道发生了什么,感觉就是很陌生和奇怪。
And then eventually everybody will learn more about the technology, learn more about what it's good and bad at. We'll get to a point where when it does something unexpected or doesn't think bad, we'll just get really annoyed. Yeah, very frustrated because it really impacts people's lives or it impacts people's businesses, etc. And then in the long term, I think the systems will be extremely reliable and certainly much more reliable than any human could ever be.
最终,每个人都将更多地了解这项技术,了解它的优点和缺点。当它所做的事情出乎意料或出现问题时,我们会感到非常生气和沮丧,因为它会影响人们的生活或企业等。但长远来看,我认为这些系统将极其可靠,肯定比任何人类更为可靠。
Yeah, I mean, like we work, we interact with lots of machine learning systems already. Google search is a large federated machine learning system today. It's very, very influenced by core deep learning, machine learning, etc. And it is extremely reliable. It works like running water. It's great.
是的,我的意思是,我们在工作中已经与许多机器学习系统进行交互了。谷歌搜索是一个大型联合机器学习系统,现在已经被核心深度学习、机器学习等方面所影响。它非常可靠,就像流动的水一样顺畅。它很棒。
Yeah, when's the last time you're like, I couldn't find that answer. Between Kora, YouTube videos and Google acting as this glue and fabric between it, like surfacing stuff in the one box, you know, little box that gives you like a mini scraped answer.
嗯,你上次找不到答案是什么时候?通过 Kora、YouTube 视频和谷歌作为连接和纽带,将信息呈现在一个小盒子里,你知道的,这个小盒子会提供一个超级简要的答案。
But they're not allowed to scrape Kora. Kora doesn't let them index it, right?
但是他们不能爬取科拉网站。科拉不允许他们索引它,对吧?
I think they're still in the standoff. I actually, I don't know. Sometimes I see Kora. Well, maybe this is old.
我认为他们仍在僵局中。实际上,我不知道。有时我看到Kora。嗯,也许这是旧事了。
I think you see the link, but they won't let them put it in the one box where they kind of expose the answer. They kind of make you click through and they make you log in. This is why Kora is kind of brilliant. Kora. They won't let Google take their data. Kora. It's too valuable. It is a very brilliant company.
我认为你看到了这个链接,但他们不允许将它放在那个暴露答案的单一框中。他们让你点击并登录。这就是为什么 Kora 看起来很聪明。Kora。他们不允许 Google 获取他们的数据。Kora。这个公司太有价值了。它是一个非常聪明的公司。
DeAngelo? Adam DeAngelo. Adam DeAngelo. You know, I could get him on the pod. Let's make a note. It's been like five years I've been trying to get him. He don't like to talk. He's an introvert, I think. He is, well, he's on Twitter. So Twitter.
DeAngelo是谁?Adam DeAngelo。嗯,我可以请他上我的播客节目。我们来记下来。我已经试了五年了,他不太喜欢说话,我想他是一个内向的人。不过他在推特上。所以我们可以通过推特联系他。
Yeah. Twitter Adam. What was your like to work with? He was great. He's very, very smart, very thoughtful, very long-term focused. I think he put 30 million of his own Facebook money into it, right? Well, he was the first CTO of Facebook. So I think he put 30 million, this is what I heard. Put 30 million of his own money in like the Series B or something and then like let somebody else put in like 10 or 20. Like talk about skin in the game. Yeah, I have no idea. I was bonkers. I was like, wow, that's a first.
是啊,亚当在推特工作是怎么样的呢?他很棒。他非常聪明,思考周到,长期眼光很强。我听说他投了3000万美元的Facebook钱进去,对吧?因为他曾经是Facebook的首席技术官。所以我想他在B轮融资时投了3000万美元,然后让别人投了10或20万美元。这算是非常有利益相关性的投资吧。是啊,我真的不知道该怎么反应了。我当时就想,哇,这还头一次看到这样的。
And but he's thinking 20, 30 years out. Like this is his last, first and last startup. I think, yeah, he thinks extremely long-term, which I think means that it's a competitive advantage in today's ecosystem where most, most people in general are very short-sighted.
但他在考虑20、30年后的事情。就像这将是他的第一个,也是最后一次创业。我认为,他非常注重长期规划,这意味着在当今生态系统中具有竞争优势,因为大多数人都非常短视。
I think they'll look at, oh, that where's the quick way in or where's like the, the new hot thing. Right, they don't have any revenue, right? They don't, they don't have ads. They do have ads actually. They put ads on? Oh, I didn't know there's ads on there now. They're so subtle that you can't even notice them. Whoa, I'm going to go check those out.
我认为他们会看到这个,哦,那里有快速入口或者是新的热门东西。不过,他们没有收入,对吧?他们没有广告。实际上,他们确实有广告。他们放广告?哦,我不知道现在有广告了。它们非常微妙,你甚至看不出来。哇,我要去检查一下。
Does it matter that we don't know how sometimes ML gives an answer when we go look at the algorithm? Why did this come up first? We say, well, there's a number of factors, but we don't actually know. Does it actually matter? I think non-explainable systems are already out in the wild and-
我们不知道机器学习有时候给出答案的算法,是否真的很重要呢?为什么这个问题首先出现了?我们说,嗯,有很多因素,但实际上我们并不知道。这真的很重要吗?我认为那些不可解释的系统已经到处出现了。
What's an example one in the wild? Well, Google, like before there's, yeah, before there's machine learning Google or your Facebook feed or your- Okay, so Facebook can't explain why a specific post went to the top. Well, they have, they have some information, right? Right. And they can give you a little bit, they can give you some information. But most, like, this is a problem with code more than anything. Post code systems are so big and difficult to explain that it's already a big problem.
在实际应用中,什么是一个例子呢?比如谷歌,在应用机器学习之前,或者你在 Facebook 上的信息流。但是,Facebook 不能解释为什么某个帖子会排在顶部。他们可能提供一些信息,但是这主要是编程问题,帖子系统非常庞大而难以解释,这已经是一个大问题了。
So the cost of doing a Google search and getting a non-perfect answer, or it makes- Let's say it makes a huge mistake, is very low, right? You just do the search again or you change the search a little better, you pick the second answer. No problem. If Facebook puts something at the top of your feed that's not the most relevant and the second and third one are the most relevant, again, no problem.
那么进行谷歌搜索并得到非完美答案的代价,或出现巨大错误的可能性非常低,对吧?你只需重新搜索或稍微改变搜索词来选择第二个答案,这没有问题。如果Facebook在你的动态中放置了不太相关的内容,并且第二和第三个答案更相关,同样也没有问题。
However, if you do it with a self-driving car and it makes a decision, it could be somebody's life. And if you do it with some kind of system involved in justice, like talking about using ML for justice, should a computer be able to give an answer that somebody's guilty without being able to explain it?
然而,如果您使用自动驾驶汽车并做出决定,可能会牵涉到某个人的生命。如果您使用某种司法系统,例如使用机器学习进行司法,那么计算机是否能够在无法解释的情况下给出某个人有罪的答案?
So when we get back from this break, I want to know, would you trust in the next 10 years ML and AI to make decisions? Obviously, our fine with making decisions about driving and people's life and death there, would you make it work for the justice system versus the justice system in America, which has been proven to be biased against non-white people when we get back on this week and start.
在我们回来休息期后,我想问问你,在未来的十年里,你是否相信机器学习和人工智能会做出正确的决策?显然,我们对于关于驾驶和人们生死的决策已经感到满意了,你是否认为在司法系统中也应使用 AI 技术,以此来替代美国司法系统已经被证明有对非白人的偏见呢?当我们回到这个星期开始的时候,让我们来探讨一下这个话题。
Listen, you're running a small business, you're running a startup, you need money and it shouldn't take all your time to get money to run your business. The modern way to do that, the simplest way to do that is cabbage. They allow you to access up to $250,000 in credit to run your business.
听着,你经营着一家小企业,或是一家创业公司,你需要资金,但它不应占用你所有的时间去筹集。现代的方式、最简单的方式是去 cabbage。他们可以让你获得高达 25 万美元的信用额度,用于经营你的业务。
Cabbage's application process is online and it takes just minutes to complete and get a decision. If you qualify, you can access the amount you need right away and withdraw more funds whenever you need extra capital. Cabbage has an A plus rating with the Better Business Bureau and has already provided over 200,000 small businesses with access to funding.
Cabbage的申请过程是在线的,只需几分钟就可以完成并得到决定。如果你符合资格,就可以立即获得所需金额,并在需要额外资本时提取更多资金。Cabbage在Better Business Bureau中获得了A+的评级,已经为超过20万家小企业提供了资金。
Portfolio companies have used it to cover employees over the holidays when a large client missed an invoice. That was an amazing story I heard. So I want you to get the money you need to run your small business. Today, go to Cabbage.com and use the code, twist, to get a $100 credit on your first loan statement. This is KABB, AGE. KABB, AGE.com and use the promo code, twist.
投资组合公司已经使用这个工具来为员工提供假日保险,以应对大客户未能支付发票的情况。我曾听到过这样一个令人惊叹的故事。因此,我希望你们能够获得你们经营小企业所需的资金。今天,请访问 Cabbage.com 并使用代码 "twist",以获得你的第一笔贷款中的 $100 抵用金。这是 KABB,AGE.com,记得使用促销代码 "twist"。
This is an important disclaimer. You must take a minimum $5,000 loan to qualify. Credit lines are subject to review and change and this offer ends November 30th. Two days after my birthday, individual requests for capital are separate installment loans issued by Celtic Bank member FDIC.
这是一个重要的免责声明。您必须取得最低为$5,000的贷款才能符合资格。信用额度会受到审查和变更,此优惠截止于11月30日。个人资本请求在我的生日两天后,是由成员FDIC的凯尔特银行发放的单独分期贷款。
Alright, let's get back to this amazing episode. Alright, Alexander, we're here coming around the third ad break which means I'm going to ask you like the really hard questions. Like, you warmed up, you're comfortable. Maybe let your guard down a little bit. PR guys like checking a slack room. He don't care anymore. He's out of the woods. So let's get into the tough work.
好的,让我们回到这个惊人的剧集。好的,亚历山大,我们现在进入了第三次广告休息,这意味着我要问你一些非常困难的问题。像你已经热身了,你很舒服。也许可以稍微放松警惕了。公关人员喜欢检查slack聊天室,他不再关心。所以让我们开始进行艰难的工作吧。
I'm joking. This is a PR person in the room was probably freaked out by this question but are not. I explained that the list of your Twitter and Facebook feeds or Google and that we would all agree that doesn't matter.
We could argue Facebook maybe matters if it's pushing up stuff that is fake news and they're getting some heat about that but again nobody's dying hopefully.
我是在开玩笑。房间里的公关人员可能会对这个问题感到吃惊,但他们没有。我解释说,你的Twitter和Facebook动态列表或者Google列表无关紧要,我们都是一致的。如果Facebook推送的内容是假新闻,并因此受到一些压力,我们可以争论可能会有所不同,但希望没有人会死于此。
But now we can self-driving cars. Should you have to prove how these things made a decision as opposed to having this non-ability to understand how the decision was made or does it matter if it gets the right answer 10 times better than a human in your mind. So in the situation where the car, where you're self-driving software or one of your customers is 10 times better than a human. It's proven. Should they be able to explain the one in 10 chance they have of an accident compared to a human should explain ability be required. Yes or no?
现在我们有了自动驾驶汽车。在您看来,您是否需要证明这些车是如何做出决策的,而不是无法理解决策的方式,或者说如果这些车比人类的表现要好10倍,是否会更重要呢?如果你的自动驾驶软件或客户在某种情况下比人类表现好10倍,它已被证明过。他们是否应该能够解释他们发生事故的10分之1的概率比人类更高,是否需要证明他们的能力。是还是不是?
It's a, this is a really important question again. That's why I'm asking you, the founder of scale.com was raised 100 million to empower all this. This is actually right. I think as more and more systems are governed by machine learning, it's very natural to ask like, okay, if we're trusting our lives to these systems. We are. How are we supposed to feel good about that? Am I supposed to just live with the one in whatever chance that one of these systems will just poop out and then my life will be at risk.
这是一个非常重要的问题。这就是为什么我问你,scale.com的创始人筹集了一亿美元来实现这一目标。我认为随着越来越多的系统由机器学习控制,很自然地会问,如果我们要信任这些系统,而我们的生活又依赖于这些系统,我们应该怎样才能感到安心呢?我是不是只能盼着其中一个系统万一出了问题,我的生命就面临危险呢?
So I do think that ultimately further explainability and a deep understanding of the performance of these machine learning systems is going to be needed. Now that being said, like again, there are plenty of mission critical software systems in today's world that we depend on like for example, whatever systems that we use to control the power grid or whatever system we use to control the national missile system, etc. Those are all software systems that sometimes they will just crap out.
我认为,最终需要进一步解释和深入理解这些机器学习系统的表现。话虽如此,在当今世界上有许多我们依赖的关键软件系统,比如我们用来控制电网或国家导弹系统的任何系统,它们有时会出现故障。
Like every once in a while, your database will go down or every once in a while since it will go down. And whether or not you can explain those phenomenon, that tail probability that that happens still causes real risk. So in a way, you're saying you're being held to a higher standard. Why do you think you're being held to a higher standard in the systems that came before?
就像每隔一段时间,你的数据库会崩溃,或者因为某些原因会崩溃。而无论你能否解释这些现象,这种尾部概率的发生仍然会带来实际的风险。因此,在某种程度上,你正在说你要接受更高的标准。你认为为什么以前的系统要对你要求更高呢?
Well, what, yeah. I have a serious, but I'm interested in your question. What I'm saying is there is that we live in a world where randomness is a reality, right? And so accepted. So there's as these systems end up launching and end up being more and more important, I think it's important that we realize that there are always tail probabilities that bad stuff happens.
嗯,你问的问题很有趣,我很认真地回答。我想说的是我们生活在一个随机性是现实而又被接受的世界里。所以,随着这些系统越来越重要,我们必须认识到总会有一些极小的概率,会出现不好的事情。
Now that being said, I do think it is the responsibility of people who operate these systems is responsibility of the people who make these systems to have an understanding of the performance of these systems and also ensure that they are doing everything they can to make sure that these systems are performing as well as they can, which ultimately comes down to, okay, if I'm trading this model and I'm trading on so and so data set, how do I make sure that the data is unbiased? For example, we talked about the facial recognition cases. How do I make sure that there's, it is properly representative? How do I make sure that there's no weird artifacts in the data that would cause something bad in the model?
话虽如此,我认为那些运营这些系统的人以及设计这些系统的人有责任了解这些系统的性能,并确保他们尽一切努力确保这些系统的表现尽可能好。这最终归结到,如果我正在交易这个模型并且正在使用某个数据集进行交易,我应该如何确保数据没有偏见?例如,我们谈到了人脸识别案例。我应该如何确保数据被正确表示?我应该如何确保数据中没有奇怪的图像,可能会导致模型出现问题?
Yeah. And then how do I, like how do I trace back these issues, right? Yeah. And I think, I think very much so we're, we're, the whole machine learning community is understanding these issues and building them in, but it's, it's more about how do you build these systems that are, that are robust built on, on large data sets that are, that are very diverse. And more diverse, the data set, the less biased there should be in it. Exactly.
对的。那么我要怎样才能追溯这些问题呢?我觉得整个机器学习社区正在了解这些问题并建立相关系统,但更重要的是,我们该如何构建具有强大韧性、建立在大型、多样化数据集上的系统。数据集越多样化,其中偏见就应该越少。确切地说。
Except if you said, okay, we got a couple of states. Let's make a, a justice system based on it. And then all of a sudden you're like, okay, let's do the whole United States and then you find out, gosh, the whole United States justice system is biased. And if we were to build on that data set, people with African-American names, Latino names would be convicted more often because we actually used the data set that had bias in it.
除非你说,“好吧,我们有一些州。让我们在其基础上建立一个司法系统。”但后来你突然想,“好吧,让我们建立整个美国的司法系统。”然后你发现,天哪,整个美国的司法系统存在偏见。如果我们基于那个数据集建立司法系统,那些有非洲裔美国人和拉丁裔美国人名字的人就更容易被判定有罪,因为我们实际上使用了有偏见的数据集。
Yes. But the people, what you're saying is the people who are in this are acutely aware of it. And they are good actors who want to get it right. Or why else would they choose this as their profession? They would, there's nobody on your team or any ML team you've ever met who said, you know what? Let's put systematic bias into the system as opposed to getting the right answer. Getting biased into the system would mean your business is going to go out of business because you made a poor system.
是的。但是,你所说的人们非常清楚这一点。他们是优秀的演员,渴望做到最好。否则,为什么他们选择这个职业呢?在你们团队或任何机器学习团队中,没有人会说,“你知道吗?让我们将系统性偏见纳入系统,而不是得到正确的答案。”让偏见进入系统意味着你的企业将会因为你的不良系统而破产。
So in fact, machine learning and the people working in AI are so acutely aware of this, their intentionality would be to remove bias and make the world better. So it's, again, this technological phobia, technophobia. And we're holding technologists and machine learning to a higher standard. I think in part because we as humans are so scared of being replaced that we're going to hold that which replaces us to a standard that is one that we would never hold to human too.
事实上,机器学习和从事人工智能的人非常清楚这一点,他们的意图是消除偏见,让世界变得更好。所以,这又是一种技术恐惧症,技术恐惧。我们对技术专家和机器学习持有更高的标准,部分原因是因为作为人类,我们非常害怕被替代,因此我们会要求取代我们的技术达到一种我们从不会对人类要求的标准。
Yeah. And by the way, I think this is a, this is my opinion. This is a plate. There's this played out narrative, right? That AI is this magical thing that will come in and just replace humans at all of these very important tasks, right? And, and that's, I think that's, that's the dominant belief that a lot of people hold. But, but the reality in the actual nuts and bolts scenarios, it's, it's pretty far from that, right? We have a long way to go before, before machine learning will fully replace any kind of you, any jobs, right?
我的观点是,这是一种陈词滥调。有人认为,人工智能是一种神奇的东西,可以取代人类在很多重要任务上的工作。但是,实际情况比这要复杂得多。在不同领域,我们还有很长的路要走,才能看到机器学习完全取代人类的工作。
Yeah. And, and there is precedent for this, by the way, like when the, when the ATMs were originally, originally invented and built and, and launched, you would sort of believe, one belief you might have is that the number, as the number, as these ATMs were built, the number of bank teller jobs would, would start dropping pretty considerably. Yeah. But what actually happened is that the, the number of bank tellers in the United States actually grew pretty considerably.
是的。而且,实际上这已经有先例了,例如当自动取款机最初被发明、建造和启动时,有一种信仰是随着这些自动取款机的建造,银行职员的数量会相应大幅下降。但实际情况是,在美国,银行职员的数量实际上增长得相当可观。
And there's a number of like, sort of economic reasons you could think for what it's been. The unbank started to bank. Yeah, so, so one is that, that the, the ATMs allowed for this huge growth in the banking industry, which means that, that there's a lot more opportunity. Another is that the, you know, all those people who wouldn't get a bank account because they didn't have access to their money, kept it on their couch, were like, oh, I can get money any time. Okay, my number one fear is gone. Exactly. I will put my money in the bank, because I don't have to go there between 8 a.m. and 3 p.m.
有许多经济原因导致这个变化。不需要银行的人开始使用银行。一方面,自动取款机的普及让银行业得到了巨大的发展机遇。另一方面,许多人由于无法随时使用自己的钱而没有银行账户,他们只能将现金储存在自己的沙发上。但随着ATM的普及,他们不再有这个问题了,于是他们开始愿意把钱存入银行。他们不必再在8点到3点这段时间内前往银行取钱,所以他们将自己的钱存入银行中,这样他们的第一大担忧便消失了。
Yeah. So, that's one. Another is that the bank tellers now can focus on higher value tasks. For example, I don't know, like, like, mortgages, lines of credit, starting bank accounts, etc. Yeah, credit cards, right. Which, which means that the, the, the per, the, the value of a bank teller goes up, which means it's more valuable to invest in more bank tellers. And so, and so these, these effects are like, the second and third order effects usually mean that there's, there's way more opportunity and way more growth as these, as these sort of like, the automation slowly seeps in.
是的,这就是其中一个。另一个是银行出纳员现在可以专注于更高价值的任务。例如,我不知道,比如抵押贷款、信用额度、开设银行账户等。对,信用卡,对吧。这意味着一个银行出纳员的价值增加了,这意味着更值得投资更多的银行出纳员。因此,这些影响通常是第二和第三序的影响,这意味着随着这些自动化逐渐渗入,机会和增长会更多。
Yeah. And I mean, you think about it. We spent all this time creating these phone routing systems. Remember that where you're like, press one to go here, press two to go here. And we did that for what? 30 years. I just sent it to the phone jail system, they used to call a voice jail instead of voice mail. And we invested for 30 years in voice mail systems. Then at some point we realized, wait a second, everybody gets to people over messaging, whatever. Somebody does pick up the phone call. They probably have a very acute, important problem. And that's a chance for us to prove how great our brand is.
是的。我是说,你想一想。我们花了这么长时间开发这些电话路由系统。还记得那个,比如说按1去这里,按2去那里的吗?而我们这么做是为了什么呢?30年来,我们一直在投资语音邮件系统,曾经把它们称作语音监狱系统。然后,在某个时候,我们意识到,等等,每个人都通过信息传达,而不是打电话。如果有人接听电话,那可能意味着他们有一个非常紧急、重要的问题。这是我们证明自己品牌的伟大机会。
And to get to know our customer better. And then beat them with our other customers. Let's bring back people who pick up the phone and talk to you. It's a delightful VIP customer type experience. And now you have people adding back. And they just call it customer success. And people look at customer success, not as a cost center anymore. It used to be a cost center. How do we reduce the number of calls? Now people look at customer success as like an investment in them renewing.
为了更好地了解我们的客户,我们希望能够与我们的其他客户竞争。让我们重新回到那些亲自接听电话和与你交谈的人。这是一种令人愉悦的VIP客户型体验。现在你已经有了回报。他们只是称之为客户成功。人们现在不再把客户成功看作成本中心。以前它是个成本中心。我们该如何减少电话数量?现在人们把客户成功看作是对他们续费的一种投资。
So the SaaS people are like, yeah, if we get people to call in, when they have a problem, maybe they won't turn. Maybe they used a product more. Maybe we'll up some. So sometimes we bring the jobs back. We've got rid of phone operators and receptionists. And now we're bringing them back. We just call them customer success.
SaaS人说,如果我们让有问题的人打电话过来,或许他们就不会放弃我们的产品了,会更多地使用我们的产品,或许我们的业务会增长。所以有时我们会把工作岗位重新引入,虽然我们曾经取消了电话操作员和前台接待人员,但现在我们重新引入了这些岗位,只是给它们起了个新名字——客户成功。
Yeah, exactly. These trends are happening over and over. I mean, I think there's like helping people focus on higher and higher value work is really, I mean, that's sort of like the core of human progress in some sense. But that very much so is, I strongly believe will be the actual story of AI machine learning. And it'll have to happen more and more and more for us to be comfortable with it.
是的,完全正确。这些趋势一遍又一遍地发生。我的意思是,我认为帮助人们关注更高价值的工作,这在某种程度上是人类进步的核心。但我非常相信,这将成为AI机器学习的真正故事,并且我们需要越来越多地接受它才会感到舒适。
But a great example is truck driving. So there's a lot, all these automated truck driving companies. Yeah, lots. We work with a lot of them in Bark, Ike, etc. And there's sort of the naive view is that, hey, they're just, they're going to automate truck drivers. And like, if you look at the map of the states, truck driving is a top profession in a lot of states. It seems really bad, but actually, if you look, if you kind of like think that the system as a whole, there's a shortage, there's an actual shortage of truck drivers in the United States.
但一个很好的例子是卡车司机。所以有很多自动驾驶卡车公司。是的,很多。我们和很多这样的公司合作,比如Bark、Ike等等。从一种朴素的观点来看,他们只是要自动化卡车司机。如果你看一下美国各州的地图,卡车司机是许多州的热门职业。看起来很糟糕,但实际上,如果你从整个系统的角度来看,美国实际上存在卡车司机不足的问题。
And the median age is like 50 or something crazy. Yeah, exactly. So the sort of this, like, there's this like millennials and Gen Zs are not becoming truck drivers. So there's this, there's this kind of like instability in the market because of all this stuff, right? And, and the, the automated truck driving systems, actually what they would do is automate the, the long hole middles of these truck drivers, the boring parts, which are the boring parts, arduous that displace people from wherever their homes are, etc.
中位年龄大概在50岁左右,有点疯狂。是的,正是这样。所以有一些千禧一代和Z世代不愿成为卡车司机。因此,由于所有这些事情,市场存在一种不稳定。而自动驾驶卡车系统实际上将自动化卡车司机的长途驾驶,这些是令人厌倦的、繁琐的部分,这些部分会将人们从他们的家乡驱逐出去。
Yeah. And allow the current truck drivers to focus on these like higher value trips that are sort of like, uh, warehouse to a, a meeting point or whatnot. Yeah, Dreyage to, like the Dreyage to the factory or even the last mile. I mean, who knows? Like, maybe these trucks will change their form factor and be half the size, be automated. And when the truck gets off the road, the same truck, instead of using 18-wheelers, we might just use smaller mid-sized trucks. That'll be electric and solar powered. So you have more of them. When they get off, they become the delivery truck.
是的。让当前的卡车司机集中精力处理那些高价值的行程,比如从仓库到某个会议点等。然后考虑到从码头到工厂等短途运输,或者即将到达终点的最后一英里。或许这些卡车将改变它们的形态,变得更小,自动化,当卡车离开公路时,我们可以使用小型中等尺寸的卡车,不再使用18轮卡车。这些车将采用电力和太阳能,所以我们可以使用更多的卡车,同时这些卡车下车后,也可以用于交付。
Yeah. And they just automatically start delivering. Yeah. It can be a much better model. Yeah. So, so the, um, these, uh, these sort of like the introduction of machine learning to, uh, to improve the efficiency of the economy, it'll, it'll, it'll be slow because of how the, how in general free market economics work, it'll, it'll take effect in areas where there's an acute problem today, right? It'll happen in those places first and, uh, and it'll allow the, the current jobs exist to become higher value, more impactful, um, et cetera.
是的。他们会自动开始发货。
这是一个更好的模式。因此,通过引入机器学习来提高经济效率,它的推广会很慢。这是因为自由市场经济的运作方式决定的。它会首先在目前存在急需的领域产生影响,并允许现有的工作岗位变得更有价值、更有影响力等。
So, so the, the sort of, uh, well, we believe the true narrative will be, um, will be extremely positive, actually, versus the current narrative, which is like AI and HRI, et cetera. So, we're going to take over the world. Yeah.
我们认为真实的故事将是极为积极的,远远超过当前的AI和HRI等故事。因此,我们将要征服世界。
Silly. I mean, there is a possibility that AI could get out of control at a certain point with exponential computing. That's not far-fetched that it could do something crazy and stupid. You only think that's not far-fetched because you watched a lot of these sci-fi movies. That's the, wow.
这有点傻。我的意思是,随着计算能力的指数增长,人工智能有可能在某个点失去控制。这并非不真实,它可能会做出一些疯狂和愚蠢的事情。你认为这并不离谱,只是因为你看了很多科幻电影。哇,真是令人惊讶。
I mean, listen, if, if you were to train an AI, um, to work on a drug to kill cancer and you didn't program it properly, it could create a drug that was too aggressive because you didn't tell it, well, in the process of killing cancer, please don't make the person blind, right? Or all these other things. So, you could just forget some edge case and some general AI might think, if you said to the general AI, what, you should work on things that make the human species better and go, okay, let's kill cancer. And then it's like, oh, yeah. Or let's, let's cure this communicable disease. Great. I took cure of communicable diseases to kill everybody who have the disease currency, so it can't be communicated. Like, this sounds far-fetched, but there will be instances where they will make the wrong decision, right?
我的意思是,听着,如果你要训练一个人工智能来研制治愈癌症的药物,但你没有正确地对它进行编程,它可能会制造出过于激进的药物,因为你没有告诉它在治疗癌症的过程中,请不要让患者失明,或造成其他一些问题。因此,你可能会忽略一些边缘情况,一些普通的人工智能可能认为,如果你命令它去做让人类更好的事情,那么它就会选择研发能够治疗癌症或传染病的药物。但是,也许它会做出错误的决定,比如研发了能够治愈传染病的药物,却杀死了所有患者,以防止疾病传播。虽然这听起来似乎离谱,但在未来,这样的情况可能会发生。
Or will it be just too slow of a ramp up for us not to catch it in your mind? Yeah. I mean, I do, I think there's like, uh, yeah, there's this, uh, the, um, the thought experience always go like, oh, you'll make an errands comment to an AI and all of a sudden it'll, uh, it'll take over the world and do something that you really don't want it to do. But I mean, in reality, like, there's, uh, there's a lot of oversight over these machine learning systems right now. Like there's, there's like tens, hundreds of people who look at these models, they look at all the data that comes in and out and they look, they like, analyze everything and they, they try to figure out, okay, what is this model doing well? Was it doing poorly and how, how do we adjust to that, et cetera?
或许我们没有足够快地适应,以致于你们心中的事情我们无法捕捉到吧?是的。我的意思是,我认为总是会有这种想法实验,你会给一台人工智能发表评论,然后它就突然掌控了这个世界并做出你不想要的事情。但实际情况是,现在这些机器学习系统有很多监管。就像有数十、数百人查看这些模型,观察所有进出的数据,分析一切,并试图弄清楚这个模型做得好吗?它做得不好吗?我们应该如何调整?
So, um, I think, I think that could happen in a world where it's like, we believe we, we have low oversight of these systems. So oversight is always important in any new technology, right? It's like when we started having, uh, airplane autopilot, for example, it would be crazy to just say, okay, we've airplane autopilot. Just let it fly. Should we put any oversights over the Facebook and social media companies? They have to be clear. They, they didn't have oversight. We think the F, the FCC, like giving a fine in the review mirrors, oversight, it's not oversight. They had no oversight and we lost our democracy over it. The Russians came in and spent a rubble doing it.
嗯,我认为,在我们相信我们对这些系统拥有较低监督的世界中,可能会发生这种情况。因此,在任何新技术中,监管都是非常重要的,对吧?就像我们开始使用飞机自动驾驶时,如果只是说“好了,我们有飞机自动驾驶了,就让它飞吧”,那就太疯狂了。我们应该对Facebook和其他社交媒体公司进行什么监管呢?他们必须明确,他们没有受到监管。我们认为联邦通讯委员会(FCC)像在后视镜里罚款那样,进行监督并不是真正的监管。他们没有受到监管,我们已经失去了民主。俄罗斯投入了大量资金来干这件事。
So that is, uh, that is a, that is one take. They manipulated, they stole the Cambridge Analytica data. They did voter rolls. They tried, and whether it actually caused the election to swing, we'll never know. Perhaps, but they definitely were able to swing some portion of it. They definitely were able to manipulate it successfully. So, and what regulation is there of AI right now? There's none. You, you're, you're acting under zero regulatory environment right now in China's got a negative regulatory environment. It's, it's true that, it's true that like, um, so you should be regulated, uh, to your mission. No, no, no, no, no, this is all I'm saying.
那就是,他们操纵了、窃取了剑桥分析的数据。他们影响了选民名单。虽然我们永远不会知道这是否导致了选举结果的变化,但他们肯定能够影响选举结果的某一部分,并成功地操纵了选举。目前对人工智能有什么规定吗?没有。你在零规制环境下工作,而中国则有负面的规制环境。真的,你应该按照你的任务受到规制。不,不,不,这就是我要说的。
Well, wait a minute. You just said that you should be regulated so that we don't have problems. So which is it? Uh, I do think that there are a lot of important issues about how we, we deem what AI systems are appropriate, how we look at what they're supposed to be doing, et cetera. I do think governing bodies, the US government in particular, for example, has to take a deep look, understand the technology, determine what is reasonable, what is not reasonable, et cetera, and ultimately they're the, the, but even in their case, they're looking at the miles driven in the accidents, but they're not looking at the code that you guys are writing. They're not looking at anybody's code. They're not looking at the AI systems. They don't even have anybody on staff who could even write an algorithm, right?
等一下,你刚才说你应该受到监管,这样我们就不会出现问题。那现在你的想法是什么呢?嗯,我认为有很多关于如何确定什么样的人工智能系统是合适的、如何看待它们应该做些什么等重要问题。我认为执掌政府,特别是美国政府,需要深入研究并了解这项技术,决定什么是合理的、什么是不合理的等等,他们最终是,但即便是他们,他们也只看事故中行驶的英里数,并不看你们写的代码。他们不看任何人的代码,也不看任何人工智能系统。他们甚至没有任何人员可以编写算法,对吧?
Uh, well, that's also changing to be clear. Is it? So the, the, in general, um, I think they're looking at any lines of code in, at any of these systems. Uh, I'm not sure about the answer to that, but I do think they look at a large amount of data.
嗯,好的,为了更清楚表达,这也在改变。是吗?所以一般来说,我认为他们正在查看这些系统中的任何代码行。我不确定答案,但我认为他们正在查看大量的数据。
So these, okay, they do, yeah. So in, um, yeah, in Europe, for example, there are all these, uh, there are these ADAS systems, right? So there are these driver assistance, uh, programs or driver assistance systems and a lot of like high end vehicles that you buy today, right? Keep in the lane. Yeah, exactly. Keep in the lane. If you have like stopping a traffic, you don't need to do anything. Yeah, adaptive cruise control. Yeah, and lane change warning. Yep. Standard on BMW's, BMW said outies these days. Yeah, exactly. So there's all these, um, these systems exist. They, people buy these systems, people rely on these systems. And in the EU, for example, that were a lot of these car makers are where BMW, Audi, uh, VW, etc. are, they, um, they have a responsibility to actually, uh, both have a large data set, uh, that they, they have collected themselves that is able to validate that these systems are performing well, as well as pass, uh, a series of sort of trials and actual, uh, really?
这些,好的,它们确实存在。例如,在欧洲,有许多ADAS系统,即驾驶员辅助程序或驾驶员辅助系统,许多高端车今天你购买就具备这个系统。例如,保持车道,如果你在交通中停下来,你不需要做任何事情。自适应巡航控制,以及车道变更警告。这些都已成为宝马,奥迪等品牌的标准配置。这些系统存在的意义在于人们购买这些系统时依赖它们。例如,在欧盟,宝马,奥迪,大众等许多汽车制造商都有责任拥有大型数据集,以验证这些系统的性能,并通过一系列试验和实际测试。
Yeah, yeah. So, yeah.
嗯,好的。所以,是这样的。
But, uh, we do have a lot of different forms of data that, uh, that the government, that governing bodies place in front of them.
但是,我们确实有许多不同形式的数据,由政府机构提供给他们使用。
Well, that would be very interesting. Now, to think about it, we do crash tests for cars. You're required to give three cars or something to the government for them to just destroy in their crash tests. Yeah. But we don't require those cars to go into a lab, get taken over by the governing body and force them to go into real world testing environments. Because there's some real world testing environment where you do self-driving up north here, I think, some military base.
那将会非常有趣。现在,让我们来想一想,我们为汽车进行碰撞测试。你必须提供三辆车或其他车辆给政府,让他们进行碰撞测试。但我们不需要这些车辆进入实验室,由政府机构接管,强迫它们进入真实的测试环境。因为有一些真实的测试环境,比如在这里向北自动驾驶,在一些军事基地。
Uh, you're referring to, there's a military base that everybody uses for self-driving. It's like a town that was converted into like a self-driving town. Yeah. It's got the name of it. Yeah, a lot of these, um, a lot of these, a lot of these companies, uh, they, they buy cheap real estate. They, uh, they help with them into like these mini towns so they can create these funny scenarios. Have you ever been to one of those? Uh, I've never been, but I've definitely seen the video from that. I've seen the videos, yeah. Yeah, it's pretty cool. Like, children come darting out like a little cardboard cutouts of children to see if it hits it. This way they can do that in private. Yeah.
嗯,你说的是每个人都用于自动驾驶的军事基地。它就像一个被改造成自动驾驶小镇的城镇。是的,它有一个名字。很多这样的公司都购买廉价的房地产,并将它们改造成这些小镇,以便创造这些有趣的情景。你去过那种地方吗?我从来没有去过,但是我肯定看过那里的视频。它很酷。比如,会有一些纸板卡通儿童突然跑出来,看自动驾驶车辆是否会碰到他们。这样,他们就可以在私人场地上进行这些实验。
Uh, but it is interesting that some point the government's going to have to have people who are developers and coders actually getting into the data and understanding some portion of this, right? Uh, at the very least, they'll have to put, they'll have to create like the, the driver's test, for example, the driver's license test for, for a self-driving car. I mean, that will exist.
嗯,但有趣的是,政府在某些时候必须让开发人员和编码人员真正进入数据并理解其中一部分,对吧?至少,他们必须要创建像驾驶考试一样的东西,例如针对自动驾驶汽车的驾驶执照考试。我的意思是,那是必要的。
You believe in general AI? Uh, that will hit that at some point. AI that is just generally smart and do anything human can.
你相信通用人工智能吗?嗯,这个事情会有所折中。通用人工智能可以像人类一样聪明,具备处理各种任务的能力。
I mean, I believe in, in the, I believe in some sense. I believe in the sense that like, um, for most technological things that, uh, humans can conceive of that aren't physically impossible. If, if humans arrive, they'll happen at some point. Like I think, I think humans are like infinitely creative, infinitely ingenious, et cetera.
我的意思是,我相信在某种程度上。我相信在大多数技术方面,只要人类可以想象而不是不可能的话,它们最终会实现。我认为,人类是无限创造力、无限巧妙等等的。
Sure. I think it is very overblown the timelines that people are talking about generally AI happening. Uh, I think it is, uh, there's, I could rant about this for a while. There's a lot of things that are wrong about like the common arguments.
当然可以。我认为人们普遍对人工智能的时间表过分夸大其词。嗯,我认为有很多事情可以反驳这一普遍观点。我可以对此长话短说。普遍观点存在很多错误。
Um, one of which is people say that, uh, like if Moore's Law keeps going, then we'll have all this exponential compute and that's going to like, uh, that's going to be a lock it. Yeah, it's going to mean it's only a matter of time before we produce these general AI. Now, not to mention quantum computing. Yeah, exactly. It's involved.
嗯,其中一个观点是,如果摩尔定律持续发挥作用,那么我们就会有所有这些指数级的计算能力,这将会成为一个锁定因素。是的,这意味着我们生产出这种通用人工智能只是个时间问题。更不用说量子计算了。是的,这很复杂。
So yeah, I mean, Moore's Law is going to, is going to be dead. Yeah, exactly. It's going to be dead. And then, uh, quantum computing is very far away, despite recent press releases, et cetera. Um, and so that, I think that leg of the argument is not actually that strong.
所以,我的意思是,摩尔定律将会死亡。是的,没错,它将不复存在。尽管最近有新闻发布,等等,但量子计算机还很遥远。因此,我认为这个论点并不是那么强有力。
And then I also think it's not even clear that if you have infinite compute, that you, you'll be able to produce general AI, I think that's very unclear. Uh, infinite compute helps narrow AI because you're doing a number of scenarios and, you know, playing out every scenario and go the, the game, uh, the stone based game, many more permutations than poker, many more permutations than chess, which is a finite data set. So yeah, more compute power on those things certainly get you quicker, definitely, or even just throwing people into a random video game like open AI is sure. Definitely. But generally, I taking somebody who've mastered chess and then saying master go and then master, you know, fortnight or master, impressionist painting, it's different. It's very different. It's very, it's, it's, so the argument, uh, one of the arguments goes that once you have enough compute, you can basically, uh, you can basically simulate, um, you can create artificial life by basically simulating evolution.
然后,我认为即使你有无限的计算能力,也并不清楚你能否创造出通用人工智能,我认为这非常不清楚。无限计算能力有助于狭义人工智能,因为你正在执行多种场景,对每种可能性进行推演,而基于石头的游戏具有比扑克牌更多的排列组合,也比象棋更多,因为数据集是有限的。所以,对于这些任务来说,增加计算能力肯定会更快,或者甚至只需要将人们扔到类似 open AI 这样的随机视频游戏中即可。但通常来说,将已经精通棋类的人,请他去精通围棋,占卜之类的,是截然不同的。非常不同。因此,其中一个观点认为,一旦你拥有足够的计算能力,你基本上可以通过模拟进化来创造出人工生命。
So that's, okay. So that's, that's one of the more Vogue arguments. Uh, okay. Let me just see if I understand that you have so much compute power that you can say, start with this tiny, whatever it is, you know, piece of bacteria, whatever, then grow it and grow and grow an entire evolutionary system.
这就是一个“时尚”观点之一。那么,如果我理解正确的话,您拥有如此多的计算能力,可以从一个微小的细菌或其他生物开始,不断生长并演化整个生态系统。
Yes. To the point at which there is a human-like species and then grow that human-like species in whatever number of scenarios with a big brain into whatever comes after us. Yeah. Even, I mean, even if you just grow the human, the, like the human-like species that's as intelligent as, then you're, that's kind of good. That would be general AI as well.
是的。到达一个类似于人类的物种,并在任意数量的场景中使这种类似人类的物种具有大脑,然后使其成为我们之后的任何形式。是的。即使只是培养与人类一样聪明的类人物种,那也不错。那也算是通用人工智能。
Yeah, exactly. Because general AI would normally, most people would define general AI as not even being smarter than us, but being as smart. Yeah, exactly.
是的,完全正确。一般人类智能通常被定义为甚至不比我们更聪明,而是一样聪明。是的,完全正确。
Huh. Um, so, so that's an interesting approach. Uh, yeah. But somebody would have to code that and program that and build the systems to do that. It's not just going to magically happen, right? Yeah, exactly. It's not, it's very unclear if that's even possible. But that's, um, that's an argument. That's, I honestly think that's the most plausible argument, but, um, but, uh, but it's so, it's, um, it's very much so science fiction in the sense that it is, uh, we're not close to being able to even validate the hypothesis. So I, I think, um, I, uh, yeah, I don't believe in general AI anytime soon, uh, despite what the pundits will say.
哦,这是一个有趣的方法。但是,必须有人编写和程序化它并构建系统来实现它。它不会像魔术一样自动发生,对吧?是的,确切地说,目前尚不清楚是否可能实现。但这是一个论点。老实说,我认为这是最有可能的论点,但它非常类似于科幻小说,因为我们甚至还无法验证假设。所以,尽管专家们说什么,我不认为通用人工智能即将到来。
Yeah, what's the next big mind blowing AI project, narrow AI project? Let's say that most people are in considering right now after self driving, which is the one that's captured our attention.
嗯,下一个让人惊叹的AI项目,窄人工智能项目是什么?我们可以说,在自动驾驶之后,大多数人都在考虑这个项目,它已经引起了我们的注意。
Well, I think there's, uh, I think there's a bunch of really boring ones. Okay. Um, so the boring ones are like, hey, can you automate form processing really well? Like, like paper form processing or, oh my god, that is boring. It's super boring, but it'll be big. Uh, just like, what, like I have to fill out a form to get my driver's license and you know, use AI or, yeah, well, anyway, I, uh, we'll move on.
我认为有许多非常无聊的问题。比如说,你能很好地自动化表格处理吗?比如纸质表格处理?哦天啊,这太无聊了。虽然很无聊,但这是个庞大的市场。比如说,我需要填写一份表格来获取驾驶执照,你可以使用人工智能技术处理吗?好了,我们继续吧。
But, uh, yeah, um, there's a lot of boring examples. Uh, there's a, uh, replying to email. That's kind of the dope one in Gmail now. Do you have you seen that?
嗯,有很多无聊的例子。比如回复电子邮件,在Gmail中确实是一项很酷的功能。你有没有看到过呢?
Yeah, it's great. It's pretty demented how fast it's getting good and it's personalized, right?
是啊,这太棒了。它进步得非常迅速而且还是个性化的,是吧?
Uh, yeah, I would believe it's personal. I think it's personalized too because it's starting to use my lingo.
嗯,是的,我相信这是个人的。我认为它也是个性化的,因为它开始使用我的术语。
Yeah.
好的。请翻译成中文,并表达完整的意思,尽量使语言简单易懂。
So I'm like, I would never use that. And then I'm finding myself like, wait a second, it's finishing the sentence in my voice. And then you're like, wait a second, my voice is pretty narrow. I'm a human. Yeah. Um, so giving you suggested replies is actually kind of low-hang you fruit.
我本来觉得我从来不会用那个东西,但是突然发现它是用我的声音完成了句子。然后你又会想,等一下,我的声音很狭窄。我是个人类。所以给你建议的回复其实很容易。
(注:低挂果实(low-hanging fruit)是指很容易摘到的果实,这里指给出建议回复很容易。)
Yeah, it's pretty, and that's like, I would, I would say that's like kind of a boring one.
嗯,它很漂亮,但我觉得有点无聊。
Yeah. Um, but I think there's ones, I think there's applications that have like, uh, pretty large scale economic impact. Okay. So, so for example, all this automated radiology, uh, and automated like medical imaging work, um, is, uh, is very impactful. And the technology is, uh, like the core technology is good enough given enough data to actually make that possible. So I get my lung scan because I was a smoker and they're doing lung cancer. Now they send those X-rays to India to be reviewed by technicians there, uh, who go through it or even, um, heart rate monitors for 24 hours because that's the cheapest labor with the highest, you know, ability. But that can, all that data can just be done by a computer better than a human could ever do it.
嗯,我认为有一些应用程序具有很大的经济影响力。例如,自动化放射学和自动化医学成像工作的应用非常有影响力。只要有足够的数据,核心技术就足够好,就可以实现这一点。我之所以要做肺部扫描,是因为我曾是吸烟者,他们正在做肺癌检查。现在他们将这些 X 光片发送到印度,由那里的技术人员进行审查,甚至进行 24 小时的心率监测,因为那是最便宜的劳动力,但所有这些数据都可以由计算机更好地完成,比人类做得更好。
Yeah. I mean, so for example, so in general globally, there's a huge shortage of doctors. I believe like, um, ridiculous. I believe, yeah, the, uh, the world health organization published something is like 10x shortage in doctors globally. Right. Um, so, uh, so it, like there is, there's this massive shortage. And if you can, if you can fulfill some of this demand with automated systems that are much more scalable, there's a, there's a huge amount of value.
是的。我是这样想的,世界范围内普遍存在医生短缺的问题。我相信这是荒谬的,世界卫生组织发布的报告显示全球医生短缺是10倍。所以说,存在严重的医生短缺。倘若可以通过更具扩展性的自动化系统来满足其中一部分需求,将创造巨大的价值。
Um, and I think it's, it's easy for us to think in the United States, uh, that like, hey, it's not clear what the lift would be. This seems like it'll just automate jobs or whatnot in the US. Yeah. Um, but that's because you, like you already have access to the stable infrastructure that, or not everybody, but, um, but a lot of people already have access to the stable infrastructure that is healthcare, right?
嗯,我认为在美国,我们很容易认为,不清楚这项技术的优势在哪里。这似乎只是会自动化美国的工作或其他类似的东西。是的,但这是因为你已经有稳定的基础设施,比如说医疗保健,很多人(虽然不是所有人)已经拥有了这个稳定的基础设施。
Yeah, no, as bad as our health system is here in America or flawed, probably the better word, um, it's not like somebody's not going to be taken to an emergency room, right? Like, and there's other places where they're just never going to have access to a doctor or maybe once a year.
是的,尽管我们在美国的医疗系统很糟糕或者说有缺陷,更好的说法可能是缺陷,但不像其他地方那样不会有人被送到急诊室,对吧?类似的,还有一些地方的人们可能永远没有机会看医生,或者只有一年看一次医生的机会。
Yeah.
好的。
And getting an x-ray might be out of the question because of the cost.
由于费用过高,进行X光检查可能无法实现。
Yeah. Not just the cost of the x-ray, but the cost of actually interpreting the x-ray. Yeah.
没错。不仅是X光的成本,而且还包括解读X光的成本。没错。
So you think x-rays are the big one?
你觉得X射线是最重要的吗?意思是询问对方是否认为X射线是最为重要的医疗技术。
Well, it's all, all the forms of medical imaging, right? Like x-rays, ultrasounds, cat scans. Are you working on that yet? We do work with a bunch of this data. Yeah.
好的,这包括所有的医学影像形式,对吧?像X光片、超声波、CT扫描等等。你现在正在处理这方面的工作吗?我们确实正在处理这些数据。是的。
And I think this is a, I think. Computers can do it better now than humans or they can queue it up for a human to review more efficiently.
我认为这是个...我认为。现在电脑能够比人类更好地处理这件事,或者它们能够把它排队等待人类更高效地审查。
They can, yeah, a lot of times it's, uh, they, they do some of the work and then the humans can, can do it more.
他们可以,很多时候,它们会完成一部分工作,然后人类可以完成更多工作。
Got it. So like first pass.
明白了。就像第一遍过程一样。
Yeah, yeah. Or, or even, um, or even marking out problem zones and an amade of the work.
是的,甚至还可以标记出问题区域并制作工作的示意图。表达的是在处理某个任务时,可以指出问题所在并制作相应的工作示意图。
Oh, God. So annotating it so the doctor can then.
哦,天哪。这样医生就可以将其注释了。
意思是,有些内容需要加注释,这样医生才能理解它。
Yeah.
好的。请尽量易懂地翻译,以表达清楚的意思。
Start on second base.
从二垒线出发开始。意思是指在比赛中,一个球员从二垒线开始下一步行动。
Yeah, exactly. Um, so I, I think a lot of those systems are, are, um, will be very impactful. Uh, I think that there's, there's a lot of other boring things that people don't think about. Um, and then, and then I think that like, uh, I think that more and more, a lot of the things that, I mean, really the, like, sort of the market forces are the, the things that like, there's either incredible demand for, or the things that people don't like doing are going to, like, are going to be the, the, the clearest things to work on.
是的,完全正确。我认为很多这些系统将产生很大的影响。我认为还有很多人们不考虑的无聊的事情。而且我认为越来越多的事情,实际上是市场力量所驱动的,即用户有极大的需求,或是人们不愿意做的事情,这些将是最明显的研究方向。
I think education is going to be a huge one. Like the adaptive learning where kids can sit in front of a computer and it starts to learn from looking at their facial expressions when they're frustrated or on the, to, to, cusp of being frustrated. I know this sounds like really like, uh, dystopian. But if the computer was watching the child and the child is frustrated at a certain math problem and then it takes them back 20% to an easier math problem and they can tell from the facial expression that they're enjoying it and that they're feeling confident.
我认为教育将会成为一个巨大的领域。比如自适应学习,孩子们可以坐在电脑前,计算机通过观察他们的面部表情来学习,当他们感到沮丧或者处于沮丧的边缘时,可以根据情况做相应的调整。我知道这听起来似乎有些反乌托邦,但如果计算机能够观察并了解孩子的情绪,当孩子对一个数学问题感到沮丧时,它会把难度降低20%,让孩子从一个更简单的数学问题开始,如果计算机能从孩子的面部表情中看出他们感到愉悦和自信,那就更好了。
And then when they feel not confident, they can push a little bit into that. You're, hey, I know you're uncomfortable. Let me walk you through this again or I get the sense that you might want me to work through this again with you. Can you imagine what a Khan Academy with machine learning and adaptive learning and AI could do? Yeah. I think anything in a narrow AI project to teach people how to learn.
当他们感觉不自信时,可以稍微往那个方向推一下。你可以说,嘿,我知道你不舒服。让我再跟你解释一遍,或者我感觉你可能想让我再和你一起解决这个问题。你能想象一下,如果汗学院结合机器学习、自适应学习和人工智能,会有什么效果吗?我认为这是一个狭义人工智能项目,教人们如何学习。
I haven't heard of a project before, but imagine for literacy, there's still lots of people in the planet who can't read and write. Yeah. No, I think, uh, I think it's a clear application. There are, um, I mean, it's fun to talk about healthcare. There are separate problems where education systems are like, are pretty broken and, uh, and are, are not ripe places for, for a lot of economic opportunity. But, um, but these, like a system like you're talking about is, is really, uh, I mean, it was easy to make, right? It's only a matter of time.
我之前没有听说过这个项目,但是想象一下,现在地球上还有很多人不会读写。是的。不过,我认为这是一个明显的应用。虽然谈论医疗问题很有趣,但是教育系统存在另外的问题,非常糟糕,不是经济机会的萌芽地。不过,像您所说的这样一个系统,实际上非常容易创造出来,只是需要时间。
Yeah, yeah. I mean, I think it'd be easier than self-driving or similar challenges. It's very easy. So the easier than self-driving, the challenge of figuring out when someone is frustrated based on their facial, based on a photo of the face is very easy. That's done. Yeah. But combining that with some adaptive learning technology, well, then it's just about, um, it's about understanding what's a hard question, what's an easy question. Yeah, that should be super easy.
嗯,我觉得这比自动驾驶或类似的挑战要容易一些。这很容易。因此,基于某人的面部照片判断他们是否感到沮丧的挑战比自动驾驶更容易。这已经完成了。但是,结合一些自适应学习技术,就是要理解什么是困难问题,什么是容易的问题。是的,这应该非常容易。
Yeah. Nobody's put that glue together. Isn't it amazing that we don't find, I would bet you it probably exists somewhere. We got to find that out. If somebody on the pod is listening and there's an adaptive learning system using AI and facial recognition to kind of understand where the students at. See, that's where I think technology, it's really interesting when you combine two things.
对啊,没人把那个胶水组合起来。不过,令人惊讶的是,我敢打赌它可能在某个地方存在。我们必须找出来。如果播客上有人正在聆听,且使用了AI和面部识别的自适应学习系统来理解学生所处的位置,那就太棒了。你看,当你把两件事情结合起来时,技术真的很有趣。
Like the, for every dystopian, terrorizing thing about facial recognition, you can think of there's 20 you could think of that would actually be amazing. Like, if you knew somebody who was walking on the golden gate bridge and you knew they were despondent and considering suicide, you could literally know that a person walking across the bridge was doing so with the potential of jumping off the bridge. Yeah. I mean, there's a lot of, there's boring machine learning that's really great, right?
就像任何关于面部识别的具有恐怖效果的未来主义事物一样,你能想到的每一个缺点都会有20个优点。比如, 如果你认识一个正在金门大桥上走路而且他情绪低落,考虑自杀,你可以确切地知道这个人正在走在能够跳下大桥的位置。是的。我的意思是,有很多无聊的机器学习真的很棒,对吧?
Which is, um, like Apple watches, for example, or a lot of these like things wearables. Um, Apple watches are set up with an algorithm that can, it basically looks at the accelerometer and how fast you're moving, etc. Yeah. And it can detect when you have a hard fall. Yeah. So if you fall with an Apple watch, it'll detect they have a hard fall. And if you don't respond to it with sometime period, it'll call an ambulance to you actually. Incredible.
这就像苹果手表等可穿戴设备。苹果手表配备了一个算法,可以基本上查看加速度计以及你移动的速度等信息。它可以检测到你有严重的跌倒。如果你摔倒了,它能检测出是否严重,如果你在一段时间内没有反应,它还会直接为你叫救护车。非常令人惊叹。
Which is like, it's a super, now that's science fiction. Yeah, it's crazy. It's actually really crazy, right? And that exists today. It exists today. It's amazing. By an Apple watch, you could save your life, right? Yeah. But, uh, but there's a lot of like boring uses of machine learning that, uh, and this is what, this is like, this is why really it, people should view it as like this, uh, this like crazy, incredible thing.
这简直就像是超级科幻,太疯狂了。它实际上真的非常疯狂,是吧?而现在它已经存在了。这很神奇。通过一个苹果手表,你可以挽救自己的生命,对吧?但是,有很多利用机器学习实现的无聊用途,这就是人们应该将其视为疯狂而不可思议的原因。
I think a lot of people do, but, but, uh, adding to the fire, it enables all of these things that, um, that you couldn't have done before. Uh, and so it, it just, it enhances the sort of like capability set of, of the technology that we've built pretty considerably.
我想很多人都这样认为,但是增加这个功能可以让所有这些之前无法实现的事情成为可能。它显著增强了我们所构建技术的能力集,这是一件好事。
Hmm, we're all this B in 20 years. When you're 42, what do you think the world's going to look like an AI machine learning? If you had to describe it, obviously your company will be a publicly traded trillion dollar company putting that aside.
嗯,在20年后,我们都会成为这个B。当你42岁时,你认为AI机器学习将会是什么样子的世界?如果你必须描述它,显然你的公司会成为一家公开交易的万亿美元公司,暂且不提这个。
Uh, you'll be the richest man on the planet, but putting that aside, what will the world in machine learning and AI look like? Well, wake up in the morning and AI will interact with us, how? Uh, it, it's a good question.
你将成为地球上最富有的人,但撇开这个不谈,机器学习和人工智能的世界会是什么样子呢?嗯,每天早上醒来,AI会如何与我们交互呢?这是一个不错的问题。
I mean, I think it's almost, um, uh, well, one thing is it's very hard. You can see of, right? Because it'll sort of be like big ideas aren't big ideas from day one.
我的意思是,我认为这几乎是一件非常困难的事情,你能理解吧?因为一个大的想法不是从一开始就变成一个大的想法。
There's sort of like slow ideas that snowball and snowball and snowball and then eventually it's sort of like this huge thing that everybody thinks is, is kind of changed the world. Um, so, so it's hard to conceive of how these things happen today, but ultimately I think, uh, the, the sort of the dream and system is, is sort of as a, uh, as a, I mean, a lot of people like this idea of the assistant, right? Like, like a, like a machine learning assistant.
有一种缓慢的想法,就像雪球滚动,滚动,不断增大,最终形成了一件所有人都认为改变了世界的巨大事情。很难想象这些事情如何发生,但我认为,这种梦想和系统最终可以成为像机器学习助手这样的想法,很多人都喜欢这个想法。
But ideally it's, it's some system that you can basically, you interface with it through, uh, through voice or through typing or basically through language. Yeah. And you're able to, uh, dictate, um, like questions or things that you want on the world, et cetera, and it's able to understand that reason through it and then, um, and then understand what the, what the result is.
理想情况下,这是一种系统,你可以通过语音、打字或语言等方式与其交互。然后,你可以用口述的方式提问或者表达想要知道的信息,它可以理解并回答你的问题。并且它还能理解你的需求并给出相应的结果。
And then, uh, a lot of the AI that we're building today, a lot of the machine learning we're building today, which a lot of it is sort of core perception technology, like just understanding what's happening in general, like knowing that there's a car there or knowing that there's a sofa there or whatnot, a lot of that will sort of be a base layer of intelligence that powers the next layer.
然后,我们今天构建的大量AI、机器学习中很多都是基于核心感知技术的,比如只是理解大致发生的事情,比如知道有一辆车或者一个沙发等等。这些将成为下一层智能的基础层,用于提供更强大的AI技术。
And then there will be a base layer of like reasoning or whatnot that powers the next layer and so on. Yeah. It's very interesting. You believe in this like brain interface stuff, neural link? Uh, that is, uh, well, I, I don't have any.
然后会有一个像推理或其他之类的基础层,驱动下一层,如此类推。是的,这非常有趣。你相信这种大脑接口的东西,神经连接吗?那个,嗯,我没有……
I don't have any deep inside on it. I, I know, uh, one of the neural link founders, I think they're working on cool stuff. Um, I don't know. All these things, the question is like, what's the killer app, right? Like what are you actually going to want to use that thing for, um, and what's feasible and where it's like the intersection of those things?
我对此并没有深入的了解。我认识一位神经链接的创始人,我知道他们正在研发很酷的东西。但我并不确定,因为所有这些东西,问题是如何找到杀手级应用呢?你会真正想用那个东西来做什么,什么是可行的,以及这些因素的交集在哪里。
Yeah, ordering food without anything. You just think what you want in a burger shows up. So Uber eats plus neural link means like you and I be looking at each other and I'd be like cheeseburger and you'd be like cheeseburger and I'd be like bacon and blue cheese and you'd be like chatter and turkey bacon and then in 15 minutes or so out.
嗯,不需要任何餐单就可以点餐。你只需要想起自己想要的汉堡,它就会出现在你的面前。所以,Uber Eats 和神经链接一起,就像你和我面对面地看着对方,我说奶酪汉堡,你说奶酪汉堡,我说培根和蓝纹奶酪,你则说切达干酪和火鸡培根,然后大约 15 分钟左右就可以送达了。
But is that that much better than Uber eats? Yeah. I'd be incredible because you would literally have to not spend the 60 seconds to think about ordering it or pressing the button.
这真的比Uber eats好那么多吗?是的。这将是难以置信的,因为你真的不需要花60秒钟去考虑订购它或按下按钮。
Of course, it's not. It's not much different, but it's going to be kind of mind blowing. It is kind of mind blowing today that you can just take out your phone and order with like three clicks and get food. And it used to be like, I don't know, five minutes on the phone making four phone calls seeing who's open. Yeah, I mean, this was the big, by the way, this was like the whole thing with chatbots.
当然不是。虽然它并没有太大的不同,但它会让人感到惊奇。今天,你只需点几下手机就可以点餐并获得食物,这确实让人惊叹。过去,我们可能需要花费五分钟的时间打四通电话来询问哪家店开门。顺便说一下,这也是聊天机器人的一个重要应用场景。
Right? When when chatbots, when there's the chatbots craze, everybody thought like, oh, this is great. It's so much easier.
对吗?在聊天机器人时代,每个人都认为这很棒。它非常简单易用。
But then if you think about the actual number of clicks that you have to make, if you click like 60 times, you get something done with the chatbots versus like three or four times you get like your hamburger from McDonald's or whatnot. What was the world like before the internet?
然后,如果你考虑实际需要点击的次数,如果你点击了60次,使用聊天机器人就能完成一些事情,而仅需点击三到四次就能从麦当劳和其他地方获取汉堡,两者的差别很大。互联网之前的世界是怎样的?
You don't know. Well, I can read books. You don't remember a time before the internet. When you were seven years old, it would have been 2005. You would have been on a broadband connection at home. Did you ever use a dial-up modem?
你不知道。那我可以读书。你不记得互联网之前的时代。当你七岁时,是2005年。当时你家里有宽带连接。你有没有用过拨号调制解调器呢?
I did use a dial-up modem. Really? The dial-up tone. Yeah, really. I remember that. Yeah. For like, keep in mind, I'm from New Mexico. So it was, yeah, not a lot of bread ban out there. A backcountry part of town. A part of the world. Yeah. Well, I mean, are your parents still there? Yeah, my parents are still there. Are they still working there? My father's retired. My mom's still working. Wow. Yeah. Some breaking bad county country, right? Yeah, I'm actually watching Breaking Badger for the first time now. Oh, really? What season are you on? Season five. Yeah. It gets better and better. The first season is so slow. I skipped the first two seasons, but yeah. It gets crazy and I just watched the El Camino. Like they made a movie that takes place after the end of Breaking Badger. And I actually enjoyed it very much, but it's definitely top 10.
“我曾经用过拨号调制解调器。”
“真的吗?那种拨号音。”
“是的,真的。我还记得。”
“哦,我知道了。你来自新墨西哥州,那里bread ban不多,像是一部分人烟稀少的小镇。”
“是的,我父母还在那里。我父亲已经退休了,我母亲还在工作。”
“哇,那就是《绝命毒师》的故事背景吧?”
“是的,我现在第一次看《绝命毒师》。我已经看到第五季了,它越来越好看。第一季剧情过于缓慢,我跳过了前两季,但后面越来越疯狂了。我刚刚看完了《欧康尼奥》。这是一部发生在《绝命毒师》结局之后的电影,我非常喜欢它,绝对是前十。”
Have you watched the sopranos yet? That ended before you were born, I think. I've watched a good chunk of this. Here's what you do. This Christmas break. You're going to be off. Take a break. Is my advice to you as a 22 year old? Actually take a break over the holidays. Watch binge watch the sopranos. It lit. I mean, it was one of the first shows that had a lot of plot lines going at once. Not as many as Game of Thrones introduced or some of these really high density ones.
你看过《黑道家族》吗?我觉得那个是你出生之前结束的。我看了很多集。接下来,我会给你一个建议。这个圣诞假期,你可以放松一下,作为一个22岁的年轻人,我建议你好好休息一下,抽时间来看看《黑道家族》。这是非常好看的。这个剧集是其中几部同时有很多情节的电视剧之一,虽然没有《权力的游戏》或某些高密度的电视剧多。
At some point, television writers realized if they made it more dense and harder to follow, you would actually get more out of it. And then because DVDs existed, people could go back and watch the previous seasons on Netflix or by the DVDs. And it was in the best interest of the people making the TV shows to make them more dense, to create more characters, to create more plot lines and make it more complex because they drove more DVDs sales. Think about that as a system. The DVD was such a popular format and was so profitable that it impacted the art. Because before that, they said every episode needs to stand on its own. If you've never watched an episode of the Brady Bunch, this episode of the Brady Bunch, you don't need any prior knowledge. So then you were like, it was almost like Groundhog Day. You're waking up every day and you don't even know there's ever been another episode of Gilligan's Island because they're all just singular pieces of work.
在某个时候,电视剧作家意识到如果他们让剧情更加密集和难以跟进,你实际上会从中得到更多的乐趣。然后由于DVD存在,人们可以回去在Netflix上观看以前的季节或通过DVD。对于制作电视节目的人来说,让它们更加密集,创造更多的角色和情节线,并使它更加复杂是符合他们最佳利益的,因为这样可以推动DVD的销售。将其视为一个系统。DVD是如此受欢迎且利润丰厚,以至于影响了艺术。因为在那之前,他们说每一集都需要独立存在。如果你从来没有看过《布雷迪一家》,那么这一集,你没有任何先前的知识就可以看。所以你会像《第二春》一样,每天醒来,甚至不知道《吉利根岛》还有其他的剧集,因为它们只是单独的作品。
But the sopranos was another TV show called The Shield where the first to make them very intense, lots of characters, lots of themes, and then having story arcs that would go over seasons. And so you'd have multiple arcs over multiple seasons. And of course the wires considered the king of the genre. And I've only gotten five episodes into the wire and I stopped because my wife wants to watch with me. Okay.
但《黑道家族》并不是唯一一部叫做《盾牌》的电视剧,它让观众感受到很强烈的紧张感,有很多人物和主题,并且有跨越多季的故事弧线,所以你会在多个季节中看到多个弧线。当然,《纸牌屋》是这个类型的王者。我只看了五集《纸牌屋》,现在停下来等着我的妻子和我一起观看。
We'll end here with our favorite new game show. That's the game show where we pull up your tweets. And we say like, retweet or block, you're saying it. Well the audience is going to do it too. Here we go. We're going to pull up your first tweet. Now you're querying your mind going, what tweets have I done? Here's your first tweet. Many folks believe there's a smooth continuum between good people and great people. In my experience, there's a huge band gap between good and great. What you're referring to here is like a band like an electron band. Yeah. Yeah, the electrons, there's like these gaps where they won't be the only thing. They can't cross. Exactly. Great people are clever, determined, fight hard, moral oil and hardship and strategic. It's obvious when somebody's great. And the counter to this is, hey, it is obvious as well when they're just good. So the difference between good and great is exponential in your mind. I would believe that. Yeah. To me, this is a like and a retweet. Woo! I like it. You get both.
我们最喜爱的新游戏节目就到这里结束了。那就是展示你的推文的游戏节目,我们会说出“点赞”、“转发”或“屏蔽”,你来决定。现在观众也要参与进来。我们现在要展示你的第一个推文,你可能在想,“我发了哪些推文呢?”你的第一个推文是:“许多人认为好人和伟人之间是平滑的连续状态。但在我看来,好与伟的差异非常大。”你这里所指的是一个类似于电子能量带的带隙。对,电子就是有那些不能跨越的缺口。没错,伟大的人是聪明、坚定、勇于战斗、在困境中能保持道德和策略性思维。当有人真的很伟大时,这是显而易见的。而与之相对的是,当有人只是好时,也是显而易见的。所以在你的心目中,好与伟大之间的差距是指数级的。我相信这也是一篇推文和转发。太棒了!
Alright, here we go. Now, here's one that people said might have been a little callous. No, I'm joking. How to build something insanely great. According to Alexander Wang, you could follow him. He's Alexander, underscore Wang. No e. It's just a and dr underscore Wang. Build something you care about. Number two, find users. Listen to them. Three, improve it every single day. This is the one people leave out. Four, find people who inspire you and convince them to work with you. Ah, yes. Five, repeat. Two to four for years. Correct. Just five simple steps. Yeah, the devil's in the details there. It's really hard to improve every single day, isn't it? Super hard. Super hard. You get more people, it gets harder. Yeah. I mean, there's a, this is what somebody told me. So this is a second hand. They told them they're kung fu teacher told them this or something. You're either, every day you're either getting better or you're getting worse. There's no, there's no sideways, no saying the same. Yeah. And so your products are either getting better or getting worse. Right. Because if you are not improving it, it's deprecating and a competitor is improving. And your users are getting used to this average product.
好的,我们开始吧。这里有一个人们说可能有点冷酷的问题。不,我开玩笑的。如何建造一个非常棒的东西。据亚历山大·王(Alexander Wang)说,你可以跟随他的步伐。他的名字是 Alexander underscore Wang,没有 "e",只是 a 和 dr underscore Wang。第一步,建造你关心的东西。第二步,找到用户并且倾听他们的需求。第三步,每天都不断改进。这是许多人忽视的一步。第四步,找到那些能够激励你的人,说服他们与你一起工作。第五步,重复执行第二步到第四步,坚持多年。只需要五个简单的步骤。但很难每天不断改进,对吧?非常困难。团队越多,难度越大。有人告诉我,这是他们的功夫老师告诉他们的。每天你要么进步,要么退步。没有平行,没有停滞。这样说,你的产品要么进步,要么退步。因为如果你不不断改进产品,它就会退化,而竞争对手则在不断改进。同时,你的用户也会习惯于这款平庸的产品。
Okay, here we go. Watching YC alumni demodays, if you thought one, none of these companies are as good as mine. Number two. That's not what it says. I'm joking. I'm joking. I'm like, what have I got to be something to?
好的,我们开始吧。看着YC的校友展示日,如果你觉得这些公司一个都不如我的好。第二点。这不是字面意思。我是开玩笑的。我在开玩笑。我是说,我为什么要完全符合那个标准呢?
Okay, watch your, I say what many ideas derivative to the hot financing's up today, Door Dash Compass, Checker, Hems, etc. Two, lots of duplicate. 175, greater saturation point for startup ideas in all likelihood. Greater than one of the unhipped ideas will be the big company. So what you're saying here is, being a follower, it never becomes a big business and maybe 175 is just too big. It's too many.
好的,注意听,我讲的是今天众多热门融资项目中的许多衍生创意,如Door Dash Compass、Checker、Hems等。其次,这些创意大部分都存在重复现象。175个创业创意已经接近饱和点。很有可能,这些创意中有一个会成为大公司。所以你的意思是,作为一个追随者,这些创意永远也不可能成为大企业,也许175个创意已经太多了。
That's what, yeah, that's what I was saying. I think there's, there's, there's, did you go to YC? We did YC, yeah. Okay. So what do you think, when you went, how many people were there and what do you think about this ginormous class size? A hundred companies. Even then there was like, there was duplicates. There were people working on similar stuff. What do they do when there's people working on competitive ideas in the same class? There's one thing for class, the class. That creates enough tension amongst the loyalty and, oh, now you've got, you're on the cap table of two competing companies, but in the same class?
这就是我所说的,是的,这就是我在说的。我想有,有,有,你去过YC吗?我们去了YC。好的。那么当你去的时候,有多少人在那里,你对这个庞大的班级规模有什么看法?有一百个公司。即使在那时候,也有一些重复的内容。当有人在同一个班级中工作竞争性的想法时,他们该怎么办?对于班级的一件事,这就足以在忠诚度之间产生足够的紧张感,噢,现在你已经在两个竞争的公司的董事会中了,但是在同一个班级中?
I think they're les a fair about it. I think they just, they let it go. They give a lot of love. They give everybody love. And they're like, go ahead. Yeah. Fight it out. Yeah. Interesting. What's the right number? I don't know. 50. I think a hundred is fine. I mean, again, it's like, it's your, your black swan farming. So, you know, takes as many as it takes.
我觉得他们对此并不太在意。我认为他们只是放任不管,给每个人都爱。他们就像是说:“来吧,打起来吧,有意思。”什么是正确的数量呢?我不知道,50个?我认为100个也可以。再说了,这都是你的黑天鹅农业,需要多少就拿多少。
Yeah. That's what people don't understand. The systems like the, like, why combinator or just Silicon Valley in general seem broken to a lay person who's not part of the system because they look at and say, this is too many failures here and too many derivative ideas and these people seem unqualified. And this company got too much money. And then what they don't realize is that chaos that we talked about at the beginning of the show can lead to people being given permission to try something outlandish that then in fact changes the world in a way that nobody could have determined, which is the definition of a black swan is that you could not have seen it coming until you've seen it.
是的。人们不理解这一点。像why combinator这样的系统,或者整个硅谷系统对于一个不属于这个系统的普通人来说似乎是有问题的,因为他们看到的是太多的失败和派生的想法,这些人似乎没有资格。这家公司得到了太多的钱。然后他们没有意识到,我们在节目开头谈到的混乱可以导致人们被允许尝试想象性的事情,这也会以一种没有人可以预见的方式改变世界,这就是黑天鹅的定义,直到你看到它之前,你是无法预见它的。
Yeah. Because up until the point of the black swan, nobody ever believed there were anything other than white swans. Yeah. Exactly. What a great book. Really good. Did you read anti-fragile? yeah. Great. That's my favorite.
是的。因为在黑天鹅出现之前,没有人相信世界上有白天鹅之外的鸟类存在。是的,没错,真是一本好书。你读了《反脆弱》吗?是的。非常棒。那是我最喜欢的一本书。
Yeah. Developing companies and systems that do better in chaos. yeah. What a tremendous idea when you think about it. The world's getting more chaotic and you're doing better. Trump. Captain Chaos. yeah. No, he's the. It seemed to leave is really good. Oh, I thought you were saying Trump. No, no. No, no, no. No, no, no. I'm not. No opinion on Trump. But.
是的,开发能在混乱中表现更好的公司和系统,这真是一个了不起的想法。随着世界变得越来越混乱,你的表现变得更加出色。特朗普。混乱船长。没错,他就是。似乎离开会真的很好。哦,我以为你在说特朗普。不,不是的。我没有对特朗普的看法。但是......
No, what I love about it seems you follow him on Twitter. He is brutal and awesome. He's crazy on Twitter. yeah, yeah. He's got wild. He's. He's like Steve Pinker. He's like Steve Pinker is an absolute moron. yeah. I'm like. yeah. Steve Pinker's a moron. Really? Steve Pinker's brilliant. What are you talking about? I do think. I think directness is good, but I think black and whiteness is bad. So, you know, I'm not totally going to endorse his Twitter activity.
不,我喜欢的是你在推特上关注他。他很残酷,很棒。他在推特上太疯狂了,确实。他很疯狂。就像斯蒂夫·平克。他像斯蒂夫·平克一样,是个绝对的白痴。是的。我就是这个意思。没错,斯蒂夫·平克是个白痴。真的吗?斯蒂夫·平克是个天才。你在说什么?我认为直接表达很好,但过于黑白分明是不好的。所以,你知道的,我不会完全支持他在推特上的行为。
Yeah. But I do think. I think people need to be okay with disagreement. yeah. It's so weird that you say that as the 22 year old because this whole generation that you're a part of, they're literally going to college. This is why you left it a year or there. And they're protesting when they bring somebody to campus who they don't agree with. Imagine that. You don't agree with a person. They're coming. You're diametrically opposed to their opinion. And then you protest having them there. It's like, well, you could either go to the lecture and learn about the person who you disagree with and no understanding either the enemy or the opposing side or the other side of the argument makes you so much richer because of that. And they're like, no, you're platforming them.
是的,但我认为人们需要接受不同意见。很奇怪,你作为一个22岁的年轻人会这么说,因为你所在的这一代人,他们正在上大学。这也是为什么你离开了一年。当他们邀请一个不同意见的人来校园时,他们会抗议。想象一下,你不同意某个人,但他们来了。你们的观点是完全相反的。然后你抗议他们来的行为。你可以去听讲座,了解你不同意见的人,理解敌人或者对立面的观点,这将让你变得更加丰富。但他们说:“你正在给这些人提供平台。”
Platforming. Since when does talking to somebody mean you're platforming them? Like, people are like, you talk to Steve Bannon. Bannon, you're platforming them. So, what does that even mean? yeah, I think the guy put Trump in office, he ran Breitbart. These things are having a major impact on the world. You can't have a conversation with him even if he think he's evil. I think there's a big problem when you have like, I think this is like a derivative of like too much content out there. So basically, people can anyone who has any belief can basically read enough content that reinforces that belief. Like for all these people, for example, they believe they have internally a very clear picture and a very high confidence perspective on like who these people are with they believe etc.
"平台化"。从什么时候开始和某个人交谈就意味着你在为他们创造平台了?比如,有些人会说,你和史蒂夫·班农说话。班农,你就已经为他创造了平台。那这到底是什么意思呢?是的,我认为那个人让特朗普当选,他运营过布赖特巴特。这些事情对世界产生了重大影响。即使你觉得他很邪恶,你也不能与他交谈。我认为当你有太多的内容时,会出现一个大问题。因此,基本上,任何人都可以通过阅读足够的内容来强化他们的信念。比如,对于所有这些人,他们都认为自己内心清晰地看到了这些人的真实面目,以及他们的信仰等方面的高度信心的观点。
And that's just how, by the way, this is just how human brains are wired. Human brains are wired to be like, oh, you have a couple data points. Okay, you have to believe that. Yeah, tribalistic. Right. Because it's like in, before now, it was actually, it was difficult to get these data points that all told consistent narratives, etc.
顺便说一句,这就是人类大脑的工作方式。人类大脑的工作方式就是这样:你只要有一些数据点,就会认为那是真实的,而且往往带有部落主义色彩。这是因为,在过去,获取这些数据点并构成一致的叙述是相当困难的。
But now because like, there's just so much content out there and you can like, you can read all of it and you can develop these very strong opinions. It's hard to think of other people as like nuanced human beings. Versus these like very one note kind of like figures.
现在由于网络上有如此多的内容,你可以阅读所有的内容并形成强烈的意见。这很难把其他人视为非常多面化的人类个体,而不是单一的形象。
Which is mind boggling since anybody in their life need only look at their own life, whether it's 22 years on the planet or 48 or 98. And realize how many times they've changed their mind about it and issue. You need only like, is your favorite ice cream the same for your whole life? Which your favorite ice cream right now?
这是令人难以置信的事实,因为生活中的任何人只需要看看自己的人生,无论是在这个星球上度过了22年还是48年或者98年。然后就会意识到自己在思考和观点上改变的次数有多少。你只需要想一想,你喜欢的冰淇淋是一直没有改变过吗?现在你最喜欢的冰淇淋是什么?
For me, it actually has been the same for me. Coffee ice cream, we just love that. No, no, no. Chocolate chip. Yeah. So you haven't had butter pecan in a while. You need to just try that butter pecan one time. That might change everything for you. Well, yeah.
对我来说,一直都是这样的。我们都喜欢咖啡口味的冰淇淋。不,不,不,巧克力片片的口味。嗯。你已经有一段时间没吃过奶油山核桃口味了,你得试试那种口味,也许会让你改变看法。嗯,是的。
Well, I don't know. I mean, mint chocolate chip is kind of close to heart, close to my identity. Have you been to salt and straw yet? Salt and straw. You had the mint chocolate chip there? The mint is so fresh. It feels like you're chewing on mint leaves.
嗯,我不知道。我的意思是,薄荷巧克力碎片对我来说有些亲近,靠近我的身份认同。你去过盐和秸吗?盐和秸。你尝过那里的薄荷巧克力碎片吗?薄荷非常新鲜,感觉就像你在嚼薄荷叶子。
Yeah. And we'll go right now. Everybody will see you at Salt and Straw next time on This Weaknessywerbs. Bye-bye.
没错,我们现在就走。下次在本节目中,大家会看见你在Salt and Straw。再见。