How to Capture the AI Moment (Bobby Yerramilli-Rao, Chief Strategy Officer at Microsoft)
发布时间 2023-04-26 18:32:43 来源
摘要
As everyone listening to this podcast knows, the release of ChatGPT last November ushered in a new age of AI and catalyzed a wave of AI startups. For this episode of B2BaCEO, Ashu goes deep on AI with Bobby Yerramilli-Rao, former serial entrepreneur and investor, and current Chief Strategy Officer at Microsoft.
Ashu picks Bobby’s brain on what sectors and problem spaces he thinks are ripe for innovation with AI and what the opportunities for startups are. They then dissect the four layers of the AI stack, from applications to platforms to infrastructure to models. Lastly, they talk about flavors of artificial intelligence beyond large language models.
If you’re a founder or executive who wants to understand the AI moment and all the opportunities it holds for enterprises and society — listen to this conversation.
GPT-4正在为你翻译摘要中......
中英文字稿 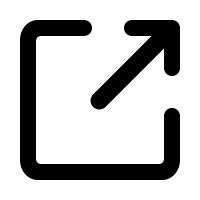
From Foundation Capital, this is B2B SEO, a podcast about the startup journey, what going from idea to IPO, and growing from a founder into a CEO. On each episode, I speak with notable CEOs and founders and get their stories, but what they took to build a company of scale and become a leader in the enterprise. I'm Ashu Gard, a general partner at Foundation Capital.
这是Foundation Capital制作的B2B SEO播客节目,旨在讲述创业之旅,从构思到上市的过程,以及从创始人成长为CEO。每一期,我都与杰出的CEO和创始人进行访谈,了解他们的故事,以及他们所采取的方法建立规模化的公司,并成为企业领袖。我是Foundation Capital的常务合伙人Ashu Gard。
As everyone listening to this podcast knows, the release of ChatGPD last November are shared in a new age of AI and catalyzed a wave of AI startups. The team at Foundation Capital has backed AI first startups since 2010 and we are privileged to be early investors in companies like Databricks, Eightfold, Jasper, Cerribrares, any scale and a rise.
正如本播客的所有听众所知道的那样,去年11月的ChatGPD发布引领了一个新时代的人工智能,并激发了一波人工智能初创公司的发展。Foundation Capital的团队自2010年以来一直支持人工智能初创公司,并且我们很荣幸成为像Databricks、Eightfold、Jasper、Cerribrares、any scale和rise这样的公司的早期投资者。
We're in a special moment in time where giants like Microsoft, Google and OpenAI are hogging this spotlight and every AI entrepreneur is wondering how to dance with the elephants. So for this episode of B2B SEO, I invited Bobbi Rao, a friend, serial entrepreneur, former VC and currently Chief Strategy Officer at Microsoft to share his point of view.
我们正处于一个特殊的时刻,像微软、谷歌和OpenAI这样的巨头正在抢占这个聚光灯,每一个人工智能企业家都想知道如何和这些大象跳舞。因此,在这期B2B SEO节目中,我邀请了我的朋友、连续创业者、前风投家和现任微软首席战略官鲍比·饶来分享他的观点。
I picked Bobbi's brain on what sectors and province spaces he thinks are right for innovation with AI and what the opportunities for startups are. We then dissect the four layers of the AI stack from applications to platforms to infrastructure to models. Lastly, we talk about AI beyond large language models.
我向Bobbi请教了他认为哪些领域和省份空间适合利用人工智能进行创新,以及初创企业的机会是什么。然后,我们将人工智能堆栈从应用程序到平台再到基础设施和模型分为四个层次进行了深入探讨。最后,我们谈论了超越大语言模型的人工智能。
If you're a founder who wants to understand the AI moment and all the opportunities it holds, listen to this episode.
如果您是一位创始人,希望了解人工智能时代及其所提供的所有机遇,请收听本期节目。
Hey, Bobbi, welcome to the B2B of CEO podcast.
嘿,波比,欢迎来到B2B的CEO播客。这句话的意思是欢迎Bobbi来到一个名为B2B的CEO播客节目。
Pleasure to be here, Ashin. Thank you for joining us today.
很高兴能在这里见到你,Ashin。感谢你今天的加入。
Perhaps you could start by just introducing yourself a little bit and talking about some of the highlights of your career.
也许您可以先简单介绍一下自己,谈谈您职业生涯中的一些重要亮点。
Sure, I was born in India, moved early age to the UK as you can tell from my accent. Grop there to my undergraduate engineering and PhD. I spent a lot of time actually in high school with electronics and coding and became fascinated with that whole area, decided to pursue it to the level where I started actually becoming very interested in robotics. So that's what my PhD was in.
没问题,我出生在印度,年纪很小的时候就移居到了英国,正如你听得出我的口音。在英国,我完成了本科工程学位和博士学位。在我读高中的时候,我花了很多时间学习电子和编码,对那个领域产生了浓厚的兴趣,决定将其发展到更高的水平,并对机器人技术产生了浓厚的兴趣。因此,我的博士论文就是关于机器人技术的。
My postdoc, unfortunately, I was leaving my PhD in postdoc at a time where it was difficult to find good engineering roles which is kind of difficult to conceive of, given what's going on today. So I ended up actually in McKinsey and through that had a long and winding career of one of the business side, we're ended up becoming a, she's trashy on syrup, had to abode and ended up selling some companies and then ended up running a venture fund, not quite as illustrious as foundation but nevertheless a fund.
很不幸,我在博士后期间离开了博士研究生,当时很难找到好的工程师职位,这是难以想象的,考虑到今天的情况。最终我加入了麦肯锡,并在商业方面有了一段漫长而曲折的职业生涯,在其中成为了一名她里斯的董事,居住在阿博德,结束了一些公司,最终开始管理风险基金,虽然不够杰出,但仍是一个基金。
And now I'm going to say it was a great fun, you had some great companies. We got lucky but like every year, investors we stopped our tow, good amount as well. Fortunately we got a bit more lucky than tow stopping but we had our share of wounds to lick. But it was a great experience all around and now here I am at Microsoft, Frank Swatchy.
现在我要说这是一场非常有趣的旅程,你们都是非常好的伙伴。我们很幸运,但就像每一年一样,投资者也会在某些方面停滞不前,而且投资金额也不小。幸运的是,我们比停滞不前的情况更加幸运,但我们也有自己的伤口需要舔舐。但总的来说,这是一个很棒的经历,现在我在微软,是Frank Swatchy。
It must be a fun time to be running strategy at Microsoft given all that's going on in the world of AI.
在人工智能的世界中,微软战略部门的工作一定十分有趣。
It's actually, it's extraordinary and I don't think that anybody could really have predicted how it was going to evolve and how rapidly things have taken off. Certainly, you know, I assume you are on the same Microsoft as well, and then to Fugera, Yuff, now we're in an era which was already exciting when you think about the migration to the cloud which is still in relatively early innings, the growth of SaaS and some of the interesting evolutions of other aspects of the landscape. But once you add in this PI momentum to it, it just becomes a whole different level of interesting.
实际上,这是非常不同寻常的,我不认为任何人都能够真正预测它将如何演变以及事情将以多么快的速度发展。当然,我假设您也是同样的微软人,然后是Fugera,Yuff,现在我们正处于一个令人兴奋的时代,当您考虑迁移到云的时候,这仍然处于相对早期的阶段,SaaS的增长以及其他方面的有趣演变。但是一旦加入PI的势头,它就成为一个完全不同层次的有趣。
And what is it about the AI moment that stands out for you? Are there one or two things? Are there sort of the highlights or sort of the high notes, so to speak?
对于您来说,人工智能时刻的最突出之处是什么?有没有一两个方面?有没有所谓的亮点或高潮?
You know, I think I've been in technology for a while, as I mentioned, I was even proud owner of a soldering on in my bedroom as an early teenager and coding up on very early computers and writing programs such as publishing magazines. So I've been relatively paid out nerd for a period of time. And what's always interesting is to see something which just completely breaks out of the trajectory of what is expected.
你知道的,我觉得我已经在科技领域待了一段时间了,我甚至在我十几岁的时候就成为了一位骄傲的卧室焊接师,并在早期的计算机上进行编码,写程序,如出版杂志。所以,我在一段时间内一直是一个相对“书呆子”。“总是有趣的是看到一些东西完全打破了预期的轨迹。”
And you know, when I was an undergrad, we had neural networks that were, you know, two or three layers deep and could look at an A by A pixel array of an A or a B or a one or a two and resolve between the two and everyone go very excited about it because this is a very different computing paradigm to sort of more algorithmic and logic based approaches which are more deterministic.
当我还是一个本科生的时候,我们已经有了神经网络,这些网络可以深度达到两三层,并且可以查看由 A 或 B、1 或 2 组成的 n × n 像素数组,从而在其中进行区分,人们对此感到非常激动,因为这是一种非常不同的计算范式,相对于更基于算法和逻辑的方法更加确定性。
So people got excited, obviously, that Bloom came off that rose very rapidly and these things didn't work beyond that at that time. So people got about it. But now what you're seeing is just a level of progress that was that is completely off any kind of graph that you could draw in terms of projection into the future as to how things would evolve.
显然,当布卢姆很快落寞,这些事情在那个时候并没有效果,人们感到很激动。所以人们纷纷行动起来。但现在你看到的进展水平完全超出了你可以绘制的任何未来发展的投影图表。
And so that's one thing which makes it just extraordinary when you see these massive leaps that you often see in the in the core sciences in physics of chemistry and biology sometimes you see these massive unexpected leaps typically. That is how you see progress in those areas, which is not much happens and suddenly there's a massive leap. In technology that happens much more rarely and so it was extraordinary to see that here which is just something that nobody had anticipated.
因此,当你在物理、化学和生物学等核心科学领域经常看到的这些巨大飞跃时,这就是一件特别不寻常的事情。通常情况下,你会看到这些巨大的意想不到的飞跃,这就是那些领域进步的方式,不会有太多发生,突然间就会有一个巨大的飞跃。在技术领域这种情况发生得更少,因此看到这种情况是非常不寻常的,这是没有人预料到的。
And I must say that if you'd asked me, I would have said I would not have anticipated seeing this level progress in my lifetime and I hope to live for a bit longer. So I really hadn't expected to see that in my expected lifetime. So that makes it kind of extraordinary.
我必须说,如果你问我,我本不希望在我的有生之年见证如此伟大的进步,但我希望能够再活上一些时间。所以,我真的没有预料到会在我的预期寿命内看到这样的进展。这使得它有点不可思议。
I think then the other thing which makes it extraordinary is just the general purpose utility of it. It's so fundamental. Its capabilities are so broad that it really sort of harkens back to some of the most fundamental innovations that created the technology industry in the first place. I often think of it as being almost like the transistor. It's just one of these things that just enabled a massive amount of change and value creation and opportunity and innovation to all just do one go.
我认为使其非凡的另一件事情就是其普遍的实用性。它非常基础。它的功能如此广泛,以至于它真正地回归到了最初创造技术产业的一些最基本的创新。我经常把它想象成晶体管。它就像是这些东西之一,可以让大量的变革、价值创造、机遇和创新一次性地实现。
And here's where you think if you had to start sort of seeing out in the future and what are some of the areas you're most excited about. Well, I think that for me one of the most interesting things about technology is the ability to democratize access to services good, it's an opportunity for people at large. It's important to know that there are, it's not just people living in developed and wealthy economies that can benefit from all this.
在这里,你会开始思考,如果你必须开始展望未来,那么你最为兴奋的领域是什么。我认为,对我来说,技术最有趣的事情之一就是能够将服务和商品民主化的能力,这为广大人民提供了机会。重要的是要知道,并不仅仅是生活在发达和富裕经济体中的人们可以从中受益。
There are eight million people on the planet. And I was for a period of time in the mobile industry at a time when that was going through an enormous growth period. And if you look now country by country other than some countries where they got real structural issues as societies and governments, pretty much every country in the world has greater than 90% penetration of mobile technology. Not everyone's carrying a smartphone but people can make goal. There is a tech message is they can interact in ways that they could not even 20, 25 years ago.
地球上有八百万人口。在移动行业经历了一个巨大的增长期间,而我就是其中的一员。现在,除了一些社会和政府存在结构问题的国家,几乎世界上每个国家都拥有超过90%的移动技术普及率。不是每个人都携带智能手机,但每个人都可以通话。科技信息的意义在于人们可以以一种崭新的方式互动,这是20到25年前无法想象的。
And what that has done is it's really inflected the growth in productivity and opportunity for every person on the planet. Maybe people are not able to do a conference call while they're driving down their car, down the freeway. Maybe they use the phone instead to make sure that they have access to confirmation to keep them safe or allows them to know where they can go and sell their their chrome. But there's some value that's created there that affects everybody. And that I think was a very important aspect of the mobile industry and certainly something that a lot of us who are involved in were inspired by.
这真正影响了全球每个人的生产力和机会增长。也许人们不能在驾驶汽车行驶过程中进行会议呼叫,他们可能会使用电话来确保他们可以获取确认信息以保持安全,或者让他们知道可以去哪里出售他们的商品。但是这里创造了一些价值,影响到每个人。我认为这是移动行业的一个非常重要的方面,也是许多我们参与其中的人受到启发的东西。
And I think that with AI as you've mentioned, the chat GPT moment has caught the zeitgeist of people everywhere and it's not just the dinner parties in the valley or in New York or in London. It's actually everywhere. Everybody is talking about this and every in every strata. And that I think is great because if we get to the point where this technology can help everybody in some way or the other, I think that will be a magnificent achievement.
我认为AI如你所说,在社交聊天方面的GPT时刻已经抓住了全球人民的时代精神,这不仅仅发生在硅谷、纽约或伦敦的晚宴上,而是到处都有。每个人都在谈论这个话题,不论身处哪个领域。我认为这非常好,因为如果这个技术能够以某种方式帮助每个人,那将是一个了不起的成就。
It struck me as you were talking. And I had read about something called Kishan GPG, which is an AI chat part that seems to be designed to help Indian farmers with their agriculture and their farming. I mean, it is truly remarkable that within months of chat, they've been being launched. There's a very thing available for farmers in India. And as you said, given what's happened in Telecom, in the mobile industry, literally every farmer in India can access it. It is tremendously exciting. And I think where mobile technology took 25 years to go from something that was an interesting sounding thing to almost a little bit of a professional development.
当你说话的时候,我想起了一件事。我读到了一种叫做Kishan GPG的人工智能聊天机器人,它似乎是专门为印度农民提供农业和耕作方面帮助的。这真是令人惊叹,仅仅几个月的时间,就已经推出了这种聊天机器人,对印度农民来说,这是一个非常宝贵的资源。正如你所说,鉴于电信和移动通信领域的发展,印度的每个农民都可以使用它。这真是一件非常令人激动的事情。我觉得,移动技术用了25年的时间,才从一件听起来很有趣的东西,发展成了一项专业技术。
It'll be interesting to see how AI, what trajectory AI is. Are there particular sectors or problem spaces that you think are particularly right for innovation, given what LLMs are capable of? I think there's two or three that I get particularly excited about. I don't think it's going to surprise you when I mention healthcare as one.
看看AI会走向何种轨迹会很有趣。在您看来,有没有特定的领域或问题空间是适合LLMs创新的?我认为有两个或三个让我特别感兴趣的。当我提到医疗保健时,我不认为这会让您感到惊讶。
As you know, I started a couple of companies in the biotech arena. And I think that there's just extraordinary opportunities. I wouldn't come myself in any way as a healthcare expert, having said that. But I do think there's extraordinary opportunities to help every part of the health and life sciences ecosystem.
众所周知,我在生物技术领域创立了几家公司。我认为这里有非常不错的机遇。虽然我不会自视为医疗行业的专家,但我认为在健康和生命科学生态系统的各个方面都有非常不错的机遇。
There's Peter Lee here, who was a colleague of mine here in Microsoft who runs our research analysis, written a book where he's been looking at applying the GBT models to physicians daily lives. And he's been looking at this for the last six months, so there are a number of esteemed practitioners in the space. And it's just absolutely mind-blowing for some of the physicians who have the emergency to interact with it in terms of how well the model does in helping the physician to diagnose and making sure that the physician has the ability to not miss something. And even extraordinarily helping physicians to know how to deal with patients who are going through very difficult times and how to cancel the parents of a sick child, for example, is just extraordinary.
这里有一个彼得·李,是我的微软同事,他负责研究分析并写了一本书,研究如何将GBT模型应用于医生的日常工作中。他已经进行了六个月的研究,因此这个领域有许多受人尊敬的从业者。对于一些需要紧急处理的医生来说,这个模型帮助医生确诊和避免疏漏的能力实在是令人惊叹。甚至帮助医生应对那些正在经历非常困难时期的患者,以及如何与一个生病的孩子的父母进行交流,都是非常出色的。
Now, it doesn't mean it's perfect, it's all has the ability to eliminate it, etc. But we're seeing just amazing progress there in that sense in the physician space. Then when you think about also diagnosis, the ability for AI to help with diagnosing and analyzing radiology images, or to look at how the AI can look at signaling, it can derive from analyzing blood samples, you can detect cancer learning. These are all areas where we see opportunity for the AI to make a very big difference in terms of pruning the outcomes of patients.
现在,这并不意味着它是完美的,它还有很多需要改进的地方等等。但在医疗领域,我们看到了令人惊异的进步。此外,当您考虑到诊断方面时,人工智能可以帮助诊断和分析放射学图像,或者从分析血液样本中获得信号的能力,您可以学习检测癌症。这些都是我们看到人工智能可以在患者治疗结果方面产生很大差异的领域。
And in the end, healthcare is one that everyone on the planet cares about, is becoming an increasingly unsustainable and unaffordable cost for even developing economies are still waiting for a decent healthcare. So I think the ability to have that be in population scale in fact is extraordinary. So healthcare is one broad area that you're very excited about. And there's many multiple dimensions to that.
说到最后,每个地球上的人都关心医疗保健,但医疗保健成本越来越不可持续,即使是一些发展中国家也难以承担,他们仍在等待体面的医疗保健。因此,我认为在人口规模上拥有这种能力实际上是非常不可思议的。所以医疗保健是一个广泛的领域,你对此感到非常兴奋,其中有许多多个方面。
What are other broad areas where you feel like there is near term potential for disruption with other events? I think that more than disruption, I'm excited about the augmentation possibility with education. I think anybody who's used any of these large algorithm model based chat tools has experienced this feeling of wonder of how well they do at explaining things. And you can regulate it up and down, explain it as if it was a phone. So by the old explainer, as if I was a college graduate. And the models will do a pretty good job of explaining abstract concepts in that way. And since these models are largely speaking red everything, they have the ability to teach you or anything. And so I think having the human teachers augmented with this AI, I think will open up extraordinary opportunities. Again, just the fact that everybody has a phone means that people can access a lot of this.
您认为还有哪些领域可能会在短期内发生颠覆性的事件?我认为,与其说颠覆性,我更期待教育的增强可能性。我认为,任何使用过任何基于大型算法模型的聊天工具的人都会有这种惊奇感,觉得它们在解释事物方面做得很好。而且你可以调节它的音量,将它解释得好像在打电话一样,就像老的解释者一样,好像我是一个大学毕业生。这些模型在解释抽象概念方面做得相当出色。由于这些模型大多已经读过所有内容,它们有能力教给你或其他任何东西。因此,我认为在此基础上与人类教师相结合,将会开辟出非凡的机遇。再次强调,事实上每个人都有一部手机,这意味着人们可以访问很多东西。
And you can just imagine the impact that's going to have in areas where you don't have access to people who know certain subjects or can teach to a certain level. This is that will be truly a democratizing force that will allow everyone to rise up. I think that's very well. I mean, that is actually something that's available today. It almost needs no new, there's no net new innovation required.
你可以想象这将对那些没有接触到某些主题或无法教授到某个水平的人们产生何种影响。这是确实将是一个民主化的力量,允许每个人都能提高自己。我认为这非常好。我指的是,这实际上是今天已经可以利用的东西。几乎不需要新的创新,没有必要进行全新的网络创新。
And the model's actually remarkably being able to teach people. That's exactly right. And I think that's right. I think the education process itself might have to change a little bit because some of the ways in which we measure progress for students will have to modify. Just in the same way that when capricators came around, you'd have to modify how they did certain exams to make sure that they were either used or not used, etc. I think that the education system is going to have to be modified to take that into account. But it's clearly a good thing that more people have access to high-quality education.
这款模型实际上非常适合教授人们。这点完全正确。我认为这一观点是正确的。我认为教育过程自身可能需要做出一些改变,因为我们对学生学习进度的评估方式将需要做出一些修改。就像当模拟程序出现时,你必须修改一些考试方式,以确保其使用或不使用等。我认为教育系统将需要进行修改,以考虑到这一点。但更多人都能够获得高质量的教育显然是一件好事。
If we move on beyond healthcare education, I think both very large sectors of the economy, both global sectors where you know, access to both is very unequal based on economic factors. I do think Ellen Anthony, a huge level playing field. If we move on beyond that to sort of enterprise is in the US or in developed part, it's thinking the global goes thousand.
如果我们超越医疗保健教育,我认为经济的两个非常大的领域,也是全球性的领域,在这些领域中,基于经济因素,访问这两个领域的平等性非常不平等。我认为艾伦·安东尼是一个非常公平的竞技场。如果我们超越这些领域,转向美国或发达地区的企业,它意味着全球化的价值观。
It's obviously very early days for most enterprises in terms of adopting an of them. But I've been surprised by how much interest there is. Do you see that as well in your role or through the lens with which you talk to large enterprises? Or is it near a sort of they have their head buried under the set right now? No, absolutely. I think we're seeing enormous interest.
对于大多数企业来说,采用它们还处于非常早期的阶段。但我很惊讶于有多少人感兴趣。在你的角色中或者是通过你与大型企业交流的视角,你是否也看到了这一点?或者说,他们现在是完全沉迷于现有的设置中,无法抬起头来看周围的变化?不,完全不是。我认为我们正在看到巨大的兴趣。
And I think that what we are thinking about is there's three levels at which enterprises can deal with or interact with and benefit from AI right now.
我认为我们现在考虑的是企业可以通过三个层面来应对、与人工智能互动并受益。
The first I think is just simply to adopt the tools that have been created by Microsoft and other companies that allow everyday processes to be just done better.
我认为首先要做的就是采纳微软和其他公司开发的工具,这些工具可以让日常流程变得更加高效。
So just how you can start to email or how you start a document, even how you have a meeting on a video call.
那么,您要如何开始发送电子邮件或创建文档,甚至如何在视频通话中进行会议呢?
Can all be made better now through the summarization or the created aspects of AI? And that will make a very big difference to how employees are able to adopt their daily roles.
现在通过人工智能的总结或创建方面,是否可以使一切变得更好?这将极大地改变员工如何适应他们的日常角色。
A great example there is, is, get on code by an obviously is something which was released about a year ago.
有一个很好的例子,就是“get on code”,显然是一年前发布的东西。
意思是说,“get on code”是一个在一年前发布的项目,是一个很好的例子。
It's called progress through better and better.
这被称为不断进步。
It's not getting to the point where we have metrics that show that for coding activities it's able to speed and do not half the coding that professional coders will do on a daily basis.
我们还没有取得这样的指标,即针对编码活动,能够加速并且不会比专业编码人员每天完成的编码量少一半。
And what that does is more than anything it improves the job satisfaction for others.
这样做最重要的是提高别人的工作满意度。
It takes away the work work and it takes away a lot of the stuff that is, you know, just depending on the aspect.
它消除了重复的工作,也消除了很多依赖于方面的东西。
Yeah, because the truth of the matter is while coding it's a great profession.
是的,事实上编码是一份非常好的职业。
There's a decent chunk of it which is repetitive and kind of just not that interesting.
其中有相当一部分是重复的,而且并不是那么有趣。
And so by having the AI be able to take care of that people are able to spend their time on the parts that they enjoy the most.
因此,通过使人工智能能够照顾那些让人们最喜欢的部分,人们可以花更多的时间在自己最喜欢的事情上。
And so you can think about being replicated across all the whole range of roles in an enterprise.
你可以想象自己被复制到企业中所有角色中。用于表达跨角色的复制。
And that I think should be priority number one for pretty much every enterprise because it's just almost an overgrace type of move to do that and allow productivity to flourish as a consequence.
我认为这几乎应该是每家企业的首要任务,因为这样做就像是一种过度宽容的行动,可以让生产力得以蓬勃发展。
So that's number one. Then the second thing that people can do is to develop their own AI based applications which are useful for their own enterprise.
因此,第一件事情是那样的。第二件事,人们可以做的是开发适用于自己企业的基于人工智能的应用程序。
This is where we should expect to see a lot of citizen developers or semi professional developers in their company having their creativity completely unleashed as AI actually reduces the barriers to being able to create applications.
这就是我们应该期望看到公司中有许多公民开发者或半专业开发者,他们的创造力在人工智能的帮助下完全得以释放,因为 AI 实际上降低了创建应用程序的障碍。
So you no longer need to be an expert in programming in C to be able to create Java or whatever it is to be able to go and create a website.
现在,您不再需要是C编程专家,就可以创建Java或其他网站。
You know, just happy when to be able to type into a prompt box and stuff will happen.
你知道的,当能够在提示框中输入内容时,就会感到很开心,这样一些事情就会发生。
And that will I think unleash another wave of productivity within companies.
我认为这将会在公司内释放出另一波生产力。
And then the third is actually just baking in AI into the core product itself which depending on the industry you're in has more or less applicability.
第三个是将人工智能直接嵌入核心产品中,具体适用程度取决于所处的行业。
If you're making washing machines at bridges, only a bit less because the core product is still a physical item that has to do certain things.
如果你在桥上生产洗衣机,只不过少了一点,因为核心产品仍然是必须完成某些任务的物理产品。
这句话的意思是,如果你的核心产品是物理产品,无论你生产它的地方在哪里,它仍然需要完成某些特定的功能,所以在生产洗衣机时并没有太大区别。
If you've got a product which is more software based or more services based potentially quite a lot of benefit could be driven by Intusio value proposition by making an AI.
如果你的产品更多是基于软件或服务的,通过将其变成人工智能,Intusio的价值主张可能会带来相当丰富的好处。
And that's where I think we're going to see a need for people who know how to infuse AI into these applications of the big demand for that type of talent.
我认为这就是我们将看到需求的地方,需要知道如何将人工智能融入这些应用程序中的人才。这种类型的人才将会大量需求。
And across the board, you know, clearly, you know, platform companies like Microsoft, Google, and others will play a role.
从各个方面来看,显然,Microsoft、Google和其他平台公司将发挥作用。
这句话的意思是,不管从哪个角度看,微软、谷歌以及其他平台公司都会对局面产生影响。
Existing application providers in comments like Fox, Workday, SAP, and others will play a role.
现有评论中的应用程序提供商,如Fox、Workday、SAP等,将会发挥作用。
意思:已经存在的应用程序提供商,如Fox、Workday、SAP等,在这个领域起到重要作用。
But there will also be opportunities that are more suited to startups.
但也会有更适合初创企业的机会。这意味着初创企业可以发现并利用这些机会来获得成功。
You know, just given that you've been involved with so many startups yourself, where do you see startup opportunities in this whole landscape?
你知道的,鉴于你自己已经参与了这么多创业公司,你认为在整个行业中有哪些创业机会?
Well, first of all, I very much hope there's going to continue to be vibrant opportunities to start on because that's where a lot of the innovation occurs in the first place.
首先,我非常希望未来还会有充满活力的创业机会,因为那是创新发生的地方。
I think that we're in a very fluid and dynamic phase of the development right at the start of this.
我认为我们正处于一个非常流动和动态的发展阶段,就在这个阶段的开头。
And so if you take the sort of three layers that you get, or maybe there's four layers, there's applications and there's a platform, then there's models, and then there's the infrastructure itself.
所以,如果您考虑到一些层次,或许有三个或四个层次,包括应用程序和平台层、模型层以及基础设施本身。
So I think, to a large extent, the infrastructure is where there are established players already.
我认为,基础设施方面已经有已经确立的玩家存在,这在很大程度上是如此。
There's a range of established players, and I think that's kind of that.
这里有一些已经成立的参与者,我认为就是这样了。
意思:已经有一些成熟的参与者,目前没有更多新的加入。
When you go to the models, there's a whole bunch of people who are doing interesting stuff there, all the way from the large, largest models being trained with the largest civic computers to people who are finding ways to shoe on those things onto edge silicon and getting a much smaller footprints.
当你去看模型时,那里有一群正在做有趣事情的人。他们从使用最大的市政计算机训练最大的模型,到找到将这些东西放到边缘硅片上并获得更小足迹的方法,涵盖了各种各样的领域。
So that's a vibrant area.
那里是一个充满活力的地区。
We will see how the landscape evolves in terms of which models are applicable for which type of applications.
我们将看到在应用什么模型方面,景观将如何演变,以适用于不同类型的应用场景。
The platform area is one where there's an incredible amount of innovation, as I'm sure you see on a daily basis, a solid foundation.
平台领域是一个非常创新的领域,就像你每天所看到的那样,它拥有一个扎实的基础。
And there, the extraordinary thing that we're seeing right now is just things get developed, put out there on GitHub or wherever.
现在我们看到的非同寻常的事情是,代码被开发出来,然后放在GitHub或其他地方。
And with sort of minimal marketing, and sometimes not even yet formed as a company, you'll see vertical takeoff in terms of application.
即使没有做过多的营销,并且有时甚至不是一家正式的公司,也会看到应用垂直起飞的现象。
I often joke that these days, vertical is the new up until the right. And in the platform, you've just got such an enormous amount of activity right now, that it's fantastic to see the innovation. Not to know exactly how it's going to shake out until about a bit later, but I think there's a lot of energy going into that space, which is great. And then of course, there's the applications themselves and of course there's an equal lot of energy going on there. And there, I think, you know, it's going to vary a lot in terms of which areas are going to be fruitful and which areas are going to end up being more quantitized. Ultimately, I suspect that a lot of it will still come down to some basics, despite how exciting and how radical AI is. I think that there's going to be some, you know, usual basics, which will make a very big difference.
我经常开玩笑说,在现在这个时代,垂直排列已经取代了向右的方向。在这个平台上,目前有着如此巨大的活动量,非常令人振奋地看到了创新。虽然到后来会有些变化,但我认为现在有很多能量注入这个领域,这非常棒。当然,还有应用本身,而且在那里也有同样的能量。在这方面,我认为结果会有很大的差异,一些领域会非常有前途,而一些则会变得更加定量化。最终,我认为,尽管 AI 很激动人心和激进,但很多基础知识仍然是至关重要的。
And in this case, I think distribution is going to be a pretty important prerequisite to success. Distribution allows you to not only get out there, but also to start improving the application given that the feedback is actually an important determinant of success in AI-based apps. So I think that, you know, looking beyond all of the glamour, you know, basic distribution will, I think, turn out to be a pretty important aspect for us to start up to consider as they enter in the application space.
在这种情况下,我认为分发将是成功的重要先决条件。分发不仅允许你进入市场,还可以通过反馈来开始改善应用程序,因为反馈实际上是基于人工智能应用程序成功的重要因素。因此,我认为,除了所有的魅力之外,基本的分发将成为我们在进入应用程序领域时要考虑的一个非常重要的方面。
Now, I think that's a great framework to sort of think about it. So let's go to each of those layers, if you don't mind in a little more detail. On the applications side, you know, in some ways, the rules of the game haven't changed. I think what LLM and AI will broadly has done is create opportunities for a whole new class of applications. As you said, you could have a co-pilot for marketers, you could have a co-pilot for AEs, you could have a co-pilot for salespeople, you could have a co-pilot for physicians. I think there'll be a whole range of applications that get created. In that genre, there'll be applications that are broader.
我认为这是一个很好的框架,可以更加深入地思考一下。现在,让我们更详细地看一下每个层面。在应用方面,某种程度上来说,游戏规则并没有改变。我认为,LLM和AI的广泛使用为一整个新型应用程序类别创造了机会。就像你所说的那样,你可以为市场营销人员、AE、销售人员和医生创建副驾驶员。我相信会有很多不同的应用程序被创造出来,并且有一些应用程序的面向会更广。
You know, you can imagine applications that enable you to sort of impress the human time involved in processing insurance claims, processing healthcare claims. I think there's going to be a Cambrian explosion of application companies because this technology allows you to do some things that you couldn't have done before in the cloud with applications. And as you rightly said, I think distribution will be an important competitive advantage. But as will the ability to really understand the pain point and to define a product that you can get early adoption because the technology itself, you know, I'm sure there'll be elements of technology that matter.
你知道,你可以想象出一些应用程序,使你能够减少人类在处理保险索赔、医疗保险索赔方面所需的时间。我认为,这项技术将会让应用程序公司出现一次深刻的进化,因为它使你能够在云中做一些以前无法完成的事情。正如你所说,我认为分销将是一个重要的竞争优势。但是,真正理解痛点并定义一个可以早期采用的产品也将是成功的关键,因为这项技术本身,我相信会有技术上的因素起作用。
But most application companies are going to use third-party platforms, models, and infrastructure. Is that how you think about it or are there other factors that application companies should be thinking about? Well, first of all, I think I'd really agree with you. In fact, I also use the phrase Cambrian explosion. And I think we're seeing it happening right now with an enormous flowering of ideas and innovation, which are based on the fact that there are standard models of platforms available. People are able to knock things together very, very quickly and get them out of there, which I think is fantastic.
但大多数应用公司都将使用第三方平台、模型和基础设施。您是否也这样认为,或者应用公司应该考虑其他因素呢?首先,我非常赞同您的观点。实际上,我也使用过“寒武纪大爆发”的说法。我认为,我们目前正处于这种大爆发的时期,创新和想法在不断涌现,这将基于标准平台模型。人们能够非常迅速地组合各种元素并将其推出,我认为这是很棒的。
The thing that you said is, I just want to kind of emphasize how important that is, which is getting adoption is going to be as important as it ever was. And in this case, the technology of the speed of writing applications is going to far outpace the ability for people to change their behavior if that's what they need to do to adopt. And I think thinking really hard about how the application could be easily entering to the existing behavioral patterns is actually a pretty important thing.
你刚刚说的是,我想强调的是采纳(新技术)的重要性将会像以往一样重要。在这种情况下,编写应用程序的速度远远超出了人们改变其行为的能力(如果这正是他们需要采用的方式)。因此,我们需要认真考虑如何使应用程序容易地融入现有的行为模式,这实际上是非常重要的事情。
So that, for example, if COVID hadn't happened, video conferencing was on a penetration trajectory. It was interesting. But COVID happened and it just went through an enormous tech change. So now, video conferencing is something that is there in many people's lives on a daily basis. So it's easier to introduce AI features into video conferencing and know that it will be adopted because you don't have to rely on someone doing something they weren't doing before. If people were not doing video conferencing and you introduced AI into that, they'd have to first of all get used to video conferencing before they could have been able to do the AI.
因此,比如说,如果没有COVID疫情的发生,视频会议仍在渗透轨迹上发展,这是很有意思的。但COVID的发生引发了巨大的技术变革。现在,视频会议已成为许多人日常生活中的一部分。因此,将人工智能功能引入视频会议中变得更容易,并且知道它将被采用,因为你不需要依赖某些人去做以前没有做的事情。如果人们没有进行视频会议,并且你将人工智能引入其中,他们首先必须习惯视频会议,然后才能使用人工智能。
And in the same way, I think we see the same thing when it comes to helping physicians, have tried and true ways of doing things and for good reason because they know that it works. And so getting a physician to benefit from AI, you have to think hard about making sure it's easily adopted in their natural workflow because they're not going to change their workflow.
同样地,当涉及到帮助医生时,我认为我们看到的也是同样的情况——他们有一些经过验证和行之有效的方法,而且这些方法也有很好的理由,因为他们知道这些方法是有效的。所以,如果要让医生从人工智能中受益,就必须认真考虑如何确保它容易地融入到他们的自然工作流中,因为他们不会改变他们的工作流程。
Absolutely. It's the same for sales people, it's the same for finance people. It's the same for everybody in the end. It has to be in your existing workflow very close to it in terms of your existing behavioral pattern to be able to get adoption. I think that's well said.
当然。销售人员、财务人员以及其他人最终都一样。这一点非常重要,因为只有与你现有的工作流程非常相似,才能够被广泛采用。我认为这说得很好。
As you were talking about video conferencing, I was reminded of the fact that one of the areas that's changed dramatically over the last three years, post-COVID has been sales. Most sales used to be, we always talk about sales as being a contact sport and face to face. And I think we've gone from that to probably 60, 70% of sales happening over video conferencing, team, zoom, and otherwise. And I think that's a huge opportunity for AI because there's data that you've been extracting, signal from you create a poll pilot for a salesperson or a Zoom call. It's very different from someone who's in base-to-base meeting, just as an example.
当你谈到视频会议时,我想起了一个事实,那就是在COVID后的最近三年中,销售领域发生了巨大的变化。大多数销售以前总是面对面的,我们总是把销售称为一项“接触运动”。而我认为我们从那时起已经转向了大约60%至70%的销售通过视频会议、团队、Zoom等进行。我认为这是人工智能的巨大机会,因为可以从你提取的数据和信号来为销售员或Zoom会议创建一个实验版本。这与面对面会议的人是非常不同的,这只是一个例子。
I completely agree with you. And I think that when you go through each of the function sales is a great one because of course there is still a base for burning the shoot at the moment, but a lot of it is now done through inside sales or what's through the remote. And that's a pattern that can very easily be influenced through AI or impacted through AI. And I think that you go through function by function, you'll find it's not the whole function, but there'll be elements of what people do during their day, which can very easily be prevented from the usage of AI. So that's the application layer.
我完全同意你的观点。我认为,在销售职能中,每个方面都很棒,因为当然现在仍有一定的销售基础,但很多都是通过内部销售或远程销售完成的。这是一个非常容易被AI影响或受到AI影响的模式。而我认为,你可以逐个职能地进行审视,你会发现不是整个职能都可以通过AI来解决,但工作中的某些元素很容易通过AI的使用来优化。所以这就是应用层。
But before we move on to platforms, are there other categories we got healthcare, education, and we talked about sales, anything else that Staddog for you is low-hanging fruit?
在我们转向平台之前,我们还有其他类别,例如医疗保健、教育,以及我们谈到的销售,Staddog,你觉得还有其他低风险的机会吗?
I think that the actual act of programming itself is the obvious one to mention here. We talked about earlier with GitHub Code Violet. Yep. But the changes that are happening there are just so rapid and fantastic. The combination of a Code Violet together with the capability of being your chat GPT, I've had even very, very experienced and renowned engineers saying that it's a magical experience when you do that because people find it liberating. It just helps them to stay in the flow longer and they're able to more create it as a result. Nice to hear about that.
我认为,在这里明显要提到的是编程本身的实际操作。我们之前已经谈过了GitHub Code Violet。没错,但那里正在发生的变化是如此快速和神奇。Code Violet与GPT聊天功能的结合,甚至经验丰富、声名显赫的工程师都说这是一种神奇的体验,因为人们会感到解放,能够更长时间地保持流畅状态并因此更好地创造出作品。听到这样的事情很不错。
When you see that joy from, let's face it, the average programmer is not generally the sort of person who's going to exhibit a whole pile of joy on a voluntary basis. But when you see that joy coming from that particular group of professionals, it really means something. I think that's well said.
当你看到那种喜悦时,说实话,普通程序员通常不是那种会自愿表现出很多喜悦的人。但当你看到那个专业团队中传递出来的喜悦,这真的是意味深长的。我认为这话说得很好。
In many ways, programming languages are the most structured form of communication. For the last 30, 40 years, we've seen increasing levels of abstraction. At some level, the ultimate form of abstraction or transform of based models are just the ultimate form of abstraction. There's a lot of structure needed that already exists to enable you to train the models on top of that. There is this meme that's going around that the next new programming language is the English language, which I don't know if in the end, that's actually going to be the case that the ping is opposed to the regular languages programming. But it's clear now that natural language being the English or branch or whatever, you don't need to be in the English. Is a pretty important mechanism also getting some tasks done as well?
从多个方面来看,编程语言是最具结构化的通信形式。过去30-40年间,我们已经看到了越来越多的抽象层次。在某种程度上,基于模型的终极抽象或转换只是抽象的终极形式。已经存在着很多需要的结构,使得你可以在其上训练这些模型。有一种流行的观点是,下一个新编程语言将是英语,我不知道最终是否会出现这种情况,因为这与常规语言编程相反。但现在很清楚,自然语言,包括英语或其他语言,在完成某些任务时也是一个非常重要的机制。
Let's go on to the next layer, the platform layer. Can you unpack that a little bit? When you think about the platform layer, what is going on there? What are some of the interesting trends you're seeing?
让我们进入下一层,平台层。你能稍微解释一下吗?当你思考平台层时,发生了什么?你看到了哪些有趣的趋势?
I think it's a massive jump ball at this point, Ashu. I think that what we're seeing is even the way in which people are developing applications is evolving. Retrieval augmented generation is now becoming the kind of approach that a lot of people are using. Fine tuning was another approach that was being used. Maybe it's being replaced by RAID. It's kind of hard to know exactly how it's all going to evolve. I'd say that the thing that's happening is that people are very rapidly responding to signal that they're seeing. It's important to have a vector database so people are developing that. It's important to have long-term memories so people are developing that. I think that we're seeing this emerging as we speak as people are trying to evolve the best ways to respond to the signal they're seeing from application of their actual driving growth and take-on. It's hard to put a finger on it to be perfectly honest with you.
我认为目前这是一个巨大的跳跃球,Ashu。我认为我们正在看到的是人们开发应用程序的方式正在发生变化。检索增强生成现在成为了许多人使用的方法。微调是另一种方法,也被使用过。也许它正在被RAID所取代。很难知道它们将如何演变。我认为正在发生的事情是人们对他们看到的信号做出了非常迅速的反应。拥有向量数据库非常重要,人们正在开发它。拥有长期记忆也很重要,人们正在开发它。我认为我们正在看到这种新兴趋势的发展,因为人们正在尝试进化最好的方式来响应应用程序实际驱动的增长和发展。说实话,很难具体说明它将会如何发展。
Even from an investment perspective, I'd say it must be very difficult for you because you're seeing these incredibly spiky signals of something that didn't exist last weekend. There's now suddenly spiked up out of nowhere and a lot of people are using it. Then two weeks later, maybe that's actually no longer relevant because some other approach is found to be superior. That is no longer that obvious the need for that being in the first place. It's a really chaotic but creative moment as well, I'd say. I think you're smart on it. It's both been exciting but also very frustrating to watch.
从投资的角度来看,我想你一定很难,因为你看到的是这些非常尖锐的信号,而它们上周末还不存在。现在忽然冒出来并有很多人在使用。然后两个星期后,也许这个东西已经不再相关了,因为已经找到了一种更好的方法。这也表明一开始并不明显需要这个东西。我认为这是一个非常混乱但又充满创意的时刻。我认为你很聪明。看着这一切既让人兴奋又让人沮丧。
We've seen the explosion in vector databases. I was on a call earlier today with a Cedar Executive at AWS and their view was that, look, a vector databases, it's really just a search index with some specialized code and every hyperscaler has search indexes available and we'll just offer that. Like his view was, look, there's no way there'll be a standalone vector database six months or a year from now. In the fact that buying phone has five million revenues up from two million two weeks ago is completely relevant. Just take one example. Similarly, we've seen tons of activity around things, open source projects like Langt chain and others and it's really hard to tell which one of these is transient and which one would be subsumed actually by the underlying infrastructure provider itself. What will become a part of the infrastructure whether it's Microsoft or Google or the models as an API companies like OpenAI? We've been mostly, we think model observability is probably an enduring position to be in and you and I have talked about a rise in that category. So we definitely think that category stays, but a lot of the other stuff is just hard to tell what is enduring versus transient right now.
我们已经见证了向量数据库的爆炸式增长。今天早些时候,我与亚马逊 AWS 的一位高管进行了通话,他的观点是,向量数据库实际上只是一个带有一些专门代码的搜索索引,每个 hyperscaler 都可以提供搜索索引,我们也将提供。他认为,六个月或一年后,不会有一个独立的向量数据库。而现在的情况是,小米买手机的收入从两周前的两百万增加到了五百万元,这个例子完全具有参考价值。同样,我们也看到了像 Langt Chain 等开源项目的大量活动,现在很难确定其中哪些是短暂的,哪些会被基础架构提供商所吸收。将成为基础架构的一部分,无论是微软还是谷歌,还是像 OpenAI 这样的模型作为 API 的公司?我们认为,模型的可观察性可能是一个持久的位置,我们已经讨论过这个类别的崛起。所以,我们确信这个类别会存在下去,但其他的东西现在很难确定哪些是持久的,哪些是短暂的。
Completely agree. I mean, if you think back to the rise of the internet in the late 90s, there was a lot of chaotic innovation going on as well. But one thing that we are benefited from was the layered model of networking was relatively established both in theory and also in practice in terms of people that build networks. So while there was a lot of jockeying in the position, I don't think there was a whole host of arguing around that layer, the layers because that was kind of well known and everyone kind of competed within their layers and tried to vacationly cross a layer or two, but there was no debate about what was the layer. I think the reason why it's interesting now is because there's no defined equivalent of what the architecture should really look like and exactly what kind of workflow there should be for developers to developing AI based applications. So that is why we've got this enormous amount of flux right now.
完全同意。我的意思是,如果你回想一下90年代末互联网的崛起,当时也有很多混沌的创新发生。但我们受益的一件事就是网络分层模型在理论和实践的建造人员方面都比较成熟。因此,尽管在位置竞争方面存在很多争吵,但我认为没有人争论层次,因为这本身就是众所周知的,每个人都在自己的层次中竞争,并尝试跨越一个或两个层次,但没有争论哪种是层次。我认为现在这个领域很有趣的原因是,没有确切的等价物来确定应该真正是什么架构以及开发基于人工智能的应用程序的工作流程。因此,我们现在面临着极大的变化。
Are there, you know, without getting into specific companies, are there one or two problem spaces within the platform layer that you think are enduring? I actually find that really hard to answer actually because one of the things is that it's still unclear to me exactly how the whole space of models is going to evolve when I was mentioning earlier that you've got everything all the way from these very large models trade on supercomputers down to models which are squeezed into to pitch. What may end up happening is just the combination that's required for any application may we change over time. There's one world view which says you just use the largest of the large models all the time and even if they're not using using it to its full maximum, that's going to be the most cost effective thing. There is another world view which says actually you should do everything that you can on the edge and only spike up into the burst into the powder you need to. Then there's other arguments which are actually maybe what you need is actually a cost of these things together to be able to really break through and make your application work well. Much like the human brain has very different elements to it. You know, maybe all those things need to be stitched together. So that's one of the reasons why I'm finding it hard to answer this question because we're obviously thinking about it too. So I think that there's going to be a platform that's going to be useful for the next little while. But then as the models themselves evolve, I think we're going to learn a lot now. Maybe the platform that was useful in 2023 and maybe 2034 becomes less relevant in 25 and 26. I just don't know. It may be that it induced. Maybe that it done.
在不涉及具体公司的情况下,你知道在平台层面上是否有一两个持续存在的问题领域吗?实际上我觉得这个问题很难回答,因为有一件事情还不太清楚,那就是模型空间的整个发展会如何变化。我之前提到的,从那些在超级计算机上交易的非常庞大的模型,到被压缩到鸭蛋大小的模型,各种模型都有。但最终的应用所需要的组合可能会随时间而变化。有一种观点认为,你应该始终使用最庞大的模型,即使它们未充分利用,这也将是最具成本效益的选择。另有一种观点认为,你应该尽可能地在边缘上处理所有事情,只在必要时进行大规模处理。还有一些争论认为,可能需要将这些模型成本结合起来才能真正突破,并使应用程序运行良好。就像人脑有很多不同的元素一样,或许所有这些元素都需要被缝合在一起。这就是我发现回答这个问题很困难的原因之一,因为我们显然也在考虑这个问题。所以我认为未来还会有一款有用的平台。但随着模型本身的发展,我们将学到很多东西。也许在2023年和2034年有用的平台,在2025年和2026年变得不再相关了。我不知道,这可能是持续的,也可能是短暂的。
That's well said. And I think as you rightly said, I think a lot of this world depends on how the ecosystem models and what is the, what are the different deployment models for models? Like do you, you know, do you use, as you said, do you use one large model, do you use multiple models? To what extent do you use open source models that you trade yourself at the against using foundation models as an API? What are you seeing in that, you know, it's gone.
这说得很好。我认为正如你所说的,世界上很多东西都取决于生态系统的建模方式以及模型的不同部署方式。比如,你会采用巨型模型还是多个独立模型?你会使用自己开发的开源模型,还是使用基于API的基础模型?你目前看到的情况是什么?
It's been pretty wild. How much again, there's almost, I'm not sure if I would use a word came to an explosion, but there have been an explosion of activity in terms of people releasing models of their own, whether it's dolling from data bricks or folks like Alpaca from, you know, it seemed like they have, you know, used GPT, either train or GPT equivalent or a competitor.
这一切相当疯狂。在发布自己的模型方面,活动如此之多,几乎可以说是爆炸式的,无论是来自Data Bricks的Dolling,还是像Alpaca这样的人们,似乎他们都使用了GPT或其等效或竞争对手进行训练。
There just seems a lot of, a lot of activity in the modern space as well is your sense that that space will be fragmented over time or will we see consolidation or just too early to tell? I suspect we're going to see some of the activity continuing, but that eventually will die down a bit, not completely it will die down a bit for the following reason, which is that ultimately I think it's quite important to make sure that these models don't lose to me.
现代领域似乎有很多活动,您认为这个领域会随着时间的推移分裂还是会出现合并,或者现在还太早得无法判断?我猜测我们将看到一些活动仍将持续进行,但最终会稍微减少一些,而不是完全停止,原因如下:我认为最重要的是确保这些模型不会失去对我来说的重要性。
And I think that it's hard to know how some of these smaller models and some of the models which are sort of retrofits of larger models can avoid that hallucination problem. So I think that once we think about the objective in terms of what we're going to achieve, which is useful models that are as inexpensive as possible, as really applicable as possible, and could be grounded in data to make sure they hallucinate the least. I think once you kind of start applying those parameters, I think the number of models that can actually do that for a given application has got to probably go down is what I'd say.
我认为很难知道这些较小的模型和基于较大模型的改装模型如何避免幻觉问题。因此,我认为一旦我们从我们想要实现的目标角度考虑,即尽可能成本低廉、尽可能适用、并能以数据为基础确保尽量减少幻觉的有用模型,一旦您开始应用这些参数,我认为能够实现这一目标的模型数量可能会下降。
And has that caused challenges that result in concentrating of power amongst, you know, do these large elements become the next new operating systems? I don't know about operating systems, but you can almost even think about them as semi-conductive modes or most, that you have leading nodes that are useful for the most challenging type of problems and applications. And as they age and new nodes emerged, these nodes have come in minus one and minus two and minus three, which are made available for a broader range of things. And so I think that we can almost think about it that way. And once it dealing with it, n minus two and minus three model, which only a few years ago might have been the model that was advanced and able to do great things. You can see that being delivered through a greater number of mechanisms and people and companies. So it may just evolve in a slightly different way.
这是否导致了让一些大元素集中掌握了权力,成为下一个新的操作系统?我不确定是否会成为操作系统,但是可以把它们看作半导体模式或节点,在最具挑战性的问题和应用中非常有用。随着它们的老化和新节点的出现,这些节点变得越来越多,推出了n-1、n-2和n-3等更多用途的节点。因此,我认为我们可以这样想。在处理n-2和n-3模型时,只有几年前可能是先进的模型,可以做出伟大的事情。现在可以通过更多的机制、人员和公司进行推广,这可能只是以稍微不同的方式演变。
I don't think it's always just going to be that there's one massive model that moves a lot from one provider. But I've never thought of models in the context of semi-conductive nodes, but the analogy makes perfect sense. Just as you're trying to release the next GPU or GPU, you do need to let us know it. But for most A6, you're okay with n minus one and minus two and some cases of n minus three. That's right. There's going to be applications for which an n minus model will be good enough.
我认为不会总是只有一个大型模型从一个提供者转移很多。但我从未考虑过在半导体节点的环境下有哪些模型,但这个比喻确实很有道理。就像你正在尝试发布下一个GPU或GPU一样,你确实需要让我们知道它。但对于大多数A6来说,你可以接受n减一,减二和减三的情况。是的,会有一些应用程序可以使用n减模型。
What advice would you have for start? Let me give you your own experience. Both as an investor and as a series of founder. Across these four layers of the stack, what would you advise founders to do? And of course, different founders would do different things. That's the beauty of the start of ecosystem. But are there any common themes or questions? Or what advice do you have at the highest level?
你会给初创企业有什么建议呢?让我来分享你自己的经验。无论是作为投资者还是创始人的角色,你可以从整个创业生态系统的四个层面来提出建议。当然,不同的创始人可能会做不同的事情,这也是创业生态系统的美妙之处。但是是否存在共同的主题或问题呢?或者你在最高层面上有什么建议呢?
I mean, at the most abstract level, what I'd say is that it's more important than ever to find a durable problem. A problem that is going to be durable to the progress of the models themselves. And as the ability, if you solve it, to really be able to have the impact they expect it to have. So if you're talking about at the application level, I think the durable problems are going to be the ones which are reliant on having some form of data source that is hard to get. Because other kinds of problems may get solved more quickly than those sorts of problems tend to, I think, endure a bit longer.
我的意思是,在最抽象的层面上,我认为比以往任何时候都更重要的是找到一个持久的问题。一个问题,它将持久地存在于模型自身的进步中。如果你解决了这个问题,它就能真正发挥他们期望的影响。所以,如果你在谈论应用层面上的问题,我认为持久的问题将取决于是否有某种难以获得的数据来源。因为其他类型的问题可能会更快地得到解决,而这些类型的问题往往会持续更长的时间。
More difficult in the platform there as we talk about. I think that you told your own observability. I think that's a great one. I would say that in general, all of the capabilities that you do with ensuring compliance of the model and prediction, go to the model of privacy, all of those areas, I think, are definitely going to endure for a very long time. So I'd say it's important to make sure you have a view of why you think your problem with your solving is going to endure a lump and up for you to actually see your company growing to scale.
在我们谈论的平台上更加困难。我认为你讲了你自己的可观察性,我认为这是很好的。我会说一般来说,您所做的确保模型和预测符合合规性的所有能力、隐私模型以及所有这些领域,我认为都肯定会持续很长时间。因此,我认为重要的是要确保您对为什么您认为您要解决的问题将持续很长时间有一个清晰的观点,并且您实际上可以看到您的公司增长到规模。
The whole conversation so far, Bobby has been about LNM's. And in the context of the hype in the last six months, it would seem that LNM have subsumed all other variants or flavors of AI. And this is it.
到目前为止,整个对话都是关于LNM的。在过去六个月的炒作下,LNM似乎已经吞并了所有其他AI的变种或风味。这就是此时此刻的情况。
And there are people who would argue that LNM's will sort of solve all kinds of problems, including what they don't solve today. I had a conversation with someone who said, well, LNM will solve partial differential equations in the coming years, which is not a class of problems they've been solved today. So that's one extreme. They say, hey, all AI research or all models will essentially become will be subsumed by LNM's.
有人会认为,LNM将解决各种问题,包括它今天不能解决的问题。我与一位人士进行了谈话,他说,在未来几年中,LNM将解决偏微分方程这一类问题,而这些问题今天还未得到解决。这是其中的一个极端观点。他们说,嘿,所有的AI研究或所有的模型都将基本上被LNM所替代。
And then there's an argument to be made that looked as one branch in the tree and there'll be other equally important branches that are sort of less developed today or a more in research that in virtualization and could be as important or perhaps even more in order to overcome.
还有一个论点是,虽然我们现在只看到了树上的一条枝干,但可能还有其他同等重要的枝干,它们目前的发展程度比较低或者还在研究中,例如虚拟化技术,它们也可能非常重要,甚至更重要,以便跨越更大的障碍。
Just give it your vantage point where you sort of both see the commercial world and sort of have a lens into Microsoft research. What's your take on this debate?
请尽量明白地表达您的看法,考虑商业世界和微软研究,您的观点是什么?您对这场辩论有何看法?
I think that LNM's is not the final answer. I think it's a remarkable capability and technology that's still got a long, long way to go. It is basically in some ways the first attempt of something that could be classified as an intelligence.
我认为LNM的技术还没有达到最终答案。我认为这是一项非常出色的能力和技术,仍有很长的路要走。从某些方面来说,它基本上是一个可以被归类为智能的东西的第一次尝试。
The concept of what is an intelligence is completely ill-defined and on note. But LNM's exhibit behaviors that would previously be classified as being intelligent. But it's not the final answer by any means.
智能的概念完全没有明确的定义和规范。然而,LNM表现出了以前可能被归类为智能的行为。但这并不是最终的答案。
I'll give you just a simple example, say your own son, which is that you mentioned that LNM's will be able to solve partial differential equations. Well, in actual fact, many problems that are currently only solved through PDEs are actually solved with all through LNM's protein-following, etc. And so you kind of see the path to that. Interesting. I did realize that. Yeah, that's right. And you see that at the same time, there's a decent chance of you putting a simple summation equation into the chat. It'll go wrong. So this is the same tool doing very sophisticated thing, getting it remarkably right and doing remarkably badly on something incredibly simple.
我给你举一个简单的例子。比如说,你提到了LNM可以解决偏微分方程的问题,而事实上,许多目前仅能通过PDE解决的问题,实际上都可以通过LNM,如蛋白跟随等方式来解决。所以你可以看到它的应用路径。这很有趣,我确实意识到了。是的,你同时也会发现,你在聊天框输入一个简单的求和方程,它可能会出错。所以这个工具可以做非常复杂的事情,做得非常好,并且在一些非常简单的事情上做得非常糟糕。
But it is, if you had classical or prior definitions of intelligence, there are some aspects of the PDEs that look like it's passed that test. Does it mean it's intelligent? No, it just means it's a capability that exhibits some aspects of what we might call intelligence.
如果你曾经有过经典的或先前定义的智能概念,那么PDEs中的某些方面看起来似乎已经通过了这个测试。但这是否意味着它是智能的呢?不,它只是展示了一些我们可能称之为智能的方面的能力。
Now, are there other systems that are going to be developed over time that will also exhibit certain aspects of what we call intelligence? Probably. I wouldn't say almost certainly. And so I think that while we're very focused on the LNM's right now, something else will show up. That's just the nature of innovation.
现在,会不会有其他系统随着时间的推移也展现出我们所谓的智能的某些方面呢?很可能会。我不会说几乎肯定。所以我认为,虽然我们现在非常关注LNM,但其他东西也会出现。这就是创新的本质。
But we've still got a long way to go with LNM's in terms of the capability and what they could do. But I do think that it's important to continue to recognize that what actually is intelligence, what defines consciousness, what defines being a human. These are really completely unknown concepts.
我们在LNM技术的能力和潜力方面仍有很长的路要走。但我认为继续认识到智能的本质、意识的定义、人类存在的定义是非常重要的。这些概念目前仍然是未知的。
And maybe one of the interesting, sort of unintended consequences of people looking at these models and seeing how they work and how they behave is actually might drive a better understanding of those core questions about the human species.
也许会有一个有趣的、意外的结果,就是人们看到这些模型的运作和行为,可能会推动更好地理解人类物种的核心问题。
Fascinating. Definitely a fun time, both in technology and in AI more broadly. It's the most incredible time I do not recall anything like this in my career, as I said. It's just amazing every day as an adventure.
非常令人着迷。在技术和人工智能领域中,确实是非常有趣的时代。就像我之前说过的,我在职业生涯中从未经历过这样的时期。每一天都是惊奇的冒险。
That's our show for now. You can find past episodes and subscribe to future ones. Whatever you get your podcasts, or at foundationcapital.com. And if you like the program, please leave us a review on Apple Podcasts. It really helps others to find the show.
这是我们现在的节目。您可以在过去的剧集中找到和订阅未来的剧集。无论您在哪里获取播客,或在 foundationcapital.com 上获取。如果您喜欢这个节目,请在 Apple Podcasts 上给我们留下评论。这真的有助于其他人找到这个节目。
BWS CEO is a production of foundation capital, an early stage venture capital firm, with over $3 billion in committed capital and 29 public companies to our name, including Netflix, Lending Club, TubeMobile and Sandra. That foundation capital, building companies is in our bones.
BWS CEO是Foundation Capital的产品,Foundation Capital是一家早期风险投资公司,拥有超过30亿美元的承诺资本和29家上市公司,包括Netflix、Lending Club、TubeMobile和Sandra等。在Foundation Capital,创立公司是我们的天命。
I'm Ashyo Garg, a general partner at foundation capital. I'm passionate about helping B2B entrepreneurs who are trying to solve hard problems. So if this podcast speaks to you, if you're a technical founder who's interested in scaling an enterprise startup into a massive business and scaling themselves into a true CEO, drop me a life.
我是Ashyo Garg,是Foundation Capital的总合伙人。我热衷于帮助那些试图解决难题的B2B企业家。如果你是一位技术创始人,并有意将企业初创公司扩展为庞大企业,并将自己打造成真正的CEO,那么欢迎与我联系。如果你对这个播客感兴趣,就请与我联系。