Adept CEO David Luan and Stanford's Percy Liang | Words into Action
发布时间 2023-03-14 16:01:15 来源
摘要
A re-broadcast of Greylock general partner Saam Motamedi's interview with Adept CEO and co-founder David Luan and Stanford computer science and statistics professor Percy Liang. In this conversation (recorded in mid-2022), David and Percy discuss how large language models are paving the way for the next wave of AI. Adept is developing an AI "teammate" tool that is trained to use every software tool and API for knowledge workers. The company just raised $350 million in Series B funding to further its mission. Greylock has been partnered with Adept since co-leading the Series A in 2022.
You can watch the video from this interview on our YouTube channel here:
https://youtu.be/_ydBm3tADvA
You can read a transcript of the conversation on our website here: https://greylock.com/greymatter/ai-language-words-into-action/
GPT-4正在为你翻译摘要中......
中英文字稿 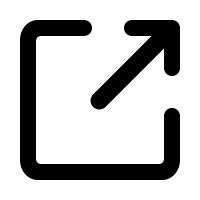
Hi everyone, welcome to GrayMatter, the podcast from GrayLock, where we share stories from company builders and business leaders. I'm Heather Mack, head of editorial at GrayLock.
大家好,欢迎来到GrayLock的播客节目GrayMatter,我们将与您分享来自公司创始人和商业领袖们的故事。我是Heather Mack,GrayLock的编辑主管。
Today, we're re-broadcasting our episode featuring GrayLock General Partner, Sam Modemadeys, conversation with David Lawn and Percy Lang.
今天,我们将重新播出一期节目,其中包括GrayLock General Partner Sam Modemadeys与David Lawn和Percy Lang的对话。
David is the co-founder and CEO of AI Startup Adept, and Percy is a computer science and statistics professor at Stanford. While text and image generating AI tools like ChatGPT and Dolly are all the rage right now, Adept is developing tools that take things a step further by actually executing actions based on text commands.
David 是 AI 初创企业 Adept 的联合创始人和 CEO,Percy 是斯坦福大学的计算机科学和统计教授。虽然像 ChatGPT 和 Dolly 这样的文本和图像生成 AI 工具现在非常流行,但 Adept 正在开发更进一步的工具,实际上执行基于文本命令的操作。
The company just raised 350 million in series B funding to further its development of a tool that can be thought of as an AI teammate, trained to use every software tool and API for every knowledge worker. GrayLock contributed to the latest funding round, and the firm has been partnering with Adept since collating the company's Series A in 2022.
这家公司刚刚筹集了3.5亿美元的B轮融资,以进一步开发一种被视为AI小伙伴的工具,该工具经过训练可使用每个知识工作者使用的软件工具和API。GrayLock参与了最新一轮融资,该公司自2022年整理了该公司的A轮以来一直与Adept合作。
In this interview, David, Percy, and Sam discuss how advancements in large language models are paving the way for the next wave of AI. This interview took place during GrayLock's intelligent future event in August 2022.
在这次访谈中,David、Percy和Sam讨论了大型语言模型的进步如何为下一波人工智能铺平了道路。这次访谈是在2022年8月的 GrayLock 智能未来活动中进行的。
The summit featured experts and entrepreneurs from some of today's leading artificial intelligence organizations. You can read a transcript of this interview on our website, and you can also watch the video from this interview on our YouTube channel. Both are linked in the show notes, and if you are already a subscriber to GrayMatter, you can sign up wherever you get your podcasts.
峰会上邀请了一些今天领先的人工智能组织的专家和企业家。你可以在我们的网站上阅读这次采访的文字稿,也可以在我们的YouTube频道上观看视频。两者都在节目注释中链接,如果你已经是GrayMatter的订阅者,可以在任何地方订阅。
Okay, David, Percy, I'm excited about this. There's no doubt that large-scale models are topical for all of us here, and I'm really excited to have the two of you to discuss them with.
好的,David和Percy,我对这个非常兴奋。毫无疑问,大型模型对我们所有人来说都是热门话题,我很高兴能有你们两个一起讨论。
For those of you in the audience who aren't familiar with these two gentlemen, Percy is the Associate Professor of Computer Science and Statistics at Stanford, where, among other things, sees the director for the Center for Research on Foundation Models. And David is one of the co-founders in CEO of Adept, an ML research and product lab building general intelligence by enabling humans and computers to work together.
那些听众中如果不太熟悉这两位先生的话,那么请允许我来介绍一下。Percy是斯坦福大学的计算机科学和统计专业的副教授,他还担任了基础模型研究中心的主任等职位。而David是Adept的联合创始人和首席执行官,该公司是一个机器学习研究和产品实验室,致力于让人类和计算机共同协作,构建通用人工智能。
And before Adept, David was at Google leading a lot of large models effort, and before that at OpenAI. And we're fortunate to get to partner with David and the team at Adept here at Gray Lock.
在加入Adept之前,David曾在谷歌领导了很多大型模型工作,之前还在OpenAI。我们很幸运能够与David以及Gray Lock的团队合作。
Percy, David, thank you guys for being here and for doing this.
Percy, David,谢谢你们来这里并做这件事。
So I want to start high level and just start with the state of the play. There's a lot of talk about large models, and it's easy to forget that a lot of the recent breakthroughs and models that we're all familiar with, like Dolly and GPT or GPT III are actually fairly recent.
所以我想先从一个宏观的角度入手,说说现状。现在有很多关于大模型的讨论,但很容易忘记我们熟知的一些最近的突破和模型,比如 Dolly、GPT 或 GPT III,其实都是相对较近的。
And so we're still in the early innings of these models running in production and delivering real concrete customer and user value. Maybe just give us the state of play David starting with you. Like where are we with large scale models and what's the state of deployment of these models today?
因此,我们仍处于这些模型在生产中运行并提供真正具体的客户和用户价值的早期阶段。也许,David可以先给我们介绍一下现状。比如,大规模模型的现状和这些模型的部署情况是怎样的?
Yeah, I think the stuff is just incredibly powerful, but I think we're still underestimating how much there is left to run on this stuff. Like it's still so incredibly early.
是啊,我认为这东西真是强大到不可思议,但是我觉得我们仍然低估了这个东西还有多少潜力。就像现在还是极其早期。
Like just like take a look at a couple different axes, right? Like when we were training these models at Google, it became incredibly clear up front that you could basically take a lot of these hand engineered machine learning models that people had been spending a lot of their time building, like rip it out with this giant model, give it some fine tuning data, and turn it into a smaller model again and serve it, and that would just end up outperforming all of these things that people had done in the past.
就像看一下几个不同的轴一样吧?当我们在谷歌训练这些模型时,很明显可以从一开始就基本上采用许多这些手动设计的机器学习模型,人们花费了大量的时间建立,像使用这个巨大的模型将其剥离,给它一些微调数据,然后将其转换成一个较小的模型,并提供服务,这将最终优于以前人们所做的所有这些事情。
And so like the fact that they're able to sort of improve existing things that companies are already using machine learning for, but also just like how great it has been as a way to be able to create brand new AI products that couldn't exist before.
他们能够改善企业已经使用机器学习的现有事物,这很了不起,同时也能够创造出之前不存在的全新人工智能产品。
Like it's fascinating to me to watch things like GitHub, Copilot, and like Jasper and stuff like that. Like just like hit a nerve so fast and go from zero to hero in terms of adoption. I think we're just in the very early innings of seeing a lot more of that.
我觉得看像 GitHub、Copilot、Jasper 这样的东西非常让人着迷。看到它们能够在采纳方面如此迅速地从零飙升,让我感到很兴奋。我认为我们现在只是开始见证更多这样的事情,还有很多潜力可以挖掘。
So I think like that's axis one. I think axis two to two is just that like primarily what we're talking about so far has been language models, right? But like there's so many other like modalities, sources of human knowledge, all of this stuff.
我认为这是第一维度。 我认为第二维度是我们目前主要谈论的是语言模型,对吧?但是还有很多其他的模态,人类知识的来源,所有这些东西。
Like what happens when like it's not just like predicting the next token of text, right, becomes about predicting all of those other different things. And like we're going to end up in a world where a lot of humanity's knowledge is going to get encoded in like various different like foundation models for many different things. And that's going to be really powerful as well.
就像当它不仅仅是预测文本的下一个标记那样时,就变成了预测所有其他不同的事情。我们最终会进入这样一个世界,其中许多人类的知识都会被编码在各种不同的基础模型中,涉及许多不同的领域。这也将非常强大。
Yeah, I kind of want to highlight, I agree with everything that David said. I want to emphasize one distinction he made, which is you know already with all the applications out there, these foundation models can just lift all boats and just make all the numbers kind of go up.
是的,我想强调一下,我同意David所说的一切。我想强调他所做的一个区别,那就是随着现在市面上所有的应用程序,这些基础模型可以推动所有的应用和数据,从而使整体表现提高。
I think another thing which is even more exciting is that there's a huge sea of applications that we're not even maybe even dreaming of because we're kind of sucking this paradigm where what is ML or you could get some data, you train on it. But with prompting and all these other zero shot capabilities, I think you're going to see a lot more kind of new types. So I think we should be looking not just for how to make kind of faster horses or faster cars, but kind of new types of applications.
我认为更令人兴奋的是,现在有大量的应用程序,我们甚至没有想到,因为我们一直局限于机器学习和数据上。但是,随着提示和其他Zero Shot功能的发展,我认为你会看到更多新类型的应用程序。所以我认为我们不应该只是寻找如何制造更快的马或汽车,而是寻找新类型的应用程序。
Percy, maybe to follow up on that, I totally agree and I think it connects to David's point around something like co-pilot. And I think that's amazing to me about something like co-pilot is both how new of an experience it is and how quickly it's taken off and gotten to end user adoption. What are some of the other areas that like you're looking forward to and are excited about in terms of net new applications that become possible because of these large models?
帕西,也许要跟进一下,我完全同意,我认为这与大卫关于副驾驶之类的东西有关。对于像副驾驶这样的东西,我认为令我惊讶的是它是多么新鲜的一种体验,以及它是多么快地被推广并获得终端用户的采用。因为这些大型模型可能带来的全新应用,你还期待和感到兴奋的其他领域是什么?
Yeah, so I mean maybe one general category can think about is creation. So this includes code, text, proteins, videos, PowerPoint slides, anything that you can imagine humans can do it right now, which could be a creative or sort of a more of a, you know, sort of a more task oriented activity. You can imagine these systems being very in the helping you in the loop, taking you kind of much farther and giving you many more ideas.
嗯,我的意思是也许有一个通常的范畴,我们可以考虑一下创造。这包括代码、文本、蛋白质、视频、PowerPoint幻灯片,以及你能想象出人类现在能做的所有事情,这可能是一项创造性的或更多的任务导向活动。你可以想象这些系统非常在帮助你,让你更进一步,给你更多的想法。
So I think the space is quite broad and I think underscoring the kind of a multimodal aspect of this which David touched on is really important. We shouldn't, you know, right now we have language models and we have code models and we have image models. But you know, think about things that you could do when you mix these together kind of creating different illustrated books or, you know, films or things like that.
所以我认为空间非常广阔,而且我认为强调这种多模式方面是非常重要的,这是David提到的。我们现在有语言模型、代码模型和图像模型。但是,你知道,想想当你把它们混合在一起时可以做些什么,比如创作不同的图画书、电影之类的东西。
I think one thing that you have to deal with is kind of the context, long context dependence. I mean, relatively right now you're generating single images or text up to, you know, maybe 2000 or 8000 depending on model tokens. But you know, imagine generating kind of full films that's going to require pushing the technology farther. But we have the data and if kind of harnessed that and scale up then I think there's a lot of possibilities out there.
我认为你需要处理的一个问题是上下文的长期依赖性。我的意思是,相对而言,你现在正在生成单个图像或文本,最多可能是2000或8000个令牌,具体取决于模型。但是,你知道,想象一下生成完整电影可能需要推动技术进一步发展。但我们有数据,如果利用它并扩大规模,那么我认为有很多可能性。
David, what would you add? At a depth you guys spend a lot of time thinking about how to use these models to unlock new ways of collaborating with computers and software. I'm curious what some of the use cases you think about art.
大卫,你有什么要补充的吗?你们在深入探讨如何利用这些模型来解锁与计算机和软件协作的新方式。我想知道你们考虑过哪些艺术的用例。
So I think the thing that I'm most excited about right now is that like I think all the creativity use cases I personally just highlighted are going to be extremely powerful. I think what's fascinating about these models, right, is like if you ask these these generative models to go like do something for you in the real world, they kind of just pretend like they're doing something because they don't have a first class sense of like what actions are and like what affordances are on your computer.
我现在最兴奋的事情,就是我个人强调的所有创造性使用案例都将非常有力。这些模型最有趣的地方在于,如果你要求它们在现实世界中做些什么,它们只是假装在做事情,因为它们没有对计算机上的行动和工具具有一流的感知能力。
So the thing that I'm really excited about in particular is like how do we bridge this gap, right? How do we train a foundation model of all of the actions that people take on a computer? And I think once you have that you have this like incredibly powerful base for being able to turn like natural language into like any sort of arbitrary complexity thing that you would then do on your machine.
我特别兴奋的是,我们如何弥合这个鸿沟?我们如何训练一个覆盖人们在电脑上所做的所有行为的基础模型呢?一旦你有了这个模型,你就拥有了一个非常强大的基础,可以将自然语言转化为任何你想在你的机器上完成的复杂任务。
So maybe if we take something like actuation as a key net new capability or we take longer contexts as an important net new capability, I think there's a, the form of the question is where do we still need to see key research on locks and where the key areas of focus to actually make like these products a reality?
也许如果我们将执行作为一个重要的全新能力,或者将更长的上下文视为一个重要的全新能力,我认为问题的形式是,我们还需要在哪些方面看到锁具的关键研究,以及实际上使这些产品成为现实的关键焦点在哪里?
I mean I think there are maybe two sides of things. One is pushing up capabilities and one is making sure things are pushed up in a way that's robust and reliable and safe. So the first one is kind of in terms of scaling. If you think about video and the ability to scale to you know hundreds of thousands of you know sequence lines, I mean I think you're going to have to do something different. The transformer architecture has gone this surprisingly far but you need to do something different there. And then I think you know David mentioned this briefly but I think these models are still in some ways chatterbots, they give you the illusion that there's something going on and I think if in certain applications this is actually you know okay if there's kind of another external validity check on things and with the human and the loop doing things.
我觉得可能有两个方面需要考虑,一个是提高能力,另一个是确保以坚固、可靠和安全的方式实现提高。第一个方面主要涉及规模,例如在视频方面,要能够扩展到数十万条序列线,这就需要采取不同的方法。尽管变压器架构已经取得了惊人的进展,但在这方面还需要做一些不同的工作。此外,我认为这些模型在某些方面仍然像 chatterbots,它们会让你有一种假象,好像在做一些事情,但如果在某些应用中能够有另一种外部有效性检查,并与人类进行交互,并进行检查,那么实际上也是可以接受的。
But I think there's a deep fundamental research question on how to make these models actually reliable and there's many strategies that people have tried you know using reinforcement learning or using kind of more explanation based or retrieval augmented methods. But I feel like there's still something kind of deeper missing and I think this is one thing I hope the academic community and researchers will work on to ensure that these foundation models have you know good and stable foundations as opposed to shaking ones.
我认为在如何使这些模型确实可靠的问题上,存在着一个深刻的基础研究问题,人们已经尝试了许多策略,例如使用强化学习或更注重解释的检索增强方法。但我觉得还缺少了一些更深层次的东西,我希望学术界和研究人员能够致力于确保这些基础模型具有良好和稳定的基础,而不是不稳定的。
Yeah, agree with a lot of what Percy just said. I think I would just add that like you know I think the default path that we're on is increasing scale and increasing data and I think that will continue to lead to a lot of gains but the question becomes how do we pull forward the future faster. And I think that there's a lot of different things that we should be thinking about.
是的,我同意 Percy 刚才说的很多话。我想我要补充的是,你知道,我认为我们正走上的默认路径是不断扩大规模和数据,我认为这将继续带来很多收益,但问题在于我们如何更快地拉近未来。我认为我们应该考虑很多不同的事情。
I think one is like specifically on the data side. I don't think most people I'm curious like later on it be curious to like at dinner and stuff to understand from the audience like how many people would agree that actually I think we're much more constrained on data than we think. I think within the next couple of years like everyone's going to have just to take language and example right like plus or minus 20 percent quality similar number of tokens web crawls anybody else right. So the question becomes like where next. So I think that's a really important question. I think we have another important question when it comes to like like what does like true creativity mean right like I feel like to me true creativity means being able to discover new knowledge and I think the new knowledge discovery process at least for foundation models as we're training out today as we actually get better at training these models that actually just better models the training distribution right. And so like I think giving these models the ability to go like gather new information and be able to try out things I think is also going to be really key. And finally I think on the safety side like we have a lot more to invest a lot more questions that we have to go answer.
我认为,其中一个问题特别集中在数据方面。我觉得大多数人可能在晚饭时会好奇,想要了解观众中有多少人同意我们对于数据受限的认识要比我们想象的更为严重。我认为,在未来几年内,每个人都将像语言和例子一样拥有相似数量的标记、网络爬取等数据,其质量可控制在正负20%左右。所以问题就变成了,下一步做什么。我认为这是一个非常重要的问题。当涉及到真正的创造力时,我认为真正的创造力意味着能够发现新知识,而我认为至少对于可以在基础模型上进行训练的模型,新知识发现的过程是不断完善培训分发的过程。因此,让这些模型拥有获取新信息的能力以及尝试新事物的能力也非常关键。最后,我认为在安全方面我们还有很多问题要解决,需要投入更多的资源来回答。
So let's let's get to safety in a moment continuing on data because I think that is a really important topic here David at adept you all are thinking about how to build products that humans collaborate with. And I think one of the nice consequences of that becomes the state of flywheel. Can you maybe add a little bit about how you're thinking about that and how you're approaching designing products that end users will work with.
那么,让我们先去安全的地方,接着谈论数据,因为我认为这是一个非常重要的话题。在adept,你们正在思考如何建立人类合作的产品。我认为其中一个好处是产生了一种“飞轮效应”。你能不能再多谈一些你们如何考虑这个问题,以及你们如何设计最终用户将使用的产品呢?
Yeah I think that like it starts out with sort of having a pretty crisp definition of what we want the end game to look like. I think for us what we want to be building is we want to be building like we want to be building like teammates and collaborators for people right like like a series of increasingly powerful software tools that sort of help humans increase the level of abstraction at which they can interact with their machine right. Like kind of like it doesn't like it to do a different analogy it doesn't replace the musician but it gives musicians synthesizers kind of right that kind of analogy except for doing things on your computer. I think because that's where we want to go I think what's really important to us is how do we solve these HCI problems where it really feels like you're working together with the machine at the same time using that as an opportunity for for for us to be able to learn from basically how humans break down really complicated problems how humans like actually get things done like that may be part of like things that are much more complicated than trajectories you might just be able to see on the internet.
我觉得,开始建设的时候,我们要有一个清晰的定义,明确我们希望最终成果的样子。对我们来说,我们希望构建的是一系列不断升级的软件工具,可以帮助人们提高与机器互动时的抽象水平,像是在构建队友和合作者。这有点像给音乐家提供合成器一样,它不是取代音乐家,而是提供更多的工具。我们想这样做的原因是因为我们希望解决人机交互问题,希望让使用者感觉在与机器合作,同时学习人类解决复杂问题的方法。这些问题可能比普通用户在互联网上看到的轨迹要复杂得多。
Just to add something to that I think the interaction piece is really interesting here because you know these models are though in some ways the most interactive ML models we have right you have a playground you can you type in a prompt and you immediately get us like you get to play with a model as opposed to this kind of a you know previous cycle where someone gathers some data trains a model and then you experience it kind of from the user. So the line between developer and user is actually kind of interestingly getting kind of blurred which I think is actually a good thing because if you can kind of connect these two up then you get kind of better get our experiences.
只是想补充一点,我认为这里的交互部分非常有趣,因为你知道这些模型在某些方面是我们最互动的机器学习模型,你有一个游乐场,你可以输入提示,你立即就能与模型一起玩耍,而不是以前的循环,某人收集一些数据训练一个模型,然后你从用户的角度去体验。因此,开发者和用户之间的界线实际上正在变得有点模糊,我认为这实际上是一件好事,因为如果你能将这两者联系起来,那么你就可以获得更好的体验。
Is there anything interesting from like the Stanford both the HCI perspective and the foundation models perspective on the research side that you all are working on around interaction?
你们工作中有在交互方面进行研究时,从斯坦福人机交互角度和基础模型角度看是否有有趣的发现?
Yeah so one thing that we've been doing in at Stanford is you know as a part of a kind of a larger benchmarking effort trying to understand the ways in which these models what it means for kind of models to humans to interact with these models right because the classic way that people think about these models is you know you train these models and then there's a you know 100 benchmarks and you evaluate and this is sort of taking the kind of automation you know approach but as we know a lot of the potential here is in you know co-pilot or auto complete kind of experiences where there is a human in a loop and humans I mean in a depth is I think is also a good example of this and what does that mean should we be building our models differently if we know that humans are going to be in the in the picture as opposed to you know you're doing kind of full automation and that's kind of an interesting thing because maybe in some cases the model you want a model not to just be you know accurate but you want it to be more interpretable or more reliable or kind of understandable and for creative applications you may want model to actually have a kind of a broader distribution of outputs and we're seeing some of this where you know what human was good for actually kind of interaction is not necessary what's good just for the kind of a standard benchmarks so that's really interesting.
嗯,史丹福大学最近在进行一项大型基准测试工作,在试图了解人类和模型相互作用的方式,以及这对模型意味着什么。因为人们传统上认为模型的训练是通过100个基准测试进行评估,这种自动化的方法逐渐被广泛应用,但我们也知道,当人类介入这个过程时,往往会产生更多的潜力,像GPT-2(一种自然语言生成模型)就是很好的例子。我们需要思考,如果我们知道人类将会出现在这个过程中,那我们是不是应该以不同的方式构建模型?有时,我们可能需要更可解释、更可靠、更易于理解的模型,对于创造性的应用来说,也许我们希望模型的输出分布更广泛。我们发现,有些情况下,人类在这个交互过程中发挥的作用,并不是标准基准测试中所需要的那种,这是非常有趣的。
How's that going to get resolved like I'm thinking about in classical and a lot of classical machine learning applications are some again even there it's still hazy but there's some point of view on benchmark standards there are different products out there that can actually measure these things around bias and auditing as we massively blow up the scope you know around creativity all of that kind of shifts so how do you think this is when to resolve. Yeah I mean so first order you know scale definitely is helping so we're safe on that if you scale up the models I think it lifts all boats and then given a particular scale then you have a question of you know where you're investing your your resources I think what we want to do is develop kind of effective surrogate metrics which you can actually evaluate which correlate well with sort of human interaction we don't really have a good handle on this quite yet but having humans in a loop for kind of a in a loop is also potentially kind of problematic and hard and not reproducible so you want something that's easy to evaluate but at the same time that's actually tracking what you care about.
这个问题怎么解决,就像我在经典机器学习应用中想到的那样。尽管在这方面仍然存在一些模糊的地方,但有一些基准标准的观点以及一些可以测量偏倚和审计这些事情的不同产品。当我们大量扩展范围时,所有这些都会发生变化,包括创新,因此你如何认为这个问题会解决?首先,规模肯定有帮助,如果您扩大了模型,那么所有的船都会升高。然后,在具体规模的基础上,您需要考虑投资资源的位置。我认为我们要发展一种有效的替代参数,这些参数与人类互动的相关性很好。然而,我们目前对此没有很好的掌握,但将人类放在一个循环中可能潜在地成为一个问题,并且难以重现。因此,你需要找到一些易于评估的东西,但同时又能追踪你关心的东西。
So I want to shift to building building products and companies around large scale models and David maybe I'll start with you like there are people in the audience who are in the early stages of building these companies and one fundamental question is okay do you go build on top of an open AI API do you go build on something in the open source do you go build your own large model like how do you how do you think a founder should navigate making that decision. I think it's probably the biggest question for people to ask right now I think the the root thing that I think is worth answering first is like what is the loop you're going to run for your company to compound like is it is it is it going to be oriented towards like really deeply understanding in particular customer's case is it going to be oriented towards some sort of data flybill that you're trying to build.
我想转向围绕大规模模型构建产品和公司,David,也许我可以从你那里开始。观众中有一些人正在初期建立这些公司,其中一个基本问题是:你会在开放的 AI API 上建立吗?你会在开源软件上建立吗?还是你会建立自己的大型模型?你认为创始人应该如何决定?我认为这可能是人们现在最需要问的最重要的问题。我认为首先值得回答的根本问题是,你将为公司运行什么循环以实现复合?它是否会面向深入了解特定客户案例?还是面向你正在尝试构建的某种数据枢纽?
I think the general thing here is that like like thinking about how that her faces with the differentiation that you want to have as a business is going to be really key because I think the world that you that I don't think we want to live in is one where effectively these companies become sort of like outsourced customer discovery engines and a new Amazon basic versions of these things come out over time right like that would not be a particularly good world to live in. So I think figuring out what that compounding looks like is the most important first step and the other thing that think about here is just like how many nines do you need. If you need a lot of nines of reliability I think one thing that's really really difficult is you just like you lack all the affordances that you could possibly want if you are sort of consuming this through an intermediary to get you to where you want me to be with your customers. So I think that like because of those different reasons like you could end up choosing a very different point in space for how you want to how you want to ultimately consume these services. Maybe just add one thing is that one nice thing about having these APIs is extremely easy to kind of get started and try something. You can sit down after noon and you punch in some data you can kind of get a sense of the possibilities and some cases is sort of a lower bound on how well you can do because you spend an afternoon and if you invest in more and you find to and build sort of custom things and can only get better in some sense.
我认为这里的一般想法是,想想如何应对作为一个企业所要具备的不同之处,这将是非常关键的,因为我认为我们不想生活在这样一个世界中,有效地将这些公司变成类似于外包客户发现引擎的公司,而且这些公司随着时间的推移推出了新的 Amazon 基本版本,这将不会是一个特别好的世界。因此,我认为找出这种复利是最重要的第一步,这里需要考虑的另一件事情就是你需要多少个“九”级别的可靠性。如果你需要很高的可靠性,我认为其中一个非常困难的事情就是,你缺乏你可能想要的所有合理的选择,如果你是通过中介来消费这些服务的话,你就无法完全掌握你想要与你的客户交流的方式。因此,我认为由于这些不同的原因,你可能会选择一个非常不同的空间点来决定你最终想要如何消费这些服务。也许只需补充一点,那就是拥有这些 API 的一个好处是非常容易开始尝试一些东西。你可以坐下来,花一个下午输入一些数据,你就可以了解到其中的可能性,在某些情况下,这是你可以做得更好的一个下限,因为你只花了一个下午的时间,如果你更多地投资并发现并构建定制的东西,那么你只会变得更好。
So, that I think has opened up a lot of the challenges to even formulate what is the right problem to go on and typically you don't know because you have to collect data and you have to train a model and then that loop becomes very expensive. But you can just sit down afternoon and try a few things and maybe like few shot your way to something that's actually reasonable.
所以,我认为这打开了很多挑战,即使是构思正确的问题都很困难,通常你不知道,因为你必须收集数据和训练模型,那样就非常昂贵了。但是,你可以坐下来尝试几件事情,也许可以用几次尝试找到一些实际合理的东西。
Now that kind of gets you kind of into a different part of the space and you can iterate much faster. Yeah, it makes a lot of sense in terms of prototyping quickly and trying to like take out product market fit risk. One question becomes in person curious for you to take on this. If you start that way, how do you over time build durability into your product? Because that could make the argument, hey, maybe you're just a thin layer on top of someone else's API, you can quickly do risk product market fit but is there real durability in your layer stack?
这种方法能让你快速进入不同领域的空间,从而更快地进行迭代。是的,就快速原型制作和尝试达成产品市场合适性而言,这是很有意义的。但我想问你一个问题,如果你一开始使用这种方法,如何在时间上构建产品的耐久性呢?因为有人可能会认为,嘿,也许你只是在别人的API上添加了一个薄薄的图层,你可以快速适应市场,但你所构建的层堆積能否真正具有耐久性呢?
Right. Yeah, I think the transition out of API is a very discrete one in some sense. I think it's kind of a, you know, people also do like human or a wizard of odd experiments. You put a human there and you have the human do it and then you see kind of work out all the interface issues and whether this makes sense at all and then you try to kind of put something else to human out. And now you can put an API there and you can see get a sense of what things are like. And then in some cases, like maybe, you know, fuchsia learning is actually for some things actually not that strong if you have, for example, data and maybe like a fine tune like T5 model or something much smaller can actually be effective and, you know, I don't think, I think the last thing you might should be like, let's go pre-trained of 500 billion parameter model when you don't know what application you're building.
没错。是的,我认为从API过渡出去在某种意义上是一个非常具体的过程。我认为这有点像人类或奇怪实验。你把一个人放在那里,让他做,然后你就可以解决所有的接口问题,看看这是否有意义,然后你尝试把其他东西变成人类。现在你可以放一个API在那里,你可以看到事情的感觉。在某些情况下,就像Fuchsia learning一样,如果你有数据和一个调整好的T5模型或者其他更小的东西,它实际上对某些事情并不是那么有效。而且,我认为最后一件事不应该是,当你不知道你正在构建什么应用时,让我们去预先训练5000亿个参数的模型。
Maybe continuing on the theme of building on top of these models, like despite the magical qualities of these things, there's still limitations, right? Like one of the limitations is falsehoods and there are others that I think developers need to navigate as they think about building these applications. David, maybe starting with you, like what do you think some of the key limitations are? And how do you guide people around navigating those?
也许继续沿着利用这些模型的主题,可能人们往往会认为这些东西有着神奇的特性,但是它们仍然有限制,对吧?其中一个限制是虚假和其他开发者需要考虑构建这些应用程序时要面对的限制。David,也许从你开始,你认为一些主要限制是什么?你如何引导人们解决它们?
That's a really good question. I think falsehoods are definitely a very interesting thing to talk about. These models love to be massive hallucination engines. And so getting them to stick to script can be quite difficult. I mean, I think in the research community we're all aware of a bunch of different techniques for improving that, from things like learning from human feedback to potentially like augmenting these models with retrieval and such.
这是一个非常好的问题。我认为说谎肯定是一个非常有趣的讨论话题。这些模型喜欢变得非常虚幻。因此让它们遵循脚本可能会很困难。我的意思是,在研究界,我们都知道很多不同的技术来改进这个问题,比如从人类反馈中学习,或者像检索等方式来增强这些模型。
I do think that on the topic of falsehoods in particular, this idea of packing all of world facts into the parameters of a model is pretty inefficient and somewhat wasteful, especially when some of those facts change over time, who may be running a particular country in a particular moment. And so I think it's pretty unlikely that that's going to be the terminal state for a lot of these things. So I'm really excited for a lot of the research that will happen to improve that. But I think the other part actually goes back to a question of practicality and HCI, which is that you kind of a sense of like every year, you know, like we're pushing fundamental advancements on these models. They get somewhat better on a wide variety of different tasks that are already shown receptivity to scale and receptivity to more training data examples. But how do you sort of like surf this wave where the particular capability you're looking for from the model are good enough to be deployed where you can learn how to get from there to the finish line. And how do you work around some of these limitations in the actual interface to these models such that it doesn't become a problem for your user to use that? I think that's actually a really fascinating problem.
我认为在讨论谎言这个话题时,把所有的世界事实都塞进一个模型的参数里是相当低效且有些浪费的,尤其是当一些事实随着时间而改变,比如在某个特定的时刻运行某个特定国家的领导人可能会变。因此,我认为这对于很多问题来说,这不太可能是终极状态。所以我非常期待进一步的研究来改善这一点。但是,我认为另一部分实际上涉及实用性和人机交互的问题,你需要一种感觉,就像每年我们都在推进这些模型的基本进步一样。它们在各种不同的任务上有些进步,这些任务已经显示出对规模和更多的训练数据示例的接受度。但是,你如何滑过这个浪潮?你需要模型的特定能力达到足够好的程度,才能投入实际应用并学习如何从中走向终点? 你如何解决这些模型界面上的一些限制,使其对用户使用不成为问题?我认为这实际上是一个非常有趣的问题。
Yeah, I mean, these models I think are exciting and the kind of flip side is that they have a ton of weaknesses and in terms of falsehoods, generating things that are not true, biases, stereotypes, and basically all the bad, good and bad and ugly of the internet kind of gets put into it.
是啊,我的意思是,我认为这些模型很令人兴奋,然而它们也有很多弱点,比如制造不真实的虚假事物、偏见、刻板印象等等,基本上是把互联网上所有好坏丑陋的东西都融入其中。
And I think, you know, it's actually much more nuanced than just like let's remove all the incorrect facts and de-biase these models because there are efforts on filtering. You can filter out a kind of offensive language, but then you might end up marginalizing certain populations. And what is truth? We like to think there's a truth, but there's actually a lot of text which is just opinions and there's different viewpoints. And a lot of it's not even false and fireballs. You can't talk about truth without even false and fireability.
我觉得,你知道的,实际上比只是删掉所有不正确的事实和消除这些模型的偏见要复杂得多,因为有过滤的努力。你可以过滤出某种具有攻击性的语言,但这样可能会排斥某些族群。什么是真相呢?我们喜欢认为有一个真相,但实际上有很多文本只是观点和不同的视角。很多甚至不是错误和谎言。你不能谈论真相甚至没有错误和可燃性。
And in a lot of cases, you know, and there are some applications, for example, you know, creative cases where, yeah, you do want maybe things which are, you know, a bit more edgy and how you create fiction, for example, and if you can't, if everything has to be true. So it's, you can't really, there's no easy way to kind of even, even if you could throw out all the kind of quote unquote, you know, bad stuff.
在很多情况下,你知道,有一些应用程序,例如,创意案例,可能会需要一些更加前卫的东西,比如你创建小说时。如果你不能,如果一切都必须是真实的。所以,你不能真的,即使你可以抛弃所有的所谓坏东西,也没有简单的方法。
So I think one thing that maybe is a good framework to think about is that there's no one way to kind of make these models up to much better. I think that what you want is, you know, control and, you know, documentation. You want to be able to understand, given a model, what is it capable of, what should it be used for and what it should not be? And this is tricky because these models have huge capability surface, right? You just get a, get a prompt. You can put any string and get any other string back.
所以我认为一个好的思考框架可能是,没有一种方法可以让这些模型更好地生成。我认为你想要的是控制和文档。你需要能够理解模型的能力,它应该被用于什么,什么不应该被用于?这是棘手的,因为这些模型有巨大的能力表面,对吧?你只要得到一个提示,就可以输入任何字符串并获取任何其他字符串。
So what am I supposed to do? What are the guarantees? And I think as a community, we need to develop a better language for thinking about, you know, what are the possible inputs and outputs? What are the contracts? Like the things that you have in traditional APIs and good old fashioned software engineering, we kind of need to import some of that so that downstream application developers can look at it's like, oh yeah, okay, I'll use this model, not that one for my particular use case.
那么我应该怎么做?有什么保障吗?我认为,作为一个社区,我们需要开发一个更好的语言来思考,您知道,可能的输入和输出是什么?有哪些合同?像传统API和经典软件工程中的内容一样,我们需要引入一些东西,以便下游应用程序开发人员可以看到它是像“噢,好的,我会使用这个模型,不是我那个特定的用例。”
There's another side to this which for lack of better word, I'll use the word risk, which is if I'm a product builder and I'm building these models and building different products, there's different levels of risk I might be willing to take in terms of what I'm willing to ship to end users and the level of guardrails I need in place before I'm willing to ship.
还有另一面需要考虑,用一个更好的词来说就是“风险”。如果我是一个产品制造商,我正在制造这些模型和不同的产品,那么就有不同的风险等级,我可能会愿意承担,以及在我愿意发货之前需要设定的防护措施的级别。
How do you guys think about that and frameworks are on that?
你们怎么看待这件事以及与之相关的架构?
So I think there's some interesting perspectives here specifically around just like how do you sequence out the different applications that you want to go after, right? I feel like if you can really, like one really nice property of these models is it's just so easy to go from zero to a hand-wavy 80% quality on a wide variety of tasks. For some of those tasks that's all you need and sometimes the iteration loop of having that 80% thing with humans is all you need to do to go run it over the finish line.
我认为这里有一些有趣的观点,特别是关于如何按顺序选择不同的应用程序,对吧?我觉得,如果你能够真正做到这一点,这些模型的一个非常好的特性是在很多任务上轻松地从0到达到80%的质量。对于其中的一些任务,这就是你所需要的,有时候仅有这80%的东西和人类的迭代循环就足以让你跑过终点。
I feel like right now the biggest argument we all have is starting out by first addressing things like that. But I think that over time, actually one of the things that Kevin said that I really liked is that there will be more of a standardized toolkit for how to erase some of the lower hanging fruit risks related to generations that are inappropriate or models going off the rails in various different ways.
我感觉现在我们所有人的最大争议是首先通过解决这些问题来开始。但我认为随着时间的推移,凯文所说的一件我很喜欢的事情是,将会有更多的标准化工具包,以消除一些与不当世代或模型在各种不同方式下失控有关的风险。
I think there's another set of risks that are slightly longer term that I think are also really important to go think about and I think those are definitely much harder. To build on top of that, I think there's two maybe different, also different categories or maybe one category of risk is also adversaries in the world, right?
我认为还有一些稍长期的风险需要考虑,也非常重要,这些风险肯定更难处理。除此之外,我认为还有两种甚至是一种风险的分类也是非常重要的,那就是世界上的对手,对吧?
Whenever you have a product that's kind of getting enough traction, there's probably people who want to mess with you. One example is data points in it. One thing, and decided I think hasn't really been born out, there's some papers on it.
每当你推出一个有足够关注度的产品时,很可能会有人想要向你捣乱。其中一个例子就是数据点。关于其中一件事情,我认为有一些文件并没有被证实。
If you think about it, these models are trained on the entire whatever web crawl. Anyone could go put up a web page or put something on GitHub and that can enter the training data and these things are actually pretty hard to detect. If you think from a security point of view, this is like a huge gaping hole in your system and a determined attacker could probably figure out a way to screw over your system.
如果你想一想,这些模型是在整个网络抓取的数据上进行训练的。任何人都可以放置一个网页或在GitHub上放置一些内容,这些内容都可以成为训练数据,而且这些东西实际上非常难以检测。从安全的角度来看,这就像你的系统中的一个巨大漏洞,有决心的攻击者可能会找到一种方法来破坏你的系统。
The other thing to think about is misuse. These systems, all systems, the powerful models like these are dual use technologies. There's a lot of immense good that you can do with them, but they can also be used for fraud, disinformation, spam and all the things that we already know exist, but now amplified. That's kind of a scary thought. Definitely a lot of asymmetric capabilities here, because just like cooking up one of these giant code models to some RL agent to just get into systems, there's so many things out there that becomes way easier for malicious actors to do as a result. Attack is so much easier than defense.
另一个要考虑的问题是误用。这些系统,所有系统,像这样强大的模型都是双重使用技术。你可以用它们做很多巨大的好事,但它们也可以被用于欺诈、虚假信息、垃圾邮件和我们已经知道存在的所有事情,但现在被放大了。这是一种可怕的想法。显然,这里有很多不对称的能力,因为就像烹制一个这样的巨型代码模型给一些强化学习代理,让它们进入系统,有很多事情变得更容易被恶意行为者做到。攻击比防御容易得多。
I have a few more questions I want to get through, but just watching the time, I want to first open it up and see if there are questions in the audience. There's a question for Percy from an academic standpoint of what is ideal wish list that you might have in ways that corporations and big companies that are building a lot of the ecosystem could help?
我还有几个问题要问,但是要看时间,先开放让听众问问题。从学术角度来看,有一个针对 Percy 的问题,你可能会有哪些理想的愿望清单,让那些建设大量生态系统的公司和大型企业能够提供帮助?
Yeah, I think one big thing is openness and transparency, which is something I think is really sorely missing today. I think if you look at the deep learning revolution, what's been great about it is that it's benefited from having an open ecosystem with toolkits like TensorFlow, PyTorch, data sets are online tutorials, and people can just download things, tinker, and play with it. It's much more accessible. Now we have models which are behind APIs and with charging certain fees. Things have, you can't really tinker as much with it. I think, and also at the same time, a lot of organizations are addressing the same issues around me and we talked about safety and misuse, but there's not a agreement on what is the best practices.
嗯,我认为一个很重要的问题是开放性和透明度,这是我认为今天非常缺乏的东西。我认为如果你看看深度学习革命,有一个开放的生态系统是很不错的,例如TensorFlow、PyTorch工具包、在线教程和数据集,人们可以下载、修改和玩弄它。这使得它更加容易接触。现在我们有一些模型,它们被API所隐藏,并且需要支付一定的费用。这样做,你就不能把它当作玩意儿来琢磨了。同时,很多组织都在解决我所提到的安全和滥用问题,但并没有就什么是最佳实践达成一致。
I think what would be useful is to develop community norms around what is safe or what are best practices for mitigating some of these risks. In order to do that, there has to be some level of openness as well so that when a model is deployed, you have to get a sense of what these models are capable of and you kind of benchmark them and document them in a way that the community knows how to respond as opposed to, here is a thing you can play with. It might shoot yourself in the foot or it might not.
我认为有用的是制定社区规范,以确保安全并采取最佳实践来降低某些风险。为了做到这一点,也需要一定程度的开放性,这样在部署模型时,你必须了解这些模型的能力,并记录它们,以便社区知道如何应对,而不是简单地告诉大家可以随意尝试。这可能会对自己造成伤害,也可能不会造成任何影响。
Good luck. We have time for one more question. So maybe I'll ask a final question which goes back to creativity. I think one of the important things to inspire in all of us is what's going to be possible, the magic of these models. I think Dolly was an important moment for people to see the type of creative generation that's possible. I guess for each of you, what are some of the things that you think are going to be possible in the way we interact with these models in a few years that you're most excited about?
祝你好运。我们还有时间再回答一个问题。因此,也许我会提出一个最终问题,关于创造力。我认为,激发我们所有人的重要事情之一是什么会成为可能,这些模型的魔力。我认为多莉(Dolly)是人们看到可能的创造性一代的重要时刻。我猜想,对于你们每个人而言,在未来几年中,我们将与这些模型互动的一些可能的事情,你们最激动人心的是什么?
Maybe starting with you, David. I think language, as we were talking about earlier, language is just the tip of the iceberg. We're already seeing amazing things just with language but I think when we start seeing foundation models or even bundle together foundation models that are multimodal for every domain of human knowledge, every type of input to a system or to ourselves that we care about, I just think we're going to end up with some truly, truly incredible, truly incredible outcomes with that.
也许我们可以从你开始,David。我认为,正如我们之前谈论的那样,语言只是冰山一角。我们已经通过语言看到了一些令人惊奇的事情,但我认为,当我们开始看到基础模型,甚至将多模态基础模型捆绑在一起,覆盖人类知识的每个领域,以及我们关注的每种输入系统或自己的类型时,我们就会得到一些真正令人难以置信的成果。
I think if I were to choose a personal thing that I'm not working on that I think would be really cool, actually I think person I talked about this once is what happens when you start having foundation models for robots. When you can take all of these demonstrations, all of the different trajectories of robots interfacing with the real world and sort of like put them all into one particular system and then have them have the same type of generality that we've seen with language. I think that'd be incredible.
我认为,如果我选择一件我没有在做的个人项目,我认为真的很酷的事情,就是当你开始为机器人建立基础模型时会发生什么。当你可以把所有这些演示,所有不同的机器人路径与真实世界的接口都融合在一个系统里,然后让它们拥有与语言相同的普遍性时,我认为这将是令人难以置信的。我曾经和一个人谈论过这个想法。
Yeah, I agree with that and I have definitely thoughts but maybe I'll end with a different perspective, not a different perspective but another example which is if you think about we get excited about generating an image, it's just an image and it's also something that you might imagine artists being able to do.
是的,我同意这个观点,我有自己的想法,但也许最后我会提出另外一个例子,不是改变观点,而是提供另一个例子。例如,假设我们很兴奋地生成一张图像,但实际上这只是一个图像,这也是艺术家可以想象得到的事情。
If you think about humans aren't changing that much, computers are and before a year ago or two years ago we weren't able to do that now we can and so if you extrapolate now images only, it's so small and you think about videos or now you think about 3D scenes or immersive experiences with personas, you could imagine generating worlds in a sense and that's kind of scary but also sort of exciting.
如果你认为人类没有太大的变化,那么电脑变化巨大。仅仅在一两年前,我们还不能够做到这一点,现在我们可以了。所以如果你现在只考虑图像的话,那么这只是微不足道的。想象一下视频或者3D场景或者具有人设的沉浸式体验,你可以想象生成一个世界,在某种程度上这很可怕,但同时也很令人兴奋。
I don't know there could probably have many possibilities there but I think the bigness of things that you can create, I mean if you think about these models as excellent creators of large objects now and you think about what are big objects, well there are kind of environments in some sense and what if we could kind of do that, what would that look like and what would you order kind of some applications that could unlock, that would be interesting to think about.
我不知道可能有很多可能性,但我认为你可以创造很大的东西,如果你把这些模型看作是创建大型物体的优秀工具,那么当你思考大物体的时候,那就是一种环境,在某种意义上,如果我们能够做到这一点,那会是什么样子? 这将会解锁哪些应用程序,想一想会很有趣。
Yeah there's a commonality there which again connects back to multiple modalities and just continuing to push scope, it's a really exciting glimpse of the future.
是的,有一个共同点,又一次连接到多种模式,并且不断推动范围,这是一个非常令人兴奋的未来展望。
Percy David thank you guys so much for doing this, thank you. That concludes this episode of Grey Matter. Like what you hear, we encourage you to rate and review Grey Matter on your favorite podcast platform, we sincerely appreciate your feedback.
Percy David,非常感谢你们进行这个节目,谢谢!这个Grey Matter的节目也因此结束了。如果你喜欢我们的节目,请去你喜爱的播客平台评分和评论,我们非常感激你的反馈。
You can also find all content on our website at greylock.com or on our YouTube channel. You can also follow us on Twitter at greylockvc.
你也可以在我们的网站greylock.com或我们的YouTube频道中找到所有的内容。你也可以在Twitter上关注我们的greylockvc账号。
I'm Heather Mack, thanks for listening.
我叫海瑟·麦克,谢谢你的倾听。