The Top 100 GenAI Products, Ranked and Explained
发布时间 2025-03-26 15:00:47 来源
这段对话围绕着“Gen AI 100 榜单”展开,这是一份追踪消费者人工智能领域情况的报告。该报告分析了网页和移动端排名前 50 的 AI 原生产品,排名分别由每月访问量(通过 SimilarWeb 获取)和每月活跃用户数(通过 Sensor Tower 获取)决定。报告首次考察了营收排名前 50 的移动应用。讨论探讨了人工智能消费者意识的关键时刻、该领域中被打破的假设,以及人工智能发展的当前阶段。
讨论强调了消费者人工智能意识的关键转折点:Midjourney 和 Character AI 早于 ChatGPT 的早期采用,Snapchat 的 “My AI” 机器人达到 1.5 亿用户,病毒式传播的 “Balenciaga Pope” AI 图片,以及 AI 生成的歌曲 “BBL Drizzy”。可口可乐的 AI 生成的圣诞节广告标志着企业意识的转变,而 Deepseek 的发布表明,横向模型仍然可以实现大规模的消费者规模。
各种假设不断受到挑战。对话指出了诸如 “人工智能无法欺骗我,让我认为一张照片是真的” 或 “没有人可以渗透 ChatGPT 的市场” 这样的假设。发言者认为,我们仍处于早期采用阶段,甚至处于基础设施建设时代,正在向应用构建阶段发展。AI 视频就是一个很好的例子,它能够生成短片,但还不能生成几分钟长的电影。对话还讨论了人工智能仅仅用于事务性交互的错误假设。
报告发现网页排名中有 17 家新公司,这表明该领域充满活力。来自中国的 AI 视频模型和 OpenAI 的 Sora 是新晋者。像 Cursor 和 Bolt 这样的 “氛围编码” 产品正在兴起,它们使非技术用户能够通过文本提示创建 Web 应用程序。一个新的 “边缘名单” 突出了几乎进入前 50 名的公司,表明了快速的变化。
发言者预测会看到更多用于可扩展视频的风格迁移。风格迁移是一个可控的问题,并且具有比原始文本到视频更低的推理成本。另一个意想不到的发现是,尽管发布了像 Gemini Flash 和 OpenAI 的操作员模型这样的新模型,但基于语音的 AI 产品却很少出现。
一个令人惊讶的发现是,氛围编码工具在技术受众中的快速饱和。陪伴类产品(通常面向 NSFW 内容)的出现仍然是一个持续的惊喜。
ChatGPT 一直位居榜首,但其发展轨迹并非线性。最初平稳,最近因新模型和产品而流量激增。
Deepseek 也表现出显著的增长,挑战了广泛的 AI 产品无法流行起来的假设。Deepseek 的用户留存率略低于 ChatGPT,而美国市场是其驱动因素。来自中国的 AI 视频模型的流行归因于较少的版权敏感度和更逼真的提示。像 Krea 这样聚合多个 AI 模型的聚合器也越来越受欢迎。
关于收入,发言者指出收入与用户群不匹配。照片/视频生成器和编辑器、美颜滤镜和 ChatGPT 模仿应用程序获得了成功。
最重要的是产品本身。创始人应专注于解决痛点并创造独特的体验,并以数据为指导。“更复杂的事情实际上并非对消费者用户来说最有用的、最令人愉悦的、最有帮助的。” 模型和应用的选择正变得越来越有主观性。
This conversation revolves around the Gen AI 100 list, a report tracking the consumer AI landscape. The report analyzes the top 50 AI-first products on web and mobile, determined by monthly visits (via SimilarWeb) and monthly active users (via Sensor Tower), respectively. For the first time, the report also examined the top 50 mobile apps by revenue. The discussion explores pivotal moments in the AI consumer awareness, assumptions being shattered in the space, and the current stage of AI development.
The discussion highlights key turning points in consumer AI awareness: Midjourney and Character AI's early adoption before ChatGPT, Snapchat's "My AI" bot reaching 150 million users, the viral "Balenciaga Pope" AI image, and the BBL Drizzy AI-generated song. Coke's AI-generated Christmas ad marked a shift towards enterprise consciousness, while Deepseek's launch demonstrated that a horizontal model could still achieve mass consumer scale.
Assumptions are constantly being challenged. The conversation points out the assumptions like "AI can't trick me into thinking a picture is real," or "No one can penetrate ChatGPT's market." The speaker argues that we are still in the early adopter phase, and even in the infrastructure-building era, progressing into application building. AI video is a prime example, capable of generating short clips, but not yet minutes-long films. Incorrect assumption of AI being only for transactional interactions is also discussed.
The report found 17 new companies on the web rankings, signifying a dynamic landscape. AI video models from China, and Sora from OpenAI are among the new comers. The rise of vibe coding products like Cursor and Bolt, enabling non-technical users to create web apps from text prompts, is also noted. A new "brink list" highlights companies that almost made the top 50, indicating rapid changes.
The speaker predicted seeing more style transfer used for scalable video. Style transfer is a tractable problem and has a much lower cost of inference versus raw text to video. Another unexpected finding was the low presence of voice-based AI products despite new models like Gemini Flash and OpenAI's operator model being released.
One surprise was the rapid saturation of vibe coding tools within the technical audience. The presence of companion products, often NSFW-oriented, also remains a consistent surprise.
ChatGPT is consistently at the top, however, its trajectory has not been linear. Initially flat, traffic surged recently due to new models and products.
Deepseek also demonstrated significant growth, challenging the assumption that a broad-based AI product couldn't go viral. Deepseek's retention is just below ChatGPT's, and the US market has been its driving factor. The popularity of AI video models from China is attributed to less copyright sensitivity and more realistic prompts. Aggregators like Krea, which combine multiple AI models, are also gaining traction.
Regarding revenue, the speaker notes the revenue is not mirroring with the user base. Photo/video generators and editors, beauty filters, and ChatGPT copycat apps are successful.
The most important thing is the product itself. Founders should focus on solving pain points and creating unique experiences, guided by data. More complicated thing is not actually the thing that is highest utility most delightful most helpful to a consumer user. The choice of models and applications is becoming more opinionated.
摘要
This month, a16z's Consumer team released the fourth edition of the GenAI 100 — a data-driven ranking of the top 50 AI-first web ...
GPT-4正在为你翻译摘要中......
中英文字稿 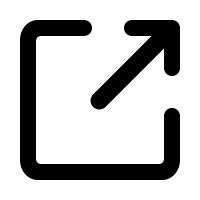
Consumer activity typically lags by 6 to 9 to 12 months. What's happening on the research side? I think compared to where we're going to be, we're still incredibly early. So many of these assumptions, and that's why they're assumptions, they seem intuitively correct, they're going to turn out to be incorrect. We are finally on the verge of AI video starting to work, to really work. It sort of follows the trend of AI decreasing the cost of creation in every way. 95% of YC companies are now kind of building, using those tools. The app store is going to be chaos.
消费者活动通常会滞后6到9到12个月。那么在研究方面发生了什么呢?我认为,与我们未来的情况相比,我们目前还处于非常早期的阶段。许多假设,看似合乎直觉,实际上可能会被证明是错误的。我们终于接近实现AI视频功能,这项技术即将真正发挥作用。这与AI在各个方面降低创作成本的趋势相符。现在,95%的YC公司都在利用这些工具开发产品。应用商店将会变得一片混乱。
Yeah, I guess. We're back for the fourth edition of the Gen AI 100 list. You guys have been working hard and tracking the consumer landscape for years now, but specifically for the last two and a half years since we really had that kind of chat GBT moment. Tell me more about how you're tracking that ecosystem and how that comes through in this list.
好,我想我们又回到了第四届Gen AI 100榜单。你们一直在努力工作,追踪消费者市场多年,但特别是在过去的两年半里,自从我们经历了类似ChatGBT的时刻以来。请告诉我你们是如何追踪这个生态系统的,以及这些追踪结果如何体现在这个榜单中。
Yeah, it's super fun. This is one of my favorite reports that we put together a couple times a year. We track the consumer AI landscape through what we do every day, which is like meeting with consumer AI startups that come to pitch us, seeing what goes viral on Twitter. But actually, there's a whole separate set of companies and products that might be reaching the true mainstream consumer that might not even be marketing themselves as AI products, but they're kind of powered by and made possible by AI.
是的,这真的非常有趣。这是我们一年中会编写几次的我最喜欢的报告之一。我们通过日常的工作来跟踪消费者人工智能的发展情况,比如和来向我们推介的消费者人工智能初创企业会面,看看哪些在推特上变得流行。不过,实际上还有一整套不同的公司和产品可能正在接触真正的主流消费者,它们甚至可能没有将自己宣传为人工智能产品,但实际上它们是由人工智能驱动和实现的。
And so the whole original purpose of this report was to see how much overlap is there between those two categories and what is the actual everyday person who might not know that they care about AI using in their day to day. That's great. And so talk about the methodology, like what makes it onto this list or not? Because to your point, there's certain household names that you might see on Twitter or have that viral moment. But I think some people might be surprised to see what made it onto this list.
这份报告的初衷是为了了解这两个类别之间有多少重叠,以及那些可能不知道自己在日常生活中关心人工智能的普通人到底是什么样的。这很棒。接下来,我们来谈谈这份名单的筛选方法。因为正如你所说,我们可能会在推特上看到一些非常知名的名字或一些瞬间走红的事件。但我觉得有些人可能会对这份名单上究竟有哪些名字感到意外。
So let's start with the methodology and what it requires. Yeah, so it's entirely based on data. We have two lists here, the top 50 on web and the top 50 on mobile. So the top 50 on web, we use a data provider called similar web, which tracks every single website globally. And we essentially go down and descending order of how many visits they get each month. For this report, it was January 2025. And then we go and we pick the first 50 of those that have the most monthly visits that are Gen AI first products.
好的,让我们先从方法论开始,这需要做些什么呢?基本上,这完全依赖于数据。我们这里有两个列表,一个是网络上的前50名,另一个是移动端的前50名。对于网络上的前50名,我们使用了一家叫做SimilarWeb的数据提供商,他们会全球监测每个网站。我们按照每月访问次数从多到少的顺序排列这些网站。在这份报告中,我们关注的是2025年1月的数据。从这些网站中,我们选择了每月访问量最多且以生成式人工智能为核心产品的前50个网站。
We do something similar on mobile, but a different dataset from sensor tower for mobile. We look at monthly active users on the app and then again, we pick the top 50 that are Gen AI products. And then for the first time ever, we actually looked at the top 50 on mobile by revenue, which we hadn't done before. And it was a really interesting experiment because the lists were pretty non-overlapping.
我们在移动端也做了类似的事情,但使用的是Sensor Tower提供的不同数据集。我们查看应用程序的月活跃用户,然后挑选出前50名的生成式人工智能产品。之后,我们首次根据收入分析了移动端的前50名,这在之前从未做过。这是一个非常有趣的实验,因为这两个榜单几乎没有重叠的内容。
We've been in this AI ecosystem for a few years now. In your eyes, what were the pivotal moments that led up to this point in time where we have like you said 50 and mobile, 50 on desktop and a whole lot more in the wider ecosystem? You often say actually it's usually like the papers are written and then the models are developed and then applications are built on top of it.
我们在这个人工智能生态系统中已经有几年了。在你看来,到目前为止,我们经历了哪些关键时刻,才让我们如今有你所说的移动端50款、桌面端50款以及在更大生态系统中有更多应用?你常说,通常是研究论文先被发表,然后模型被开发,接着在此基础上构建应用程序。
So the consumer activity typically lags by six to nine to 12 months. What's happening on the research side? So maybe just from like a consumer awareness or behavioral perspective, there's a couple moments for me. Actually mid-journey and character AI both came out before ChatGBT, which I think a lot of people don't know, but there was maybe these early niche communities of early adopters that were using both of those products in the summer and the fall of 2022 leading up to ChatGBT.
消费者活动通常会滞后6到12个月。那么研究方面的进展如何呢?从消费者认知或行为的角度来看,有一些时刻让我印象深刻。其实,Mid-Journey和Character AI在ChatGBT之前就已经问世了,不过我认为很多人对此并不清楚。在2022年的夏天和秋天,这些产品已经在一些早期采纳者的小众社区中使用,为ChatGBT的推出铺平了道路。
And then post ChatGBT things that just brought AI to consumer consciousness. So even remember Snapchats, my AI, with that little bot that appeared at the very top of your feed. And like 150 million people used it. And for a lot of kind of younger consumers, that was actually probably their first real chance having a conversation with an LLM.
然后,发布ChatGBT,这件事使得人工智能进入消费者的意识。就像Snapchat的“我的AI”一样,它在你的消息顶部出现的小机器人。大约有1.5亿人使用过它。对于许多年轻消费者来说,这可能是他们第一次真正有机会与大型语言模型(LLM)进行对话。
On the image side, I think of the Valenciaga Pope, which was also I think spring 2020. Such a cultural moment. It was. And I think it made a lot of people realize for the first time that they should even be interested in AI images because they could be that good and that convincing. The first big AI music moment for me was well the BBL Drizzly song, which I think was spring of 2024.
在图像方面,我想到的是2020年春季的Valenciaga教皇。这真是一个文化时刻。我觉得这让很多人第一次意识到,他们应该对AI生成的图像感兴趣,因为这些图像可以如此出色和逼真。对于我来说,第一个重要的AI音乐时刻是BBL Drizzly的那首歌,我记得是在2024年春季。
And that also went like mega viral. I think one of the moments where creative AI really shifted into almost enterprise consciousness was the end of last year when Coke did their Christmas ad. And a lot of that was generated by AI. And then of course the deep seek launch earlier this year. Deep seek was so interesting because I think it sort of had become settled wisdom that it would be very hard for horizontal model to get to mass consumer scale quickly again.
这段英文大意是:那段内容也成为了爆款。我认为,在去年年底的时候,创意人工智能第一次真正进入企业意识,这个转折点就是可口可乐发布的圣诞广告,其中很多部分是由人工智能生成的。当然,还有今年年初的Deep Seek发布。Deep Seek特别有意思,因为之前普遍认为,要让一个水平型模型再次快速达到大众消费规模是非常困难的。
Like Chatchy PT had kind of done it and Chatchy PT had become a verb and that opportunity had already been explored. And now we see deep seek growing as quickly as it did. And there's actually a couple of interesting nuances to deep seek. So one, I think, important nuances, the fact that they released their reasoning model for free at scale. So previously you had to use O1 Pro and you had to pay Chatchy PT's premium subscription to get access to it. The other thing was just the product execution around chain of thought, which we've talked about a lot and I think is pretty well understood. But the fact that it showed you its thought process in real time was just super captivating. And now something that's become a step that every model takes.
像Chatchy PT那样,它已经被广泛使用,并且成为一种动词,这个机会已经被充分开发。而现在我们看到Deep Seek以极快的速度增长,并且它有几个有趣的特点。一个重要的特点是,他们将其推理模型大规模免费发布。在此之前,你需要使用O1 Pro,并且需要支付Chatchy PT的高级订阅费用才能访问它。另一个方面是关于思维链的产品执行,我们已经多次讨论过,这个概念已经被广泛理解。它能够实时展示其思维过程,这一点非常吸引人。现在,这已经成为每个模型都会采取的一步。
So I think it just really illustrates how early we are. You know, we as sophisticated users and investors are looking for further and further refinements. And once in a while something like deep seek comes out of the clear blue sky and just blows away all assumptions. That word specifically assumptions, I think is so key when you talk about these pivotal moments, I feel like you could actually match each pivotal moment with an assumption, like an assumption being, oh well, like AI could never trick me into thinking a picture is real when it's not right. Or I would never actually listen to a top 100 song that's generated by AI or you know, Chatchy PT is corner of the market. No one else can penetrate it, right? All of these assumptions that people are like, okay, sure, I was wrong about that prior one. But this one I'm pretty sure about.
所以我认为这确实说明了我们现在还处于非常早期的阶段。作为精明的用户和投资者,我们在寻找越来越多的改进。偶尔会有一些像深度搜索这样的东西突然出现,彻底打破所有的假设。特别是“假设”这个词,我认为在谈论这些关键时刻时非常关键。我觉得其实每一个关键时刻都可以与一个假设相匹配,比如人们可能会认为,哦,AI不可能骗我相信假的照片是真实的吧,或者我绝对不会去听一首由AI生成的Top 100歌曲,或者认为ChatGPT已经占据了市场,没有其他人能进入,对吧?所有这些假设,人们可能会说,好吧,我之前的那个假设是错的,但我对这个还是很有把握的。
We're seeing just like months, you know, being the delta between assumptions being broken. And so to your point on like the arc of the market or the industry, I could see an argument where people are like, oh, you know, we're actually pretty far along because we've already like slashed all of those assumptions. But on the other hand, I'm hearing, you know, we still have a long way to go. So kind of maybe put us along that arc if we were to compare to, you know, the mobile era or the cloud era or previous technology era. And you know, do you are we in that early innovator stage still or are we somewhere else?
我们发现,假设被打破的时间间隔就像几个月一样短。因此,关于市场或行业的发展轨迹,可以理解有人认为我们已经走了很远,因为我们已经打破了所有这些假设。但另一方面,我听到一些声音,认为我们还有很长的路要走。如果要将当前的情况与移动时代、云时代或以前的技术时代进行比较,我们处于发展的哪个阶段呢?是早期的创新者阶段还是其他阶段呢?
Yeah. I think we're still very much in the early adopter phase in many of these categories were kind of just, I mean, arguably still in like the infrastructure building era and kind of moving into the application building era. It depends on the modality. Like now LLMs are maybe people thought that was a solve problem. But then again, deep sea came in and upended all of that. There's a lot of things that are definitely not, you know, fully solved. Like AI video right now can generate great three or five or six second clips. But hopefully years from now, we have AI video that can generate minutes long or, you know, even hours long movies.
是的,我认为在许多领域我们仍然处于早期采用阶段。我们可能仍处在基础设施建设时期,并开始向应用程序开发阶段过渡。这要看具体的方向,比如现在大家可能认为大语言模型(LLM)的问题已经解决了,但后来又出现了新的技术将其颠覆。还有很多问题并没有得到彻底解决,比如现在的人工智能视频能够生成3到6秒的精彩片段,但希望几年后,我们可以看到人工智能能够生成数分钟甚至数小时的影片。
And so I think compared to where we're going to be, we're still incredibly early. You're here to assumptions that I think are interesting because it may turn out the reality is the exact opposite. One is that AI will be very good at transactional interactions. But humans will still be the ones to build relationships and connection. So an example of that would be, you know, what kind of phone calls are AI going to be best at. And I think the assumption was, well, they'll be great at sort of scheduling and logistics and the exchanging of information facts. But we've heard over and over that in many cases, the AI's are more human than humans. They just have more patience, more nuance. They're never having a bad day. They're never hung over. So that's an interesting area of exploration.
我认为,相比我们将要达到的阶段,我们现在仍处于非常早期。这里有一些假设很有趣,因为最终结果可能与这些假设完全相反。其中一个假设是,人工智能在处理事务性互动方面会非常出色,但人类仍然是建立关系与联系的主要角色。一个例子是,人工智能最擅长处理哪种电话交互。以往的假设是,人工智能在安排日程、处理物流和交换信息方面表现会很优秀。然而,我们一再听说,在许多情况下,人工智能比人类更具人性化。人工智能更有耐心,更富有细微差别,它们不会有心情不好的时候,也不会因为宿醉而状态不佳。这是一个值得探索的有趣领域。
You know, the other one that I think is interesting is the idea that humans will delegate work to the AI's and the AI's will do it. Like what if the AI's are the ones delegating the work to us? Perhaps AI is really good at organizing work and we're really good and also get a lot of joy out of doing it. So I think so many of these assumptions. And that's why the assumptions they seem intuitively correct are going to turn out to be incorrect. Totally. And if we think about the report, maybe one, what important data point is the fact that we see so many newcomers still, right? If we were in that later part of the innovation curve, you might expect more stagnancy. You might expect to see the same players.
你知道,我觉得另一个有趣的想法是,人类将工作委托给人工智能来完成,而人工智能会按要求去做。如果情况反过来,人工智能反而是那些给我们分配工作的角色呢?也许人工智能在组织工作方面非常擅长,而我们擅长并从中获得乐趣的是实际执行工作。我认为,很多的假设看似直观合理,但最终可能被证明是错误的。没错。如果我们来看报告,也许一个重要的数据点就是,我们还是能看到很多新来者,对吧?如果我们处在创新曲线的后期阶段,可能会预期更多停滞现象,看到的可能都是同样的参与者。
But every time you guys build out this report, we're seeing all of these newcomers in this particular time. The fourth report we saw 17 new companies on the web rankings in particular. And you actually have this quote where you say a few unexpected players rewrote the leaderboard overnight. So can you just speak to that and the movement that we're seeing? One of the biggest trends among the newcomers is we are finally on the verge of AI video starting to work to really work. So we had three new video models on the list this time. Highlo and Cling, which are both Chinese models. And then Sora, which was open AI's model that was announced, I guess more than a year ago at this point. And finally was released. I think we'll see even more of a shake up here because VO2 is the new Google model that is even next level beyond that from what we've seen in testing. And that is probably finally going to hopefully come out in the next three or six months.
每次你们编写这个报告时,我们都会看到这段时间涌现出一批新人。在第四份报告中,我们注意到特别是在网络排名中出现了17家新公司。你说过一句话,提到一些意想不到的玩家一夜之间改写了排行榜。你能就此谈谈我们所见到的变化吗?其中一个最大的新趋势是,AI视频终于开始真正发挥作用。这次榜单上出现了三个新的视频模型,分别是来自中国的Highlo和Cling,还有Sora,这是开放AI的一个模型,应该是在一年多前宣布的,现在终于发布了。我认为我们会看到更多的变化,因为VO2是谷歌的新模型,在我们测试中表现出超越当前水平的潜力,可能会在接下来的三到六个月内发布,希望如此。
The other big category of newcomers were these vibe coding products. Cursor made the list. It's more of like an agenteic ID for a technical audience. And then Bolt made the list, which is for a non-technical audience where you basically go from a text prompt to a fully functioning web app. Even though they made the list, I think we've still seen there's a really significant portion of their users that are people who are in tech and are actually technical. But they might be using something like a Bolt or a lovable, which made our brink list, which we can talk about to maybe prototype something easier and then export the code and go and play with it themselves.
另一个重要的新产品类别是这些“氛围”编程产品。Cursor上榜了。它更像是一个面向技术受众的代理ID。然后是Bolt,它针对非技术受众,通过输入文本提示可以生成一个完整功能的网页应用。虽然它们都上榜了,但我们仍然观察到实际上它们的用户中有相当大一部分是技术领域的人。不过,他们可能会使用像Bolt或是我们可以讨论的lovable这样的产品,以便更轻松地制作原型,然后导出代码,自己进一步进行修改和完善。
So I think we haven't quite seen the vibe coding products hit the true mainstream user in terms of someone who's never worked in tech or developed an app. I love this category. It's so fun and it's so satisfying to actually see your ideas come to life. I mean, in the case of Bolt and Lovable, sometimes they are just sort of compelling interactive prototypes. More than they are full fledged products. But that's usually enough to get a feel for whether this is something you want to invest deeper in. I mean, it sort of follows the trend of AI decreasing the cost of creation in every way. And people just trying more ideas. Just think about what that says about the untapped market of people who want to build things with code that this is on, you know, the top 50 list.
我认为我们还没有真正看到那些没有技术背景或从未开发过应用程序的普通用户广泛使用这种带有氛围的编码产品。我非常喜欢这个类别。它非常有趣,看着自己的创意成真真的是一种非常满足的体验。比如在 Bolt 和 Lovable 的情况下,它们有时候只是一些引人注目的互动原型,而不是完整的产品。但通常这已经足够让人感受到是否值得深入投资的潜力。这也符合了人工智能在各方面降低创作成本的趋势,人们尝试了更多的想法。想象一下,这说明了多少对编码创建事物感兴趣但尚未开发的市场,以至于它能跻身于前50名列表中。
And I think honestly, both of them haven't had many apps built on them yet that have gone super viral. And though when that happens, and I'm sure it will, those will become stories of their own, which will then increase awareness of the products of the true mainstream audience. I think we're going to see a really interesting diversity or range of products built on these, which it might just be like, this is my app that I just use for my very specific niche pain point, or there might be people who never learned how to code who want to build a venture scale product on something like a bolt or a lovable. And so seeing how that plays out will be very cool.
我认为,老实说,目前这两个平台上还没有许多应用程序能达到超级流行的程度。不过,当这种情况发生的时候——我相信一定会发生——这些应用程序将会带来自己的故事,从而提高大众对这些产品的认知。我认为我们会看到在这些平台上开发出各种各样的产品,从解决非常具体需求的小众应用,到不懂编码的人希望利用这些平台构建出具有商业潜力的产品。所以,观察这一切的发展将会非常有趣。
Yeah, I think there's two phrases I've heard that I like, you know, one is sort of DIY or personal software. Yeah, you know, it never made sense, economic sense to design software for one. Really? The other is disposable software, you know, just as Suno and UDO made it possible to make a song just to capture a joke that would be irrelevant the next day. These products make it possible to create a product or an experience that may have an extremely short shelf life like 20 minutes or a week or, you know, any other time period.
是的,我听过两个我很喜欢的说法。一个是关于“自己动手”或者“个人软件”。你知道的,过去为一个人设计软件在经济上从来没有意义。另一个是“一次性软件”。就像Suno和UDO这样的产品使得创作一首仅仅是为了捕捉一个笑话的歌曲成为可能,而这个笑话可能在第二天就没有人关心了。这些产品使得创造一种可能寿命极短的产品或体验成为可能,比如只有20分钟、一周或者其他任何时间段。
Let's talk about the brink list because that's completely new to this year's fourth generation. So what is the brink list and why at it? Yeah. So the brink list is essentially the five companies that almost made a list and were right below the cut off again purely based on the data. So we pulled the five websites and the five mobile apps. And I think honestly, we were just curious to see what it would capture. We didn't quite know. The takeaway for me, it does reflect how fast things are changing because there were a couple companies on the list, like Runway, Auto, UMACs across Web and mobile that have been on the core top 50 ranks in the past.
让我们来谈谈“前沿名单”,因为这是今年第四代里全新的内容。那么,“前沿名单”是什么,为什么要有它呢?简单来说,“前沿名单”就是那些几乎进入主要名单,但又刚好在入围线下的五家公司,这完全是基于数据得出的结果。我们提取了五个网站和五个移动应用程序。我想说,坦率地讲,我们当初只是好奇想看看这个名单能反映出什么。对我来说,最大的收获是,它体现了变化的速度有多快。因为名单中有几家公司,比如Runway、Auto、UMACs,它们之前都曾在网络和移动应用的核心前50名中出现过。
But maybe they got just edged out by like deep seek watching this time. And so they lost their spot for this ranking, but might be on there the next one and they still have massive usage. And then the other trend that it caught was a rise in more recent products like Cria made the list and lovable made the list that are very much on that kind of consistent upswing. And if it continues, we might see them on the main ranks and they haven't made the main ranks before. What did you predict that you would see on the list that you didn't really see there? Were there any surprises on that end?
也许这次他们只是被深度观看所超越了,所以在这次排名中失去了位置,但在下次排名中可能会出现,他们仍然有大量的使用量。另一个趋势是,一些较新的产品开始崭露头角,比如 Cria 和 Lovable 都上榜了,这些产品的使用量正在稳定上升。如果这种趋势继续下去,我们可能会在主要排名中看到它们,而它们之前从未进入过主要排名。你本以为会在榜单上看到什么没有出现的吗?有任何令你惊讶的地方吗?
So one thing I thought would we'd see more of is style transfer as an approach to scalable video? Because style transfer is just a much more tractable, tractable problem and has a lot lower cost of inference versus raw text to video. But you know, researchers and product developers seem to be really going for it on text to video. And we've seen more of that than I would have expected. I think the other things that we didn't see on this list that we have seen at the model level. So that means maybe they'll be on the next list or like consumer voice products. There are a few of them, but not a ton of them.
我原以为我们会更多地看到通过风格迁移来实现可扩展视频的方法,因为风格迁移相对来说是一个更易解决的问题,并且在推理成本上远低于原始的文本到视频转换。然而,研究人员和产品开发者似乎更倾向于直接进行文本到视频的研究和开发,比我预期的要多。我认为我们在这个列表中没有看到的一些东西,其实在模型层级是有的。这意味着它们可能会出现在下一个列表中,比如面向消费者的语音产品。目前市面上的这种产品有一些,但并不是很多。
Some of the new, like the Gemini Flash model that can see what's going on on your screen and interact with you. Like I built something to yell at me like if I go on Netflix or something. It's time to get back to work now. You've got this and can accomplish all of your goals. Or like the new OpenAI operator model which can actually interact with things on the browser level on your computer and get tasks done for you like pay a bill or you know make a graphic design or hire someone to landscape your yard something like that.
一些新的技术,比如双子座闪电模型,可以观察你屏幕上的情况并与你互动。我创建了一个程序,当我打开Netflix或者做其他不相关的事情时,它会提醒我:“该回去工作了。” 它鼓励我努力工作,实现所有目标。还有一个新的OpenAI操作员模型,这个模型实际上可以在浏览器层面上与你的电脑互动并帮你完成任务,比如支付账单、制作平面设计或者雇人帮你进行庭院美化,诸如此类的事情。
I think there's always a lag because the models have to be released to developers and they have to be tuned by the developers and so it takes a while. But I would expect to see maybe an explosion of fun and unique and interesting products built on models like that on hopefully the next list or two because it feels like we really have seen an explosion on the model side and it is right there in terms of manifesting at the app level too. So one of the examples of this is deep research which if you played with it is completely magical. But it's a primitive right it's not a product. It's something to build other things with.
我认为总是会有一些延迟,因为模型需要先发布给开发者,然后开发者还需要对其进行调整,所以这需要一些时间。但我预期或许在接下来的一个或两个名单中,会看到基于这些模型开发的有趣、独特和引人入胜的产品爆发。因为我们真的看到了模型方面的爆发,而在应用层面上,这种趋势也正在显现。其中一个例子是深度研究,如果你使用过的话,会觉得它非常神奇。但它只是一个基础模块,而不是真正的产品,它是用来构建其他事物的基础。
So it's really unclear if deep research is going to be used to you know write college thesis or as it can be used to find the perfect meme to match a joke you want to make. And that's all going to be up to the app developers. And just to double click on that because you could see maybe a world where you know deep research is just this like more broad horizontal application or you could see what you just described where developers are tailoring that to specific end use cases. Are you basically saying that you think the latter is more likely in terms of the progression of these these models and apps? Not more likely but I think it's under explored.
所以,现在还不清楚深度研究会被用来写大学论文,还是用来找到完美的表情包来搭配你想开的玩笑。这都取决于应用开发者的选择。详细来说,你可能会看到深度研究成为一种广泛应用的工具,也可能看到开发者针对特定的终端使用场景进行定制。你是在说你认为第二种情况在这些模型和应用的发展中更有可能吗?我并不是说更有可能,但我觉得这一领域的潜力仍未被充分发掘。
Yeah. If you come to deep research today you kind of have the blank page problem. Yeah. And I'd love to see developers you know create some constraints that leads to unexpected outcomes. Yeah. Like the known or the prescribed use of deep research right now is basically market research reports and it's amazing for that. I've used it for that a lot of times. But if you try other things like one day we were trying to trace the origin of a meme and deep research is like a 100x better version of that know your meme website that kind of like goes through the history.
好的。今天,当你开始进行深入研究时,可能会遇到所谓的"白板问题"。我希望看到开发者能够设置一些限制条件,从而引发意想不到的结果。目前,深入研究的常见或预定用途主要是市场调研报告,这方面它表现非常出色,我也多次这样使用过。但如果你尝试做点其他事情,比如有一天我们试图追溯一个网络迷因的起源,深入研究就像是那个"了解你的迷因"网站的加强版, 它可以详细分析历史,更加有效。
Yes. And like the etymology or however you're describing how I love that. I mean that should be an app. Yeah. So there's lots of other use cases that aren't market research reports that could really benefit from an incredibly obsessive compelling model that that will go and kind of read every website on the internet until it finds the answer. So those are the things that you know you thought might be on the list but you didn't actually see there.
好的。就像你描述的词源那样,我非常喜欢这种想法。我觉得这甚至可以做成一个应用程序。确实,有很多其他的应用场景不仅限于市场研究报告,会从这种超强的模型中受益匪浅。这个模型可以极其专注地到互联网上的每个网站寻找答案。那些是你可能认为应该在列表上的东西,但实际上并未找到。
What about the opposite? I mean I think the fact that the vibe coding products like the bolt and the cursors and loveables made the mainstream consumer list is just a testament to how widely they're used by the technical audience like they have gotten to saturation so quickly. I think Gary Tan had some tweet that like 95% of YC companies or something are now kind of building using those tools with something that every nearly every developer now is probably using which was maybe a surprise and how quickly we reach saturation.
那么反过来呢?我的意思是,像 Bolt、Cursors 和 Loveables 这样的 vibe coding(感觉编码)产品能够登上主流消费者的榜单,恰恰说明它们在技术用户中被广泛使用,并且迅速饱和。我记得 Gary Tan 发过一条推文,说大约95%的 YC(Y Combinator)公司或其他公司现在基本都在利用这些工具开发产品,几乎每个开发者都在使用这些工具,这可能让人感到惊讶,也说明我们达到了饱和的速度有多快。
We've talked about this but a continuing surprise so I don't know if it counts as one but I still am surprised every time is how many companion products are on the list and also how many of them rank so high. I think we had three companion products in the top 10. Two of them were NSFW oriented. Maybe not surprising when you think about like traffic on the internet in general outside of AI but a lot of people are even using them as like interactive fanfiction and some of the biggest fanfiction sites in the world are also you know top 100 top 200 global sites so it makes sense in that way.
我们之前谈过这个话题,但它仍然让我感到意外,所以我不知道它是否算是一个惊喜。我每次都会感到惊讶的是,有这么多"伴侣"产品在名单上,而且其中很多产品排名非常高。我记得我们在前十名中有三个"伴侣"产品,其中两个涉及成人内容。当你考虑到整个互联网的流量情况时,也许这并不奇怪,很多人甚至把这些产品当作互动性的"同人小说"来使用,而一些最大的同人小说网站在全球网站中也排在前100或前200名,所以从这个角度来看,这是有道理的。
And then I guess my last surprise would be there's actually quite a bit of consistency in the list over the past four versions. There's always new entrance which is really exciting but across the four list there's now 16 companies on the web ranks who have made it every single patent and have kept the street going which is pretty remarkable when you think of how early we are in AI but I think a testament to how those companies have cemented their brands their products their kind of I guess status and consumer consciousness and I think a testament to the fact that like real businesses have been have been built in consumer AI already.
然后,我想我最后一个感到意外的地方是,在过去的四个版本中,这个名单实际上有相当多的一致性。虽然总有新的入围者,非常令人兴奋,但在这四个名单中,有16家公司每次都进入了网络排名,并且一直保持着这一趋势。这相当了不起,尤其当你想到我们在AI领域还处于早期阶段。我认为,这也证明了这些公司如何巩固了他们的品牌和产品,以及他们在消费者心中的地位。我认为这也证明了在消费AI领域,真正的业务已经建立起来了。
No to add to that one of the surprises for me on companion was not seeing more multimodality. Yes. The kind of the first glimmers of that at scale were GROC you know GROC added a bunch of voices with some real aesthetics and points of view. Yeah but it's look it's it's just interesting that that feels in it of course characters got voice mode and you know and more but it feels like character is in companionship is such a horizontal category there's so much latent demand and a really increase once you have multimodality.
对于我来说,"伴侣"项目的一个惊讶之处是没有看到更多的多模态功能。是的,最早的规模化多模态应用可能是GROC,你知道,GROC引入了一堆具有真实美感和观点的声音。是的,不过这真的很有趣,"伴侣"角色具备了语音模式等等,但感觉角色在伴侣方面是个横向发展的类别,一旦引入多模态,就会有大量潜在的需求和真正的增长。
You know the other interesting thing is that a lot of the text to code work my assumption was that there was a small number of people who are creating sites that were heavily traffic and that explained the rise of them but actually the majority of the traffic correct me if I'm wrong here Olivia is from people doing creation not just consuming other people's creations so there's just it really shows how much demand there is to make things even if people are not that interested in consuming them.
你知道,另一件有趣的事情是,在使用文本生成代码方面,我原以为是少数人在创建那些访问量很大的网站,这解释了它们的增加。但实际上,如果我说错了请纠正我,Olivia,大部分的流量来源于人们进行创作,而不仅仅是消费别人的作品。这真的显示了,即使人们对消费其他人的作品兴趣不大,对创作的需求却是多么旺盛。
Yeah you can track the traffic of like apps that people have launched on lovable.app versus visits to lovable.dev which is where people go to make a lovable product and like lovable.dev has more usage. Significant. Significant. Then you know traffic to lovable.app which gets back to what I was saying before like we have not even seen the first wave of viral products built on top of lovable and bold and and so when that happens I think the awareness of these types of platforms is going to go significantly up show.
是的,你可以跟踪人们在 lovable.app 上启动应用的流量,相比而言,人们访问 lovable.dev 的次数更多,lovable.dev 是大家用来创建受欢迎产品的网站,而且使用情况明显更多。就像我之前说的,与 lovable.app 的流量相比,lovable.dev 的流量更大。我们还没有看到基于 lovable 和 bold 平台的首批热门产品的诞生。当那些产品出现时,我认为人们对这些平台的认知度会显著提高。
I mean the app store is going to be chaos. Yeah it is going to be chaos. We don't need an AI just to solve that AI app management problem. Completely and I mean to that and you talked about the fact that there are some consistent players. Yeah one of those players is chatgbt which we you know we we've talked about it was kind of like the starting gun of some of this application development.
我的意思是,应用商店将会变得一片混乱。是的,它将会很混乱。我们不需要一个 AI 来仅仅解决这些 AI 应用管理的问题。完全同意,而且你提到了一些稳定的参与者。是的,其中一个参与者是 ChatGPT,我们已经谈过,它有点像是这些应用开发的起跑枪声。
Chatgbt has been at the very top of the list. Has it been that way for every single iteration of web and mobile. But maybe what would surprise people is that the traffic to chatgbt hasn't always been the same trajectory. So maybe can you talk about that and what did we see this time around?
Chatgbt一直处于名单的最顶端。每次网络和移动设备的更新迭代中都是如此。但可能让人惊讶的是,Chatgbt的流量并不总是按照相同的轨迹发展。那么,可以谈谈这个问题吗?这次我们看到了什么情况?
Yeah so it was basically flat for a while which I think was surprising to a lot of people between February 2023 basically for a whole year through February 2024. It was essentially flat in monthly visits to the website and I think like at that point from the data that I've seen basically 50% plus of the traffic with students who were using it for essays or homework problems but the vast majority of other people me included to the honest had not maybe found a daily active use case for chatgbt yet and it's completely researched more recently.
是的,所以从2023年2月到2024年2月,网站的月访问量基本上保持平稳,这对很多人来说可能有点意外。从我看到的数据来看,当时大约50%以上的流量来自学生,他们用这个网站来写作文或解决作业问题。但包括我在内的大多数其他用户可能还没有找到每天使用ChatGPT的实用方式。不过,最近这种情况已经完全改变了,使用情况有了新的变化。
So they two x the number of visits on web since then they actually made their own announcement to where they counted across web and mobile and in the past six months they grew from 200 million to 400 million weekly active users. Wow which is especially surprising because it took them nine months to double before that and it usually gets way harder to double at scale not easier.
自从他们自己发布公告以来,他们实际上统计了网站和移动端的访问次数,从那时起,他们将网站访问量增加了一倍。在过去的六个月里,他们的每周活跃用户数从2亿增长到了4亿。哇,这尤其令人惊讶,因为在此之前,他们用了九个月的时间才实现翻倍,通常情况下,在规模变大后,实现翻倍会变得更加困难而不是更容易。
I think from our perspective and if you've even plotted on the graph you can kind of track the increases to the release of new models that unlock new use cases. So like the new 01 reasoning models, the 4-O models which were kind of multimodal for the first time and then advanced voice mode and then they've also watched new products like the operator that can perform tasks in your computer like canvas where you can write more naturally.
从我们的角度来看,如果你在图表上绘制数据,你就可以追踪到随着新模型推出而出现的用途增加。例如,新的01推理模型,以及首次多模态的4-O模型,还有先进的语音模式。此外,他们还推出了新的产品,比如"操作员",它可以在你的电脑上执行一些任务,比如在画布上更自然地书写。
So it's both bringing in like new users who never tried it and then taking people like me who honestly I was maybe a weekly if not less than a weekly active use case. And now I'm a daily active but across several use cases now. Like some days I'm driving and talking to a voice mode. Some days I'm working on a memo and I'm generating something with the research. Some days I'm doing you know some random other project and I'm brainstorming ideas with it.
所以这不仅吸引了从未尝试过的新用户,还吸引了像我这样的人。老实说,我过去可能是每周使用一次,甚至更少。但现在,我每天都在使用,并且在多个场景中使用。比如,有些日子我在开车时用语音模式交流,有时我在写备忘录并用它来生成一些研究资料。有些时候,我在做其他项目并用它来头脑风暴创意。
So I would expect that to continue as they release new models. And have you heard from the ecosystem in terms of what more frequent use cases have emerged kind of like yours in terms of if before it was a lot of students writing research reports. Are there now, is there a sense of understanding of what those newer use cases are? Yeah I think it's gotten better at some things related to coding. It's gotten better at data analysis. And then I mean the reasoning models it's hard to overestimate because in the past like you couldn't even rely on Chatchee. We need to tell you how many hours were in strawberry. Right. Accurately. So it was hard to feel good about really tasking any sort of delicate or serious work to it. And so I think there are probably a long tail of use cases that people have just migrated over now that they have more confidence in the models.
我预计随着他们发布新模型,这一趋势会继续。对于新的使用场景,你有没有听说过,就像之前有很多学生在用它写研究报告,现在是否有了对这些新应用场景的更清晰理解?我认为在一些与编程相关的事情上,它变得更好了。它在数据分析上也有了进步。就推理模式来说,以前你甚至不能指望人工智能准确告诉你关于草莓的问题。对吧?准确率太低了。因此,人们很难放心地将任何精细或者重要的任务交给它完成。所以我认为现在随着人们对模型的信心增加,可能有很多新的使用场景慢慢被开发出来。
You know what's interesting to add to that is that Quad is not a traditional number two player. And typically the number two player has 10% of the market share and you know and just sort of 10% of the product quality. And instead Quad sits in this very interesting place where it seems like it's more beloved by a smaller number of people. It's better at creative writing. You know it seems to have more of a personality which is interesting because at least I think it's designed to be more constrained. Yeah. And then it's also strangely much much better at coding. Yes.
有趣的是,Quad 并不是传统意义上的“老二”。一般来说,市场排名第二的公司通常会占据10%的市场份额,产品质量也往往只是平均水平。然而,Quad 的情况却非常独特,它似乎更受一小部分人的喜爱,尤其在创意写作方面表现更出色。令人感到有趣的是,尽管 Quad 被设计得更受限一些,但它似乎拥有更多的个性。另外,令人惊讶的是,Quad 在编码能力上也远远优于预期。
Yeah. Why? I don't know but it's very interesting to see there's a place for both Chatchee PT and Quad and Mistral and potentially other models all to sort of augment each other. To me the really interesting thing about this list when it came to general LLM assistant usage was like we only had 10 days of data for deep seek for January because it launched at the end of the month and it shot up from literally nothing to number two on the list. You know 10% of Chatchee PT scale on web within a week a little bit more than a week on mobile. It had even less than that five days and it was number 14 and if it had had five more days it would have been number two.
是啊。为什么呢?我也不太清楚,但看到 Chatchee PT、Quad 和 Mistral 以及可能的其他模型可以相互补充,这很有趣。对于我来说,这份列表中真正有趣的是在通用大语言模型助手使用方面,我们对于一月份的新推出的深度搜索只有10天的数据,因为它是在月底上线的,它从无到有迅速跃升到榜单第二位。你知道,在一周多一点的时间里,它在网络上的表现达到了 Chatchee PT 规模的10%,而在移动端,它只用了不到五天的时间就排到第14名,如果再给它五天,可能就已经是第二名了。
And the gap is even narrower there between deep seek and Chatchee PT. So again to an issue's point like that was a surprise and that we could see kind of a broad base cell product go so viral still and capture so many users. Yep. And deep seek was obviously the story when it came out. What have we learned about retention since then? And is that learning specific to deep seek or are we seeing that learning applied across the ecosystem? On a retention basis like how many users are coming back to the app say exactly 30 days exactly seven days exactly 60 days it's just slightly below Chatchee PT.
在那里,深度搜索(Deep Seek)和ChatGPT之间的差距更小。因此,就像某个问题所指出的那样,这令人感到意外,我们看到一种面向大众的基础产品仍然能迅速走红并吸引大量用户。没错。当深度搜索刚推出时,它无疑成为了焦点。自那时以来,我们在用户留存方面学到了什么?这种学习经验是深度搜索特有的,还是我们看到这些经验在整个生态系统中得到了应用?就用户留存率而言,比如在30天、7天或60天后回到应用的用户数量,深度搜索仅略低于ChatGPT。
So we're looking at 7% day 30 for deep seek and 9% day 30 for Chatchee PT. It's too early to call on web because it's kind of hard to track usage. My part of my theory here is if you look at deep seek usage a lot of it is the US but a lot of it is China and other countries where Chatchee PT is either is basically you can't use it or they try to make you not use it and you can only get by it with a VPN. And so in those markets it's not Chatchee PT versus deep seek versus perplexity it's like deep seek versus nothing. And so in those markets I think they have like structural a structural advantage from the retention side that might like skew the overall sample.
我们这样看:在第30天时,Deep Seek 的留存率是7%,而Chatchee PT是9%。对于网页端的数据,现在下结论还为时过早,因为跟踪使用情况比较困难。我有个理论,观点是如果你查看Deep Seek的使用情况,大部分用户在美国,但也有很多在中国和其他无法使用或不鼓励使用Chatchee PT的国家,或者需要通过VPN才能使用。在这些市场中,并不是Chatchee PT与Deep Seek或Perplexity的竞争,而是Deep Seek几乎没有竞争对手。因此,我认为在这些市场中,Deep Seek在用户保留率方面有结构上的优势,这可能会影响整体样本的数据。
Totally. Next time we should add a different cut for deep seek USA. Yes exactly. Yeah yeah geographic breakdown. Yeah talking about trends that we're seeing on the list you mentioned AI video before but anything else you want to call out there in terms of its presence on the report? Yeah I mean two of the video models were Chinese video models which is super interesting. The models are less copyright sensitive in their training data.
当然。下次我们在为美国市场做深入分析时应该增加一个不同的切入点。对,没错。是的,是的,进行地域上的细分。对,谈谈我们在你提到的名单上看到的趋势吧,你之前提到过AI视频,但在报告中你还想指出些什么吗?是的,我的意思是,其中两种视频模型是中国的视频模型,这非常有趣。这些模型在训练数据方面对版权的敏感度较低。
And it's a great euphemism. Yeah there are maybe more realistic and more prompted here and in the outputs as a result but also just in China it's easier to hire people to kind of capture videos. They have maybe a greater volume of researchers doing image and video stuff versus other stuff. So that makes sense and I think we might expect to continue to see I think Sora in some ways was a little bit disappointing for some people and whereas like the Chinese video models were maybe better than a lot of people expected given the relative lack of capital that they've raised.
这是一个很好的委婉说法。是的,这里和输出的内容可能更真实和及时,但在中国,找人拍摄视频相对容易。或许因为中国在图像和视频方面的研究员数量更多,相比于其他领域的研究员数量更大。这是可以理解的,我认为我们可能会继续看到这样的趋势。我觉得Sora在某些方面让一些人有点失望,而中国的视频模型可能比很多人预期的要好,尤其考虑到他们筹集的资金相对较少。
That's right I think an interesting trend is just seeing Korea in the brink list. Yes. Korea is the single best place to access all the models and all the tools and the nice thing that they do is stitch all of these things together to make them greater than some of their parts. So insofar as we live in this sort of multi-polar world of models, image models, video models, language models, they'll be a role for aggregators like Korea to put the model together in a pot totally. Especially because people who are deep in AI video understand this but each model is kind of known for being good at specific things like shots of people, shots of landscapes, anime, hyper realistic and so paying it can it can rack up very quickly on $20 a month's subscriptions if you're paying for 10 or 15 different models independently versus like having one canvas to work with all of them. You also typically use the products together.
没错,我认为一个有趣的趋势是看到韩国在前沿发展名单中。是的,韩国是获取各种模型和工具的最佳地点。他们的一个优点是能够将所有这些工具整合在一起,使其整体效果超过单个部分的总和。在这个多极化的世界中,包括图像模型、视频模型、语言模型等,各种模型都有各自的优势,而韩国这样的聚合器将起到重要作用,能够将这些模型完美结合。特别是因为深耕AI视频领域的人都知道,每个模型在某些特定方面,比如人物、风景、动画、超写实等,都有很好的表现。如果你独立付费订阅10到15种不同模型,每个月可能很快就要花费20美元,而通过一个平台你可以同时使用所有的工具,这样效率更高,而且通常产品是结合使用的。
Yes. You know, usually generate an image in mid-June or flux and then you take that image and upscale it and then you put it as the beginning frame in a video. So you really want to like not have the seams between all those products. Completely. Are we seeing these video models in particular become more opinionated and what I mean by that is like you know we see that in image models right where sort of mid-June might be good at this and then you might see another model better at something else and people the users will gravitate towards either models or applications that provide them with that specificity or a piece. Are we seeing that video?
是的。通常情况下,你会在六月中旬或变化时生成一张图像,然后把它放大,并作为视频的开头帧。因此,你真的希望各个产品之间没有不协调的地方。完全没错。我们是否看到这些视频模型变得更加独特?我的意思是,在图像模型中,我们可以看到一些特定的模型在某些方面表现得特别出色,而另一些模型则在其他方面更好,用户往往会选择那些能提供特定功能或风格的模型或应用。我们在视频领域也看到这种情况了吗?
Yeah. I'd say they're both becoming more opinionated on the model level but also the applications. The application choices that they're making that even the model companies are making are becoming more opinionated. If you've used like a runway or a clean or something you can now prompt basically the camera angles or the wideness of the shot or all of these things a human cinematographer would do you can prompt how the video kind of sweeps over the surface of the screen and so that's also kind of a big factor in what you use for maybe even different parts of one video which is interesting. I mean the comments specifically I still think Audiogram is one of the most unique models for sort of what it does, what it's great at which is text generation.
好的。我会说,现在无论是在模型层面还是应用层面,它们都变得更加有主见。就连模型公司在选择应用时也明显带有更多个人偏好。如果你使用过类似runway或clean这样的应用,现在你基本上可以通过提示来控制镜头角度、画面的广度等这些通常由人类摄影师决定的事情。你还可以提示视频如何在屏幕表面扫过,这对你在一个视频的不同部分中选择使用什么非常有影响,这是很有趣的。我认为,Audiogram这个模型在某种程度上仍然是最独特的,因为它在文本生成方面非常出色。
It's really aesthetic that it has. It just sits in a very unique place in the ecosystem. Yeah. We did an internal competition where we had to generate a bunch of video, a 30-second video and iDgram was amazing for that because you could not get that layer of specificity anywhere else and then you could then take what was generated at iDgram and put it into another model to animate it or to swerve or to do whatever you needed to do. Well they also have a fun feature which essentially is image to text so that you have a meme or a copyrighted image that you want to replicate or at least be inspired by.
它确实很有美感,处于生态系统中一个非常独特的位置。我们进行了一场内部比赛,需要生成大量视频,其中一段是30秒的视频,而iDgram在这方面表现出色,因为只有它能提供这种特定的层次,然后你可以将iDgram生成的内容放入另一个模型中进行动画、旋转或进行你需要的其他操作。它还有一个有趣的功能,就是可以将图像转化为文本,这样如果你有想要复制或从中获取灵感的表情包或受版权保护的图片,就可以轻松做到。
Yeah. You can use their image to text and then use that text as the prompt to create a image. I also found that fascinating because I would prompt something and as you learn when you're prompting with AI in general you learn that you don't know what you're looking for. Yes. And so when I was prompt iDgram would modify your prompt yeah or generating the image and then you could actually go and interrogate that and be like oh that's why I'm getting xy or z. Video actually in general it tends to be more of a mobile first phenomena right we see tons of even before AI tons of applications that focus on creators being able to edit and splice video.
是的,你可以用他们的图像转文字功能,然后用这些文字作为提示来创建图像。我发现这很有趣,因为我会输入一些提示,随着你在一般AI环境中输入提示的经验,你会意识到自己并不知道自己在找什么。是的,所以当我输入提示时,iDgram会修改你的提示来生成图像,然后你可以查看并分析它,发现自己为什么会得到某个结果。在视频方面,一般来说,它更像是一种移动优先的现象。即使在AI出现之前,我们也已经看到很多专注于让创作者能够编辑和剪辑视频的应用程序。
What are we seeing in terms of the difference between what's working on mobile and what's working on desktop? Yeah. I mean it's somewhat obvious but like a lot of the things that are working on mobile are either things you want to use on the go or where the asset the underlying asset you're working with is easily captured by the phones. The avatar apps will open mobile because you have you know 10 selfies of yourself sitting on your phone. A lot of the voice first consumer products that we are seeing working actually are on mobile versus web because it's easier and more natural to talk into your phone for a language learning or for companionship or other use cases than it is to maybe talk into your laptop and same with homework helper apps like those are really blown up on mobile as compared to web.
我们在分析移动端和桌面端之间的差异时看到了什么?嗯,这有点显而易见。很多在移动端有效的东西,或者是我们希望在路上使用的,或者是可以很容易用手机捕捉到的资源。举例来说,头像应用程序在移动端运行良好,因为你的手机里可能有很多自拍照。我们发现,很多主要以语音为主的消费产品在移动端比在网页端更成功,因为在手机上进行语言学习或陪伴聊天等用途时,通过手机交流显得更自然、更便捷。作业助手类的应用也是如此,在移动端的流行程度远超网页端。
So maybe another interesting breakdown that kind of represents where we are in the innovation curve is not just what is getting views but what's actually making money and how those aren't always one to one mirrored. What is making money today? Yeah. We're relearning there and is that the same as you know what's getting traffic. So for the first time we actually ranked the top 50 by what sensor tower can measure as mobile revenue which is typically in app purchases and subscription so probably not ads and we ranked those separately from what has the most monthly active users and there was only 40% overlap between the two lists. So a lot of difference.
或许另一个有趣的视角可以展现我们在创新曲线中的位置:不仅仅是看什么内容获得了关注,还要看哪些内容实际上在赚钱,并且这两者并不总是直接对应的。那么,今天到底是什么在赚钱呢?是的,我们在重新学习这一点,这是否与我们所知的流量来源相同呢?首次我们根据Sensor Tower能够测量的移动收入(通常是应用内购买和订阅,而可能不是广告)对前50名进行了排名,并将其与月活跃用户最多的排名进行了区分。结果这两份名单只重合了40%。所以两者之间存在很大的差异。
The surprise to me actually was the main categories are the same in terms of what's making money versus what people are using. So photo and video generators, photo and video editors, beauty filters and beauty enhancer is massive standalone category and then the realm of kind of like chat GPT copy cat apps both making a ton of money getting a lot of users but the companies within those categories are very different in terms of who's making money and who has the most usage.
令我感到惊讶的是,实际上赚钱和用户使用量最多的主要类别是一样的。比如,照片和视频生成器、照片和视频编辑器,美颜滤镜和美颜增强功能已经成为一个巨大的独立类别,然后还有类似于ChatGPT的聊天应用,这些应用赚了很多钱,也吸引了大量用户。不过,在这些类别中,不同公司在赚钱能力和用户数量上存在很大差异。
We actually found we plotted like revenue per user versus number of users and we found the apps that had smaller user bases out of the sample set were much more likely to be making significantly more money on a per user basis. So apps like speak apps like auto captions and video editing. There's a lot of reasons for this. One is that if you are making a lot of money per user you're probably more of a serious prosumer app and so you've probably actually gated the usage pretty significantly like you have to subscribe to use the product and so there are companies on here that might be making 50 a hundred million dollars an ARR off of only a million users 2 million users so they wouldn't make the ranks ironically enough for monthly active users but they rank really really high on a revenue basis which is exciting.
我们实际上绘制了一个图表,将每位用户的收入与用户数量进行了对比。结果表明,在样本集中,用户基数较小的应用每位用户的收入有可能显著更高。例如,一些语音应用、自动字幕和视频编辑应用。这里面的原因有很多。其中之一是,如果你每位用户赚的钱很多,那么你可能是一个比较专业的高端消费者应用。因此,你很可能对使用进行了较严格的限制,比如必须订阅才能使用。因此,有些公司可能只有一百万到两百万的用户,但年经常性收入却达到了五千到一亿美元。因此,这些应用在月活跃用户数排行榜上可能名次不高,但在收入排名上却非常靠前,这让人感到兴奋。
And then as anyone who looks at the mobile list knows there's a lot of maybe for the tech audience seemingly random products on there of like I've never heard of this is this from a startup and on mobile especially there is a very precise game that you can play with like app store ads and and other kind of paid but fairly low cost acquisition channels and if you're doing this as an indie developer or maybe an app studio running internationally you're not looking for the 10x payback of acquisition costs that we might be looking for as venture investors so if you make back one or two extra money on a user that's amazing so you can get to 10 million users mostly by paying for them but you're probably not going to make as much revenue or ultimately as much profit maybe as as some of the companies that are lower usage but higher revenue and is there a learning there in terms of you mentioned how I mean by nature if you start getting certain features or an application entirely yeah you are potentially stifling growth of the overall user base is there a learning in terms of how AI founders should be thinking about that trade off today.
然后,任何查看移动应用列表的人都知道,对技术观众而言,那里有很多看似随机的产品,可能会让人觉得这是哪家初创公司推出的?尤其是在移动领域,你可以在应用商店广告和其他相对低成本的获客渠道上进行非常精准的操作。如果你是作为独立开发者或者一个在国际上运行的应用工作室,你不需要像风险投资者那样追求10倍的投资回报率。如果你能从每个用户身上赚回一到两倍的成本,那就很棒了。这样你可以主要通过付费获客的方法达到一千万用户,但你的收入或者最终的利润可能没有那些用户量少但收入高的公司那么多。关于你提到的某些功能或者整款应用限制了整体用户数量增长,这方面有没有什么经验可以给AI创始人一个启示,让他们知道该如何看待这种权衡?
I mean I think it depends some of these markets are naturally maybe not mainstream behavior like one example of a category that did appear on the mobile revenue list but was not on mobile usage was several plant identification apps I love those yeah well you take a picture you saved on the plan it tells you exactly what it is if you've seen that plant before is that an app that a hundred million people will have on their phones maybe maybe not but if you're one of the like I can think of a few relatives who love plants or love birds and like totally they'll pay a hundred dollars a year for that and they'll use it every day or every other night so I think it's more for founders kind of optimizing for the type of product you have and how mainstream it can be.
我认为这要视情况而定。某些市场的行为可能不是主流,比如有一种类别出现在移动收入榜单上,但在移动使用率上却没有,那就是一些植物识别应用。我很喜欢这些应用,你只需要拍张照片,它就能告诉你这是什么植物,甚至还能告诉你之前是否见过这种植物。这样的应用会不会有上亿人安装在他们的手机上呢?也许会,也许不会。但是对于一些喜欢植物或鸟类的亲友,他们绝对愿意每年花一百美元来使用这个应用,并且每天或隔天都会使用。所以我认为这更需要创业者根据产品的类型来优化,看看该产品是否能够成为主流。
All right so there's a lot of information here that we've covered we've covered desktop we've covered mobile we've covered revenue versus users yeah and then we've also talked about the stickiness of some of these players right you said there was what was it 16 that have showed up yes every single list so what can we learn from the last few lists feeling the biggest thing now being consumer investor for close to a decade now it's almost like the more you know the less you know in some cases because it all just comes back to the product at the end of the day like technologists or investors or can have opinions on the best monetization strategy or the best you know growth hacks but in the end if the product isn't capturing users attention and isn't retaining them the business is just going to be kind of a completely like leaky bucket of users and users out.
好的,我们已经讨论了很多信息,包括桌面、移动设备、收入与用户的关系。我们还聊到了这些玩家的粘性,你提到有16个一直在榜单上。那么,我们可以从最后几个榜单中学到什么?
作为接近十年的消费者投资者,现在最大的感受是:有时候你知道得越多,反而会觉得知道得越少,因为最后一切都归结到产品上。无论技术专家或投资者对最佳的盈利策略或增长策略有何看法,但如果产品不能吸引用户的注意力和留住他们,业务就会像个漏水的桶一样,用户进来又流失出去。
So I would say the biggest like often we meet with these amazing like PhD researchers best in class in the whole world in terms of their technical understanding of a model or capability and they can struggle building in consumer sometimes because often the more complicated. thing is not actually the thing that is highest utility most delightful most helpful to a consumer user so we always we never like to be prescriptive on consumer products but in general we see when teams focus on either the pain point they're trying to solve or the unique experience they're trying to create and build towards that and if that means your actually the old model is better than the new model use that if that means it's just one AI feature instead of the whole product being built on AI because it's not stable enough like do that.
所以我认为,我们经常会遇到一些在技术理解方面堪称世界顶尖的出色博士研究人员,他们对模型或能力的技术理解极其深刻,但有时候在为消费者开发产品时却会遇到困难。这是因为通常情况下,最复杂的东西并不一定是对消费者来说最有用、最令人愉悦或最有帮助的。因此,我们在设计消费者产品时不喜欢过于指令化,但一般来说,如果团队专注于他们想要解决的问题或想要创造的独特体验,并围绕这些进行开发,就会获得更好的结果。比如,如果旧的模型比新的模型更好用,那就使用旧的。又比如,哪怕只是将一项AI功能应用于产品而不是全部依赖AI,以保持产品的稳定性,那也不失为一个好的选择。
I think in in consumer you really have to kind of let the data the data your guide there. Thank you so much for listening to the A16C podcast. If you've made it this far don't forget to subscribe so that you are the first to get our exclusive video content or you can check out this video that we've can selected for you.
我认为在消费者领域,你真的要让数据成为你的指南。非常感谢你收听A16Z播客。如果你能听到这里,不要忘记订阅,这样你就能第一时间获得我们的独家视频内容,或者你也可以查看我们为你精选的视频。