Intelligent Money
发布时间 2023-04-12 00:46:00 来源
摘要
Fintech has perhaps the most to gain from artificial intelligence technology. Even slight improvements in forecasting default rates on a loan or cash flows of a business can have a dramatic economic impact. But for the most part, fintech has been left out of the conversation, partly because there is low margin for error in a regulated space. Encouragingly, this is starting to change. Ramp, a fintech startup that is noted for being one of the fastest-growing companies ever to hit $100M in ARR, is among the fintech frontrunners using automation and machine learning to help customers with expense management, payments, reporting, and more. The upside of automated, intelligent, personalized, and more secure financial services with the help of AI is in reach. Greylock investor Seth Rosenberg spoke with Ramp CEO Eric Glyman and Greylock's Reid Hoffman for a wide-ranging discussion on how AI is impacting every profession today – and how there is considerable room for it to impact financial services in the future.
You can watch the video of this interview here: https://youtu.be/q5Rhetnw_64
You can read a transcript of the interview here: https://greylock.com/greymatter/intelligent-money/
GPT-4正在为你翻译摘要中......
中英文字稿 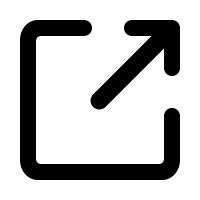
Hi everyone, welcome to Gray Matter, the podcast from Graylock where we share stories from company builders and business leaders. I'm Heather Mack, head of editorial at Graylock.
大家好,欢迎来到Graylock的Gray Matter播客,我们在这里分享公司建设者和商业领袖的故事。我是Graylock的编辑部负责人Heather Mack。
Today, Graylock investor Seth Rosenberg leads a discussion on opportunities bringing AI into Fintech. Seth spoke with fellow Graylock investor Reid Hoffman, an Eric Gliman, who is the CEO and founder of Fintech startup Ramp, while machine learning has been around for decades.
今天,Graylock的投资者Seth Rosenberg主持了一场讨论,探讨将AI引入金融科技领域的机会。Seth与同为Graylock投资者的Reid Hoffman以及金融科技初创公司Ramp的CEO和创始人Eric Gliman进行了对话。虽然机器学习已经存在了数十年,但仍然存在许多机会可以将其引入金融科技领域。
The recent advancements in large language models and the launch of ChatGPT has created a Cambrian explosion of applications and investor interest. AI has quickly become the enailing technology of our time, and it's impacting nearly every industry Graylock invests in.
最近大语言模型的进步和ChatGPT的推出引发了应用和投资者兴趣的寒武纪爆发。人工智能迅速成为我们这个时代的关键技术,影响着Graylock投资的几乎所有行业。
Financial services represents 25% of the global economy and has perhaps the most to gain from better prediction models. Even slight improvements in forecasting default rates on a loan or casual of a business can have dramatic economic impact. But so far, Fintech hasn't left out of the conversation, partly because of the low margin for error and a regulated space.
金融服务占全球经济的25%,并且可能从更好的预测模型中获得最多的利益。即使是对贷款违约率或企业出现罕见情况的预测略有改进,也可能带来巨大的经济影响。但是到目前为止,由于误差较小且受监管空间的限制,金融科技在这一谈话中并未被排除在外。
But these problems are being solved quickly. Just recently, Bloomberg announced Bloomberg GPT, a large language model trained on clean financial data. The upside of automated, intelligent, personalized, and more secure financial services with the help of AI is in reach. And Ramp has become a leader in the space.
但这些问题正在迅速解决。就在最近,彭博社宣布了彭博GPT,这是一个基于清洁的金融数据训练的大型语言模型。在AI的帮助下,自动化、智能化、个性化和更安全的金融服务的优势已经实现。Ramp已成为该领域的领导者。
Seth spoke with Reid and Eric to better understand how AI is impacting every profession today and how it could further impact financial services. This conversation was recorded in front of a live audience of founders, investors, developers, and technologists. You can watch the video of this interview on our YouTube channel and you can read a transcript on our website. Both are linked in the show notes.
塞斯与瑞德和埃里克交谈,以更好地了解人工智能如何影响今天的每个职业以及如何进一步影响金融服务。这次谈话是在创始人、投资者、开发人员和技术专家的现场观众面前录制的。您可以在我们的YouTube频道上观看这次采访的视频,并在我们的网站上阅读转录。两者都在节目备注中链接。
Now here's Seth Rosemberg, Reid Hoffman, and Eric Glamon. Hey everyone, welcome. Thank you so much for taking some time out of your Friday evening to spend some time together. The joke is that every Fintech investor is now an AI investor, but obviously at Graylock we're investing in Fintech and AI, and we're also investing in the New York community. And so I thought this would be a good opportunity to bring everyone together.
现在是Seth Rosemberg、Reid Hoffman和Eric Glamon。大家好,欢迎来到这里。非常感谢你们抽出时间在周五晚上一起度过。有一个笑话是每个金融科技投资者现在都是人工智能投资者,但是在Graylock,我们投资于金融科技和人工智能,同时也投资于纽约社区。所以我想这是一个很好的机会把大家聚在一起。
The space is moving so quickly, right? And we're lucky to have people like Reid and Eric and Kevin and everyone else in this room who are kind of in the middle of this. So with that, I mean, I don't think I'd ever be really into the intro. Obviously, Reid Hoffman, Eric Glamon, to your own founder of Ramp. But let's get into it.
空间运动得非常快,是吧?我们很幸运有像Reid、Eric、Kevin和在这个房间里的其他人这样的人,他们处于这个运动的中间地带。因此,我觉得自己永远不可能真正喜欢介绍。显然,Reid Hoffman、Eric Glamon和你自己都是Ramp的创始人。但是,让我们深入了解一下。
So Reid, I wanted to start off by for you to just kind of introduce the topic. We've been investing in AI for a long time, but there seems to be this explosion over the last six months of investor interest, applications. So what's going on?
Reid,我想先请你来简要介绍一下话题。我们已经投资了很长时间的人工智能,但是在过去的六个月里,投资者的兴趣和应用似乎出现了爆炸式增长。那么,是怎么回事呢?
Give us a kind of state of play. Look, macro frame is what's really going on here is the application of scale compute to create interesting computational artifacts. We began to see that in the earlier stages with things like AlphaGo, Alpha0, and the Go results, by the way, the protein folding stuff comes more of that lineage that it has large language models.
给我们一个现状的概述。看,宏观框架实际上是运用规模计算来创建有趣的计算艺术品。我们在早期阶段就开始看到了这种趋势,比如AlphaGo、Alpha0和围棋结果,顺便说一下,蛋白质折叠的研究更多是来自这种谱系,而不是大型语言模型。
And then people started showing, opening up, I'm more specifically, there was some Google brain, other kinds of things, part of some of our investments. Start showing that you could do, out of training, out of like, one to two trillion tokens of language data. This creates an amazing kind of artifact that doesn't just do the kind of like, oh, look, great, the declaration of independence as a sonnet or translate my poem into Chinese, all of that kind of selfless course it does, but also does coding, also does legal, also does medical, also can get a five on the biology AP exam, and all the rest is, and this is the path we're on with this.
然后人们开始展示自己,开放自己,更具体地说,有些是谷歌大脑,其他的一些东西,是我们投资的部分。开始展示,你可以在没有培训、无需像样的数据环境下,创造出数量达一到两万亿的语言数据令牌。这创造了一种惊人的艺术品,不仅可以像“看,独立宣言成了十四行诗”或“将我的诗翻译成中文”这样无私地展现,还可以进行编码、法律、医学方面的处理,甚至可以在生物学AP(高级文凭)考试中获得五分。这就是我们当前的路径。
And so that's why, generally speaking, it's under the term artificial intelligence, because most of these amazing things are things that we would previously have looked at as cognitive achievements. But part of the prediction is not just that we'll be continued to be an amazing set of things coming from AI.
所以,通常来说,这就是为什么它被归纳为人工智能的术语的原因,因为大部分这些惊人的东西都是我们以前视为认知成就的事情。但预测的一部分不仅仅是我们将继续从人工智能中获得惊人的事物。
So another of sets of my partners, Sam, Motometi, and I wrote an article last fall that said, every professional will have a co-pilot that is between useful and essential within two to five years and define professional as you process information and do something on it. That's everybody in this room. Plus doctors, plus small business owners, plus legal, plus, plus, plus, plus, plus, you know, developers, et cetera.
那么,我和我的合伙人之一Sam、Motometi,去年秋天撰写了一篇文章,指出每个专业人士都会在两到五年内拥有一位副驾驶员,对于处理信息和实现目标来说至关重要。这包括这个房间里的每个人,还有医生、小企业主、法律界人士、开发人员等等。
That's just from the large language models. Now presume that what's happening there and finance and all the rest, presume that what's happening there isn't just going to, because you can think about industry impact will be is that's true very professional, every industry, highest professionals, what that transformation looks like.
那只是来自大规模语言模型的结果。现在假设在金融和其他方面发生的事情,并不仅仅是因为你可以考虑到行业的影响力是真正的专业,每个行业都有最高的专业人员,那个转型看起来像什么。
But I think we will see in addition to amazing things from large language models, I think we will see other techniques of the use of this kind of scale compute to create things. We'll see melds of them in various ways. You see some of that with Bing Chat going, okay, here's we've got scale compute and server, which has truth and identity and a bunch of other stuff, along with a large language models and here's what revolutionizes the search place and part of when Kevin and who's here and others and I were looking at this, we said, oh, because we saw this in August of last year, it's always easy to predict the future when you're seeing it with your own ads. And we said, okay, let's get ready and start building stuff right. That's what's going on across AI. And so it's extremely substantive and what's more, we're just dipping our toes into this.
但我认为除了大型语言模型带来的惊人成果外,我们还将看到其他利用此类规模计算创造事物的技术。我们将以各种方式融合它们。你可以在Bing聊天中看到一些,好吧,这里我们有规模计算和服务器,它拥有真相、认同和一堆其他东西,以及大型语言模型,这就是改变搜索领域的所在。当凯文和其他人和我一起看这个时,我们说,哦,因为我们去年8月看到这个,所以预测未来很容易。我们说,好的,让我们准备好并开始建立东西。这是AI领域正在发生的事情,非常实质性,更重要的是,我们只是尝试问询。
This is not like, oh, it's a hype moment, it's a big thing. This is like, that's like if you were saying, you know, back in 1992, 1992, 1993, oh yeah, the internet's really hyped right now. Oh yeah. So that's AI. Yeah, thank you. Yeah. It is always an optimist, but in the last 12 months, there have been many partner meetings at Greylock where he's kind of rung the bell of pay attention to this. This is meaningful and it's around the corner and I think we're all seeing that happen. And then Chad G.B. came out. Yeah, exactly. Yes.
这不仅是一场短暂的炒作,而是一件大事。这就像是你在1992年或1993年说:“哦,互联网现在很被炒作。”这就是AI,谢谢。虽然始终是一个乐观主义者,但在过去的12个月里,在Greylock的许多合作伙伴会议上,他总是喊出警告,注意这个事情。我认为我们都可以看到这已经在发生了,然后Chad G.B.也出现了,是的,完全正确。
So, Eric, so yeah, maybe just give us an overview of what RAMP does, how everything that we just described is affecting your business and Fintech weren't probably. Absolutely. I'm in wonderful to be here. So, RAMP is a finance automation platform. We're focused on functionally workflow and productivity related to movement of money. We're known for operating the fastest growing corporate card in the US, built payment software, expense management, and account automation and the like. And all of our products are designed with the intent of helping customers spend less money, most venture products are designed with the same or more, and spend less time. And so, really, what we're focused on is workflows that companies need to run in order to disperse funds, close their books and everything in between.
嗨,Eric,好的,也许您可以先给我们介绍一下RAMP做什么,我们之前讨论的所有内容对您的业务以及Fintech行业的影响是怎样的。当然可以。很高兴能在这里。那么,RAMP是一个金融自动化平台。我们专注于与资金流动相关的功能性工作流与生产力。我们以运营美国增长最快的公司卡而闻名,同时还开发了支付软件、费用管理、账户自动化等产品。我们所有的产品都是为了帮助客户节省更多时间和金钱,与其他公司的产品有所不同。因此,我们真正关注的是公司需要运行的工作流,以便分配资金、结账等。
I think the effect on AI from the business has been, frankly, profound. From even the founding year of the company where there's simple things in expense management, like text to receipt, match it to the proper transaction is a very simple machine learning to today when you think about accounting. Ultimately, these are generally accepted accounting principles. These are rules fundamentally of how transaction should be categorized and coded. And as you think about what are the patterns and how can you learn from the 10,000 plus businesses, 100,000 folks, losing, using, automating, both keeping of records, risk management and assessment to even go to market, even in the way that ramp has been able to grow so efficiently, has come down to embracing AI and our sales characteristics and lead routing and mapping the like. And so, happy to go deep, but there's probably 10 core work streams all throughout the business that are leveraging in some way from pattern matching to generative use cases as well. Yeah, that makes sense.
我认为商业对人工智能的影响非常深刻。从公司成立的那一年起,就出现了一些简单的支出管理问题,例如将文本转换为收据,将其与适当的交易匹配,这是一种非常简单的机器学习。到如今的会计领域,这些都是被广泛接受的会计原则。这些规则本质上是交易应该如何分类和编码的基础。当你思考有哪些模式和如何从10000多家企业、10万人中学习,以及记录保留、风险管理评估甚至是市场进入方式,连我们的销售特征和引导路线映射等都变得更加高效,这都归功于我们对于人工智能的拥抱。因此,我们非常乐意深入探讨,但在整个业务中,可能有大约10个核心的工作流在以某种方式利用模式匹配和生成性用例。是的,这很有道理。
So one interesting topic is, and Rita, no Sam Alman talks a lot about this, where the original narrative of AI was that it was going to automate more basic tasks, like more solvable problems like accounting or law. And the original thesis was that the last things to be automated were poets and photographers and graphic designers. It turns out that that's actually been the reverse, right, with at least this first wave of generative AI, where the creativity gives the models a little bit of leeway for making mistakes. And at least the current state of these generative models, they don't lead to 100% accuracy. But in fields like healthcare or like financial services, 100% accuracy is required in many cases.
一个有趣的话题是关于AI最初的故事,说的是它可以自动化更基础的任务,比如像会计或法律这样已知的问题。最初的观点是,最后需要自动化的是诗人、摄影师和平面设计师。但实际上事与愿违,至少在这一波生成式AI中是这样的,创造力给了模型一些犯错的余地。而目前这些生成模型的水平并不能达到百分之百的准确性,但在像医疗保健或金融服务等领域,有许多情况需要达到100%的准确性。而且,Rita和Sam Alman也提到过这个话题。
And so, what's your take on how fields like finance are going to be able to leverage these models? Well, first, the question is not 100% correct, because there is no 100% in human accuracy. I presume most of the people in this room would know that if you were asked to have an average radiologist read your x-ray film or a trained AI, you should take the trained AI if it's an average one. If it's the best one, or like one of the best ones, take that and better yet take them two together. So there is no such thing as human infallibility anywhere, including in finance. It's especially true. So, and so part of what we have to do is we have to kind of figure out now part of the thing is we know how to hold human systems accountable and what accountability law looks like. And what the error rate within human and what's acceptable when it's a person plus machine or when it's machine driven, those will be relevant variables.
那么,你认为像金融这样的领域如何利用这些模型呢?嗯,首先,这个问题不是百分之百正确,因为人类准确性没有百分之百。我认为,大多数在场的人应该知道,如果让一位平均水平的放射科医师读取你的X光片,或者让一台经过训练的人工智能,你应该选择训练有素的人工智能。如果是最好的那个人工智能,或者像最好的那个人工智能一样,最好选择两者一起。因此,任何地方都不存在100%的人类无误性,包括金融领域。这一点尤其真实。所以,我们要做的一部分是现在要弄清楚的一部分,我们知道如何让人类系统承担责任,以及什么是责任法律。人类和机器的误差率以及当有人加入机器或机器驱动时,可接受的误差率是相关的变量。
Obviously, we worry about things like, you know, for example, AI applied to credit decisioning of do you have, you know, systematically biased data. Now one of the benefits is to say, well, that becomes now a scientific problem, which you can then work on and fix as opposed to the human judgment problem where you say, well, we have that human judgment problem in how we're allocating credit scores or creditworthiness or parole or everything else today. And our human system, the machine system may start out looking like it's going to system and tie the old one, but we can fix it so that we can move past that, especially in person plus machine. And that's, I think, one of the things you're going to see with AI across the number of fields, including in finance and some of the early areas.
明显地,我们关注像人工智能应用于信用决策这样的事情,你知道,例如,你是否拥有系统性偏颇的数据。现在其中的好处是,这变成了一个科学问题,你可以修复它,而不是一个人类判断问题,你可以说,好吧,我们今天如何分配信用评分,信用价值或假释或其他事情上面存在着人类判断问题。我们的人类系统,机器系统可能一开始看起来像是要超越旧的系统,但我们可以修复它,让我们可以超越它,特别是在人与机器结合的时候。我认为,这是您将在一些早期的领域中看到人工智能应用的事情之一,包括金融领域。
I did publish a book last week called Impromptu with GPD 4 as a co-author where the central thesis was it's not artificial intelligence, it's augmentation intelligence, right? And obviously in various ways, it is also artificial intelligence, but it's the look at how you amplify people, like the co-pilot thing that I was saying earlier. And as an exemplar of that, now I did deploy my own personal team in helping with this, we started writing the book in January, right? So with that, we got it out, and there are physical copies, now the physical copies are more print on demand from Amazon, but we got it out last week, right? So that's the kind of thing of the proof and the pudding with, like, think about how this augments human activity. Again, augmentation amplification, that's the pattern to be thinking as how you go forward.
我上个星期确实出版了一本书,名为《即兴合著人工智能4》,其中央论是“这不是人工智能,而是增强智能”,对吧?当然,在各个方面上,它也是人工智能,但是我们要看的是如何增强人类能力,比如之前我说的联合飞行员的例子。作为这方面的一个典范,现在我部署了我的个人团队来协助这个项目,我们从一月份开始写这本书,所以,我们发行了实体书,现在实体书是亚马逊即时印制的,但我们在上个星期把它发行了出去,对吧?所以这就是证明人工智能增强人类活动的关键。再次强调,增强才是未来的发展方向。
And of course, there's a whole bunch of stuff when you begin to think about FinTech, Fintech, Fintech products, how you operate this Fintech company, where this touches. Yep, that makes sense.
当你开始考虑金融科技、金融科技产品、如何运营这个金融科技公司以及相关领域,当然会有很多内容需要考虑。没错,这很有道理。
And Eric, I think you have a pretty similar point of view around this topic. Anything you'd add in terms of, even just practically, like, how you build guardrails when you're dealing with sensitive information and NAA. Yeah, on the Fintech side of it, I mean, I think it's just like a very unique industry where it's such a large sector of the economy, which, as most of the world, and again, I think it's accelerating, but it went from no phones to flip phones to iPhones.
埃里克,我想你在这个话题上有一个相似的观点。在实际操作中,你会添加什么,例如如何在处理敏感信息和NAA时建立防护措施?是的,就在Fintech方面,我认为这是一个非常独特的行业,它在经济中占据了如此大的部分,就像世界上大多数地方一样,我认为它正在加速发展,但从没有手机到翻盖手机再到iPhone,这是一种独特的行业。
People still have the same credit cards in their wallet, same bank accounts. And I think in a way, folks in this room know, the Fintech industry blew open over the past five years, and actually software first orientation business models that can also move funds and get involvement workflows have started to spring up in a major way. So there's a lot of opportunities around it.
人们仍然在钱包里使用着相同的信用卡,同样的银行账户。我认为,这个房间里的人都知道,过去五年中,金融科技行业得到了大规模的发展,实际上,以软件为导向的商业模式也开始大量涌现,可以移动资金并获取参与工作流程。因此,这领域存在着很多机会。
When I think about some of the guardrails and patterns, like I agree with Reade, inherently, there's never been perfect when you think about underwriting, you know, best efforts. When you think about fraud, you take patterns to understand and prevent. And so I think perfection is actually quite rare. I do think the co-pilot model is a powerful one when you're thinking about, for us, one of our core customers is accountants. Where they need to be going and pattern matching and taking the learnings and bringing that back to augment and speed up is a very quick pattern, but also most of customers. Most employees are not accountants, don't know it, and being able to simplify and just as encoding over the past set of months. It's gone from, you know, primary language of coding from Python arguably in a year or two, it may be English is the primary way.
当我想到一些防护栏和模式时,我同意Reade的观点,本质上来说,在承保方面从来没有完美,你知道,尽力而为。当你考虑到欺诈时,你会采取模式来理解和预防。因此,我认为完美实际上是相当罕见的。我认为联合驾驶员模型是一种强大的模型,特别是对于我们的一个核心客户——会计师。他们需要进行模式匹配和学习,然后将其带回来增强和加速模式。但是,大多数员工不是会计师,不了解这一点,因此能够使编码过去数月的简化和编码过去数年可能成为主要方式的英语,这是一种非常快速的模式,也是大多数客户。
I think those same patterns you'll find in workplace productivity, expense management, accounting, vendor, intelligence, and that and the like. And so I think it's really thinking about where needs to be a high degree with risk and underwriting and may not want in making all the decision you want to see in back test.
我认为你会在工作效率、费用管理、会计、供应商、情报等方面找到同样的模式。因此,我认为应该仔细思考哪些领域需要高度的风险评估和保险承担,而不是在所有的决策中都采取回测结果。
And there's ways you can get it hereistically. Whereas if you have operational loops in, that co-pilot design pattern has been an emerging first way to deal with it. And actually, I'm not to put them on the spot, but I think Kevin Scott is the CTO of Microsoft who's here who pointed out to me is like, oh, what's the largest programming language in the world? English.
还有方法可以通过启发式的方式来获取它。如果你有操作环路,那么副驾驶员设计模式是处理它的一种新兴方法。实际上,我不想把他们置于困境中,但我认为出席此次活动的微软CTO凯文·斯科特向我指出了这一点:哦,什么是世界上最大的编程语言?英语。
Yeah. And I just want to double down on the point you made around, you know, AI is not perfect when other humans. And in many cases, AI actually performs better. How do you think we deal with kind of the political, social, regulatory kind of mindset shift? Right?
是的。我想要强调你提到的观点,即人工智能在面对其他人类时并不完美,而在很多情况下,人工智能表现得更好。你认为我们应该如何处理政治、社会和监管方面的心态转变呢?对吧?
Like one example is, you know, autonomous vehicles, right? Where, okay, you know, autonomous vehicles are safer than humans. Yet we need to, we're not necessarily socially comfortable with autonomous vehicles making those mistakes. So part of the reason why I would say that we need to own it as a society is every year that we delay shifting to basically autonomous vehicles on the roads, we're killing 40,000 people, blood's on our hands. So the important thing is say it's actually worth saving. And it won't be, look, you still won't get below a thousand or something. I don't know what the number is. It'll be a lot, but you'll save a ton of lives. And so you say, look, we have to solve these accountability issues. We have to solve these kinds of things.
嗯,就比如说自主驾驶汽车,你知道的吧?自主驾驶汽车比人类更安全。但我们并不一定习惯让自主驾驶汽车犯错。所以,我认为作为一个社会,我们需要承担责任的一部分原因是,每年推迟转向基本上都是自主驾驶汽车,我们就在杀害40000人,我们要为此负责。所以重要的是说它其实值得拯救。虽然可能还是会有一千左右的人死亡,我不知道具体数字,但你可以拯救很多人的生命。所以你要说,看,我们得解决这些问责问题,我们得解决这些问题。
And by the way, it's very similar when you get to, you know, obviously, when you look at the press and government, there's all this buzz about, oh my god, AI, and it's going to have some job impacts and we should slow it down all around. And obviously, there's all this discussion about competition with China and so forth, which is important. But here's a way of making a tangible current duty for looking at it, lying of sight to a AI doctor and an AI tutor on every cell phone. Deliverable, cheaply enough that anyone who has a smartphone can have access. Every month you delay that, think about what the human cost of that delay is. That's when I talk to government folks. They say, think about it this way. I'm not saying don't ignore data, don't large companies and ecosystems. I'm not going to say those are all relevant variables. But like one of the classic ways that democracy fails, all climate and everything else is, what about children and grandchildren and all these other people? How do we help them? And this is part of, it literally is buildable today with the technology. It's just a question of how soon and how do we get there? Yeah. And yeah, doubling down on that kind of optimism, right? That's, I'm happy to be optimistic. That's not optimism.
顺便说一下,当你看到媒体和政府都在谈论人工智能,提到它会对就业产生影响,表面上我们应该在全球范围内放慢发展速度时,情况非常相似。当然,人们也在讨论与中国的竞争等重要问题。但现在可以实际行动的方式是,在每个手机上都配备一个AI医生和AI辅导员,以便每个人都能负担得起它。如果你每个月都拖延一次,就会想到它给人类造成了多大的代价。当我和政府官员谈话的时候,他们说,把问题想成这样。我并不是说忽略数据、大公司和生态系统的问题。我不会说这些都是相关变量。但是,民主失败的经典方式之一是,所有的气候、其他一切都是什么关系,我们如何帮助孩子、孙子和其他人。而现在这部分实际上在技术上可以建立,只是问题在于有多快,如何实现而已。是的,我很乐意坚定这种乐观主义,这不是一种乐观主义。
That's truth. I mean, yeah, I don't think you have to convince this on the resume. There's a bunch of founders in this room, right? And also people who are like, who want to start companies, right? And so maybe this will be starting with Eric. Let's say you hadn't started ramping, not the kind of CEO of ramp. You see this kind of Cambrian explosion in an AI and you see these advancements that that reads describing. What are the most interesting opportunities? Like what type of startup would you build? Yeah. I mean, I think in some sense that many of the companies behind AI are often focused on productivity in the workplace.
这是真的。我的意思是,是的,我不认为你需要在履历上说服这一点。在这个房间里有很多创始人,对吧?还有一些像你这样想要创立公司的人,对吧?也许我们可以从Eric开始。假设你还没有开始做ramp,也不是ramp的CEO,你看到了这种智能技术的大爆发,你看到了Ried所描述的这些进展。最有趣的机会是什么?你会建立什么样的创业公司?是的。我认为在某种意义上,许多从事人工智能的公司往往关注的是职场生产力。
I think in some sense, reveals where many of these interesting use cases are put differently. I think when you look at a lot of knowledge work fundamentally based in data, where by default almost all of it is digital. If you can start to get involved in those workflows in both the movement of funds and reduction of work and augmentation of this world, I think there's a lot of interesting things. And so look, I think one of the most interesting areas is, frankly, around accounting. There's a lot. It is fundamentally pattern based. There's a large set of folks who need to look at repeated data, both within a company and you can learn across. So there's data network. There's proprietary data. There's some network effects. There's clear patterns. And some personalization that needs to take place. And I think when you start to combine those efforts, I think accounting is a very interesting space.
我觉得,在某种程度上,可以发现许多有趣的用例被不同地应用了。我认为,当你深入了解基于数据的大量知识工作时,几乎所有的工作默认都是数字化的。如果你能在资金流动、工作减少和更好地增强这个世界流程中参与其中,那么我认为有很多有趣的事情。所以看吧,我认为最有趣的领域之一是会计。这是一个根据模式进行的基础工作。有许多人需要查看重复的公司内数据,你可以在其中学习。所以,有数据网络,有专有数据,有一些网络效应,有明显的模式和一些需要个性化的工作。当你开始结合这些努力时,我认为会计是一个非常有趣的领域。
I do think in Fintech, particularly, I think there's both the ability to have better risk assessment and fraud fighting as well as probably a great opportunity for fraudsters too. I wouldn't recommend it as a venture-funded business, but when you think about a lot of it. It's not a US domicile one, but yes. It turns out these are big businesses. It's just not based here. Russian VC is like the V. Yes. I think that's true. It's a lot of opportunity. When you can generate someone's look, face, sound, predict information about them. And so I think both sides around that's going to be very significant opportunities. And last, just, you know, secularly even outside of the AI, the notion that core financial service products, which once were locked if you were a bank, you could store money and move money, now those requirements will need to be thoughtful about regulations and the effect it has.
我认为在金融科技方面,特别是在风险评估和打击欺诈方面有更好的能力,同时也可能存在欺诈者的巨大机会。我不建议它作为风险投资的业务,但是当你对它有很多想法时,这不是一个美国的本土产业,但它是一个大生意,只是不在这里。俄罗斯的风险投资就像V一样,我认为这很真实,它是很多机会,可以识别出某人的外貌、声音和预测信息。所以我认为在这方面的双方都会有很大的机会。最后,即使在没有AI的情况下,金融服务的核心产品,曾经是只有银行才能存储和转移资金,现在这些要求需要考虑到政策法规和影响。
You know, it's a much more competitive and I think in a good way, open field that allows innovation that has happened in the rest of the world should no longer miss financial services. And so I think it's quite exciting.
你知道吗,现在的金融服务领域更加竞争激烈,但我认为这是一种很好的开放方式,能够鼓励创新,让世界其他地方发生的创新不再错过金融服务。所以我觉得非常令人兴奋。
And read just along these lines, you know, there's a debate on whether startups or incumbents are kind of better positioned to take advantage of these advancements in large models. What's your framework in terms of, you know, which incumbents are best positioned to take advantage of this wave and which opportunities are more available for new entrants?
你知道,有一场辩论在进行,争论的焦点是初创公司还是现有公司更能够利用这些大型模型的进步。你的框架是什么呢?你认为哪些现有公司最有优势来抓住这个机遇,哪些机会对新进入者更加开放?
Well, the short answer is there's such a tsunami of stuff here. There is massive opportunity all around. So the usual kind of false dichotomy question is, it's only going to be, you know, Microsoft, OpenAI, Google, and everything, and too bad for everything else. No. Are there going to be things that these companies are going to dominate and do? Yes. But there's tons of room for other things among them, you know, like last year with Mustafa Silliman, former co-founder DeepMind, venture partner at Greylock. We co-founded Infliction. Unfortunately, I won't be able to talk much about Infliction. We will talk more about it in a month. We'll come back to town. And that's a startup opportunity. So you're putting our money where our mouth is and doing this.
嗯,简短的回答是这里有很多东西,有大量的机会。因此,通常会有一种错误的二分法问题,认为只有微软、OpenAI、谷歌等这些公司才有机会,其他公司都很遗憾。不是的。这些公司会掌握和实现一些事情吗?是的。但是,在其中还有大量的空间可供其他公司使用,比如去年与DeepMind的前联合创始人Mustafa Silliman以及Greylock的创投合伙人共同创办的Infliction。不幸的是,我不能多谈论Infliction,我们将在一个月内回到城市并谈论更多。这是一个创业机会,我们正在投资并努力实践。
And we have obviously a variety of great companies adept with David, you know, Crest, Snarkle, etc. We have a whole stack of AI companies that are, you know, invested in and going. So now, to the more broad thing is there's going to be a combination of I think two broad trends. One broad trend is the mega models, which are super valuable and important in this. A bunch of ways that will turn out to be super valuable and important.
我们显然有许多与David合作的伟大公司,比如Crest、Snarkle等等。我们有一整堆投资的AI公司正在发展。现在,更广泛的趋势是会有两个大趋势的结合。其中一个广泛的趋势是超级有价值和重要的巨型模型。有很多方式证明它们会超级有价值和重要。
But if you take interesting areas like medicine, law, coding, you say, okay, we're going to spend $500 million to make the larger, better model of this and it's going to be 20% better. Well, in those you're going to do it. We live in, we have an internet distribution, major distribution ends and they're like, okay, the 20% better product will just naturally have with no-thing else. Some network effects because everyone can get it through the internet, right, as a way to do that. And so the people doing they really big models and putting that in, which will be a small n number of. And I don't think only Microsoft Google Open AI, I think there will be, you know, one to five others.
但如果你选择像医学、法律、编码这样有趣的领域,你会说,好的,我们将花费5亿美元来制作更大、更好的模型,它将比现在的产品提高20%的水平。在这些领域,你会这样做。我们生活在一个有互联网分发的时代,主要的分发端口就像一个自然的网络效应一样,如果每个人都可以通过互联网得到它,那么这种20%更好的产品就自然而然地产生了。所以那些制作大型模型的人将会是一个很小的n数目。我认为并不只有微软、谷歌和Open AI,我认为还会有其他1至5个公司。
Well, Open AI is a good example of, you know, you want to predict about five years ago. Yes. But you actually would have because you did invest in it. Yes. But most people would have. Yes, although the investment was from my foundation because it was like, no, actually, in fact, this project from AGI is a very good thing. We had a discussion around the partnership table of should we do this? And we're like, okay, no revenue plan, no go to market plan. We have a responsibility or LPs to put it something into that is doing that. So, you know, in retrospect, if you could have said, hey, we look at now, then we would have said, how much of it can we take? But, you know, that's always the easy part of investing as the 10-year look back. And so, but anyway, so there will be this large channel.
嗯,Open AI 就是一个很好的例子。你想预测五年前会发生什么,它就是一个很好的例子。是的,但你实际上投资了它。是的,但大部分人都会投资它。是的,尽管这个投资是来自我的基金会。我们当时在合作伙伴讨论会上讨论,我们应该做这个吗?我想,其实这个 AGI 项目是非常棒的。我们没有收入计划,也没有推出市场计划,但我们有责任为我们的有限合伙人投资一些正在做这方面工作的事情。所以,回顾当时,如果我们现在看一下,我们可能会说:“我们能投多少?”但这总是投资的容易部分,因为它看的是十年回顾。但无论如何,将会有这个大型渠道。
And then there also will be a whole bunch of smaller models for all kinds of reasons, especially to something in finance that does a specific kind of accounting or fraud, other kinds of that, something that may run on your phone, and there will be a whole bunch of those things. So, for example, you know, GBD3 was very expensive to run the last compute run on. You know, about a month ago, I saw something was maybe, you know, this is a swag, you know, 80% of GBD3 that, you know, cost $3 million to make, right? And this is partially this other channel of stuff that will be happening, images, text, other kinds of things. And both of those will have great economic opportunities in them.
然后,还会有许多更小的模型,用于各种各样的原因,特别是对于进行特定类型的会计或欺诈的金融业务、在您的手机上运行的其他类型等事情,将会有很多这样的事情。例如,您知道GBD3上一次计算运行非常昂贵。大约一个月前,我看到了一个可能是80%的GBD3,它的成本达到了300万美元。这部分是另一种可能发生的事情,如图像、文本和其他类型的内容。这两者都将有伟大的经济机会。
And the thing that people a little bit too much mistake go to is it's just because I have an AI tech. So, actually, there's a lot of what you go to market, what's your business model? How does that, like, how do you competitively position? How do you create a mode? Is the motor network effectors, is it something else? Those will broadly still play into how you're thinking about how the tech disrupts things. So, the short answer is that it's an only large income, isn't only startups, it's massively both.
人们经常会犯一个错误,就是认为我的成功仅仅来自于我拥有AI技术。实际上,进入市场、商业模式、竞争定位、创造竞争优势等因素,这些仍然是我们考虑技术如何打破常规的重要因素。因此,简而言之,成功并非只由大公司或初创企业来实现,而是庞大的双方共同促成。
Yeah. That makes a lot of sense.
嗯,那很有道理。
And, you know, Eric, on this topic of, you know, large language models versus more fine-tuned models, I'm curious, you know, as it relates to ramp, obviously, you have a huge kind of workflow opportunity of just applying some of these large language models to your existing product, you know, having better underwriting, etc. Are there any opportunities for you to also invest in some fine-tuned models and some of your own AI?
嗯,你知道,埃里克,关于大型语言模型和更精细调整的模型,我很好奇就它们与 Ramp 相关的话题,显然,你可以有大量的工作流机会,只需将一些这些大型语言模型应用于现有的产品上,使得评估更好等等。那么,在您能否也投资一些经过精细调整的模型和一些您自己的人工智能方面,是否也有机会呢?
I mean, I think it's a super interesting and present question for a lot of practitioners, people building startups that do you bet on either A, the mega models, or B, more fine-tuned in-house development models, and frankly, somebody, you're curious for your view and opinion on it. You know, it seems to me, I think there's very unhappful general answer. It depends. It depends, very much. It's the more detailed conversation that we'll get to, maybe, X or Y.
我觉得这是一个对很多实践者、创业者来说非常有意思的问题。他们正在思考投资于 A、超级模型还是 B、更细致的内部开发模型。老实说,我很好奇你对此有何看法和观点。你知道,我觉得这个问题的答案很难一概而论。要看具体情况。也许我们可以进行更详细的交流,讨论一下 X 或 Y 方案。
Fair enough. Yeah. Anyway, so I didn't mean to interrupt your answer. It's totally, we'll take it again, but I think that's right.
好吧,没问题。额,我不是有意打断你的回答。完全可以再来一遍,但我觉得这样没错。
Ultimately, what do I think? So first, I think that for a variety of use cases, like relying on, like, I wouldn't bet against what's happening in the mega models themselves to, for the vast and general use cases, empowering or experienced generalized use cases using that. But there are a variety. I would be thinking as folks building businesses of, are there proprietary, is there proprietary data that's involved in the work flow of your business? Is there a data effect? Is there some level of personalization? And as you run it through, does the experience get better for every customer? And I think even back to some of the themes that we've been touching on, there may be broad-based risk and underwriting that once you start getting data, you can apply it and turn it on. But there may be even smaller use cases and loops from tagging transactions to understanding more about specific merchants and learning from the set and sharing that back out that you can tune to your model. And I think makes sense for two models.
最终,我认为什么呢?首先,我认为在许多用例中,比如依赖于的用例,我不会反对超级模型中正在发生的事情,为了广泛和普遍的用例,使用它来赋能或者经验化的一般化的用例。但是,有各种各样的用例。作为构建企业的人,我会考虑到,您业务工作流程中是否涉及专有数据?是否存在数据效应?是否有某种程度的个性化?当您运行它时,每个客户的体验是否更好?我认为即使回到我们一直在接触的一些主题,可能存在广泛的风险和承保,一旦您开始获取数据,就可以应用它并打开它。但甚至可能存在更小的用例和循环,从标记交易到更好地了解特定商家并从中学习并分享回来,您可以调整您的模型。我认为这对于两个模型都是有意义的。
I think one of the other questions specifically for finance, whereas most of these mega models have been trained primarily, whether it's text or images in some cases code, I think that there are larger models being built on numbers, relationships, accounting. And so I think the answer will evolve over time. I think in an outset of the training set of the mega models, I think the functional answer today is training more locally. But being ready and thinking about, is your stack prepared to make a switch in a value? Because I think that just the core infrastructure and couldn't have all of this is changing so rapidly. And so building in a way where you need to change things out, I think is important in this style of architecture.
我认为其中一个财务方面的问题是特别设计的,而这些巨型模型大部分是专门训练在文本或图片或代码等某些情况,我认为有更大的模型正在基于数字、关系、会计建设中。因此,我认为答案会随时间而演变。我认为现在在巨型模型的训练集中,功能性答案是更本地化的训练。但需要准备好并考虑,您的堆栈是否准备好在价值上进行转换?因为我认为,核心基础设施还有很多改变,所以以需要更换的方式构建是在这种架构风格中很重要的。
Everything Eric said is exactly right. Here's also Amplify, which is if your theory of the game is a thin layer around the AI model, it better be playing on the trend of the large models. You better be anticipating the large model. If that's not your theory, then the small model or the self-run model or whatever can itself go. But what happens is people go, well, I just put a thin model layer around it. You're like, well, the large models are going to blow you out of the water almost every single time. Unless you just happen to be the, I'm trading the next large, the large models of back end, I'm just trading the next one, next one, next one, I have fun. That can be a strategy too. But anyway, that would be another principle to add to it.
埃里克所说的一切都准确无误。还有 Amilify,这意味着如果你的游戏理论是在 AI 模型的薄层之上,就最好跟随大模型的趋势。你需要预测大模型的趋势。如果这不是你的理论,那么小模型或自主运行模型等就可以自行退出。但现实情况是,人们会说:“我只是在它周围加了一个薄模型层。”你会发现,大模型几乎每一次都会将你击败。除非你正好是“交易下一个大模型”的人,这样做可以成为一种策略。总之,这是需要添加到原则中的另一个原则。
Thank you. One of the final topics here, I wanted to double click on obviously these models are very powerful. We talked about the risk for using these for fraud, phishing attacks. I guess I'll pass it to Reed. Obviously, we're investors in abnormal securities that's taken the other side of that, which is using AI to detect phishing attacks and protect people. There's tons of security applications of this. And unfortunately, tons of offense applications through. Yeah. And so, yeah, I'm curious. And you see the exact same thing. Exactly, yeah. What are the vectors of offense defense? How do we put guardrails around this technology as a society and then also as a business? How should you think about the risk factors here?
谢谢。这里是最后一个话题,我想详细谈谈这些模型非常强大的问题。我们已经讨论了使用它们进行欺诈和网络钓鱼攻击的风险。我想让 Reed 来说。很明显,我们是异常证券的投资者,这是使用 AI 来检测网络钓鱼攻击并保护人们的一方面。这有很多安全应用。不幸的是,也有很多攻击应用。是的。所以,是的,我很好奇。你也看到了完全相同的东西。确切地说,什么是进攻和防守的向量?作为一个社会和企业,我们该如何在这项技术周围设置保护措施?在这里,你应该如何考虑风险因素?
So, one of the things I'll start with, paradoxically, is a defense on some of the criticism on the opening of I gets because people say, well, it's open AI. It should be open source, etc. And people go, oh, the people were releasing open source models.
所以,我首先要说的事情之一,具有讽刺意味的是,是对于人们在I gets应用开源上的一些批评进行辩护。有些人说,它应该是开放AI,应该是开源的。人们会说,哦,这些人在发布开源模型。
People say that. Yes, people say that. I don't say that. But people say that. And you know, academics want it because they want to access the open source models and entrepreneurs want it because they want to be able to build on it.
人们说这个。是的,人们说这个。但我不这么说。但人们说这个。你知道,学者想要它,因为他们想要访问开源模型,企业家想要它,因为他们想要能够在其基础上建立。
Oh, I understand all this stuff.
哦,我明白这一切。
The problem is that an open source, large language model of sufficient capability is a built-in fishing tool, right? Just to be really clear.
问题是一个开源的、具有足够能力的大型语言模型就像是一个内置的钓鱼工具,对吧?只是为了非常清楚明白。
It's like, here, you want to do cyber-fishing. We have it. We could do it right now. And so you have to be much more careful about open source in these things.
就好像你想要进行网络钓鱼一样,在这里,我们有这个。我们现在就可以这样做。因此,在这些事情中,你必须更加小心对待开源。
I mean, for example, to be clear about something last year, Gali from open AI was ready four months before it launched. Why? Because they took the extra four months and they said, well, it could be used for these kind of bad things.
我是这个意思,举个例子,为了让事情更清楚,去年 Open AI 的 Gali 在它发布前就准备好了四个月。为什么?因为他们利用了这多出来的四个月来考虑可能会出现这种不好的情况。
It could be used for child sexual material. It could be used for revenge porn. It could be used. We don't want any of these cases. And we're going to spend the extra time to really make sure that these are very difficult to do with our tool.
这个工具可能被用于制作儿童色情资料。可能被用于报复性色情。它可以被使用。我们不想要这些情况发生。我们会花费额外的时间来确保我们的工具非常难以做到这些。
And by the way, part of the reason why they offer it through an API is we can be paying attention. And we go, what's that? Let's fix that, right? That is.
顺便说一下,他们通过API提供服务的部分原因是让我们能够关注。当我们发现问题时,我们可以进行修复,对吗?就是这样。
And you get other companies that go, oh, we're heroes because we're releasing the open source models. And the open source models cause a notable increase in this kind of garbage distributed on the internet. And terrible impact for the stuff. And by the way, it's not financial, it's about holding it in the stuff.
有些公司会说:我们发布了开源模型,我们是英雄。但是这些开源模型会导致在互联网上分发这种垃圾内容的数量显著增加,对产品产生了可怕的影响。顺便提一下,这不是财务问题,而是关乎产品的质量。
So I'd say one kind of area is to say, well, we got to be much more thoughtful about the good outcomes because by the way, doctor, tutor, hugely important outcomes, fraud prevention, cyber prevention.
我想说的是,我们需要更加关注好的结果,因为医生、导师、防欺诈和网络安全等方面都是非常重要的结果。
And so one of the other things is because it's being driven primarily by commercial entities versus governmental entities, all of them are going, we don't do weapons, right? And by the way, a respectful, honorable position.
所以其中另一个原因是因为商业实体主导而不是政府实体,所以他们都会说,我们不生产武器,这是一个有尊重、有荣誉的立场。
But on the other hand, I'm okay. There are going to be weapons coming out of this. And you need to understand them because you can't defend against them if you don't understand them.
另一方面,我还好。这将会有武器出现。你需要理解它们,因为如果你不理解,就无法防御它们。
So like I've been going around to just about every lab that has a major effort going, no, no, you should work on some weapon stuff too. You should learn advanced security procedures. You shouldn't do like the weak-ass stuff like the NSA, which is a contractor can run out the door with them. That would be bad, right? But you should do it. But you should figure that out because we should be in advance that we're not vulnerable to them.
所以我到了几乎每个大型实验室里,嗯,不,不,你也应该做一些武器方面的事情。你应该学习高级安全程序。你不应该像NSA那样做软弱的事情,他们的承包商可以随便带走。那样是不好的,对吧?但是你应该做这个。但你应该想出来解决办法,因为我们应该尽早确保我们不会受到他们的攻击。
Because there are real vulnerabilities. And of course, when you see that as a venture firm like Railaugh, we start going, oh, we should start investing a lot of security companies. And we should do this, because we've got to make this happen.
因为存在真正的漏洞。当像 Railaugh 这样的风险投资公司看到这一点时,我们会开始考虑投资更多的安全公司。我们必须要这样做,因为我们必须要让这个变得更加安全。
I think we say that every year regardless.
我觉得我们每年都这么说,不管怎样。
Well, but maybe 10x this year.
嗯,但也许今年会增加十倍。
Yes.
好的。如果需要,我可以进行改写。
Can I want to end this part of the discussion just on an optimistic note of, I haven't been optimistic. Yes, exactly. Yeah, this is just the realism. The realist read.
能否问一下,我想在乐观的情况下结束讨论。我一直没有很乐观。是的,完全正确。这只是现实主义。现实主义者的观点。
So let's project five years out, right? What impacts will this technology have on just regular people? And what are you most optimistic for?
所以让我们将展望五年,对吗?这种技术将对普通人产生什么影响?您最乐观的方面是什么?
I mean, I think it's funny. Like, many of my competitors, their founders were around 1800s. They were top hats. These organizations are not built thinking about how much time it takes for people. They have all the time in the world. Tens of thousands of employees in time is functionally free.
我觉得这很有趣,就像我的很多竞争对手,他们的创始人都是在19世纪。他们戴着高顶帽。这些组织并没有考虑人们花费多少时间去完成任务。他们有无限的时间。数以万计的员工的时间在功能上是免费的。
But it's not for most people. And when I think about most aspects of financial services, there's an incredible amount of busy work that's required, whether it's in applications and reviews, in submitting expense reports, in doing accounting, and doing procurement, if you're not with things cost.
但这并不适用于大多数人。当我想到金融服务的大多数方面时,就会发现需要付出大量的繁琐工作,无论是在申请和审核中,提交费用报告,做会计,还是进行采购(如果你没有牵涉到成本方面的问题)。
And if you collapse the amount of work, it takes in order to get at more data and understand what's happening in the world and have the world's knowledge given to you more rapidly, personalized to the problems you're solving.
如果你能缩短获取更多数据并了解世界发生了什么以及针对你正在解决的问题提供更快速,更个性化的世界知识所需的工作量,那将是非常好的。
Or have work done for you, it's incredibly freeing. I mean, for us, I mean, one of the most common things that customers will say about ramp is, I don't think about it, expense anymore. Expense reports is anymore.
让别人为你做工作,真是太让人自由了。我的意思是,对于我们来说,客户最常说 Ramp 给他们带来的好处就是:现在我不用再想报销单怎么弄了。
I have time actually to do the interesting strategic work, not just to go and collect receipts in tag transactions.
实际上我有时间去做有趣的战略性工作,而不是只是去收集标签交易的收据。
And I think that's a very small and early sign of things to come. And so in many ways, allowing people to be more strategic focused on higher level work and more interesting and profound questions, I think is the potential. Yeah, I think by the way, I've only said because I think it can transform not just the productivity, but also the joy and meaningfulness of the stuff.
我认为这只是一件小小的征兆,预示着未来即将发生的事情。因此,让人们更加专注于更高层次的工作和更有趣、更深刻的问题,我认为这是可能的。是的,我这样说是因为我认为它不仅可以提高生产力,而且可以带来员工的快乐和存在感。
That's actually one of the things that's frequently mistaken. I think you highlighted that very well. So with both my grailock and Microsoft tats on, I kind of thought about this through a lens of a company.
那其实是经常被误解的事情之一。我认为你说得很好。所以,身上同时戴着我的Grailock和Microsoft的图案,我开始从公司的角度思考这个问题了。
You said, okay, say you gave everyone the power as being 10x. And because the classic press dilemma, like, oh my God, the people could be laid off in the business. It's like, okay, so let's walk through the departments. We had 10x sales people. Are we going to lay off any sales people? No. 10x sales would be great. Let's take it.
你说,好的,假设你给了每个人十倍的能力。由于经典的窘境,比如说,天啊,企业可能会裁员。那么,让我们走过部门。我们有十倍的销售人员。我们会裁员吗?不会。十倍的销售人员会很好。我们来实施吧。
You know, marketing people. Well, you might have different functions because the person who's doing the data entry and others, they're like, yeah, we know those functions. We don't need as much. But by the way, do you want less marketing? Do you now have a competitive bar about how you're marketing is? I have the same number of marketing people. Product. Yeah, engineering, probably, you know, like, you know, blah, blah, blah, blah. Probably even accounting.
你知道,市场人员。其实你们可能有不同的职能,因为做数据录入和其他工作的人,他们知道那些职能。我们不需要那么多。但是,你现在想要更少的市场营销吗?你是否对自己的营销手段有了竞争标准?我有同样数量的市场人员。产品。是的,工程,可能,你知道,啦啦啦啦。甚至会计。
Does you now you can do all kinds of different kinds of accounting and analysis of the business and a bunch of other stuff? Again, marketing is a change. Now, it doesn't mean it's all roses in Utopia, but like we've walked through a huge number of departments looking at this and gone, no, this is going to just be helping these companies operate a whole lot better.
你知道吗,你可以做各种不同类型的商业会计和分析,以及其他许多事情吗?再次强调,市场营销是一种变革。现在,这并不意味着在乌托邦中一切都是美好的,但我们已经走过了大量的部门来看这一点,并且得出结论:这将会帮助这些公司更好地运营。
It's not going to create any kind of, it will be some workforce transition in terms of what skills and what are you focusing on. Customer service, you might have less, right? So, it does mean again zero. But if you look at the overall package, you go, actually, in fact, this is not a, this is a workforce transformation.
这个句子的意思是:它不会创造任何种类的工作岗位,但在工作技能和关注的方面会发生一些员工变动。例如,客服可能会减少一些,但这并不等于完全没有。但如果你看整个情况,你会发现这其实是一种工作力量的转变。
所以,中文翻译可以说成:这并不会创造任何新的工作岗位,但这意味着在员工技能和工作重心上会有一些调整。例如,客服可能会减少一些,但这并不意味着一无所获。如果你看整个方案,你会发现这其实是一种工作力量的转型。
And then, you know, when I'm talking to the US folks, you go, well, what have we been doing for the last 20 years? We've been putting customer service jobs in Indian and Philippines as well. You know, this is actually, in fact, not actually a big workforce problem. And so, the optimistic thing is, actually, in fact, I think this will be productive. And I think part of the reason I didn't start where I normally start, which is also the joy of work will be a lot better.
然后,你知道,当我跟美国人谈话时,我会说,我们过去20年都在把客户服务岗位放在印度和菲律宾,现在才出现这个问题。事实上,这并不是一个真正的大问题。因此,乐观的是,我认为这将会是有益的。而我之所以没有像通常那样开始谈论的原因之一,是因为工作的乐趣也将得到更好的体现。
And by the way, I presume, given the tech and everything else that everyone here has played with chat LGBT at least, like, if you, if, if, if you didn't stay up at night, kind of go, oh my god, I kind of don't understand you. But it's fun. It's interesting. Right. So you should tell my girlfriend that I will maybe it's totally normal. Yeah. Oh, sure. That may cost you a bourbon. Yeah. Sure.
顺带说一下,我假定每个人在这里都使用过LGBT聊天技术,如果你没有熬夜体验过,肯定会有些不理解,但是这很有趣、很有意思。你可以告诉我的女朋友,这是很正常的事情。当然,你可能需要请客喝波旁威士忌。是的,没问题。
Well said. I was thinking maybe we'd open up to the audience for maybe just a couple of questions and then we can let everyone eat. Anyone, anyone have any questions for for reading Eric? So there was an art gallery open here that you know about a few days ago at one of the most prestigious galleries in the course. And it was it was a creator who had made all of the art presented with Dolly too.
说得好。我在想,也许我们可以对观众开放几个问题,然后让大家吃点东西。有人有什么问题要问 Eric 读者吗?有几天前在这里有一个艺术画廊在这里开张,你知道是在这门课最负盛名的画廊之一。展示的所有艺术作品都由同一位创作者和 Dolly 一起制作。
And I think you it would be fair to say that some of the most, you know, it was public art opening. Anyone could walk in and see it. And there were some of the most, you know, famous photographers and artists and film directors in the world there. And then we're all depressed because they felt like this is the this is like this is it. This is the beginning of the end. I mean, one of the photos was you could have easily thought was a Dion Arbus the twins photo, but you know a new work.
我觉得可以说,一些最知名的摄影师、艺术家和电影导演参加了公共艺术展览,这意味着任何人都可以走进去看,场面非常壮观。但是大家都感到沮丧,因为他们觉得这是末日降临的开始。其中一张照片让人很容易就想到了黛安·阿巴斯的《双胞胎》照片,但它是一幅新作品。
So what do you have to say to those people? So first I a bit of history on that particular one because it's kind of fun. And then then what I would say. So the history is a Benet Miller amazing film director, money ball, et cetera, et cetera, friend of a number of ours mine here. Happen to be in town having lunch with Mustafa Suleiman, my co-founder and flexion.
那么,你对那些人有什么话要说呢?首先,有点儿关于那个事情的历史,还挺有趣的。然后我会说什么。历史是这样的,本内特·米勒是一位了不起的电影导演,拍了《金球时代》等等,还是我们这里一些人的朋友。他碰巧在城里,和我的联合创始人和弯曲的创始人穆斯塔法·苏莱曼一起吃午饭。
And I said, oh come join us. You know you like this AI stuff. And so we were talking about what was happening. This was last year about image stuff. And I looked at Benet and I went, you know, I think opening I would give you early access to to Dolly. Would you be interested in Benet because he's better but yes. And I went, okay, great. And I called Sam on my way to the airport and I said, hey, I kind of promised Benet open access. Are you cool with that? And he's like, yeah, okay, great.
我说:哎,来加入我们吧。你知道你喜欢这个AI东西。然后我们讨论正在发生的事情。这是去年我们在谈论关于图像方面的问题。我看着Benet说:“你知道吗,我想提前给你提供Dolly的早期使用权限。你有兴趣吗?”因为他比较好,但是他点了点头说:“好的。”我说:“太好了。”我在去机场的路上给Sam打了个电话,说:“嘿,我答应了Benet提供他开放访问权限,你觉得可以吗?”他说:“可以的,太好了。”
And a month later, Benet shows me this amazing art. It's not that I couldn't even even thought the Dolly was capable of doing. I was like, dude, this is really good. We got a short to the opening of people. I don't think they have any. Because part of what's happening with this, these, you know, like hundreds of billion private models is turning computer science in a natural science. Right? Like it's like, whoo, whoo, you could do this stuff.
一个月后,Benet向我展示了这个惊人的艺术品。这并不是说我连想象都不敢想得到Dolly能做到这个。我就像,哥们,这真的太棒了。我们要为人们的展览短暂停留一下。我不认为他们已经看过这些了。因为这个的一部分是,这些数十亿的私人模型正在将计算机科学转变为自然科学。对吧?就像,哇,哇,你可以做这样的东西。
And you want to unleash someone creative doing it. And so, you know, it was like, okay, and we did that. And it was all great. And ends up at the negotiating.
你想要释放一个有创造力的人去做它。所以,你知道,就像,好的,我们就这样做了。结果在谈判时一切都很好。
Now, given that I haven't, that we're on camera and I haven't said to this person, I was talking to a musician last July. And I said, I, we have a program at OpenAI that can either give me an original ex like a Celine Dion, et cetera, and create that song or can give 15 seconds of it and create the rest.
现在,由于我没有告诉这个人,相机正在录制,所以我得说一下,我去年七月曾跟一个音乐家聊过天。我向他介绍了 OpenAI 的一个程序,可以创作出类似Celine Dion等歌手的原创音乐,也可以通过给出15秒的音乐片段来生成完整的歌曲。
I know that right now you're feeling terrified. Bad, right? You're like, holy shit. My job is done. Here is why I think you should excite your creativity. Because you can go do that. And then when it plays out, you're four minute bit. You can go, oh, the spit between second 25 and 35, that's really good. And the spit between a minute 30 and a minute 48, that's really good. And I'm going to take that and make something a lot better.
我知道你现在感到非常害怕,不舒服,对吧?你会感觉“天哪!我的工作没救了”。我认为你应该激发你的创造力。你可以去做那些事情。当事情顺利进行的时候,你可以回过头来看你那4分钟的细节,比如在第25秒到第35秒之间,还有在1分钟30秒到1分钟48秒之间的部分,这些地方真的很不错。然后你可以把它们拿来做些更好的东西。
This can be a tool for amplifying me. Right? Yes, you have to learn the new tool. That's the transformation. That's it. So yes, if you're like, I refuse. I'm really good. I'm just a extrex for now. And I don't need any new tools. Okay, we're the little harder now. Right for that. But if you go, oh my god, I could use this tool. And I, because by the way, who are these people? They have amazing artistic sense.
这个可以是增强我的工具,对吧?是的,你必须学会这个新工具。这就是变革。就是这样。如果你真的不想要,觉得自己已经非常好,只是现在的状态,不需要新的工具。那么,现在就变得困难了。但是,如果你说:“哦,天啊,我可以用这个工具。因为,顺便说一下,这些人有非常了不起的艺术感。”
Like, for example, part of what Bennett taught me was what is Bennett's like genius is that he thinks intensely visually as a director. Now we're giving the tools. I go type it all in here. And he can also then create art. Because he doesn't, well, it's because he's genius at that stuff. Right? It's an amplifier. And that's the thing.
举个例子,Bennett教给我的一部分就是他作为导演强烈的视觉思维方式,这也是Bennett天才的地方。现在我们提供了工具,我把所有内容都输入到这里,然后他就可以创造艺术了。因为他擅长这些东西,这就是他的天赋。对吧?这就是个放大器,这就是重点。
Now, to show yes, they have to learn the tools. And they go, well, I don't want to learn the tools. Well, well, look, I drive a horse and buggy and I don't want to learn the car. Okay, that's a choice. Right. But go learn the car. Like do that. That's what the general answer is. That is pretty cool to see creative minds. Yes, these tools in the hands of creative minds. I had no clue. By the way, so it's a thing to go see it. Go see it. I had no clue. Until Bennett went, oh, let me show you a few things. I was like, oh, we're all.
现在,为了展示他们的赞成,他们必须学习工具。他们会说,嗯,我不想学习工具。好吧,那是一个选择。但是去学习那个车。这就是一般的答案。很酷看到有创造性想法的人使用这些工具。我以前不知道,直到Bennett告诉我几件事情。要去亲眼看看。
Maybe we'll do you, we'll have a new one more time. One more, one, two more questions. This question's for you to so up, you know, as the founder of one of the foundation model companies, we're out like Stanford two weeks ago. They took 50,000 in pairs from the 175 billion perimeter model from open AI. And they fine-tuned on a 13 billion perimeter model off it, costs like 600 dollars. And it's as good as the open AI model. How do you do modes of holding now that anyone can just replicate your save the art model just having access to it? Not the architecture or anything, just the inputs and outputs.
也许我们会再做一遍,我们会有一个新的机会。再来,一个、两个问题。这个问题是给你的,作为一家基金会模型公司的创始人,我们在两周前就像斯坦福一样。他们从Open AI的1750亿参数模型中取出了50,000对,并对其进行了13亿参数模型的微调,成本大约600美元。它与Open AI模型一样好。现在既然任何人只需要访问输入和输出,就可以复制你的模型,那你如何保持优势呢,不考虑架构或其他因素?
Very deep question and great question. And the fundamental answer is it's unknown and we're discovering it. There is no simple answer to that question. For the will be, some answers to that question. It's just, who is it? Who is this huge ocean?
这是一个非常深刻、非常好的问题。根本的答案是我们不知道,我们正在探索它。这个问题没有简单的答案。对于将来会有一些答案,只是,它是谁呢?这个巨大的海洋是谁?
And I got a ship and I'm going to launch it. Where's the glean? What does it do? Now, a little bit to the earlier point is that there were a bunch of things that still we have learned in software entrepreneurship about, well, actually, in fact, you get kind of certain kinds of system integration. You have a good go-to-market by rallying something else. You have a data set, stuff that you were talking about earlier. Things that say, oh, that gives me, like, it isn't that all of the old wisdom about entrepreneurship and software entrepreneurship has just been dumped off the side of the boat. Right.
我得到一艘船,我要把它发射。谷物在哪里?它有什么作用?现在,先前的一点是,关于软件创业,我们仍然学到了一堆关于某些系统集成的事情。你有一个好的去市场方式,可以集合其他东西。你有一个数据集,像你之前谈到的那些东西。这些事情说,哦,那给了我,不是说所有有关创业和软件创业的老智慧都已经被抛到了船外。没错。
Now, you have to, it's a technological platform change that when I say it's bigger than any other, it's because it's the crescendo. You can't have it without the internet. You can't have it without mobile. You can't have it without cloud. It takes all of those and amplifies them. And that's why it's completely changing the game all these things.
现在,你必须接受一个技术平台的转变,如果我说这比任何其他的都要更大,那是因为这是顶峰。没有互联网是不可能的,没有移动设备也不可能,在没有云的情况下也无法实现。它需要所有这些元素的结合,并将它们扩大。这就是为什么这些事情完全改变了游戏的规则。
So, yes, but that doesn't mean that the old business model, the old business wisdom of kind of make all of those folks, but all of those of the trades are still relevant. But they're transforming and while figuring out which ones matter in which ways now.
所以,是的,但这并不意味着旧的商业模式、旧的商业智慧现在无关紧要。但是它们正在转型,我们正在弄清哪些是最重要的,哪些方面现在最重要。
So, it was a great question for everybody. Yeah, thanks for that. Just like further that question on this idea of, like, will LLM just be commoditized? Is first mover advantage just so much more important now? Because, and when opening I released the plugins today, I thought that was brilliant. Because now it's like, that's first, the chat GBT was becoming commoditized anyway. And now it's like, oh, that's interesting. Now, they have plugins. That's going to be hard for an else to get plugins.
所以,对于每个人来说,这是一个非常好的问题。是的,谢谢你。就像进一步探讨这个想法,LLM是否只是商品化了呢?现在先发优势是否更重要?因为当我今天发布插件时,我觉得那很棒。因为现在第一个是聊天GBT已经在变得普遍化了。现在,他们有了插件。那么其他人要想获得插件就会很困难。
So, I agree with that idea of like traditional models still applies. Exactly. And the short answer is, some of it, this is the reason why it's a work in progress.
所以,我同意传统模型仍然适用的这个想法。完全正确。简单地说,有一部分是适用的,这就是为什么它还在不断改进的原因。
Some of it will be completely commoditized. It will be kind of like it's, it's the cost, the compute, electricity, etc., and you can get it from three or four different players. And it's kind of like which one do you buy? Well, you know, I like this soft drink versus that soft drink, you know, kind of thing.
有些东西将被完全商品化。就像成本、计算、电力等一样,你可以从三到四个不同的供应商那里获得。然后就像你会选择喜欢哪种软饮料一样,你会选择哪个呢?
But which ways do you make it non-commodity? And how do you do that? Maybe some of it's in the tech? Maybe some of it's in the business? Maybe some, like, classic is a network of developers doing plugins. That's the classic play.
但是,你如何使它不再是商品呢?你如何做到这一点?也许其中一些涉及技术?也许其中一些涉及业务?也许一些东西像经典网络开发者做插件的方式。那是经典的做法。
So, of course, and, you know, I have the privilege and honor who I've been working with the open IT and they're very smart.
所以,当然啦,你知道,我有幸和荣幸一起工作过的是开放式IT团队,他们非常聪明。
Alright. I think let's break there. But thank you, Reed. Thank you, Eric. Thank you so much for being here. I hope you have great conversations with the people here. And yeah, enjoy the evening.
好的,我想我们可以在这里结束了。但是谢谢你,里德。谢谢你,埃里克。非常感谢你们能来这里。我希望你们能和在场的人们有很棒的交流。好的,享受这个晚上吧。
That concludes this episode of Grey Matter. You can watch a video of this interview on our YouTube channel and you can read a transcript on our website. Both are linked in the show notes.
这就是本期《灰色物质》的全部内容了。你可以在我们的YouTube频道上观看这场访谈的视频,也可以在我们的网站上阅读其文字记录。两者都已在节目注释中提供了链接。
And if you aren't already a subscriber, Grey Matter, you can sign up wherever you get your podcasts. I'm Heather Mack. Thanks for listening.
如果你还不是 Grey Matter 的订阅者,你可以在收听播客的任何地方进行订阅。我是 Heather Mack,谢谢您的收听。