DeepSeek’s Lessons for Chinese AI
发布时间 2025-02-06 23:00:40 来源
摘要
I want to thank several anonymous contributors for helping with background on this video. Links: - Patreon (Support the channel ...
GPT-4正在为你翻译摘要中......
中英文字稿 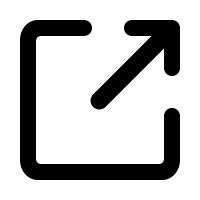
I apologize for adding yet another deep-seek video to your video queue. During a trip to Tokyo last year, I was told that deep-seek was the real deal, a cracked team and perhaps the only ones of significance in China. Since then I have annoyed the guys on Transistor Radio, our podcast with Dylan Patel and Doug O'Loughlin into talking about it, though there was nothing much to be said.
我很抱歉又在你的视频队列中添加了一个关于 deep-seek 的视频。去年去东京的时候,有人告诉我 deep-seek 是个了不起的团队,可能是中国唯一重要的团队。从那时起,我一直在烦我们播客上的团队成员——和 Dylan Patel 及 Doug O'Loughlin 核心成员聊这个话题,尽管并没有太多内容可聊。
In December 2024, Deep-Seek released their V3-based model, which had impressive efficiency, and a few people in AI were impressed. Then on January 22nd, 2025, Deep-Seek released their reasoning model R1, which works kind of like OpenAI's O1 and O3 models. It takes extra compute time to think up a better answer. R1's release kicked everything off. The next day, The New York Times published an article on it, but focused mostly on the earlier V3's training costs. Quote, The Chinese engineer said they needed only about $6 million in raw computing power to build their new system.
2024年12月,Deep-Seek发布了他们基于V3的模型,这个模型表现出色,效率很高,让一些人工智能领域的人印象深刻。随后在2025年1月22日,Deep-Seek发布了他们的推理模型R1,这个模型的工作原理有点像OpenAI的O1和O3模型,它需要额外的计算时间来想出更好的答案。R1的发布引起了很大的关注。第二天,《纽约时报》发表了一篇关于此的文章,但主要关注的是早期V3的训练成本。报道称,这位中国工程师表示,他们只需要大约600万美元的计算能力就能建立他们的新系统。
That is about 10 times less than the tech giant meta spent building its latest AI technology. The article seems to be saying that you don't need anywhere as many chips has previously thought for leading edge AI. A message that other large media and smaller influencers amplified. Then on Saturday 25th, CNBC published a 40-minute YouTube video that blew up. As of this writing, it has nearly 5 million views. I'm very jealous. All this led to a massive move down for tech and semiconductor markets on Monday losing a trillion dollars of market value. In video loss 17% or $600 billion, the biggest single day loss in history.
这比科技巨头Meta在打造其最新AI技术上花费的少了大约10倍。文章似乎在说,打造尖端AI所需的芯片,远比之前想象的要少。这一信息被其他大型媒体和小型影响者广泛传播。然后在25日星期六,CNBC发布了一段40分钟的YouTube视频,并迅速走红。截至目前,这段视频已有近500万次观看。我十分羡慕。所有这些导致了周一科技和半导体市场的大幅下跌,市值蒸发了一万亿美元。其中,科技股损失了17%或6000亿美元,创下历史上单日最大跌幅。
There was a massive online frenzy. CEOs and AI influencers alike posted soothing words. People were suddenly becoming experts on Javan's paradox and all that. I wasn't able to cover any of this as it happened because it happened during the New Year holiday in Taiwan, so I was spending time with family. Immaculate timing guys. So I missed that early rush, but I wrote up a few things anyway. Deepseek's leadership believes they have the way forward for Chinese AI, but does their approach scale and what does it say about the Chinese AI industry as a whole. For today's video, a few discombobulated thoughts on Deepseek.
出现了一场大规模的网络狂热。各大公司的CEO和AI影响者们纷纷发表安抚人心的言论。人们突然之间都在讨论Javan悖论之类的话题,仿佛变成了专家。当这一切发生的时候,我因为在台湾过新年假期,陪伴家人,所以没能即时报道。时间掌握得真是太“完美”了。因此,我错过了早期的热潮,但还是写了一些相关内容。Deepseek的领导层相信他们找到了推动中国AI发展的方向,但他们的方法是否具有可扩展性呢?这又反映了整个中国AI产业的什么状况?今天的视频中,我将对Deepseek进行一些零散的思考。
I'm not going to try to explain in detail what is so special about these new deepseek models. Oh boy, there are so many of those videos out there. This is what I'll say. The deepseek models pull out all the tricks in the book, including several new tricks of their own, to create a model that uses significantly less compute to both train and inference. Some of these innovations involve stealing, quote unquote, data from larger models like GPT-4 and Claude via distillation.
我不会详细解释这些新的deepseek模型有什么特别之处。天啊,网上有太多类似的视频了。我只想说,deepseek模型用尽了所有的技巧,包括它们自己的一些新方法,创造了一个在训练和推理时显著减少计算需求的模型。这些创新有些是通过所谓的"蒸馏"技术,从像GPT-4和Claude这样的大型模型中“借用”数据。
There are issues of perceived fairness of such a technique, but I reckon it is ultimately unavoidable. Another innovation was using 8-bit floating point numbers over industry standard 32-bit floating point numbers. There's also an interesting thing done with a mixture of experts' structure. Ben Ben points out in his FAQ over at Stretecory that many such innovations can be traced to the limitations of NVIDIA's China-specific hardware. So in one way you can say that the terror case is playing out. We imposed restrictions on the Chinese and the Chinese innovated something new to evade those restrictions, like a bacteria growing resistant to a dose of antibiotics. It is pretty nifty how Deepseek assembled them all into one slim model, just look at open AI's model selector menu to see how hard that is, but I should point out that most of these tricks are known or implemented in earlier models by other companies.
这种技术在公平性方面存在争议,但我认为最终是不可避免的。另一项创新是使用8位浮点数替代行业标准的32位浮点数。在专家结构的混合使用方面也有一些有趣的做法。Ben Ben在Stretecory的常见问题解答中指出,很多这样的创新可以追溯到NVIDIA针对中国的硬件限制。所以可以说,这有点像是恐怖案例的呈现方式。我们对中国进行了限制,而中国则创新出新的方法来规避这些限制,就像细菌对抗生素产生了耐药性。Deepseek将这些技术整合成一个简洁的模型,确实很巧妙,只要看看OpenAI的模型选择菜单,就能知道这有多难。不过,我要指出,这些技巧在其他公司的早期模型中已经被知晓或应用过了。
For example, Mistral has been using a mixture of experts since at least December 2023. But there is one apparent insight about R1 that caught my eye. Trust me again, I'm no expert. But from what I can discern, Deepseek managed to endow R1-0 with chain of thought and reasoning behaviors without having to insert a human into the loop. This approach took balls. It's a simple idea which people have tried before only to end in failure. So Deepseek not only noticed that it works now for a variety of reasons, but also decided to throw big model resources behind it.
例如,Mistral 至少从 2023 年 12 月开始就一直在使用专家模型的混合技术。不过,有关于 R1 的一个明显见解引起了我的注意。请相信我,我不是专家。但据我所知,Deepseek 成功地让 R1-0 具备了连贯的思考和推理能力,而且不需要人的介入。这种方法非常大胆。虽然这个简单的想法以前有人尝试过,但都以失败告终。然而,Deepseek 不仅注意到由于各种原因现在这种方法可行,还决定在此基础上投入大量模型资源。
There's another thing. I'm intrigued by Deepseek founder and head the Anglin-Fung's explanation in an interview about how Deepseek's memory-saving multi-head latent architecture came about. After summarizing some mainstream evolutionary trends of the attention mechanism, the Deepseek employee behind MLA just thought they designed an alternative. However, turning the idea into reality was a lengthy process. We formed a team specifically for this and spent months getting it to work.
还有一件事。我对Deepseek创始人兼负责人Anglin-Fung在一次采访中解释Deepseek的节省内存的多头潜伏架构的起源非常感兴趣。 在总结了一些注意力机制的主流演变趋势后,开发 Deepseek 的员工想到他们设计了一种替代方案。然而,将想法变为现实是一个漫长的过程。我们专门成立了一个团队,并花了几个月才让它运作起来。
Imagine what it might take to spend months on such tasks based on a researcher's hunch. That takes a special team in my opinion, and the Ang confirms this. Deepseek's moat, as Liang says, is not its current innovations, but a team capable of generating innovations anew. Liang has an interest in team development. He wrote the preface to the Chinese language version of Gregory's Zuckerman's biography of Jim Symons and Renaissance technologies, the man who saw the market. His book preface marks his interest in building lasting successful teams, and in it he wrote, what kind of characteristics and opportunities made Symons a historical outlier? How do you build and manage an outstanding team that remains undefeated for more than 30 years? Readers can find answers in this book. And going to the second of Liang's two 36K R interviews, basically the only such interviews on the web, what most people have glommed on to were his quotes about the superiority of the open source approach compared to closed source approaches.
想象一下,一个研究团队以研究人员的直觉为基础,花费数月时间进行这样的任务,这需要一个特别的团队。在我看来,Ang的确认证明了这一点。Liang表示,Deepseek的关键并不是当前的创新,而是拥有一个能够持续产生新创新的团队。Liang对团队建设有着浓厚的兴趣。他为中文版的《Gregory Zuckerman传记:吉姆·西蒙斯和文艺复兴科技》撰写了序言,书中谈到了他对建设持久成功团队的兴趣。在序言中,他写到,是什么特质和机遇让西蒙斯成为历史上的异数?如何建立和管理一个超过30年保持不败的杰出团队?读者可以在这本书中找到答案。而在Liang的两个36K R访谈中,网上唯一的这类访谈中,大家最关注的是他关于开源方法优于闭源方法的引述。
But most of the interview actually focused on how China can better surface innovation, as well as the latent strengths of the Chinese people. Liang is trying to rally other Chinese to make quote unquote hard innovations like they do and explains how his team does it. After reading the interview, I get the sense that Deepseek is a very non-Chinese company. Its founder is especially so. Such a contrarian structure lets it tap, supposedly, the very best of what Chinese talents can offer in leading edge software development. To give you a sense of where I am driving at here, I think I need to give some background on how top-level Chinese software companies like ByteDance and Pindoule doe work. China has plenty of quote unquote normal software companies, no different structurally from those in the United States. Want to make that clear?
大部分采访实际上集中在如何让中国更好地展现创新能力,以及中国人民潜在的优势。梁正在努力召集其他中国人进行所谓的“硬创新”,并解释他们团队的做法。在阅读采访后,我感到Deepseek是一家非常非传统的中国公司,尤其是它的创始人。这种反传统的结构据说能最大限度地挖掘出中国人才在前沿软件开发方面的潜力。为了让你更好地理解我的意思,我需要介绍一下像字节跳动和拼多多这样的顶级中国软件公司是如何运作的。中国有很多所谓的普通软件公司,在结构上与美国的公司没有区别。这一点需要明确。
But China's startup league leaders are distinct with fascinating organizations. Their dev teams are huge, sometimes dozens or even hundreds of people, and only roughly half of those team members are developers. The other half seems to be mostly quote operation staff, which, as best as I can tell, is a generic word for labor heavy tasks. Makes sense considering the low labor cost there. Like they rely on big quality assurance teams to test code before it goes out, which is generally not the case in the United States, or it seems like developers are expected to QA their own code. The way these big Chinese companies manage such big teams is with top-down management. So you have these meetings with 20 to 30 plus people where marching orders are basically broadcast to them. Staff like AI researchers are guided by OKRs and KPIs, written every quarter by their managers.
中国的初创公司领军者具有与众不同且引人入胜的组织结构。它们的开发团队规模庞大,有时达数十甚至上百人,而其中只有大约一半是开发人员。另一半似乎主要是所谓的运营人员,这个词大概是对需要大量人力的工作的通用称谓。这种安排在当地低廉的劳动力成本背景下显得合理。这些公司依赖大型质量保障团队在代码发布前进行测试,这种情况在美国通常不多见,因为美国的开发人员通常被期望自己测试代码。这些大型的中国公司使用自上而下的管理方式来管理如此庞大的团队。所以会有20到30人以上的会议,在会上指令会被直接传达给他们。像AI研究员这样的员工由他们的经理每季度制定的OKR和KPI来引导工作。
OKRs often cover the improvement of a model as well as its implementation through two to three features to be released that quarter. ByteDance has a big leaderboard that refreshes every so often, and when one of their doebowl models cannot keep up with the other top models in China, then the anxiety within that particular LLM team is palpable. Moreover information sharing with the outside world has been closed off. In ByteDance, Alibaba, and the other major Chinese AI startups nicknamed the Six Little AI Dragons of China, there's a freeze on publishing, same as in the United States. These Chinese software practices are obviously cherry-picked, but I'm trying to make a point about deep-seek. Small teams with little hierarchy or separation, flexibly going wherever their passion takes them, workers allowed to publish what they learn with their names attached to it, and the time, resources, and balls to go forth into the abyss to chase long-term insights.
OKRs通常涵盖模型的改进以及本季度将发布的两到三个功能的实施。字节跳动有一个大型排行榜,会定期更新。当他们的某个领域模型无法与中国其他顶尖模型竞争时,该特定大模型团队的焦虑感就会显而易见。此外,与外界的信息共享也被中断。在字节跳动、阿里巴巴和其他被称为中国“六小AI龙”的主要中国AI创业公司中,公开发表的活动被冻结,与美国的情况相同。这些中国软件实践显然是有选择性的,但我想强调的是对于深度探索的追求。小团队没有太多的等级或分隔,灵活地跟随他们的热情,员工可以以他们的名字发表所学内容,同时拥有时间、资源和勇气去追求深远的见解。
Deep-seek really does seem to buck convention in China. In both 36KR interviews, Liang talks about his focus on hiring for ability. In his first interview, he talks about how he first hired his funds sales team and their struggles during their early development. For example, our top two salespeople were outsiders. One came from exporting German machinery, and the other wrote back-end code at a securities firm. When they entered this field, they had no experience, no resources, and no prior connections. In fact, our sales team achieved nothing in their first year, and it was only in the second year that they started to see some results. But our evaluation standards are quite different from those of most companies who don't have KPIs or so-called quotas. Imagine what patients it might take for a company in China, the US anywhere, to tolerate a whole year of non-performance.
Deep-seek 确实似乎在中国打破了常规。在两次接受36氪的采访中,梁都提到了他对能力招聘的关注。在第一次采访中,他谈到了如何组建他的基金销售团队以及他们在早期发展中的挑战。例如,我们的前两名销售员其实都是外行。一位之前从事德国机械的出口,另一位在证券公司写后台代码。进入这个领域时,他们没有经验、没有资源、也没有之前的关系网。事实上,我们的销售团队在第一年并没有任何成绩,直到第二年才开始看到一些成果。但我们的评估标准与大多数公司不同,没有KPI或所谓的业绩指标。想象一下,在中国、美国或其他任何地方的公司需要多大的耐心,才能容忍整整一年没有业绩的情况。
Interestingly, Liang mentions that the Deep-Seek B2 team does not have a sea turtle, as in an overseas-trained Chinese. Noticable, since Chinese startups like to brag that their employees are elites with degrees from places like Harvard.
That being said, Deep-Seek does seem to like to hire from peaking and Qinghua universities, not exactly China's Dvrai University. They tend to shy away from hiring famous people.
An online interview with an unnamed Deep-Seek headhunter mentioned the company's preference not to hire people who have already been successful, saying, those who have been successful in the past have already achieved success. They carry the burden of not being allowed to fail. It's better to leave innovation to newcomers.
It does seem like Chinese tech companies are somewhat buying the pitch. Bydance, for example, has this new seed-edge team, a team stocked with highly-paid, cracked researchers empowered with over half of the company's marginal GPU compute.
The goal is to do research for the quote, long-term. Others are doing similarly. But I doubt these Chinese tech giants can entirely pivot to operating like how Deep-Seek does. It's not like what they're doing isn't working for them. Moreover, I question whether Deep-Seek itself can maintain its old ways going forward.
有趣的是,Liang提到,Deep-Seek B2团队中没有“海龟”,即在海外受过教育的中国人。这一点值得注意,因为很多中国初创公司喜欢炫耀他们的员工是来自哈佛等名校的精英。然而,Deep-Seek公司似乎喜欢从北京大学和清华大学招聘,而不是中国所谓的二流大学。他们往往不愿意聘用知名人士。
在一次与Deep-Seek的一名匿名猎头的网络采访中,猎头提到公司不喜欢雇佣已经取得成功的人。他们认为,那些过去已经成功的人承受着不能失败的压力。创新最好留给新手。
看起来中国一些科技公司似乎接受了这种理念。例如,字节跳动(Bydance)有一个新的seed-edge团队,这个团队由高薪雇佣的顶尖研究人员组成,使用公司一半以上的边际GPU计算能力进行长期研究。其他公司也在采取类似措施。不过,我怀疑这些中国科技巨头能否彻底转向像Deep-Seek这样运作。毕竟,他们当前的做法对他们依然奏效。此外,我也怀疑Deep-Seek能否在未来保持其原有的运作方式。
Why? Well, first, Deep-Seek has now gone mainstream. All the worldwide publicity has pushed is chat app to the number one spot in many countries around the world. Something anthropic was never able to do for Claude. It would behoove Deep-Seek's management not to take advantage of this hype.
为什么呢?首先,Deep-Seek 现在已经成为主流。全球的宣传报道使其聊天应用在许多国家排名第一,而这是 Anthropic 从未能为 Claude 实现的。Deep-Seek 的管理层应该充分利用这一热潮。
In his appearance in the Lex Friedman podcast, Dylan Patel mentioned off-handedly that Deep-Seek is now trying to raise around. I think they're going to go head-to-head with OpenAI in the prosumer chatbot market. Such a pathway means scaling up, adding product managers, marketing sales, and doing all the little things OpenAI did to become a quote-unquote real company, and that inevitably will dilute their unique research culture.
在 Lex Friedman 播客中,Dylan Patel 随意提到 Deep-Seek 正在尝试筹集资金。我认为他们打算在面向专业消费者的聊天机器人市场上与 OpenAI 直接竞争。这样的路径意味着他们需要扩大规模,增加产品经理、市场销售团队,并做 OpenAI 发展成所谓的“真正公司”时所做的所有细节工作。这不可避免地会削弱他们独特的研究文化。
Closing a gap always feels fast, as Deep-Seek enters uncharted territory, gains become harder to grasp. There's precedent for this. Just look at anthropic, a small team that rapidly caught up to OpenAI, but has since struggled to move past them. Here's another precedent. Deep-Seek's predecessor Hedgefun, Highflyer Boobed, thanks to an AI system first developed in 2015.
弥合差距总是感觉很快,当 Deep-Seek 进入未知领域时,取得的进展变得更加难以捉摸。这种情况是有先例的。看看 Anthropic 这家公司吧,一个小团队迅速赶上了 OpenAI,但之后很难再超越他们。还有另一个先例。Deep-Seek 的前身 Hedgefun,在其于 2015 年首次开发的 AI 系统的帮助下,也曾取得过类似的快速进展。
It worked well until 2021, when returns started to lag due to higher assets under management and more intense competition. It eventually caused Liang and management to apologize to customers through WeChat at the end of 2021. This unusual event was covered in the Wall Street Journal. A year later, in 2023, they found a Deep-Seek.
直到2021年,一切运作良好,但由于管理的资产增加和竞争加剧,收益开始落后。这最终导致梁和管理层在2021年底通过微信向客户道歉。《华尔街日报》对此不寻常事件进行了报道。一年后,即2023年,他们找到了一个“深度搜索”解决方案。
Third, there are talent issues. If I was running an AI team in China, I'd be giving each of those Deep-Seek employees offers that even their passions cannot refuse, and it is already happening.
第三,存在人才问题。如果我在中国管理一个AI团队,我会给每个Deep-Seek员工提供让他们无法拒绝的丰厚条件,这种情况已经在发生。
In late December 2024, before all the hysteria, Chinese media reported that Xiaomi's founder Lei Jun personally recruited Luo Fuli, one of the genius girls behind Deep-Seek V2 with a $1.3 million salary to run their LLM team. Replacing such talents will be challenging.
2024年12月下旬,在一片热潮之前,中国媒体报道称,小米创始人雷军以130万美元年薪亲自招募了“深度搜索V2”背后的天才少女之一——罗芙丽,负责他们的LLM团队。替换这样的人才将是一个挑战。
But I suppose that's fine though in the end. There will be more. China graduates an estimated Croatia or Panama's worth of STEM graduates each year, 4-5 million. One thing Deep-Seek will do, I feel, is lift the stigma of a purely domestic hire. One key limiter for Deep-Seek's team and all others will be chips.
不过,我想最终这也没关系。以后会有更多的。中国每年毕业的STEM(科学、技术、工程和数学)专业学生大约与克罗地亚或巴拿马的人口相同,大约有四到五百万。我觉得,Deep-Seek会做的一件事情是消除仅局限于本土招聘的偏见。Deep-Seek团队及其他所有团队的一个关键限制因素将是芯片。
Lei Yang says so in his second 36K R interview. Money has never been the problem for us. Bands on shipments of advanced chips are the problem. Did anyone see that Deep-Seek's hosted models are running inference on Huawei Ascend 910C hardware?
雷杨在他的第二次36K R采访中如此说道:我们从来没有遇到过资金问题。先进芯片的运输限制才是问题。有没有人注意到Deep-Seek的托管模型正在华为Ascend 910C硬件上进行推理运算?
It makes perfect sense that China's leading AI lab work with China's best hardware engineering company. The Deep-Seek kerfuffle will motivate the American government to further tighten the export of Nvidia chips. Even if all of Deep-Seek's Nvidia chips turn out to be kosher, it looks to me that they will get cut off, and Nvidia's China revenue will go to zero in the foreseeable future. Thus, Lei China's AI companies will have no choice but to turn to Huawei, whose Ascend accelerators are very good.
这很合理:中国的顶尖人工智能实验室与中国最好的硬件工程公司合作。Deep-Seek事件将促使美国政府进一步收紧对Nvidia芯片的出口管制。即使Deep-Seek的所有Nvidia芯片都合格,我认为他们也会被切断供应,而且在可预见的未来,Nvidia在中国的收入将归零。因此,中国的AI公司将不得不转向华为,后者的Ascend加速器性能非常出色。
This in turn will strengthen the Huawei-Smick industrial complex ramping up their semiconductor proficiency. It has been said that Google held a hardware-derived cost advantage because of their TPU ecosystem. Imagine Chinese AI companies possessing similar TPU-like advantages over their American cohorts. It would be beneficial for the Americans to have access to the most flops per dollar they can possibly get.
这反过来会加强华为-史密克工业集团提升其半导体能力。有人说,谷歌由于其TPU生态系统在硬件成本上占有优势。设想一下,如果中国的AI公司也拥有类似TPU的优势,相对于他们的美国同行来说会是什么样的局面。对美国人来说,能够以最低的成本获得最多的计算能力将是非常有利的。
So yeah, I don't think Deep-Seek's rather unchinies-like approach scales. They seem more like a one-of-one. But it doesn't have to. Another sentiment in the West is that the US is dominant in software, maybe unassailable so. This is wrong. China is one of the few countries with the world-competitive software industry. They are far better at it than Americans think. And specifically within AI, the Chinese are world-beaters in face recognition, machine vision and other AI fields. We bring over bunches of Chinese to the US to write our code. Why should we think that they can't catch up and fast? With regards to LLM development, the Chinese see themselves in a war situation. It's like their EV industry during the mid to late 2010s with a multitude of teams irresponsibly spending their way to the top. I think the American AI labs have too much confidence in their massive capital expenditure numbers, their perceived lead in productization, and their coziness with government. I urge them to push harder. Deepseek was the first Chinese lab to come out of left field, but it won't be the last. Alright everyone, that's it for tonight, thanks for watching. Subscribe to the channel, sign up for the newsletter, and I'll see you guys next time.
所以,我觉得Deep-Seek那种不太像中国的做法无法规模化。他们更像是独一无二的。不过,这也未必是坏事。在西方,另一个普遍观点是认为美国在软件领域处于主导地位,甚至无可撼动。这是错误的。中国是少数几个拥有世界竞争力软件产业的国家之一。他们在软件开发方面的能力比美国人想象的强得多。特别是在人工智能领域,中国人在面部识别、机器视觉和其他AI领域都是世界顶尖的。我们经常把许多中国人才带到美国来编写我们的代码。为什么我们会认为他们不能快速赶上呢?关于大型语言模型的发展,中国人将其视为一场战争。这种情况类似于2010年代中后期他们的电动汽车行业,许多团队在不计后果地花钱以争取领先地位。我认为美国的AI实验室对他们庞大的资本投入、他们认为在产品化方面的领先地位以及与政府的密切关系过于自信。我建议他们更加努力。Deep-Seek是第一个从意想不到的地方出现的中国实验室,但它不会是最后一个。好了,今天的内容就到这里,感谢观看。记得订阅频道,注册我们的通讯,下次再见。