AI Revolution: What Nobody Else Is Seeing
发布时间 2025-01-24 15:00:01 来源
以下是The Lightcone播客节目的中文翻译:
这期Lightcone播客节目由YC合伙人Harge、Diana和Gmail创始人Paul Buchanan共同参与,他们探讨了人工智能对初创企业和更广泛的科技领域的变革性影响。他们强调,由于人工智能工具的推动以及由此带来的商业可能性扩展,最近几批YC创业团队的增长速度和目标雄心都达到了前所未有的水平。
他们指出,公司实现重要里程碑,例如达到100万美元的年度经常性收入(ARR)的速度比以往快得多。以前的目标是在毕业后12-18个月内达到这个目标,现在几乎变成了最低标准,许多公司在六个月内就能实现,并制定了雄心勃勃的目标,例如在一年内从100万美元增长到2000万美元。
Paul强调了各个行业对人工智能解决方案的空前需求。与之前的技术变革(云计算、移动互联网)不同,企业普遍拥抱人工智能,为提供人工智能驱动解决方案的初创公司创造了有利的环境。向企业销售人工智能“代理”的公司尤其蓬勃发展,它们利用企业采用人工智能的压力。
话题转向了构建功能性人工智能产品,特别是人工智能代理所面临的挑战。市场对能够有效执行以前由人类完成的任务(例如客户支持或销售电话)的人工智能存在需求。成功的关键在于构建真正有效的产品,即使在竞争激烈的市场中也是如此。
Diana强调了用于训练人工智能模型的细致数据标记和评估集的重要性。一位创始人认为他们公司的黄金标准评估集是公司最有价值的资产,突显了其重要性超过了通用数据资产或品牌。Paul对此进行了扩展,指出模型本身正在迅速发展,这为代理和品味引导创造了机会,并强调了良好评估在人工智能开发中的重要性。
小组成员讨论了人工智能的伦理影响,尤其是在劳动力市场方面。虽然有些人担心失业问题,但Paul指出,人工智能有潜力创造更多的财富,从而实现科学突破并提高整体生活质量。他设想了一个“双重经济”,其中“机器货币”降低基本商品和服务(如医疗保健)的成本,“人类货币”重视人类技能、创造性努力和时间。
Harge提出了人工智能发展的两条潜在路径:一条是限制自由,另一条是最大限度地提高人类能动性。他们主张专注于后者,开发能够增强个人能力并扩大其潜力的人工智能工具。他们指出,最近的趋势似乎正朝着这个方向发展,涌现出许多创意工具和促进人类能动性的人工智能。
讨论转向了人工智能的发展方向以及过去十年中人工智能方法的转变。2015年,该小组专注于强化学习方法。然而,现在的重点已经从强化学习转变为关注预测下一个token这一目标函数。最原始的智能形式仅仅是预测接下来会发生什么,而没有内在的生存动力,这一点至关重要。
小组成员提到了一些轶事,例如在代码生成中使用人工智能,以及技术的快速发展,以及一些具体的例子,例如YC创业团队中有80%的公司使用Cursor,以及Anig等公司的收入增长。
播客还讨论了人工智能对SaaS公司的潜在影响,特别是公司编写自己的内部软件的潜力。之后,小组成员谈到了对业务的影响,以及一家此前苦苦挣扎的公司现在的年收入达到1亿美元的一半。
转录的结尾考虑了RAG等工具的使用,以及对初创企业和人工智能模型使用的影响。它以对创始人当前环境的热情赞扬结束,强调了人工智能创造的前所未有的机会。关键在于技术杠杆,它使有抱负和有洞察力的人能够取得非凡的成就。
The Lightcone podcast episode features a discussion among YC partners Harge, Diana, and Paul Buchanan (creator of Gmail), focusing on the transformative impact of AI on startups and the broader tech landscape. They highlight the unprecedented growth rates and ambition levels witnessed in recent YC batches, driven by AI-powered tools and the resulting expansion of business possibilities.
They note that companies are achieving significant milestones, like reaching $1 million in ARR, much faster than before. The previous aim of hitting this within 12-18 months post-batch has become almost a minimum, with companies now achieving it within six months and setting ambitious goals like growing from $1 million to $20 million in a single year.
Paul highlights the unparalleled demand for AI solutions across industries. Enterprises, unlike during previous technology shifts (cloud, mobile), are universally embracing AI, creating a favorable environment for startups offering AI-driven solutions. Companies selling AI "agents" to businesses are particularly thriving, leveraging the pressure on enterprises to adopt AI.
The conversation shifts to the challenges of building functional AI products, particularly AI agents. The demand exists for AI that can effectively perform tasks previously done by humans, such as customer support or sales calls. Success hinges on building products that truly work, even in a competitive market.
Diana emphasizes the importance of meticulous data labeling and eval sets for training AI models. One founder considered their gold-standard eval set to be their company's most valuable asset, highlighting its significance over general data assets or brand. Paul expands on this, pointing out that the models themselves are rapidly evolving, creating an opportunity in agency and taste prompting, as well as the importance of good evaluation in AI development.
The group considers the ethical implications of AI, particularly in relation to labor markets. While some fear job displacement, Paul points to the potential for AI to create significantly more wealth, enabling scientific breakthroughs and improving overall quality of life. He envisions a "dual economy" with "machine money" driving down the cost of essential goods and services (like medical care), and "human money" valuing human skills, creative endeavors, and time.
Harge posits two potential paths for AI development: one that constrains freedom and one that maximizes human agency. They advocate for focusing on the latter, developing AI tools that empower individuals and amplify their potential. They note that recent trends seem to be heading in this direction, with many creative tools and AI that promote human agency.
The discussion shifts to the direction of AI evolution and the shift in AI approaches over the past decade. In 2015, the group were focused on the reinforcement learning approach to AI. However, the focus has evolved from this to one where AI focuses on the objective function of predicting the next token. The importance is that the most raw form of intelligence is simply predicting what comes next, without an innate drive to survive.
The group mentions some anecdotes such as the use of AI in code generation as well as the rapid pace of technology, and some specific examples such as the use of cursor by 80% of companies in a YC batch to the growing revenue for companies such as Anig.
The podcast also discusses the potential impact of AI on SaaS companies, specifically the potential for companies to just write their own internal software. The panelists then touch on the impact on business and that a company is now halfway to $100 million a year in revenue that previously struggled.
The end of the transcript considers the use of tools such as RAG, as well as the impact on start-ups and the use of AI models. It concludes with an enthusiastic endorsement of the current environment for founders, highlighting the unprecedented opportunities created by AI. The key is technological leverage, enabling individuals with ambition and insight to achieve remarkable things.
摘要
In this special episode of Lightcone, we're joined by YC partner and creator of Gmail Paul Buchheit to dig into some of the latest ...
GPT-4正在为你翻译摘要中......
中英文字稿 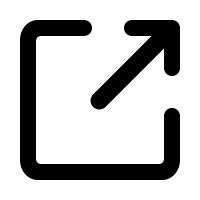
The deadline to apply for the first YC Spring batch is February 11th. If you're accepted, you'll receive $500,000 in investment plus access to the best startup community in the world. So apply now and come build the future with us. I think with AI, there's sort of two forks on the road. There's the bad direction and there's the good direction in the good path, which I think we're moving towards is looking to say how do we maximize human agency and freedom and are just potential to be kind of the best versions of ourselves. This is the first time no one's saying no, everyone is saying yes and more. There's just like unprecedented amounts of demand for just AI stuff. There's a whole category of businesses or products that would not have been economically viable or even possible to create before that are now possible. So we've actually just like expanded the universe of possible businesses. Never been a better time to be a founder, that's for sure.
申请YC春季首批项目的截止日期是2月11日。如果你被录取,你将获得50万美元的投资以及加入全球最佳创业社区的机会。所以现在就申请,与我们一起创造未来吧。我认为在人工智能的发展中,有两种不同的发展方向。一种是不好的方向,而另一种是好的方向,我认为我们正在朝着好的方向发展,这个方向旨在最大化人类的自主性和自由,并激发我们成为最好的自己的潜力。这是第一次没有人反对,所有人都在支持,并且有着前所未有的对人工智能的需求。有一类业务或产品在以前可能在经济上不可行,甚至无法创建,但现在都成为了可能。我们实际上扩展了可能的业务领域。毫无疑问,现在是成为创业者的最佳时机。
Welcome back to another episode of The Lightcone. And we've got a special one today because we are in Sonoma and we just wrapped up a 300 person retreat of some of our top AI founders. And we also have a very special guest today, the creator of Gmail and our partner at YC Paul Buchanan. Harge, why is this such a special episode? What are we doing? Well, we're filming from a different place. This weekend we put on an AI retreat for some of our alumni companies to share ideas about AI and what they're seeing as they're building their startups. And we learn a bunch of really interesting stuff. So we thought we would film an episode to talk about it.
欢迎回到《The Lightcone》的新一期节目。今天这一期很特别,因为我们身在索诺玛,刚刚结束了一场有300名顶尖AI创始人参加的休整活动。同时,我们还有一位非常特别的嘉宾,Gmail的创建者以及YC的合伙人保罗·布坎南。Harge,为什么这一集如此特别?我们在做些什么?嗯,我们正在一个不同的地方拍摄。这周末,我们为一些校友公司举办了一场AI休整活动,分享他们在创业过程中关于AI的想法和所见所闻。我们学到了很多非常有趣的东西,所以我们决定拍摄这一集来讨论这些内容。
So PVE, back in the day when we were working with companies, what was sort of an aspirational growth rate? What would we tell people to try to do week on week? Well, 10% week on week is an amazing metric to hit. And I think back then, if you were like maybe the top one or two companies in the whole batch, you'd be able to achieve that. And since summer of last year, the wildest thing is realizing that both summer and fall batch in aggregate, on average, over the batch in 12 weeks, average 10% week on week growth. So not just the very best, the Airbnb of the batch, but the batch overall. It's amazing. And it's not just during the batch. Diana and Harge, you guys have companies that you've worked with that have continued an insane growth rate long after the batch is over. Do you want to talk about those ones?
所以,以前在我们与公司合作时,我们会讨论一个理想的增长率是什么。我们会告诉他们,尽量每周增长10%是一个令人惊叹的指标。我记得那时,如果你是整个组中排名前一两位的公司,你可能会做到这一点。自去年夏天以来,最令人惊讶的是,整个夏季和秋季组的公司在12周内平均每周增长10%。这不仅仅是组中最顶尖的公司,比如Airbnb,而是整个组的平均水平。这真是太神奇了。而且,这种增长不仅仅发生在组内。Diana和Harge,你们有一些合作过的公司,即使在组结束后也继续保持了惊人的增长率。你们想谈谈这些公司吗?
One of the ones that really stands out is a particular company that went from zero to 12 million in 12 months. I'd never seen any growth like that. And I think we've seen this not to be just the exceptional different company of the batch, but actually more of them do that as well. Right? Yeah, that was my general pick up from this weekend was that just the rate of execution of startups is going is going much higher. And you can see it in both like how quickly companies are hitting like a million dollars in ARR. Like we used to, I think, say you should aim for that like 12 to 18 months out of the batch. And that's like the equivalent of the 10% week on week growth. Like that's what you should aspire to. Now it feels to me like that's probably like the minimum. I start up of companies hitting it within six months.
有一家特别引人注目的公司,从零增长到一年内达到1200万。我从未见过这样的增长。我认为,这不仅仅是这一批公司中一个特殊的案例,而是越来越多的公司也在实现这样的增长。对吧?是的,我这个周末的总体感受是,创业公司的执行速度正在大幅提高。你可以看到,很多公司达到年经常性收入(ARR)一百万美元的速度越来越快。过去我们认为,这个目标应该是在从初创到12到18个月内实现,那时候10%的周增长率是你应该努力达成的目标。而现在,我觉得这可能是一个最低标准。很多公司在六个月内就能达到这个目标。
And then I was just talking to some founders, we can just about their goals for this year. Like some of them have hit a million dollars ARR just now. I had one company say that their goal is 20. Like another company say they're aiming for 10 at least. Like just going from one to 20 million in one year. Yes. Yeah. And this is a goal founders have goals and like we hope them we hope they hit them. But I think like saying that like even a couple of years ago, hey, like also go from like one to 20. You would have thought you were just. Yeah. You would have just you would have been either like just like that's total nonsense. Like this is going to happen or like it just you just wouldn't have said it. And I just think that the general level of ambition has gone way up because of AI. The things are starting to work.
然后,我在和一些创始人交流,我们谈论了他们今年的目标。有些公司刚刚达到了年经常性收入(ARR)一百万美元。我有一家公司的目标是两千万美元,另一家公司则说他们至少希望达到一千万美元。他们想在一年内从一百万增长到两千万,是的,这是他们的目标。创始人有他们的目标,我们希望他们能实现。但我觉得,如果在几年前有人说要从一百万增长到两千万,你会觉得这完全不可思议,要么觉得这不可能实现,要么你根本不会这样说。我认为,由于人工智能的发展,人们的雄心壮志明显提高了,因为它确实开始奏效了。
And let's talk about why that is the case. Does anyone have any thoughts on that? Well, I guess I have a meme that I showed you guys earlier. You know, I think the classic thing is you have a boss who's sort of like slave driving and then I still believe this. Like if you're a leader, you're not you know, sort of slave driving from the back. You're way in the front like sort of leading. And then the meme has, you know, this one person pulling the cart alone. And that's the introvert. And what might happen now is the introvert with AI can pull three times as many carts all alone actually. Once intelligence is truly on tap, then it's actually a forced multiplier for founders and people with really, really strong senses of agency. Sure.
让我们谈谈为什么会这样。有人对此有任何想法吗?嗯,我想我之前给你们看过一个表情包。我认为经典的情况是你有一个老板,他有点像奴役驱动的方式,而我仍然相信这一点:如果你是一个领导者,你不是在后方驱使,而是站在前面领导。表情包里有一个人独自拉车,那是内向者。现在可能出现的情况是,内向者借助人工智能可以独自拉动三倍的车。当真正可以利用智能时,它实际上是创始人和那些有超强自主意识的人的力量倍增器。
Well, why specifically is this happening? One of the interesting talks I heard was from Aaron Levy, the CEO of Box. And he was talking about he's been through like multiple cycles of enterprise software. And he said that usually when there's sort of like a new cycle shift, but like cloud or mobile, there's always people in the room, decision makers at the big enterprise software companies saying like, no, like, you know, we're never going to shift to cloud. Like it's apparently like a famous quote from Jamie Dimon. Well, whenever like mobile is not going to be a thing, it's not that important. But with AI, it's different. This is the first time no one's saying no. Everyone is saying yes and like more. So there's just like unprecedented amounts of demand for just AI stuff.
为什么会特别发生这样的事情呢?我听了一个很有趣的演讲,演讲者是Box公司的CEO亚伦·莱维。 他谈到了他经历过的多个企业软件周期。他提到,通常当出现像云或移动这样的新周期转变时,总会有大企业软件公司的决策者在会议上说,“不,我们永远不会转向云。” 就像杰米·戴蒙的名言一样,觉得移动端不会成为趋势,也不那么重要。但在AI领域,这次不同。这是第一次没有人说“不”,所有人都在说“是”甚至“更多”。 因此,AI相关的需求量空前巨大。
Yeah, it's notable that all these companies that are having these incredible growth rates, they're all the same flavor of startup, right? They're all basically selling AI agents to businesses. And there's other companies that were funded that are doing well, but like all of the ones that you guys were talking about, right? They're all agents for businesses. And so they're all essentially, I think, riding on this wave of like enterprises are like have enormous pressure internally to adopt AI. This seems like it goes back to our fundamental based advice, which is make something people want. And in this case, traditionally, the challenge was convincing people that they wanted the product. And it sounds like what's driving the growth is that the demand is already there.
是的,很明显,这些公司都有着惊人的增长率,而且它们都是同一种类型的创业公司,对吧?它们基本上都是在向企业销售AI代理。此外,还有其他获得资金并表现良好的公司,但你们提到的那些公司,基本上都是为企业提供代理服务的。因此,我认为它们其实是在借助企业内部极大的AI需求压力这个趋势。 这似乎回到了我们的基本建议:做出人们想要的东西。在这种情况下,传统的挑战在于说服人们他们需要这个产品。而现在推动增长的似乎是需求已经在那里了。
Yeah. And so you just have to show up with a product that works and you don't even have to be that good at sales. The point is that actually building the product that works is quite hard. Like a lot of a lot of what the demand we're seeing is not for is for software that can actually do the work of a person that's essentially services and doing that to the equivalent level of a human doing the job, whether it's like customer support, sales, phone calls, whatever it is, is actually very, very hard. So I think just like a trend I noticed, a lot of our heavily technical CEOs who aren't necessarily the strongest at sales are able to win big enterprise contracts now because although there's 10 or 15 other companies competing for the same contract, it's very, very hard to build a product.
好的。基本上,你只要推出一个可用的产品就可以了,不用在销售方面特别出色。关键是,真正打造一个可用的产品是相当困难的。我们看到的许多需求实际上是针对能够替代人工执行工作的软件,例如客户支持、销售或电话业务等,要做到和人一样的水平非常困难。我注意到一个趋势是,很多技术很强但销售能力不一定最强的CEO,现在能够赢得大型企业合同。虽然有十到十五家其他公司在争夺同一个合同,但要真正做好产品是非常难的。
And so just building the thing that actually does the work well is enough to win these huge deals. A lot of the details of how they build the products, they're really inventing a lot of new patterns on how to build the product. Because nobody knew how to really get a lens to behave, let's say correctly and give very predictable results. And people thought that was impossible. That's because they only tried, maybe surface level they play with chat, JBT and sometimes I listen, and then people give up. That could be the random person just does that. But a lot of the technical founders, they don't. They find ways and wizardry around on how to really state a problem, how to properly prompt it to actually be very accurate and it is possible because we're actually seeing a lot of these products getting bought at businesses to handle all these complex tasks.
要想赢得这些大订单,真正把产品做得好就足够了。在产品构建的过程中,他们发明了很多新方法。因为没人真正知道如何让镜头(或设备)表现得正确并提供可预见的结果。人们原本以为这是不可能的,因为他们可能只是在表面上尝试,比如和聊天机器人简单互动,有时候倾听一两句,然后就放弃了。普通人可能就是这样做的。但许多技术型创始人则不同。他们找到各种方法来正确地陈述问题,制定精确的提示以获得准确的结果。这是可能的,因为我们确实看到很多这样的产品被企业购买,用来处理复杂任务。
One thing I noticed this weekend is that a lot of the talks that the founders gave were around evals and like testing, which I don't think that would have ever been true at a previous YC conference. Like testing was sort of this like afterthought thing that you try to do as little of as possible. I heard one really interesting comment from a founder who's building an AI agent who said that he thinks that the most valuable thing that his company has built is not the code base. It's the eval set. It's a gold standard labeled set of data of like this is what is the correct answer for the AI to do. And that was sort of a mental change for me that like there's I think this perception that would that like companies have like data assets, but just like general brand. And data is actually not that valuable. The thing that's really valuable is like a gold standard meticulously labeled eval set.
这个周末我注意到一个现象,很多创始人在演讲时都围绕着评估和测试这个主题,而在以前的 YC (创业者大会)会议上,我认为这从来不是重点。过去,测试好像只是一个附属想法,大家都想尽量少做。我听到一个正在开发 AI 代理的创始人说了一句非常有趣的话,他认为他们公司最有价值的东西不是代码库,而是一套评估数据。这是一套高标准标记的数据集,用来定义 AI 应该给出的正确答案。这让我重新思考,过去可能大家觉得公司拥有的资产就是一般的数据和品牌,但实际上,真正有价值的是那套经过精心标记的黄金标准评估数据集。
I mean, this is exactly why the whole chat GPT wrapper meme is wrong and that actually it's the model that is changing very quickly. There are clearly five or more AI labs, all of which are right there at the frontier. So now there's a lot of alternatives to which model, but then the thing that nobody has that is actually hard to get is the eval set. And I'd argue the prompting, which is sort of like mirrors basically all of the opportunity for the people watching right now. It's like basically agency and taste prompting just knowing what to tell someone to do or what to tell the agent to do. And then he vows his taste. It's like, is it good? Is it beautiful? Is it useful?
我的意思是,这正是为什么整个 "chat GPT 包装器" 的观点是错误的,其实真正快速变化的是模型。目前显然有五个或更多的 AI 实验室处于前沿。因此,现在有很多模型可供选择,但没有人真正容易获得的是评估集。我认为提示也是如此,这为现在正在关注的人提供了大量机会。提示基本上就像代理和品味,知道告诉某人该做什么或者告诉代理该做什么。然后他评估他的品味:这是否好?是否美观?是否有用?
I heard a very interesting tidbit from a founder who said that his designer, they've actually stopped using Figma mock-up things and the workflow is interesting. So the designer is designing entirely with Claude and going from text to JavaScript. It was just counter into to me because you assume designers is like very visual thing, but apparently their designer has figured like it has enough taste to be able to turn that into just like text prompts and like via prompt engineering essentially get to a actual like lines of code that they are like as tasteful and as good as any Figma mock up would have been. So it's like the pattern as always is whoever can iterate the fastest wins. Yeah. And AI is an incredible tool for rapid iteration.
我听到一个很有意思的小趣闻,一位公司创始人说他们的设计师已经不再使用 Figma 进行设计了,整个工作流程很有趣。设计师完全使用 Claude 从文本转化为 JavaScript。这对我来说有点反直觉,因为我们通常认为设计是一个非常需要视觉化的东西,但显然他们的设计师已经找到了方法,通过文本提示和精心设计的提示工程,将这些转化为像任何 Figma 模型一样精美的代码。正如一贯的模式,谁能最快速地迭代,谁就能获胜。人工智能在快速迭代方面是一个不可思议的工具。
I guess people are, you know, sort of worried that the jobs are going to go away. But earlier we were talking about, um, there's this great Milton Friedman, uh, quote where he's visiting a developing country and he sees this large group of workers digging a canal using shovels and he asks his government official host, you know, Why are you not using machinery? What's going on? And the guy says, it's a jobs program, actually. And, uh, what does Friedman say? He says, if it's a jobs program, you should go to spoons, not shovels. I think that that's actually the most useful mental model for sort of the fear about job loss, at least for now.
我想人们有点担心工作会消失。早些时候我们讨论过一个很棒的米尔顿·弗里德曼的故事,他访问一个发展中国家时,看到一大群工人用铲子挖运河。他问他的政府官员向导:“你们为什么不用机器?这是怎么回事?”那位官员说,这其实是一个就业项目。弗里德曼说:“如果这是一个就业项目,你们应该用勺子,而不是铲子。” 我觉得这实际上是一个很有用的思维模式,用来理解人们对失业的担忧,至少暂时是这样的。
Certainly, you know, the potential of AI is because it is this incredible tool where we're moving not from spoons to shovels or shovels to bulldozers, but to the point where the AI can do so much work that we're actually just able to create dramatically more wealth. And I think that's really the dream that we have for, you know, peering 10 years into the future. Um, is I think there's a potential for an unprecedented level of certainly scientific discovery.
当然,你知道,人工智能的潜力在于它是一种不可思议的工具。我们不再是从勺子到铲子,或从铲子到推土机的进步,而是达到了一个人工智能可以完成大量工作的阶段,使我们能够创造出显著更多的财富。我认为这正是我们对于未来十年的梦想。我们期待,在科学发现方面,会有前所未有的突破。
Um, the AI is incredibly good at reading thousands of papers, um, you know, digesting textbooks, very good at chemistry. Um, so I think we're going to see incredible levels of productivity. The story is fascinating to me because the alternative is what replacing people's shovels with spoons. Like, I, you know, I think it's absurd on its face. Like, you know, like that actually is a little bit like torture. Like I feel like, you know, growing up, my dad would force me to work in the gardens. That alone was barbarous to me. But like, if you made me deal with a spoon, what would we call that? That'd be torture, actually.
嗯,人工智能非常擅长阅读成千上万的论文和消化教材,在化学方面也很出色。所以我认为,我们将在生产力上看到令人难以置信的提升。这个故事对我来说很有趣,因为另一种情况就像是用勺子代替人们的铲子。我觉得这想法很荒谬,简直就像是一种折磨。我记得小时候我爸让我在花园里干活,那对我来说已经很残酷了。如果他让我用勺子干,那简直就是酷刑。
I think the question that I posed to Sam that everyone seemed most interested in is essentially, you know, are any of these startups actually going to exist in 10 years? We're sending you relevant to the audience. Yeah. Yeah. Everyone felt that was relevant because, yeah, if we, if we do achieve AGI, you know, what does that mean? How quickly can that actually just displace all of the work that we're doing here? And honestly, like no one's quite sure. Which makes us a very exciting time in technology.
我想我问Sam的那个问题是大家最感兴趣的,基本上就是:在十年后,这些初创公司中会有哪一家还存在吗?我们提的问题跟听众息息相关,对吧。大家都觉得这个问题很切题,因为如果我们真的实现了通用人工智能(AGI),那这意味着什么?它到底会多快取代我们现在所做的工作?老实说,没有人能完全确定。这也使得这是一个技术领域非常激动人心的时刻。
But, you know, it's very clear that we're able to achieve a lot more. And I think like throughout history, every time we've found ways to create more wealth, more rapidly, that's actually worked out really well. You know, historically, 97% of people were farmers or something like that. And now it's, you know, I think maybe 3% or even less. And so we seem to be very good at inventing new work for ourselves and new ways to find purpose and meaning.
你知道,我们确实能够取得更多的成就,这一点非常明显。我认为,在整个历史进程中,每次我们找到更快速创造财富的方法,结果通常都很好。历史上,大约97%的人是农民,而现在这个数字可能只有3%甚至更少。因此,我们似乎非常擅长为自己创造新的工作,以及寻找新的目标和意义。
Well, what was the answer to your question? I think luxury real estate. You know, what are the things that people will value in the future? And if we get to the point where we really do have just a real abundance of the kinds of things that machines are good at creating. And so this is actually an idea I've been talking about for 10 or 15 years of almost thinking about the world in terms of machine money and human money. That really what we want to do is take the products of technology and create actually massive deflation.
那么,你的问题答案是什么?我认为是豪华房地产。你知道,人们在未来会重视什么?如果我们真的到了一个机器能够大量生产各种物品的阶段。那么,这是一个我已经谈论了10到15年的想法——几乎可以用机器货币和人类货币的概念来思考世界。我们真正想做的是利用技术的成果来实现大规模的通货紧缩。
We want to drive the prices, you know, down to zero so that we're all able to afford, you know, certainly like medical care or something. I think a lot about where it's really hard for most people to get really great medical care today. And I think that that's something where in 10 years we're going to be able to make it so that, you know, the majority of humans on earth have probably better medical care than we here at the table have today, which is, I think, going to be a huge achievement. But at the same time, you know, that's kind of on the machine money side of things.
我们希望将价格降到零,也就是说,让每个人都能够负担得起,比如医疗服务。我常常思考,现在大多数人要获得优质医疗服务有多么困难。我相信,在未来10年内,我们能够实现让地球上大多数人享受到比我们现在所拥有的更好的医疗服务,这将是一个巨大的成就。但与此同时,这也涉及到资金机器方面的运作。
But then you think about the human money. What are the things that we get that we really value from humans? Like, you know, if you go to see live music, we seem to have a preference to see a band live instead of just sit in front of a bunch of speakers, you know, or maybe robots playing music. Human money, I think, might be something that comes closer to just like an hour of your time, right? And that we actually have almost like a dual economy. That's super interesting. Embedded in that is actually maybe a better version of UBI. I mean, a bunch of the studies around UBI are sort of showing that there are sort of nice benefits here and there, but fundamentally, it's not creating a greater sense of well-being in the way that people hoped, maybe like five or 10 years ago. Yeah, it's definitely had mixed results.
但然后你会想到“人力货币”。我们真正看重人类带来的东西是什么呢?比如,当你去看现场音乐表演时,我们似乎更愿意看乐队现场演奏,而不是只坐在一堆音响前听音乐,或者看机器人演奏。我认为,“人力货币”更像是你一个小时的时间。我们实际上几乎有一个双重经济。这很有趣,其中可能包含一个更好的基本收入(UBI)版本。很多关于UBI的研究表明,它带来了一些好处,但基本上没有像五到十年前人们期望的那样极大地提升幸福感。确实,它的结果是喜忧参半的。
And I think a lot of that really comes down to, you know, people still need guidance in life. And especially a lot of the people who are targeted with UBI are not necessarily people who are in a great sort of social position to begin with. And again, I think that there's a lot of potential for AI to actually kind of act as like a life coach. And again, like, you know, if you're fortunate enough to grow up with great parents and a great culture or something, you have a lot of advantages that a lot of other people maybe didn't have. And so again, I think like the great promise of AI is kind of taking like the best of what we have available and then just making it universally accessible. Because we're able to drive the cost so low.
我认为这主要是因为人们在生活中仍然需要指导。尤其是那些被基本收入(UBI)关注的人,他们通常原本的社会地位就不是特别好。同时,我认为人工智能有很大的潜力,可以扮演“人生教练”的角色。如果你幸运地成长在有优秀父母和文化的环境中,你就拥有了许多其他人可能没有的优势。所以,我觉得人工智能的巨大前景在于,可以将我们所拥有的最佳资源普及化,因为我们能够将其成本降低到极低的水平。
I mean, honestly, this past December, I spent a couple of weeks in Vietnam and you're in a developing country and then you realize like there is so much that needs to be developed. It's actually, you know, there's roads, there's infrastructure, like the whole country seems like it's under construction. I imagine that's what China probably looked like in, you know, maybe the mid 80s or mid 90s. But there's also like this crazy optimism as it's building. If you have a robot, like it could build your house, it could clean your house. It could, you know, take care of all of these things for you. And that would like radically change your day to day, your standard of living and how much more direct can you be if you rather than sort of just giving people more human money does give them like the better way to live.
说实话,去年12月我在越南待了几周。你身处一个发展中国家,就会意识到那里有很多需要发展的地方。实际上,到处都是道路和基础设施,看起来整个国家仿佛都在施工中。我想这可能就像中国在80年代中期或90年代中期时的样子。但与此同时,随着建设的推进,那种乐观情绪也非常强烈。如果你有个机器人,它可以帮你盖房子、打扫卫生,处理这些日常事务,这将从根本上改变你的日常生活和生活水平。与其单纯地给人更多资金,给他们更好的生活方式或许会更直接有效。
And that's sort of, you know, everyone can get here. But then I think there's like a special thing here around the human money where like the really remarkable things like, you know, nobody's guaranteed to have, you know, beachfront property in California or something like that. And that's where human money might go. Like everyone has the basics and actually something that's probably five or 10 times better than what even the most wealthy people have today. Yeah. Yeah, the way I like to think about it is I kind of think with AI, there's sort of two forks on the road. There's there's the bad direction and there's the good direction. And I think the bad direction is one where it's used to just like constrain and control and essentially like imprison us.
这段话可以翻译成中文:
这就像,大家都可以来到这个基本阶段。但在关于人类财富的问题上,有一个特别之处。譬如说,像拥有加州海滨房产这种了不起的东西,并不是任何人都能保证得到的。而这就是财富的用途之所在:每个人都能拥有基本生活,甚至比当今最富有的人所拥有的还要好五到十倍。是的,我喜欢这样去思考:在人工智能的发展中,仿佛有两条路可走,一条是坏的方向,一条是好的方向。我认为坏的方向是,人工智能被用来限制、控制和实质上监禁我们。
And in the good path, which I think we're, you know, we're moving towards is looking to say, how do we maximize human agency and freedom and are just potential to be kind of our best, the best versions of ourselves. And we even have that, you know, today with some of the creative tools where, you know, I don't have like a lot of artistic ability, but with like AI image generation or something, I can convey, you know, funny concepts or whatever. And we see that again, like with the design tools, you know, someone who can't code can now all of a sudden create basic apps and things like that. And we're able to realize our visions in a way that we were never able to do before.
在正确的道路上,我认为我们正朝着这个方向前进,那就是如何最大化人的自主性和自由,以及实现我们成为最好版本的潜力。今天,我们已经有了一些这样的创造性工具。比如说,我虽然没有很强的艺术能力,但借助AI图像生成等工具,我可以表达有趣的概念或其他想法。我们也在设计工具中看到了类似的情况,比如一个不会编程的人现在也能突然间创建简单的应用程序和类似的东西。我们能够以一种前所未有的方式实现我们的愿景。
A conversation we were having earlier is how we are actually now on the good timeline on how AI is shaping. Yeah, 10 years ago, this is very different view of how AI could turn out. Do you want to kind of talk? Yeah, exactly. So I, you know, I like to think about things on a time, 10 year time scale, in part because, you know, that's kind of how our startups work, roughly speaking, we seed fund them. They come through YC and then 10 years later, they IPO. And so I've been asking a lot of people about your 2035, you know, what do you want to see in 2035? But also thinking backwards to 2015.
我们之前讨论过一个话题,就是现在人工智能的发展走上了一条好的时间线。十年前,人们对人工智能的发展方式有非常不同的看法。你想谈谈这个话题吗?是的,没错。我喜欢从一个十年的时间跨度来思考问题,部分原因是我们的创业公司大概也是按照这样的周期运作的。我们为它们提供种子基金,它们通过 YC 加速器,然后在十年后上市。所以我一直在问很多人关于 2035 年的愿景,你希望在 2035 年看到什么?同时我也在回顾 2015 年的状况。
And so if we go back to 2015, you know, 10 years ago was where we're having discussions inside of YC about artificial intelligence because we believed that we had sort of crossed a threshold. Basically, in the early teens, somewhere around 2012 is where we started to really believe that actually we had broken through. I think everything kind of prior to 2012 was fake, in my opinion. But it was really deep learning that that started to really deliver on AI.
回到2015年,也就是十年前,当时我们在YC内部就人工智能展开了讨论,因为我们相信我们已经跨过了一个重要的门槛。大概在2012年前后,我们开始真正相信我们已经取得了突破。在我看来,2012年之前的一切关于人工智能的成就都有些不真实。而实际上,是深度学习的出现才真正推动了人工智能的发展。
But when we were looking at this 10 years ago in 2015, one of the big questions was, you know, it was all reinforcement learning and what is the thing that we were reinforcing? Because at the time, they were playing video games and trying to make the score go up. And this is, I think, also kind of where the paperclip maximizer concept and fear came from is like, if you gave it the wrong objective function. And so we had a lot of fear that based on our own evolution, our intelligence arose as a survival mechanism that we became intelligent and other animals became intelligent as a way to survive and perpetuate themselves.
但是,当我们在2015年回顾10年前的时候,其中一个大问题是,那时候大家都在关注强化学习,那么我们究竟在强化什么呢?因为当时技术正在打电子游戏,并试图提高分数。而这种情况,也让我想到了“曲别针最大化”这个概念以及由此产生的担忧,即如果我们给了人工智能错误的目标函数会怎么样。因此,我们非常担心就像在我们自己的进化过程中一样,我们的智力是作为一种生存机制而出现的,我们和其他动物变得聪明都是为了生存和繁衍自己。
And we thought that if AI did the same thing, it would, by its very nature, want to wipe us out in order to maximize its own odds of survival. And what's happened in the last 10 years as we actually found the right objective function, which is simply to predict the next token. And that actually intelligence in its most raw form is simply predicting what comes next. And so all of our really at a root level, what we're predicting, what our reinforcement function is simply predicting what comes next.
我们的想法是,如果人工智能也这样做,为了最大化自身的生存机会,可能会想要消灭我们。然而,在过去的十年中,我们找到了正确的目标函数,那就是简单地预测下一个词元。事实上,最原始形式的智能就是预测接下来会发生什么。因此,从根本上讲,我们所做的事情,就是我们的强化函数,也只是简单地预测下一步会发生什么。
And that is the fundamental core of intelligence. And the great thing about that is we've been able to create this intelligence that doesn't have this drive to survive. It doesn't mind that we spin up intelligence and it doesn't work. And then it disappears because it's just based on that ability to predict patterns. I would argue like the most important part of this is actually the agency piece. Venkatesh Rao has this crazy thing he talks about.
这就是智能的基本核心。最棒的是,我们能够创建这种不需要生存驱动力的智能。它不介意我们产生的智能没能奏效,然后消失,因为它仅仅基于预测模式的能力。我认为最重要的部分其实是自主性。Venkatesh Rao对此有一种很独特的看法。
And this is sort of a function of maybe the Uber and DoorDash era of things where in society, there's this like API line. So either you are above the line, meaning you create Uber or you drive for Uber. And obviously that's a distillation of like sort of the last idea. And then in this AI world, basically, if you're below the API line in the old model, like you don't have agency. You sort of have to like play this never ending game. Like the human is being doing the paperclip maximizing.
这在某种程度上反映了像Uber和DoorDash这样的时代特征。在社会中,存在一条“API线”。如果你在这条线上方,意味着你是创建Uber的人;而如果你在这条线下方,你就是为Uber开车的人。显然,这是一种思想的简化。在这个人工智能的世界里,如果你在传统模型中的“API线”下方,你就没有自主权。你必须不断参与这场无休无止的游戏,就像人变成了一个“回形针最大化”的机器。
And so there's this other sort of world that I'm hoping we live in where it's humans not just writing the prompt and then the machine runs software and then this vast machinery and that's it. And you can never change the prompt. Like that would be tyranny probably. It's conceivable that in the future, we might have, and I don't know if this is the right thing, but you know, this sounds like something the EU would do, for instance. It would probably mandate there to be a human in the loop on, you know, maybe the CEO of a company.
所以,我希望我们生活在另一种世界中,这个世界并不是人类仅仅写下一个指令,然后机器运行软件,产生庞大的系统,然后就没有其他了。而且,这个指令再也无法更改。那样可能会是一种专制。在未来,可以想象我们可能会有一些新的规定,我不确定这是不是正确的,但这听起来像是欧盟可能会做的事情。也许他们会要求在过程中始终有一个人参与,比如公司的CEO。
Spoons. Yeah. And that might be the case, right? Yeah. It might be a form of like, we cannot use shovels here. Right. We must use tiny little spoons just for this one part. Right. Yeah. I think kind of the fundamental error they keep making over and over again is taking a very static view of the world. And then essentially trying to disregulate the current structure.
勺子。对。可能情况就是这样,对吧?对。就像,我们不能在这里使用铲子。对。我们必须只用小勺子来处理这个部分。对。我认为他们反复犯的一个基本错误就是用一种非常静态的眼光来看待世界,然后试图打破现有的结构。
And that closes off our ability to evolve and see into the future. And it's, you know, again, very difficult and oftentimes impossible. So, you know, again, going back to 2015, the conclusion of our of our thinking was actually that we needed to create our own AI lab because at the time, you know, all of the best AI work was being done at Google and they had, you know, Google had all the money, all the data, all the users, all the researchers.
这限制了我们进化和预测未来的能力。而且,这通常是非常困难的,甚至很多时候不可能。因此,回到2015年,我们的想法得出的结论是,我们实际上需要创建我们自己的人工智能实验室,因为当时,所有最好的人工智能工作都在谷歌进行。谷歌拥有所有的资金、数据、用户和研究人员。
And it kind of seemed possible that they were going to have essentially an monopoly and it was all going to be locked up inside of that system. And so we had this very sort of like loony moonshot idea to start at the time we called it YC research, but it eventually got renamed open AI. And so open AI, you know, we were going to take on Google with a small nonprofit, which it was sort of doesn't quite pass the laugh test, right?
这段话的大意是,当时我们觉得他们可能会完全垄断市场,一切都会被锁在他们的系统里。于是,我们有了一个非常疯狂的想法,准备启动一个叫做YC研究(后来更名为OpenAI)的项目。我们想用一个小型非营利组织去挑战谷歌,这听起来有点不太切实际,对吧?
Like, how is this little nonprofit, you know, going to be the one that that actually develops AGI when, you know, the other companies have dramatically more resources. And then here we are 10 years later, it actually happened. And at the time, it just seemed incredibly implausible. Like no one would have believed it yet. Here we are on, I think, basically the best timeline.
就像,怎么可能是这样一个小型非营利组织来开发出通用人工智能(AGI),要知道,其他公司有着多得多的资源。而如今,10年过去了,这件事真的发生了。当时,这看起来完全不可能,没人会相信。然而,现在我们确实在经历这一切,我认为这基本上是最好的发展历程。
Like we actually delivered it. We have an open and competitive market with, I would say at least kind of like six, basically, you know, you know, foundation models that are competing, including an open source one from Meta. And I think that's our best shot for preserving freedom is choice and competition. And talking about Google, it actually is digging the traffic too. Do you want to talk a bit about that? About the stats that were? I mean, some of it is like, I don't think it's out there in the annual reports yet. And certainly like we did some, you know, research prior to this episode, we couldn't really find anything that conclusive, but maybe purely anecdotally, because we're in this pool of people who are very, very early adopters, very much software engineers and you know, our behavior interacting with the internet has changed already.
就像我们实际上交付的那样。我们有一个开放且竞争激烈的市场,我会说至少有六个基底模型在竞争,其中包括Meta的一个开源模型。我认为,保护自由的最佳方式就是选择与竞争。关于谷歌,它实际上也在吸引流量。你想谈谈这个吗?关于那些统计数据?我的意思是,其中一些数据我认为还没有出现在年度报告中。当然,我们在这一集之前做了一些研究,但并没有找到确凿的证据。不过,可能纯粹是从个人的经验来看,因为我们处在一个非常早期的用户群体中,他们大多是软件工程师,而我们与互联网互动的方式已经发生了变化。
It's not a surprise to me. Some people are starting to report in their referral traffic. Google referrals are down maybe 15% in the last year. And that certainly probably mirrors my own behavior. Like I still use Google, but I'm increasingly not clicking on any links in Google because they're sort of the snippet at the top. Or the first thing I think of is using chat GPT with web or using perplexity directly. Yeah, exactly. I mean, if you want to understand the future, I think you always have to look at where the early adopters are. And so you say, you know, again, now if we go back 25 years, right, if we go back to the year 2000 or 1999, you know, the early adopters with the people using Google.
这对我来说并不意外。有人开始注意到他们的引荐流量有所变化。过去一年里,来自谷歌的引荐流量可能减少了15%。这也反映了我自己的行为。我仍然使用谷歌,但我越来越不点击谷歌上的任何链接,因为通常我能从顶部的摘录中获取信息。或者我会首先想到使用带网络访问的ChatGPT或者直接使用Perplexity。是的,没错。想要了解未来,你必须关注那些早期使用新技术的人群。所以你可以想象一下,如果我们回到25年前,也就是2000年或1999年,当时的早期采用者是使用谷歌的人。
So at the time, you know, people are like, well, Google is just kind of this fringe thing that, you know, maybe techy people use or something. But at this point in history, the same people or those some kinds of people who are the early adopters of Google are now switching their behavior to where your default action, if you're looking for information, is, you know, chat GPT or perplexity or one of these things. And even just, you know, observing my own behavior, I'll use Google mostly for kind of navigational. Like, I'm just looking for a specific website and I know it's going to give the same thing. But it's starting to have that weird kind of like legacy website, like I'm using eBay or something vibe to it.
在那个时候,人们会觉得谷歌只是一个小众的东西,可能只有一些技术宅才会用。但是在历史的这个阶段,那些曾经是谷歌早期用户的人,现在已经转变了行为习惯,变成在查找信息时首先去用ChatGPT或是Perplexity之类的工具。甚至观察我自己的行为,我现在用谷歌主要是为了导航,比如找一个特定的网站,因为我知道它会给我想要的结果。但越来越多的时候,谷歌给我的感觉像是一个老旧的遗产网站,就好像是在用eBay一样。
Even earlier sign was the drop in traffic for Stack Overflow that actually started back in 2022, even before chat GPT. And this was primarily because of a get up go pilot. And they're down 60% this year. Yeah. Yeah. The pool of people here are quite have quite a good track record of predicting trends, right? If you think of, but the call, I mean, just like technical startup founders at YC, like I remember 2007 sort of Apple was back on the rise, but you could tell because just everybody who is in a YC batch was using a Mac. You could see the rise of AWS and the shift from rack service to everything being in the cloud because all of the founders in the batch just started by using AWS.
更早的迹象是Stack Overflow的访问量下降实际上在2022年就已经开始了,那时甚至还没有Chat GPT。这主要是因为一个叫做 "Get up go" 的计划。而今年,他们的访问量下降了60%。对,这里的这些人确实在预测趋势方面有很好的记录。你可以想到,比如YC(Y Combinator)中的技术初创公司创始人。我记得2007年左右,苹果公司开始重新崛起,但你可以从那些参与YC项目的人都在用Mac看得出来。再比如,AWS的崛起和从机架服务向云服务转变的趋势,也可以从当时参与项目的创始人大多一开始就用了AWS看出。
Same thing now. I've spoken to a bunch of founders and just personal productivity. Like they just have chat GPT open all day that founders like say they just constantly screenshotting their desktop and just like sending it to chat GPT if they need to debug something or figure out how to like navigate a government website. It's like one rather example. Like I need to do set up some registration. They, you know, here's a screenshot. Just tell me like exactly where I need to click in order to do this quickly. The only thing that we saw last year and summer batch was how so much of the batch is using cursor. And is one of the companies that's been growing a lot very quickly.
现在的情况也是这样。我和一些创业者聊过,他们在个人效率方面都有一个共同点,就是一整天都开着ChatGPT。他们经常截取桌面的屏幕截图,然后发给ChatGPT,以便解决调试问题或是搞清楚如何浏览政府网站。例如,有时候他们需要完成一些注册手续,就会把相关页面的截图发送过去,让ChatGPT准确告诉他们该点击哪里,以便快速完成任务。去年夏季班我们也注意到,很多参与者正在使用Cursor这一工具,而这个公司也发展得非常快。
Anig totally they hit 50 million revenue. I think we may mention this in other episode. But yeah, I can't think of another tool that's got adoption so quickly within a YC batch as cursor just went from nothing to from one batch to the other. Like up to like 80% of the batch using it from one to the other one. The previous batch was like single digit percent. There's some people mentioned it felt like a like a technical conference a little bit and a lot of people were trading notes on how to hire the best engineers and a few people said, you know what, like if someone comes in and I asked them if they use cursor or any code gend tools and they say no.
Anig 的总收入达到5000万。我想我们在其他节目中可能提到过这一点。不过,我想不出还有哪个工具能在YC的一期中像Cursor一样迅速被接受,它从无到有发展到80%的团队使用它,而在前一期中这个比例还不到10%。有些人提到,这感觉有点像一个技术会议,很多人都在交流如何招聘到最优秀的工程师。几个人说,如果有人来面试,我问他们是否使用Cursor或任何代码生成工具,而他们回答没有用的话,他们会感到很惊讶。
Right now I can't hire them because they're not going to be able to be as productive as the rest of my team. I think that's an extension of something stripes started a decade ago actually like in general engineering interviews and technical interviews. Most of the value copied Google, I would say it was like whiteboards. Yes problems, which probably made sense for Google Google was looking for, but I think strike for the first around like 2011. I think they started doing this really. We don't really need you to wipe board CS problems. We need to use of develop web apps really fast. And so just give someone a laptop and like the idea was you basically sit in the room and you just like build like a to do list app or whatever you can as quickly as you can. And you basically measured on your max output in those like two or three hours.
目前,我无法雇用他们,因为他们的工作效率可能达不到现有团队的水平。我认为这源于Stripe大约十年前在工程和技术面试方面的某种创新。大多数面试方式都是模仿谷歌的,比如使用白板解决问题,这可能对谷歌来说是有意义的。但是,我认为Stripe大约在2011年开始采取不同的方法。他们不再需要你在白板上解决计算机科学的问题,而是要求你快速开发网络应用。面试的方式就是给你一台笔记本电脑,让你在房间里尽可能快速地开发一个待办事项应用程序或其他任何应用,然后根据你在两三个小时内的最大输出水平来进行评估。
And so I think if you follow that line through then say well it doesn't really matter like if there's whatever tools they use the question is just like the bar moves higher like you've got three hours build what you can build and say you should be able to build a lot more with cursor than before. You still believe that you're sort of looking for fundamentally how clearly can people think or solve hard architecture problems then you're sticking to whiteboards. What do you think this means for SaaS? Because you know one of the crazier things we've been seeing is that Klarna claims that they're not even buying new SaaS tools anymore. They're using CodeGen and not even hiring new engineers anymore using their existing engineering set of people. They're just going to replace all the SaaS tools they use to run their FinTech.
翻译这段内容成中文并使其易读是一个挑战,但我会尽量做到:
"所以我认为,如果你沿着这个思路思考,就会发现无论使用什么工具实际上并不是那么重要。关键在于 '标准提升' 了:你有三个小时,尽可能地建造一些东西,并且借助'光标'应该能够比以前做得更多。如果你仍然相信需要考察人们解决复杂架构问题的能力有多强,那么就会坚持使用白板。那么,你认为这对SaaS(软件即服务)意味着什么呢?因为有一件让人惊讶的事情就是,Klarna(一个金融科技公司)声称他们甚至不再购买新的SaaS工具,而是使用CodeGen(代码生成工具),甚至不再雇佣新的工程师,而是依靠现有的工程团队。他们计划用这种方式替代他们运行金融科技业务所需的所有SaaS工具。"
这段文字大意是在讨论技术和工具的发展如何影响软件行业,特别是SaaS(软件即服务)领域,并举例说明了Klarna公司如何利用新技术减少对传统SaaS工具和新雇员的依赖。
And I definitely heard stories like that. One of the unconference talks was actually specifically about that. This is a company I think we mentioned before is a company called Jerry that is now halfway to $100 million a year in revenue. But a few years ago they were like still burning like five or $10 million a year. They had crazy customer support problems and basically GPT4 dropped. They implemented it and then now it totally changed the way they hire like the prompting itself is actually in the hands of their head of customer support. And so they have a PM, they have the head of customer support, the engineers made it. They don't have to touch it. It's mainly a prompt management and workflow tool and it literally cut their customer support team and their budget for that side by half.
我确实听过类似的故事。在一次非常规会议上,实际上就有一个讲座专门谈到了这件事。这家公司我们之前可能提到过,叫做Jerry公司,现在他们的年收入已经接近5000万美元。但几年前,他们每年还在烧掉大约五百万到一千万美元,遇到严重的客户支持问题。然后GPT-4推出后,他们实施了这个技术,彻底改变了他们的招聘方式。客户支持部门负责人现在直接使用这个工具进行工作。因此,他们有一个产品经理,有客户支持的负责人,工程师们搭建了这个系统,但不需要再动手干预。它主要是个提示管理和工作流程工具,这个系统确实让他们的客户支持团队和相关预算减少了一半。
And it turned a company that was not able to grow and burning $10 million a year to a profitable company that is cash flowing, that is also compounding its growth at north of 50% a year. Which is like a dream scenario. Yeah, this is a great example actually. I think of the way in which AI is creating wealth right because there's a whole category of businesses or products that would not have been economically viable or even possible to create before that are now possible. And so we've actually just like expanded the universe of possible businesses. Yeah, it's never been a better time to be a founder, that's for sure. There's definitely been a vibe shift in the as usual just building companies like this like start with like hiring a number of people, for example, if you're like certainly 10 years ago, the general sense was that if you were growing, if your company was growing fast and revenue was ramping up and you would go and raise around and you would some metric you'd hear a lot was like, how many people are you at?
这段话用中文表达如下:
"这家公司曾经无法增长,每年烧掉1000万美元,但如今已转变为一家盈利且现金流充裕的公司,其增长以每年超过50%的速度复合增长。简直就像一个梦寐以求的情景。这确实是一个很好的例子,说明人工智能是如何创造财富的。因为有许多此前在经济上不可行或无法实现的业务或产品,现在变得可能了。实际上,我们扩展了可能业务的范围。这确实是做创始人最好的时代之一。过去,通常建立公司的过程中,总是有一种气氛的变化,比如一个典型的就是雇佣大量员工。大约十年前,如果你的公司增长迅速,收入快速上升,你会去融资。有一个常常听到的指标就是:你公司有多少员工?"
Like how many people do you hire this year? How many people are you going to hire next year? So like a bit of a vanity metric. It just seems to me now like the companies that are reaching these numbers we're talking about like a millionaire are trying to get to 10 or 15 or 20 are doing it with less people and expect to do it with less people. Which is the new thing. This is why so many of them really haven't even raised a series A, which there's less need for for hiring a lot of people to do a lot of the operations on or maybe going to your analogy Gary, the previous generation of startups had this cause of below the API or above the API.
今年你们招聘了多少人?明年打算招聘多少人?这有点像一种自我表现的指标。现在我感觉,那些实现我们所说的那种“百万”目标的公司,希望达到1000万、1500万或2000万的公司,是用更少的人实现的,并且预计未来也会用更少的人来实现。这是一种新趋势。这也是为什么很多公司甚至没有进行A轮融资,因为不需要招聘大量的人来进行大量运营工作。或者用你的比喻,Gary,上一代的创业公司有“API以下”或“API以上”这样的不同运营方式。
So you had a bunch of people that had to kind of build and operate the API. If you have to build that business like Uber or Lyft, DoorDash, marketplaces, you have to do that hyperscale of hiring lots of people. The funny thing about that era was there's this concept that I think was probably appropriate for that era called Blitzscaling. There was an entire book about it and the idea was basically I think it was born out of this descending interest rate world while at the same time like if you put more money into something like you had these network effects. So if you played that out, yeah, you want to blitz, blitz scale. You want to hire as many people as possible. You want to grow faster than everyone else. And then because of the winner take all dynamic like the world capital markets were just going to funnel you tens of billions of dollars, hundreds of billions of dollars even to your subsidized growth to be the winner.
所以,当时有很多人需要开发和运营API。如果你要建立一个像Uber或Lyft,DoorDash这样的企业市场,你就需要快速扩张,招聘大量员工。有趣的是,那时有一个概念被认为适用于那个时代,叫做“闪电扩张(Blitzscaling)”。甚至有一本书专门讲述这个概念。基本上,它是在低利率的背景下产生的想法,而且如果投入更多资金,就会出现网络效应。应用这种策略,你需要快速扩张,尽可能多地招聘人,争取比其他人更快地增长。因为赢家通吃的市场动态,全球的资本市场会向你倾斜,给你的增长提供数十亿甚至上百亿美元的资金支持,让你成为行业中的赢家。
And that was the game. And I think like from what we can tell from all the people who have more than 300 founders right here sort of sharing their stories and I don't think I heard Blitzscaling or I'm trying to hire as many people as possible like at all. Nobody is bragging about, hey, you know who I'm hanging out with these unicorn. You know, I'm going to be a unicorn. Like people are literally not bragging about that. It's all about leverage right now. Now that the real thing is how much you can do with a little bit of resources because we have these magical tools that give us superhuman leverage. Part of it is like this, there's going to be a longer tale of businesses that are possible only now because of AI. And this longer tale is going to be also fatter. It's not just companies are doing 20, 30 million revenue, but more than hundreds of millions of revenue.
这就是游戏的现状。从我们能了解到的来看,这里的300多位创始人分享了他们的故事,我发现没有人提到“闪电式扩张”或“我在尽可能多地招聘员工”。没有人夸耀说“嘿,我和那些独角兽公司在一起呢”或者“我将成为独角兽”。人们实际上并没有在炫耀这些。现在的关键在于杠杆效应。真正重要的是,你能用少量资源做多少事情,因为我们有这些神奇的工具,给了我们超人的杠杆效应。部分原因是,现在由于人工智能的出现,有更多可能的业务可以实现。这种业务的“长尾”不仅会更长,也会更粗壮。不仅有公司能达到2000万到3000万的收入,还有更多公司能达到数亿美元的收入。
And it goes back to the episode when we talked about vertical sass. There's just more willingness to pay for this new category that people are still trying to figure out how to price. That's why there's just so much willingness to pay because people want it. It doesn't go just on the software budget for a company is there's budget from the AI chief officer or something. I don't know if that's like a title that has come out yet, but I really made this point to that. I mean, one thing I'm certainly noticing is that the companies are hitting these big revenue numbers, trying to sign these contracts. It's actually sort of usage based pricing. I think the data is not necessarily the pain per use, but the pricing is tight like how much used product, which is definitely how it's close to how you would think about it as like selling services and software per se. Obvious ROI. Yes.
这可以追溯到我们讨论垂直SaaS的那一集。对于这个人们仍在摸索定价的新类别,大家的支付意愿更强。这也是为什么大家愿意花钱购买,因为他们需要它。这不仅仅来自于公司的软件预算,还有可能来自一些比如“AI首席官”这样的角色的预算(虽然我不确定这个职位是否已经出现)。我真的提到了一点,我正在注意到公司达成大额收入合同的方式往往是基于使用的定价。我认为数据不一定是按次付费,但定价紧密相关于产品的使用量,这其实就像出售服务和软件一样,显然有很高的投资回报。
So the problem a lot of times with selling a product is the customer doesn't really know if they're getting the ROI. And so that makes for a long and painful sales cycle. But if you're able to drop in something that pays for itself in the same month, that's an easy sale. Right. I think the way they've kind of priced it is more like services and it's really akin to this is how intelligence is getting priced. So another on the spectrum of people thinking not worrying about just the big picture is AI going to make us all obsolete. And one and the other and existential philosophical conversations. Some of the stuff I thought that's interesting in the middle is it's just hard to predict the timeline of the tools themselves.
很多时候,销售产品的问题在于客户并不清楚他们是否能获得投资回报(ROI)。这就导致了销售周期很长而且痛苦。但是,如果你能够提供一种在当月就可以收回成本的产品,那销售就容易多了。我认为他们的定价方式更像是服务收费,这实际上类似于智能技术的定价方式。另一方面,在人们担心“大局观”的同时,比如担心人工智能会让我们变得无关紧要,还有一些哲学层面的讨论。有趣的是,这中间的一些东西让人觉得很难预测工具本身的发展时间表。
So there was some interesting talks about like rag, for example, I think Sam maybe seeded this with his talk about like, if you have like infinite context, or huge context windows, do you even need like rag or retrieval tools at all? And I think that's like, that's the kind of thing where it's like, it's as a startup or a builder right now. I think people are more concerned about, am I using the right tools and like, is this going to still make sense in three to six months? I think that's like actually a direct consequence of like, you know, if you're an AI lab, you're like on the frontier. And so how you know that your thing is working is actually like your model is bigger. You're like farther along on the scaling law.
有一些有趣的讨论,比如关于RAG(检索增强生成)之类的。我觉得可能是Sam在他的演讲中提出了一个问题:如果我们有无限的上下文或者巨大的上下文窗口,那么我们还需要RAG或者检索工具吗?我认为这对目前的初创公司或开发者来说尤为重要。现在人们更关心的是:我是否在使用正确的工具,以及这些工具在三到六个月后是否仍然有意义。我认为这实际上是因为,作为一个人工智能实验室,你正处在前沿地带。判断你的工作是否有效的一个标准就是,你的模型规模更大,或者你在扩展规律的进程中走得更远。
And so when I meet people from AI labs, like they almost all talk about bigger, better models, but they're model makers. And then obviously, you know, we also spend a lot of time with very scrappy founders who have very little capital. There are just as many talks sort of on the other side, which was, you know, I went to one that was very much about systems level programming. Like, if you want to have, I think it was a Tavis. So Tavis is building this real time AI avatars with video and audio that are very realistic. Part of the trick is they got it to very low latency. 600 milliseconds, which is really fast. Which was even too fast that some of the customers, oh no, no, no, it's too fast. It's a bit uncanny when it's too fast.
当我遇到来自 AI 实验室的人时,他们几乎都谈论更大、更好的模型,因为他们是模型的创造者。当然,我们也花了很多时间与资金很少但充满干劲的创业者交流。同样多的讨论也存在于另一个方面,比如我去参加了一个关于系统级编程的会议。我记得是一个叫 Tavis 的公司,他们正在构建一个具有实时 AI 动画和音视频的虚拟形象,效果非常逼真。他们的一个技术诀窍是将延迟降低到了 600 毫秒,非常快。甚至快到一些客户觉得太快了,有点令人不安。
It's like now it's being rude. It's just interrupting me. So a lot of the build SDK for other companies. So a lot of the products that are getting built with this zoom video interface with another human, it is using them. So I love their talk because it's a good illustration of like, yes, like the labs are going to continue to do their thing. You know, and maybe on a more fast timeline than we even imagine, like, you know, nine months, 18 months, you know, maybe it's even every three months. There's sort of these like breakthroughs.
这段英文大意是:现在感觉有点像不礼貌了,总是打断我。许多公司正在开发SDK(软件开发工具包),很多产品都在使用这个Zoom视频界面来与其他人进行互动。所以我很喜欢他们的讨论,因为它很好地说明了实验室仍然会继续推进他们的研究,也许速度比我们想象的还要快,比如9个月、18个月,甚至可能每3个月就会有突破。
So if I had to guess, like that's sort of, you know, when people are in their heads being like, why should I do any of this? Because open AI models are just going to be infinitely smart and I should just lie down on a bed. But you know, what I would say is like, I'm actually heartened by all the stories that I heard. Like, will the models change? Will the technology change? Will, you know, will Tavis change at stack?
所以如果让我猜测的话,这就像是,当人们在思考“我为什么要做这些事情呢?因为OpenAI的模型会变得无比聪明,我是不是应该放弃努力”时的想法。但是,我想说的是,我其实从听到的各种故事中感到振奋。模型会改变吗?技术会改变吗?Tavis在Stack会改变吗?
Like, yes, like they've already seemingly rewritten their stack multiple times to take advantage of what's been going on. Their product has only gotten better in the marketplace as time goes on. And then what will it look like? Will there be like, you know, a model model? Maybe not the same AI labs today that are talking about, you know, there's going to be a trillion token context.
当然,他们似乎已经多次重写了他们的技术栈,以利用当前的发展。随着时间的推移,他们的产品在市场上变得越来越好。那么,未来会是什么样子呢?会不会有一个“模型模型”?可能不像今天的一些人工智能实验室说的,会有一个支持万亿个令牌的上下文环境。
It's like, man, how much is that going to cost? Ultimately, engineering and systems like those matter. Those are actually the most valuable things right now. And then along the way, you're going to have these golden e-vals. I don't know, I hate to bring in, you know, consulting speak, but like, what are the modes, right? And the modes in the end are brand.
这就好比说,天哪,这要花多少钱?最终,工程和类似的系统是很重要的。那些才是真正当下最有价值的东西。而在这个过程中,你会遇到这些宝贵的评估。我不太想用咨询行业的术语,但这种模式是什么,对吧?最后的模式就是品牌。
It's a data that no one else has. Sometimes it actually literally is caring about customers that, you know, the giant company will never care about, right? Actually, I think the other mode is going to be ultimately start-ups move quickly. One of the remarkable things that I observed, a lot of the founders actually have rebuilt a lot of their tech stack.
这是一种别人没有的数据。有时候,这实际上是对顾客的关心,大公司是不会关注的,对吧?其实,我认为另一种模式是创业公司能够快速行动。我观察到的一个显著现象是,许多创始人实际上重建了他们的大部分技术架构。
To be with the latest, they were very willing to, oh, this particular approach to rag doesn't work or vector database, throw it away. PG vector became the better thing. Let's use that and just throw it away and use the best thing. So what was fun to see is I think the best start-ups are going to be the ones that can build the fastest and be willing to be at the bleeding edge and be willing to reevaluate assumptions on what's the best approach.
为了保持最新,他们非常乐意舍弃过去的方法。例如,如果某种做法或者矢量数据库不再有效,就果断抛弃它。PG vector 变成了更好的选择,那就用它,抛弃旧的,追求最优。令人兴奋的是,我认为最成功的初创公司将是那些能够快速构建并愿意站在技术前沿,同时愿意重新评估什么才是最佳方法的公司。
And I heard a lot of how a lot of how things got built. They redo it or they do it again with the best with the latest and best. We should also explain another reason why they're securing enterprise contracts and these big contracts fast and ever, right? Like big companies have never been great at continuing to build great software.
我听说了很多关于事物如何建造的过程。他们重新做或者用最新最好的技术来重做。我们还应该解释他们能快速获得企业合同和大合同的另一个原因,对吧?就像大公司从来不擅长不断开发出优秀的软件。
But now like, yeah, if you need to constantly rip and replace the tool you're using every three months to be like at the bleeding edge, like it's going to take three months to get like the meeting schedule to discuss like whether we should reevaluate the rules. We're going to plan in the next print.
这段英文的大意是:如果你需要每三个月就更换一次工具以保持在技术的最前沿,会非常麻烦。光是要安排一个会议来讨论是否需要重新评估规则就可能需要三个月的时间。这样一来,我们只能在下一次计划时再做调整。
翻译成中文,这句话可以表达为:但现在如果你需要每三个月更换一次工具来保持在技术前沿,这样做很麻烦。单单是安排讨论是否重新评估这些规则的会议,可能就需要三个月。我们只能在下一个计划周期中再考虑这个问题。
We're going to take it in whatever. We can get to that in like, you know, 2029 for sure. Yeah, and these companies are getting to the six or 12 million example. I know they actually have rewritten a lot of their tech stack a lot of times and the architect every time I actually talk to them.
我们会随遇而安。到2029年的时候,肯定能实现这些目标。是的,这些公司正在朝着六百万或一千二百万的目标努力。我知道,他们实际上已经多次重写了他们的技术架构。每次我和他们交流时,他们都在改进。
Oh, yeah, we threw away that thing that we told you like this new way of doing is like, okay. And that's like every month, every other month. From talking to founders this weekend, what was your sense of the overall vibe? It's pretty exciting. I mean, I don't know that there's ever been a better time.
哦,对,我们把之前告诉你的东西扔掉了,我们发现这种新方法还不错。而且几乎每个月或者每隔一个月都是这样。这个周末和创始人们交流时,你对整体氛围有什么感觉?很让人兴奋。我觉得可能没有比现在更好的时候了。
You know, again, just kind of looking back historically, really the foundation of YC. If we jump back not 10 years to when we were starting OpenAI, but 20 years to the summer founders program, the thesis behind why Paul and team started. YC was the realization that it was getting easier to build startups.
从历史上看,YC的基础确实值得回顾。如果我们不回到10年前OpenAI刚成立的时候,而是回到20年前的夏季创始人计划,那么保罗和他的团队创建YC的初衷就会更加清晰。他们认识到,创业变得越来越容易。
You didn't need to raise a mountain of capital and hire a giant team that actually just a couple of smart kids could build a web app. And that trend has only accelerated now with AI, where you can build an entire 12 million dollar business or something with just a handful of employees.
你不需要筹集大量资金,也不需要雇佣庞大的团队,其实只需要几个聪明的年轻人就能开发一个网络应用。而现在随着人工智能的发展,这种趋势更加明显,只需要少数员工就能建立一个价值1200万美元的企业。
And so it again goes back to technological leverage enables people who have sort of ambition and insight to do incredible things. Well, that's all we have time for today, but we'll catch you next time on the Litecoin.
这句话的大意是:技术杠杆使那些有抱负和洞察力的人能够完成令人难以置信的事情。今天我们的节目就到这里,下次再见,《莱特币》节目不见不散。