Ep4. Tesla FSD 12, Imitation AI Models, Open vs Closed AI Models, Delaware vs Elon, & Market Update
发布时间 2024-03-07 16:28:51 来源
摘要
Open Source bi-weekly convo w/ Bill Gurley and Brad Gerstner on all things tech, markets, investing & capitalism. This week, they ...
GPT-4正在为你翻译摘要中......
中英文字稿 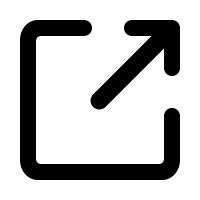
I would make the argument that every company in Delaware has to move to a different domicile because they could be sued in a future derivative lawsuit for the risk they've taken by staying in Delaware. Oh my God. You're so right. You are so right. Oh, mic drop on that. Oh, mic drop on that. Hey, Bill, great to see you. I mean, people loved when you were here last weekend person, so we got to make that happen again. But now where are you? Looks like you're in Texas somewhere. I'm back in Texas. Yes. Yeah. All right. All right. So what's on your mind? A lot of action the last couple of weeks. What's going on? One thing that I've reflected on quite a bit is just kind of how lucky we are to be a part of the venture capital industry and the startup world simply because things change so fast. And if you're a curious person, if you're someone that likes constant learning, it's really amazing. Like the stuff we're talking about, the stuff I'm listening to podcast on every day, you know, two years ago didn't exist. And now it's 80 or 90 percent, 80 or 90 percent of the dialogue. And that's just pretty well. Yeah. No, it's a, you know, our brains really aren't programmed to work in kind of these exponentials. Right. I mean, you and I both know every sell side model on Wall Street has linear deceleration and growth rates. Like we think really, you know, we're really good at thinking that kind of these linear ways. I had that thought this morning that, you know, the biggest investment opportunities really do occur around these phase shift moments. I mean, Satya talks about all the value capture occurs in the two to three year period around phase shifts, but it's hard to forecast in those moments, right? I mean, that's when you see these massive deltas, you know, in these, in these forecasts. And I just went back and looked at, for example, at the start of last year, the consensus estimate of the smartest people covering Nvidia day to day was that the data center revenue was going to be 22 billion for the year. Right. Guess what it ended up being? 96 billion. Wow. Okay. They were off almost by a factor of three or a four, right? The EPS at the beginning of last year, the earnings per share was expected to be $5.70. And now it looks like it's going to be $25. Right. Over the course of your career, have you ever seen sell side estimates off by that much on a large cap stock? I mean, just like, you know, very, very rare, like, you know, once a decade, maybe, you know, that something like this happens. Yeah. So, you know, and I've had investors say to me when the stock was at 200. Hell, you and I talked about this. You know, should we sell it all at 200 sell it all at 300 sell it at 400. And now, you know, those investors are calling me every day saying, have you, you know, have you sold it yet? Our general view is that if the numbers are going up, so if our numbers are higher than the streets number for whatever variant perception that we have, right, then the stock is going to continue to go higher. At some point, the street will get ahead of itself and its numbers will now be higher or at the same level as ours. And at that point, I think it becomes more of a market performer.
我认为每家在特拉华州的公司都必须迁移至其他住所,因为他们可能在未来的衍生诉讼中被起诉,原因是他们选择留在特拉华州所承担的风险。天啊,你说得太对了。你说得太对了。哦,这真是绝妙的想法。哦,这真是绝妙的想法。嘿,比尔,很高兴见到你。上周末你在这里的时候人们都很喜欢,所以我们必须再次让这种情况发生。但现在你在哪里?看起来你在德克萨斯某处。是的,我回到了德克萨斯。是的。好的。那你有什么想法吗?过去几周发生了很多事情。有什么事情吗?我反思过很多次的一件事就是我们有幸成为风险资本行业和初创企业界的一部分,仅仅因为事物变化如此之快。如果你是一个好奇的人,如果你是一个喜欢不断学习的人,那真是太惊人了。我们现在谈论的事情,我每天都在听的播客,两年前都没有存在过。现在它占据了对话的80%或90%。这很不错。是的。我们的大脑真的不适合在这种指数方式下工作。我是说,你和我都知道在华尔街的每份机构研究报告都有线性减速和增长率。我们真的很擅长以这种线性方式思考。今天早上我就想到了,最大的投资机会确实发生在这些变革时刻周围。我是说,萨蒂亚谈到了所有的价值捕获都发生在变革时刻的两三年里,但在那些时刻很难进行预测,对吧?我是说,那时你会看到这些预测中的巨大差距。举个例子,去年年初,覆盖英伟达每日智库的最聪明人士的共识估计是数据中心的收入将在整年达到220亿美元。猜猜最终的结果是多少?是960亿美元。哇。他们几乎错了三到四倍,对吧?去年年初,每股收益预期为5.70美元,而现在看起来将是25美元。在你的职业生涯中,你见过大型股票的机构卖方估计如此出错吗?我是说,这种情况非常罕见,可能每十年才会发生一次。是的。所以我遇到过投资者当股价达到200美元时对我说,你和我当时都讨论过这个。我们应该在200美元全部抛售,或者在300或400美元抛售。现在这些投资者每天都在打电话给我,问我们有没有卖出去。我们的一般观点是,如果我们的数字比市场的数字更高,无论我们的变异看法是什么,股价就会继续上涨。在某个时候,市场会变得过度乐观,它的数字将会变得更高或者与我们的水平持平。到那时,我认为它将更多地成为一个市场表现者。
But of course, some things will be wildly overestimated and some things will be wildly underestimated. But they, that, that sort of discontinuity really occurs around these moments of big face shifts. So speaking of a big face shift, right? We teased on the pod, I think at the start last time that I had taken a, you know, a test ride in in Tesla's new FSD 12. And I said, you know, kind of felt like a little bit of a chat GPT moment. But I think we left the audience hanging. We got a lot of feedback. Hey, you know, dig in more to that. So you and I spent some time on this both together and with the, with some folks on the Tesla team. So roughly the setup here, background, I want to get your reaction to it is about 12 months ago, the team pretty dramatically for their self driving model, right? Moving it from this really C plus plus deterministic model to what they refer to as an end to end model. That's really driven by imitation learning, right? So we think of this new model. It's really video in and control out. It's faster. It's more accurate. You know, but after 11 different versions of FSD, I think there's a lot of skepticism in the world.
当然,有些事情会被大大高估,而有些事情会被大大低估。但这种不连续性主要出现在重大转变的时刻。说到重大转变,我们上一次在节目中提到,我曾经试乘了特斯拉新的FSD 12。我说,感觉有点像聊天GPT的时刻。但我觉得我们让听众悬在了半空中。我们收到了很多反馈,最后听众都说,更深入地探讨一下。所以我们一起花了一些时间,与特斯拉团队一起进行了讨论。大概的背景是,大约12个月前,团队对他们的自动驾驶模型进行了一次相当大的调整,从这种C ++的确定性模型转变为他们所称的端到端模型。这个新模型主要是基于模仿学习的视频输入和控制输出。它更快,更准确。但在经历了11个不同版本的FSD之后,我觉得世界上有很多人对此持怀疑态度。
Like is this going to be, you know, something different? You sent me a video and I have tons of these videos, you know, floating around at the moment, you know, that really kind of shows, you know, how this acts more like a human than prior models out there. So Bill, kind of just react. You know, you've watched this video, reacted this video and give us your thoughts. You know, I think you've been a long time observer of self driving. I might even describe you as a bit of a critic of, you know, or a skeptic when it comes to full self driving. So is this a big moment that I overstayed it? And kind of what are your thoughts here?
这会是一些不同的东西吗?你给我发了一个视频,我手头有很多这样的视频,现在正在流传,实际上显示,这比以前的模型更像一个人。所以,比尔,只是简单地回应一下。你看了这个视频,对这个视频做出反应并给出你的想法。我认为你是自动驾驶的长期观察者。当谈到全自动驾驶时,我甚至可能把你描述为一个批评者或怀疑论者。这是一个我夸大了的重要时刻吗?你有什么想法?
Yeah, so, you know, one of the critiques and concerns people had about self driving is they would say that, yeah, we're 98% of the way they are 99, but the last 1% is going to take as long as the first 99. And one of the reasons for that is it's nearly impossible to code for all of the corner cases. And the corner cases are where you have problems. That's where you end up in wrecks, right? And so the approach Tesla had been taken up until this point in time was one where you would literally try and code every every object, every every circumstance, every case in like a piece of software. This X happens then why, right? And that ends up being a patchwork kind of a just a big nasty, you know, rat's nest of code and it builds up and builds up and builds up and maybe even steps on itself. And it's not very elegant.
是的,你知道的,关于自动驾驶的批评和担忧之一是人们会说,是的,我们已经完成了98%,它们是99%,但最后的1%将花费和前99%一样长的时间。其中一个原因是几乎不可能为所有边界情况编写代码。而边界情况正是你会遇到问题的地方。那就是你最终会发生事故的地方,对吧?所以,到目前为止特斯拉采取的方法是,你实际上会试图为每一个对象、每一个情况、每一个案例在软件中写代码。这种情况发生那么为什么,对吧?最终,这结果会变成一种拼凑的一种庞大的、混乱的代码,积累起来,可能甚至会相互冲突。这样的代码并不十分优雅。
What we learned this week is that they've completely tossed all of that out and gone with a neural network model where they're uploading videos from their best drivers. And literally the videos are the input and the output is the steering wheel, the brake and the gas pedal. And you know, there's a there's there's this principle known as Occam's razor, which has been around forever in science. But the the simplified version of it is a simpler approach is much more likely to be the optimal approach. Right. When I fully understood what they had done here, it seems to me this approach has a much better chance of going all the way and of being successful and and certainly of being maintainable and reasonable.
本周我们学到的是,他们完全放弃了以往的模型,转而采用了一个神经网络模型,他们从他们最优秀的司机那里上传视频。实际上,这些视频是输入,输出就是方向盘、刹车和油门。你知道,有一个叫做奥卡姆剃刀的原则,它在科学界已经存在很久了。但简化版本就是,简单的方法更有可能是最优方法。当我完全理解了他们在这里所做的事情后,我觉得这种方法更有可能取得成功,并且肯定更容易维护和合理。
It's way more elegant. It requires them to upload a hell of a lot of video, which we can talk about. And the other thing that's just so damn impressive is that this company, which is very large, hundreds of thousands of employees, made a decision so radical to kind of throw out the whole thing and start afresh. And it sounds like the genesis of that may have been, you know, three or four years ago, but but they got to the point where they're like, this is going to this is going to be way better and through the whole thing out. And I think about four months after they made the change, Elon did a drive where he uploaded and kind of streamed the drive so we can put that in the notes and people can watch it. But it's way, way different. It's way, way different. And in my mind, you know, basically with this Occam razor's notion, it's got a much higher chance of being wildly successful.
这更加优雅。这要求他们上传大量视频,我们可以讨论一下。另一件令人印象深刻的事情是,这家规模庞大的公司,数十万员工,做出了如此激进的决定,彻底放弃原有的一切,重新开始。听起来这个决定的源头可能是三四年前,但是他们最终意识到,这样会更好,于是彻底改变了一切。在他们做出改变大约四个月之后,埃隆进行了一次驾驶测试,上传并直播了这个过程,我们可以将这放在记录中,让人们观看。但是现在已经是完全不同了,变化非常大。在我看来,基于奥卡姆剃刀原理,这种方式成功的可能性更高。
Yeah, let's dig in a little bit into how it's different. Right. And you referenced a little of this. So, you know, like for example, this model does not have a deterministic view of a stop light, right? I mean, Kaparthi has talked about this before, you know, before you have to label a stop light, right? So you would basically take the data from the car. That would be your perception data. You would draw a box around a stop light. You would say this is a, you know, this is a stop light so that your first job on the car would have to be to identify that you're at a stop light. Then the second thing is you would write all of this C++ that would deterministically say when you are at a stop light, here's what the controls should do, right? And so for all of that second half of the model, you know, the heuristics, the planning and the execution, that was all driven by this patchwork that you're talking about. And that was like you would just chase, you know, every one of these corner cases and you could never solve them all.
是的,让我们稍微深入一点看看它的不同之处。你提到了一点这方面的内容。比如,这个模型并没有一个确定性的交通灯观点,对吧?我是说,Kaparthi以前讨论过这个,你知道,在你必须标记一个交通灯之前,对吧?所以,你基本上会从车里取数据。那就是你的感知数据。你会在交通灯周围画一个方框。你会说,这是一个交通灯,这样你在车上的第一步工作就是要确定你在一个交通灯前。然后第二步就是你会写所有这些C++代码来明确地说明当你在一个交通灯前时,控制器应该做什么,对吧?因此,在模型的后半部分,也就是那些启发式、规划和执行的部分,都是由你所说的这些拼凑而成的。你只能不断地追踪每一个这样的特殊情况,你永远也无法解决他们全部。
Now in this new model, it's pixels in. So the model itself has no code. It doesn't know this is a stop light per se. In fact, they just watched the driver's behavior. So the driver's behavior is actually the label. It says when we see pixels like this on the screen, here's how the model should behave, which I thought is just an extraordinary break. And I don't think there's a deep appreciation for the fact that, you know, again, because we've had 11 versions of what came before it, those were just slightly better patchwork models. In fact, I think what, what, you know, we learned was that the rate of improvement of this is order of magnitude five to 10 X better per month as a model versus the rate of improvement of those prior systems. And once again, the audacity to throw out the whole old thing and put a new thing in is just crazy.
在这个新模型中,是以像素为基础的。所以这个模型本身没有代码。它并不知道这是一个红绿灯。事实上,他们只是观察司机的行为。因此,司机的行为实际上就是标签。它表示当我们在屏幕上看到这样的像素时,模型应该如何行为,我认为这是一个非凡的突破。我认为人们并没有深刻地意识到,你知道,因为在这之前我们已经有了11个版本,那些只是稍微更好的拼凑模型。事实上,我认为我们学到的是这种模型的改进速度与之前系统的改进速度相比每个月要快五到十倍,再一次以大胆的姿态将整个老系统抛弃并投入一种新系统的做法实在是疯狂的。
One thing for the listeners, well, actually two things I would, I would mention one in terms of just how they got this going. You know, a lot of people I fear equate AI with LLMs because it was really the arrival of chat GPT and the LLM that I think introduced what AI was capable of to most people. But that, those are language models. That's what the L one of the L stands for. And these AI models that Tesla's used for, for FSD 12 are these generic open source AI models that you can find on hugging face, you know, and, and they obviously customize them. So, so there's, there's some proprietary code there at Tesla, but, but, you know, AI's been evolving for a very long time. And this notion of neural networks was around before the LLMs popped out, which is why I know they had started on this four years ago or whatever, right? But the foundational elements, you know, are there and by the way, they use, they use the hardware that we're talking about, right? They use the big Nvidia clusters to do the training. They need some type of GPU or TPU to do the inference at runtime. So, it is the same hardware the LLMs use, but it's not the same type of code. I just thought that was worth mentioning and.
对于听众来说,我想提到两件事,第一件是关于他们如何启动这个项目。很多人害怕将人工智能与LLMs等同起来,因为聊天GPT和LLM的出现真正向大多数人展示了人工智能的能力。但LLMs只是语言模型的一种。这是L的含义。特斯拉在FSD 12中使用的这些人工智能模型是可以在Hugging Face上找到的通用开源人工智能模型,并且他们显然进行了定制。因此,特斯拉拥有一些专有代码,但是人工智能已经发展了很长时间。神经网络这个概念在LLMs出现之前就已经存在了,这也是我知道他们四年前就开始研究这个项目的原因。基础元素已经存在,而且顺便说一下,他们使用了我们所谈论的硬件,他们使用大型NVIDIA集群进行训练,他们需要某种类型的GPU或TPU在运行时进行推理。因此,它使用了与LLMs相同的硬件,但不是相同类型的代码。我认为值得一提。
Yeah, no, it's a, it's a, to me, if we dig in a little bit to, you know, the model itself, you know, the transformers, the diffusion architecture, the convolution neural nets, those are all like these modular open source building blocks, right? Like the thing that's extraordinary to me, and we're going to get later in the pod to this open versus closed debate, but like, this is just this great example. You know, you talk about ideas having sex. I mean, these, these open source module, you know, kind of modular components, those have been worked on for the last decade.
是的,对我来说,如果我们稍微深入探讨一下模型本身,你知道,变压器、扩散架构、卷积神经网络,这些都像是这些模块化的开源构建基块,对我来说,令人惊奇的是,我们将在后面的对话中讨论开放与封闭之争,但是,这只是一个很好的例子。你说过有一种想法能互相交流,这些开源模块化的组件已经被研究了十年。
And now they're bringing those components together. And now all of their energy, and I want to dig into this a little bit that is really going, they're taking all these engineers who were writing the C++, these deterministic, you know, patches effectively. And now they're focusing them on how do we make sure that our data infrastructure, that the data that we're pulling off of the edge comes in and makes these models better. So all of a sudden it becomes about the data, because the model itself is just digesting this data, brute forcing it with a lot of this, you know, Nvidia hardware and outputting better models. You know, it's such a classic Silicon Valley startup thing where you need all the pieces to line up. So if you go back and watch, if you haven't watched it, if anyone's watched the general magic video, which is fantastic, it's on the internet about why general magic didn't work. And Tony Fidel, who ended up building the iPod and ran engineering for the iPhone, talks about how the pieces just weren't there. So they were having to do all the pieces, right? Right. The network and the chips, and it just wasn't there yet.
现在他们正在将这些组件结合在一起。现在所有的精力都集中在这一点,我想稍微深入探讨一下,他们正在汇集所有这些写C++代码的工程师,有效地修补漏洞。现在他们把重点放在如何确保我们的数据基础设施,我们从边缘获取的数据能够让这些模型更好地运行。所以突然之间,问题变成了关于数据,因为模型本身只是在消化这些数据,并利用大量的英伟达硬件进行 brute force 计算,输出更好的模型。这就是典型的硅谷初创公司的特点,你需要让所有的组件都能顺利配合。所以如果你回过头去看,如果你还没有看过的话,如果有人看过了关于为什么原始的幻想没有成功的那个视频,那真是太棒了,这段视频可以在互联网上找到。托尼·费德尔最终建造了iPod并领导了iPhone的工程部门,谈到了各个部分都不完整的情况。因此他们不得不承担所有的工作,对吗?网络和芯片,它们还没有准备好。
And so these models have been around, maybe ahead of the hardware. And now Nvidia is bringing the hardware and these pieces start to come together. And then the data, like, and I think one of the most fascinating things about this story of Tesla and FSD 12 is when you understand where they get the data. So they are tracking their best drivers with five cameras. And the drivers know it. They've opted into the program. And they upload the video overnight. And so, you know, talk about the pieces coming together. We found Reddit forums and stuff we can put, we can put links to in the notes where users are, our Tesla drivers are saying they're uploading 10 gigabit a night. And so, you know, you had to have the Wi-Fi infrastructure that like, like, how would it be possible to upload that much? Here's someone who's Tesla uploaded 115 gigabyte in a month, right?
因此,这些模型可能已经存在了,或许先于硬件。现在,Nvidia正在引入硬件,这些部件开始融合在一起。然后是数据,比如说,我认为特斯拉和FSD 12这个故事最迷人的地方之一是当你了解他们从哪里获取数据时。他们通过五个摄像头跟踪他们最优秀的司机。司机们知道这一点。他们选择加入这个计划。他们在夜间上传视频。因此,你知道,谈论这些部件的融合。我们发现了Reddit论坛和一些可以在笔记中放链接的东西,用户,我们的特斯拉司机们表示他们每晚上传10吉比特。因此,你必须有Wi-Fi基础设施,就像,如何可能上传这么多?这里有人的特斯拉一个月上传了115吉字节。
And so these are massive numbers. And the infrastructure five years ago, your car couldn't have done this. And you know, I think we'll talk about competition in a minute, but like, you know, who else has the capacity to do this, right? It's unbelievable to like the footprint of cars they have. And then the notion that, oh yeah, we could just go upload this data and it is a buttload of data that's coming out. Right. And even with this architecture, so you just do the math, 5 million cars, 30 miles a day, I think eight cameras on the car, five megapixels each, and then the data going back 10 years, right? This amount of shadow data, you could combine the clusters of every hyperscaler in the world and you couldn't possibly store all of this data, right? That's the size of the challenge.
因此,这些数字是巨大的。五年前的基础设施,你的车是无法做到这一点的。我认为我们会在一会儿谈论竞争,但是,你知道,还有谁有这种能力能做到这一点呢?他们车辆的数据量是相当惊人的。而且还有一种观念,哦是的,我们可以上传这些大量的数据,对吧?甚至在这种架构下,所以你做一下数学计算,500万辆车,每天30英里,我想每辆车上都有8个摄像头,每个摄像头都是五百万像素,然后数据回传十年,对吧?这种阴影数据的数量,你把全世界所有的超大规模数据中心的集群合并起来,也不可能储存所有这些数据,对吧?这就是挑战的规模。
So what they've had to do is process this data on the edge. And in fact, I think 99% of the data that a car collects never makes it back to Tesla. So you know, they're using video compression, these remote send filters. They're running, you know, neural nets and software on the car itself. So basically they, you know, for example, if 80% of your driving is the highway and it's there's nothing interesting that happens on the highway, then you can just throw out all that data. So what they're really looking for is, you know, what is the data that is a long way away from the mean data, right? So what are these outlier moments? And then can we find 10, 10s or hundreds or thousands of those moments to train the model? So they're literally pulling this compressed filter data every single night off of these cars.
因此,他们不得不在边缘处理这些数据。事实上,我认为汽车收集的99%数据从未传回特斯拉。所以他们使用视频压缩,远程发送过滤器。他们在车辆本身上运行神经网络和软件。基本上,他们,例如,如果你80%的驾驶都是在高速公路上,而在高速公路上没有任何有趣的事情发生,你就可以丢弃所有那些数据。所以他们真正寻找的是,你知道,离均值数据有多远的数据,对吗?那些异常时刻是什么?然后我们能找到10、几十甚至几千个这样的时刻来训练模型吗?所以他们每晚从这些车辆上拉取这些压缩过滤器数据。
They've built an autonomous system. So before they would have engineers look at that data and say, okay, what have we perceived here now? How do we write, you know, this patchwork code? Instead, this is simply going into the model itself. It's fine tuning the model. And they're constantly running this autonomous process of fine tuning these models. And then they're re uploading those models back to the car. Okay. This is why you get these exponential moments of improvement, right? That we're seeing now, which then brings us back to build this question. You know, Tesla has five million cars on the road. They have all this infrastructure. They have, they are collecting this data. We know there are a couple of years ahead. Think about Waymo, for example, they're still using the old architecture. It's geo fence. I don't know. They have 30 or 40 cars on a road and they're only running the. So do they have any chance? Does Waymo have any chance of competing or even adopting this architecture? It'd be, it'd be a, it's such an interesting question.
他们建立了一个自主系统。所以以前他们会让工程师查看那些数据,并说,好的,我们现在理解了什么?我们应该如何编写这些拼接代码呢?相反,现在这些信息直接输入到模型中。他们在进行模型的微调。他们不断地运行这个自主的微调过程。然后他们将这些模型重新上传到车辆上。这就是为什么我们现在看到这种指数级改进的时刻,这也让我们回到这个问题,你知道,特斯拉有五百万辆车在路上。他们拥有所有这些基础设施。他们在收集这些数据。我们知道他们领先几年。想想Waymo,比如他们仍在使用旧的架构。它是地理围栏。我不清楚。他们路上只有30或40辆车,他们只是在运行这个。那么他们有机会吗?Waymo有机会竞争或者采用这种架构吗?这是一个非常有趣的问题。
And by the way, just on one quick comment on the previous thing, you said, it's genius actually that they are, they've talked a car. What moments it should record? And so they, they, they mentioned to us an example of any time there's, you know, well, obviously a disengagement. So a disengagement becomes a moment where they want the video before and the video after. The other thing would be any abrupt movement. So if the, if the gas goes fast or if the brake has hit quickly or if the steering wheel jerks, that becomes a recordable moment. And the part I didn't know, um, which they told us, which is just fascinating.
顺便说一句,在之前的事情上,你说,他们真是天才,他们谈到了一辆车。 它应该记录哪些时刻?所以他们向我们举了一个例子,任何时候发生了,你知道,显然是一次“脱离”。 因此一次“脱离”成为他们希望在之前和之后录制视频的时刻。另一件事情是任何突然的移动。所以如果油门踩得快或者刹车踩得快,或者方向盘急转弯,这就成为一个可记录的时刻。还有一件事情是我不知道的,他们告诉我们的,那实在太有趣了。
People with LLMs have heard, you know, about reinforcement learning from human feedback, RLA, Jeff, and they've talked about how that could make it even with Gemini. They said maybe that was what caused that. Um, what, what we were told is that those moments, these moments were like the car jerks or whatever. If it is super relevant, they can put that in the model with extra weight. And so it tells the model this is this, if this circumstance arises, this is something that's more important and you have to pay extra attention to. And so if you think about this corner case, um, these corner case scenarios, which we all know are the biggest problems in self driving.
LLM的人听说过强化学习来自人类反馈,RLA,Jeff,他们讨论了这种方法如何使得与雙子座更加相符。他们说也许这就是造成这种情况的原因。我们被告知,这些瞬间就像汽车突然急转弯或者其他情况。如果这些情况非常相关,他们可以在模型中加入额外权重。这样告诉模型,在这种情况下,这是更重要的,你必须更加关注。因此,如果你考虑这种特殊情况,这些边角情况,我们都知道这是自动驾驶中最大的问题。
Um, now they have a way to only capture the things that are most likely to be those things and to learn on them. So, so the amount, the amount of data they needed to get started was this impossible amount of data with the millions of cars. And now the way that place to their advantage is they're much more likely to capture these, these, these, these let these more severe, less frequent moments because of the bigger footprint. And so you say to yourself, you know, you ask the question who, I don't know who could compete. It certainly couldn't, if, let's, let's make an assertion. If this type of neural network approach is the right answer. Yes. And I want to reason you once again, you know, Occam's razor seems that way to me, then who could compete? And one of the companies or two, you know, several of the companies would be least likely would be crews in Waymo and these things because they just don't have that many cars.
现在他们有一种方法,只捕捉最有可能是那些东西的东西,并从中学习。所以,所以他们需要开始的数据量是无法想象的,有数百万辆汽车。而现在他们能够利用的方式是,他们更有可能捕捉到这些更严重、更不经常发生的时刻,因为有更大的覆盖范围。所以你会想,你会问自己,谁能竞争呢?我不知道有谁能,如果,让我们假设一下。如果这种神经网络的方法是正确的答案。是的。我想再次向你解释,你知道,奥卡姆剃刀对我来说似乎是这样的,那么谁能竞争呢?可能竞争的公司之一或两个,你知道,其中几家公司最不可能的就是优步和Waymo,因为它们没有那么多的汽车。
And their cars cost $150,000. So if they wanted to have like the, the mattress doesn't work, you can't build the footprint. You know, and so who could, I don't know, could you, I don't know, could you put a, could you, what would it cost to build a five camera device to put on top of every Uber? I don't know, like a lot. It'd be weird. They're not going to, they're not going to do it. I mean, like, and that to me is, um, you know, when you look at these alternative models, right, if this really is about data, and remember, Bill just said an important point, which is it's not just about quantity of data.
他们的车要价15万美元。所以如果他们想要像,床垫不起作用,就无法建立足迹。你知道,那么谁能,我不知道,你能吗,我不知道,要建造一个可以放在每辆Uber车顶的五摄像头设备需要多少钱?我不知道,可能很多。这会很奇怪。他们不会这样做。我是说,对我来说,当你看这些替代模式时,如果这真的是关于数据的话,记住,比尔刚说了一个重要观点,那就是这不仅仅是数据的数量问题。
Getting magic happens around a million cars. Yes, you've got to get all that quantity of data, but to get the long tail events, right? These are events that occur tens or just hundreds of times. That's where you really need millions of cars. Otherwise you don't have a statistically relevant pool of these long tail instances. And what they're uploading, uploading from the edge, Bill, he said, each instance is a few seconds long of video and, you know, plus some additional vehicle driving metadata. And it's those events. If you only have hundreds of cars or thousands of cars, you can get a lot of data quickly.
在大约一百万辆车周围发生着神奇的事情。是的,你必须获得所有这些数据量,但是你需要获得长尾事件,对吧?这些事件发生的次数可能是几十次,甚至只有几百次。这就是你真正需要数百万辆车的地方。否则,你就没有一个统计相关的长尾实例池。比尔说,他们从边缘上传的东西,每个实例是几秒钟的视频,还有一些额外的车辆驾驶元数据。而这些事件,如果你只有数百辆车或数千辆车,你就可以快速地获得大量数据。
It's not about quantum of data. A hundred cars can produce a huge quantum of data driving a thousand miles. It's about, it's about the quality of the data, those adverse events. Yes. And, and, and I guess the other type of company that maybe could take a swing at it would be like Mobile Eye or something. The problem they have is they, they don't control the whole design of the car. And so this part where Tesla has the car in the garage at night and uploads gigabytes and puts it right into the model. Like, are they going to be able to get that done working with other OEMs? Like are they going to be able to organize all that? You know, do they have the piece on the car that says, when to record and when not to record and, and, and like it is just a massive infrastructure question, I would probably, if I had to handicap anybody, it would probably be, BYD or one of the Chinese manufacturers. Right. And if you think about there, they have a lot of miles driven in China, right? Much less so outside of China. I imagine you're going to have some of this nationalistic stuff that, you know, that emerges on, on both ends of this. Like one of the things I asked our analysts, Bill, is like, if we just step back, I think these guys have network advantage. They have data advantage. They're clearly in the lead. They have bigger H 100 clusters than the people they're competing against. I mean, they have all sorts of things that have come together here. But if you think about like, what's the so what to Tesla, right? And just in the first instance, and we'll pull up this slide that Frieda on our teammate, if you look at the unit economics of a Tesla, right? With no FSD, they're making about two and a half thousand bucks on a vehicle. If you look at it today, they have about 7% penetration of FSD. That was, let's call it through FSD 11.
这不是数据量的问题。一百辆车可能会产生大量数据,行驶一千英里。重点在于数据质量,那些不利事件。是的。我想另一种可能会尝试的公司可能是Mobile Eye之类的公司。他们面临的问题是,他们无法控制整辆车的设计。所以特斯拉晚上把车放在车库里上传几个G的数据并直接输入模型的这一部分,他们能和其他原始设备制造商合作完成吗?他们能够组织好所有这些吗?你知道,他们是否有车上的部件来决定何时记录何时不记录,这就是一个庞大的基础设施问题。如果我要给任何人下赌注,可能会是比亚迪或是一家中国制造商之一。对。如果你考虑一下,他们在中国行驶的里程数很多,是吧?在中国以外的地方远不及。我想你可能会看到一些民族主义的事情,以及涉及这两头的事。我问我们的分析师之一比尔的问题是,如果我们只是退后一步,我认为这些人有网络优势。他们拥有数据优势。他们显然领先。他们拥有比其他竞争对手更大的H100集群。我意味着所有这些因素在这里汇聚在一起。但是如果你考虑一下,对特斯拉来说有什么影响呢?首先,让我们看一下我们团队成员弗里达做的幻灯片, 如果你看一下特斯拉的单车经济学,在没有全自动驾驶的情况下,他们每辆车大约赚两千五百美元。如果你看今天的情况,他们大约有7%的全自动驾驶渗透率。这是,让我们称之为全自动驾驶11。
And those people paid $12,000 incrementally for that FSD. And as we know, you can go read about it on Twitter. People are like, yeah, it's good, but it's not as good as I thought it would be. So now we have this big moment of a step, what feels like, you know, kind of a step function, a model getting better at a much faster rate. So I asked the question, what if we reduce the price on this by half? Right? What if what if Tesla said this is such a good product? We think we want to drive penetration. So let's make it 500 bucks a month, not a thousand bucks a month. So if you assume that you have, you know, penetration, you know, go from 7% to 20%, give it to everybody for free. They drive around for a month. They're like, wow, this really does feel like a human driver. I'm happy to pay 500 bucks a month. You know, if you get to, you know, 20% penetration, then your contribution margin at Tesla, right, is about the same even though you're charging half as much. Now if you get to 50% penetration, all of a sudden you're creating billions of dollars in incremental e-bata. Now think about this from a Tesla perspective. Why do they want to drive even more adoption of FSD? Well, you get a lot more information and data about disengagements and all these other things. So that data then, you know, continues to turn the flywheel. So my guess is that Tesla seeing this meaningful improvement is going to focus on penetration. My guess is that they want to get a lot more people trying the product and they're going to play around with price. Why not? Right? Well, I think that all of these things are occurring at an accelerating rate at Tesla.
这些人分期付款支付了12000美元用于购买全自动驾驶功能。正如我们所知,你可以在Twitter上了解更多信息。一些人表示,这个功能确实不错,但并不像他们想象的那么好。现在我们迎来了一个重要时刻,感觉就像是一个飞跃式的进步,模型以更快的速度变得更加优化。因此,我问了一个问题,如果我们将价格减半会怎样呢?如果特斯拉表示这是一个很好的产品,我们希望提高市场渗透率。所以我们将月费从1000美元降低到500美元。假设你有渗透率,从7%提高到20%,免费提供给每个人使用一个月。他们会发现,哇,这确实感觉像一个人类司机。我愿意每月支付500美元。如果你达到20%的渗透率,那么特斯拉的贡献率就会大致相同,即使收费减半。如果渗透率达到50%,突然之间你就创造了数十亿美元的增量ebata。现在从特斯拉的角度来考虑这一点。他们为什么要进一步推广全自动驾驶功能呢?因为他们能够获得更多关于故障排除和其他信息的数据。然后这些数据会继续推动发展。我猜想,特斯拉会看到这种重要改进,并将专注于市场渗透率。我猜想他们想让更多的人尝试这个产品,并且他们将尝试调整价格。为什么不呢?我认为,所有这些事情在特斯拉公司都在加速发展。
And when I look around, you know, I still hear people saying Waymo's worth 50 or 60 billion bucks, but you could be in a situation on that business where it just is, you know, gets passed really quickly and they have a hard time structurally of catching up. Well, and we, you know, people have said that, and if someone has data, once again, they want to crack this, I'd be glad to state to recorrect the data. But, you know, we've been told they have a headcount similar to cruise and the cruise financials came out and they were horrific. And so I don't have any reason to believe that the Waymo financials are any different than the cruise ones. And I've always thought this model that we're going to build this incredible car. And our business model is going to be to run a service like the CapEx, like if you just build a 10 year model, the CapEx you need, like they would have to go raise a hundred billion. And there's another element that's super interesting that the team at Tesla feels very strongly that LiDAR does not need to be a component of this thing.
当我环顾四周的时候,你知道,我仍然听到人们说Waymo值500亿或600亿美元,但你可能会发现在这个行业中处于一种情况,事情会很快超越他们,并且在结构上跟不上。嗯,我们知道,人们已经这样说过了,如果有人有数据,再一次,他们想要突破这个问题,我很乐意澄清数据。
但是,我们被告知他们的员工规模与cruise相似,而cruise的财务状况已经公布,非常糟糕。因此,我没有任何理由认为Waymo的财务状况会有任何不同于cruise的。我一直认为我们要打造这种令人难以置信的汽车模型。我们的商业模式将是像资本支出一样运行服务,如果只是建立一个10年的模型,所需的资本支出,他们可能需要筹集1,000亿美元。而且还有一个非常有趣的方面,特斯拉团队非常强烈地认为激光雷达不需要成为这个系统的一个组成部分。
And so the Waymo cruise, all those approaches and mobile I are LiDAR dependent, which is a very costly piece of material in those designs. And so if this is all true, if this is how it plays out, it's a pretty radical new discovery. So one of the things I also want to talk about because one of the reasons I started going down this path is our team's been spending a lot of time with the robotics companies, new robotics companies. We have Optimus at Tesla, figure AI just raised some money from OpenAI and Microsoft. And we met with those guys and they're all doing really interesting things.
所以Waymo的自动驾驶技术,所有这些方法和移动设备都依赖LiDAR,这在这些设计中是非常昂贵的材料。如果这一切都是真的,如果事情真的是如此发展,那将是一个非常激进的新发现。因此,我还想谈谈的另一件事是,我开始走上这条道路的原因之一是我们团队花了很多时间与新的机器人公司一起工作。我们在特斯拉有Optimus,而Figure AI刚刚从OpenAI和微软募集了一些资金。我们会见了这些人,他们都在做非常有趣的事情。
But again, they're shifting their models. The robotics companies also were using these deterministic models to teach the robot maybe how to pour a cup of coffee or something. And now they're moving to these imitation models. So I was searching around the other day and I came across this video by a PhD student at Stanford, Ching Chae. And he showed how this robotic arm was basically just collecting data very quickly using a little camera on a handheld device. And then they literally take the SD card out of the camera, they plug it into the computer, it uploads this data to the computer, it refreshes the model and just based on two minutes of training data.
但是,他们又在改变他们的模型。机器人公司也在使用这些确定性模型来教导机器人如何倒一杯咖啡或者类似的事情。现在他们正在转向这些模仿模型。所以,前几天我在做搜索的时候发现了一段由斯坦福大学的博士生钱彻制作的视频。他展示了这个机械臂是如何通过手持设备上的小摄像头快速收集数据的。然后他们简单地将SD卡从相机中取出,插入电脑,将这些数据上传到电脑上,更新模型,仅仅基于两分钟的训练数据。
Now video in control out this robotic arm knows how to manipulate this coffee cup in all of these different situations. So I think we're going to see the application of these models, end to end learning models, imitation learning models, impact not just cars. I mean, 5 million cars on the road, that's probably the best robot we could possibly imagine for data collection. The challenge of course in robotics is going to be data collection. But then I saw this video and I said, well, maybe that's a manageable challenge, particularly for a discreet set of events. Yeah.
现在视频中控制着这个机械臂,它知道如何在各种不同的情况下操作这个咖啡杯。因此,我认为我们将看到这些模型的应用,端到端学习模型,模仿学习模型不仅仅影响汽车。我是说,道路上有500万辆汽车,这可能是我们能够想象到的最好的用于数据收集的机器人。当然,机器人领域的挑战将是数据收集。但是当我看到这个视频时,我说,也许这是一个可以管理的挑战,尤其是对于一个离散事件集。是的。
And the other great thing about that video of people take the time to watch it, it actually explains pretty simply how the Tesla stuff's working, right? I mean, it's just a different scale obviously, but that's the exact same thing, just at a very reduced state. Right. And you can imagine when that's just this autonomous flywheel without a lot of human intervention, and that's the direction that Tesla still has some engineering intervention along the way. But I think the engineering team working on this at Tesla is about one-tenth the size of the teams at Crucet.
这个视频的另一个很棒的地方是,如果人们花时间观看,它实际上相当简单地解释了特斯拉的工作原理,对吧?我是说,显然是不同的规模,但本质上是一样的,只是在一个非常简化的状态下。是的,你可以想象当它变成这种完全无需人为干预的自主飞轮时,特斯拉仍然在一定程度上进行了工程干预,但我认为特斯拉工程团队在这方面的人数大约是Crucet团队的十分之一。
Well, I mean, that gets back to this simplicity point, right? Like this approach removes so much complexity that you should be able to do it with less people. And the fact that you can have something better with less people is really powerful. So we talked a little bit about how models, these open source models are driving a lot of the improvements at Tesla. We seem to get model improvements and model updates every day, Bill. Maybe I just go through a few of the recent ones. And I want to explore this open versus close. Last week, we heard about Gemini 1.5. It has a huge expanded context window. And Gemini 1.5, about a chat GPT-4 level, then yesterday we get Cloud 3 announcements.
嗯,我的意思是,这涉及到简单性这一点,对吧?就像这种方法消除了很多复杂性,所以你应该能够用更少的人完成。而且能够用更少的人获得更好的效果真的很强大。所以我们谈到了一些模型,这些开源模型正在推动特斯拉的许多改进。我们似乎每天都会得到模型的改进和更新,比尔。也许我可以简单介绍一下最近一些情况。我想探讨一下开放与封闭的区别。上周,我们听说了有关 Gemini 1.5 的消息。它有一个非常大的扩展语境窗口。而 Gemini 1.5,达到了一个与 GPT-4 等级的聊天水平,而昨天我们又获得了 Cloud 3 的公告。
Their best model opus is just a little bit better than chat GPT-4. But I think the significant thing there, and we have a slide on this, is just really about the cost breakthrough that their sonnet level model can do workloads at a fraction of the price of chat GPT-4, even though it's performing at or near that quality. And then we have, you know, those models were trained on a mixture, I think, of H100 and prior version of NVIDIA chips. The first H100 only trained models, I think, will be LAMA3 and chat GPT-5. So we're hearing rumors that both of those models are going to come out in the May, July timeframe.
他们最好的模型作品只是比聊天GPT-4略好一点。但我认为其中一个重要的事情,我们在这里有一张幻灯片,就是关于他们的十四行诗级别模型能够以零头的价格完成工作负载的突破。尽管它的性能接近或达到GPT-4的质量水平。然后,我们知道,这些模型是在H100和NVIDIA芯片的先前版本的混合上进行训练的。我认为,第一个仅由H100训练的模型将是LAMA3和聊天GPT-5。我们听说这两个模型都将在五月至七月的时间范围内推出。
With respect to LAMA3 that was trained on Meta's H100 cluster, rumors are that it has Cloud 3-like performance, which is pretty extraordinary if you're thinking about a fully open-sourced model. And then chat GPT-5, which we hear is done. And they're simply in kind of their post-training safety, guardrails, their normal post-training work. And here that's going to launch sometime in May versus June. And because that one was trained on H100s, we hear it is like a 2X improvement versus chat GPT-4.
关于在Meta的H100集群上训练的LAMA3,有传言说它具有类似于Cloud 3的表现,如果考虑到这是一个完全开源的模型,这是相当了不起的。而此刻我们听说聊天工具GPT-5已经完成了。他们现在在进行后训练的安全措施、限制,以及正常的后训练工作。据说该模型将在五月份发布,而不是六月份。因为它是在H100上训练的,我们听说它比聊天工具GPT-4有了2倍的改进。
But then we hear all the rest of the frontier models are kind of in this holding pattern because they're waiting for the B100s to get launched to this Q3, Q4 out of NVIDIA, which probably means the next iteration of the frontier models will come out in Q2 of next year, Q2 of 25. That's after chat GPT-5. So Bill, if you go through this bedrock page on AWS, if you just scroll through, you see the Amazon is offering all these different models. I mean, you can run your workloads on LAMA, on Mistral, on Claude, et cetera.
但是我们听说,其余的前卫模型都处于停滞状态,因为它们在等待B100s在NVIDIA的Q3、Q4推出,这可能意味着下一代的前卫模型将在明年的Q2发布,即25年的Q2。那将是chat GPT-5之后。所以比尔,如果你在AWS的这个基石页面上浏览一下,你会发现亚马逊提供了所有这些不同的模型。我是说,你可以在LAMA、Mistral、Claude等上运行你的工作负载。
Snowflake today just announced a deal with Mistral, and they're going to have LAMA as well. I imagine Databricks will. Microsoft, you can use LAMA or you can use Mistral or OpenAI. So where do you think all of this goes in terms of the models that will actually get used by enterprises and consumers in practice? Yeah. So I have a lot of different thoughts. My first one, when this new anthropic thing came out and they list all the different math tests and science tests and PhD, and they're all listed in the same thing, I wonder if they're racing up a hill, but they're all racing up the same hill. Yeah, there's the thing.
今天Snowflake刚刚宣布与Mistral达成了一项协议,他们也将拥有LAMA。我想Databricks也会加入。微软,你可以使用LAMA,也可以使用Mistral或OpenAI。那么在实践中,你认为所有这些会如何影响企业和消费者实际使用的模型呢?是的。我有很多不同的想法。当这个新的人类学东西出来时,他们列出了所有不同的数学测试、科学测试和博士学位,它们都在同一个名单上,我想知道他们是否在赛跑,但他们都在同一个山上赛跑。是的,这是个问题。
Because they're all running the same comparative test and they're all releasing this data. And I would, I don't know if any of them are creating the type of differentiation that's going to lead to one of them becoming the wholesale winner versus the other, right? And is this type of micro optimization, you know, in a way that's going to matter to people or to the users? And it's not clear to me. I mean, I see some developers get way more excited about the pricing at the low end of those three choices than they do about the performance of the top end. So that's one thing.
因为它们都在进行同一种比较测试并发布这些数据。我不确定它们中是否有哪一个正在创造能够导致其中一个成为绝对赢家的差异化。这种微小的优化,是否会对人们或用户有所影响呢?对我来说并不清楚。我发现一些开发者对于这三个选择中价位较低的定价比对顶级性能更为兴奋。这是一点。
The second thing on my mind, I don't, I don't have a lot of logic to put around this. It's more of an intuition. I wonder if these companies can simultaneously try and compete with Google to be this consumer app that you're going to rely on to get you information. So you could call that Wikipedia on steroids, you know, Google search redefined whatever market you want to call that and simultaneously be great at enterprise models. And, and I just don't know if they can do both.
我的第二个想法是,我没有很多逻辑可以解释这点。更多的是直觉。我在想这些公司是否可以同时尝试与谷歌竞争,成为你依赖获取信息的消费者应用程序。你可以说这是“强化版的维基百科”,你知道,重新定义了谷歌搜索,无论你想称之为什么市场,同时也在企业模式方面表现出色。但我不确定它们是否能同时做到这两点。
I really don't. And maybe that'll get to the third thing, which is more the essence of your question. Like, what am I hearing about and seeing about when it comes to companies that are actually utilizing these things? The Tesla example was interesting because, you know, they start with these bedrock components that are open source. And one thing that happened in the past 20 years, it happened very slowly, but we definitely got there.
我真的不清楚。也许这会导致第三个问题,更多地涉及你的问题的本质。比如,当涉及到那些实际利用这些东西的公司时,我听到和看到了什么?特斯拉的例子很有意思,因为他们从这些开源的基石组件开始。在过去的20年里发生了一件事,尽管进展缓慢,但我们终于做到了。
CIOs at large companies, they used to be an IBM shop or an Oracle shop or a Microsoft shop. That was their platform. They slowly got to the place where most of the best say CIOs were open source first. So for any new project, they start, you know, they used to be skeptical of open source and it slipped completely the other way. Like, oh, is there an open source choice we can use? And the reason is they don't wonder there's more competition and two, they don't want to get stuck on anything.
大公司的首席信息官,过去他们可能是IBM、Oracle或Microsoft的忠实用户。那些是他们的平台。他们慢慢地走到大多数顶尖信息官都是开源优先的地步。因此,对于任何新项目,他们开始时对开源持怀疑态度,但现在完全转变了。就像:"哦,有没有开源选择我们可以使用的?" 原因是他们不想受限于更多竞争,更不想被困在某些东西上。
And so when I look at what I see going on in the startup world, they might start with one of these, you know, really well known service models that's proprietary. But the minute they start thinking about production, they become very cost focused and on the inference side and they'll just play these things off of one another and they'll run a whole bunch of different ones. I saw one startup that moved between four different platforms. And I just think that that competition is very different than the competition to compete with Google and this consumer thing.
因此,当我看到创业世界中正在发生的事情时,他们可能会从这些中的一个开始,你知道,非常知名的专有服务模型。但一旦他们开始考虑生产,他们就会非常注重成本和推演方面,然后他们会将这些事物相互对比,并运行大量不同的平台。我看到一个创业公司在四个不同的平台之间移动。我认为这种竞争与与谷歌和消费者行业竞争的竞争非常不同。
And I'll give you another example. Like I was talking to somebody, if you had a legal application you wanted to use, you'd be better off with a smaller model that had been trained on a bunch of legal data. It wouldn't need some of the training of this overall LMM. And it might be way cheaper to have something that's very proprietary or not proprietary but very focused from a vertical standpoint. You could imagine that in a whole bunch of different verticals.
我来举个例子。就像我在和某人交谈时讨论的,如果你想使用一个法律应用程序,最好选择一个经过训练的小型模型,它拥有大量的法律数据。它不需要像整体大型模型那样进行训练。并且在垂直立场上,拥有一个非常专有或非专有但非常专注的东西可能会更便宜。你可以在许多不同的垂直领域想象到这种情况。
So it just strikes me that this on the B2B side, this stuff's getting cut up and into a bunch of different pieces where a bunch of different parties could be more competitive and where those components are most likely to be open source first. Yes, yes. I mean, you're causing me to think a couple of different things. One, I've said in the past, if I was Sam Altman running OpenAI, I think I might rename the company Chat GPT and just focused on the multi-trillion dollar opportunity to replace Google. Right? Winning at both beating Google at consumer and beating Microsoft at enterprise.
因此,我觉得对于B2B行业来说,这些东西正在被拆分成许多不同的部分,许多不同的参与方可能会更具竞争力,这些组件最有可能首先是开源的。是的,是的。我的意思是,你让我想到了一些不同的事情。首先,我之前说过,如果我是领导OpenAI的Sam Altman,我觉得我可能会将公司改名为Chat GPT,专注于取代谷歌这个价值数万亿美元的机会。对吧?在击败谷歌的同时,同时赢得在消费者领域击败微软的机会。
Andy wants to beat NVIDIA at building chips. Those are three big battle fronts. And if I think about the road to AGI, building memory, building all this thing that's going to differentiate you in the consumer competition, that just seems best aligned with who they are, what they're doing. I mean, Chat GPT has become the verb in the age of AI. They replace Google at the start. Nobody's saying we're barding something. They're saying we're Chat GPT and something. So I think that they have a leg up there.
安迪想要在芯片制造方面击败英伟达。这是三个重大战场。而且,如果我考虑通往AGI的道路,制造记忆,制造所有这些将在消费者竞争中使你与众不同的东西,似乎最符合他们是谁,他们在做什么。我是说,在人工智能时代,Chat GPT已经成为动词。他们取代了谷歌的地位。没有人说我们正在用某种东西轰炸。他们说我们正在用Chat GPT和某种东西。所以我认为他们在那方面有优势。
When I look at the competition in enterprise, right, I think anthropic was up at the Morgan Stanley Conference this morning and they said they're hiring their sales force, went from two people last year to 25 this year. Think of the tens of thousands of salespeople at Microsoft, at Amazon, et cetera, that you got to go compete with. Now, of course, they're also partnering with Amazon. But when you think about that, these guys, there's going to be all this margin stacking bill. So Amazon's got to get paid, anthropics got to get paid, NVIDIA's got to get paid.
当我看着企业竞争的情况时,我想到今天早上Anthropic参加了摩根斯坦利会议,并表示他们正在扩大销售团队,从去年的两人增加到今年的25人。想想微软、亚马逊等公司的数以万计的销售人员,你必须要面对竞争。当然,他们也正在与亚马逊合作。但当你考虑到这一点时,这些公司之间将会有很多的收入分配。亚马逊需要付费,Anthropic需要付费,NVIDIA也需要付费。
Now if you use an open source model, you can pull one of those pieces of the margin stacking out, right? So now this is just Microsoft getting paid using llama, llama three or llama two. They don't have to pay for the use of that model and NVIDIA gets paid. So I think in the competitive dynamics of an open marketplace, right, that that enterprise game is going to be tough for two different reasons for these model businesses. Number one, Zuckerberg is going to drive the price, right? He's going to give away frontier esque models on the cheap. Okay.
现在,如果您使用开源模型,您可以将边际堆叠的其中一个部分拿掉,对吧?所以现在这只是微软使用lama、lama three或lama two而得到报酬。他们不必为使用该模型而付费,而NVIDIA会得到报酬。所以我认为在一个开放市场的竞争动态中,这个企业游戏对这些模型企业来说会很困难,主要有两个原因。第一,扎克伯格会推动价格,对吧?他会以便宜的价格提供前沿模型。
And that's going to be highly disruptive to your ability to stack margin. If I'm a CIO of JP Morgan or some other, you know, large institution, do I really want to pay a lot for that model? I'd rather have the benefit of open, right? Because then I can, you know, move my data around a little bit more fluidly. I get the benefit, the safety benefits of an open source model. And I'm not sending my data to open AI. I'm not sending my data to some of these places. Huge point you just made that is in addition to everything we said, which is a lot of the big companies have concerns about their data being commingled or uploaded even at all.
这将严重影响你堆叠利润的能力。如果我是摩根大通或其他大型机构的首席信息官,我真的想花很多钱来购买这种模式吗?我宁愿选择开放的好处,因为这样我可以更流畅地移动我的数据。我得到了开源模式的安全益处。我不会把数据发送给开放AI。我也不会把数据发送到某些地方。你刚刚提到的一个巨大的观点是,除了我们所说的一切,许多大公司担心他们的数据会被混合使用或上传。
Into these proprietary models. So it's not, it's, it's not just, I think the challenge for them in enterprise is not just how do I build an enterprise fleet to go compete with the largest hyperscaler in the world who are great enterprise businesses. And you've got to compete with Databricks and Snowflake, et cetera. But I think the second thing is just, you know, there is this, this bias, this tendency that you say has evolved over a couple of decades of open versus closed, which then, you know, brings me a little bit to this, you know, the weight, but wait, one more, one more element that I think that's important too for everyone to understand.
这些专有模型。因此,对企业来说,挑战不仅在于如何建立企业舰队以与世界上最大的超大规模云服务提供商竞争,他们是出色的企业业务。你必须与Databricks和Snowflake等竞争。但我认为第二个挑战是,你知道,这种偏见,这种倾向在几十年来逐渐演变的开放与封闭之间的对立,这让我想到,你知道,还有一个重要的元素,我认为每个人都应该理解。
One of the reasons open source is so powerful is because it, because it can be replicated for free, you end up with just so much more experimentation. So it turns out right now there are multiple startups who believe they have an opportunity hosting open source models. So they're propping up llama three or mrall as a server provider competing with Amazon. But they're going to tune in a little different way. They're going to play with it a different way. So you're the number of places you can go by one of these open source models delivered as a service is you have multiple choices. It's been proliferate and that creates optionality.
开源如此强大的原因之一是因为它可以免费复制,这样就可以进行更多的实验。现在有多家初创公司相信他们有机会托管开源模型。他们正在支持llama three或mrall作为与亚马逊竞争的服务器提供商。但他们会以一种略有不同的方式进行调整。他们会以一种不同的方式进行操作。因此,通过作为服务提供的这些开源模型,您可以选择前往的地方数量是多种多样的。这种扩散使得选择更加丰富。
There's just so much more experimentation that's going to happen on top of the data privacy problem, the pricing stuff you talked about. So there's a lot of different elements that make me think that that the open source component models are going to be way more successful in the enterprise. And it's a really tough thing to compete with now. Yeah, well, it kind of brings into stark relief a big, a big debate that erupted this week, you know, certainly on the Twitter's with Elon's lawsuit, you know, that he filed.
在数据隐私问题和定价方面,还有更多实验将会展开。这使我觉得,企业中开源组件模型将更加成功。现在,这是一个很难与之竞争的领域。是的,这很明显引发了一场大辩论,尤其是在Twitter上,就埃隆提起的诉讼。
And you know, part of that was about this not for profit to for profit conversion. You know, that's to me a little bit less interesting. Don't want to talk a lot about that. But it blew the doors wide open on this open versus closed debate, right? And the potential, you know, that exists here for regulatory capture. Nobody's more thoughtful about this topic than you. I think, you know, I saw somebody tweet this, this two by two matrix, you know, it says dividing every conversation, you know, up between Mark and the note and, you know, and Elon and Sam. But, you know, we saw a lot of, you know, a very sharp opinions expressed. So help us think about like the regulatory cap, the risk of regulatory capture and why this moment is so important. Yeah.
你知道,其中一部分涉及非营利组织向营利组织的转变。对我来说,这有点不那么有趣。不想多谈这个。但这使得开放与封闭的辩论完全开放了,对吗?在这里存在监管捕获的潜力。没有人比你更深思熟虑这个话题。我觉得,我看到有人在推特上发表了这个二乘二矩阵,将每次对话分成马克和诺特,以及埃隆和山姆。但我们看到很多非常明确的观点表达出来。所以帮助我们思考监管捕获的风险,以及为什么这一时刻如此重要。是的。
You know, I happened to mention this when I did my regulatory capture speech at the all-in conference. I mentioned very briefly when I showed a picture at Sam Altman that I was worried that they were attempting to use fear mongering about doomerism and AI to to build regulation that would be, you know, particularly beneficial to the proprietary models. And then after that, there were, you know, rumors that, you know, people at some of the big model companies were going around saying we should, we should kill open source or we should make it illegal or we should get the government to block it.
你知道,我在全明会议上进行监管俘获演讲时碰巧提到了这一点。我在向Sam Altman展示一张照片时简要提到,我担心他们试图利用末日主义和人工智能来制定法规,这将特别有利于拥有专利模型。在那之后,就有传言说,一些大型模型公司的人传言说我们应该打击开源项目,或者应该让它非法化,或者应该让政府来封锁它。
And then Venote started basically saying that literally like, yes, we should block open source. And that became very concerning to me. I think it obviously became concerning to Mark and Driesen as well. And for me, the biggest reason that it's concerning is because I think it could become a precedent where all companies would try and eliminate open source. And there's a good reason why. I mean, we just talked about it's a hell of a fucking competitor. Like I wouldn't want to go up against it. But but but it's also really amazing for the world. It's great for startups. It's amazing for innovation. It's great for worldwide prosperity. Think about Tesla. We just talked about all this open source that they're using.
然后Venote基本上开始说,是的,我们应该封锁开源。这让我感到非常担忧。我认为这显然也让Mark和Driesen感到担忧。对我来说,最大的原因是因为我觉得这可能会成为一个先例,所有公司都会试图消灭开源。这里有一个很好的原因。我是说,我们刚刚谈到它是一个非常激烈的竞争对手。我不想和它对抗。但是但是但是,它也对世界有着巨大的影响。对于初创公司来说是非常好的。对于创新来说是令人惊奇的。对于世界繁荣也很有益。想想特斯拉。我们刚刚谈到他们使用的所有这些开源。
Yeah. It's the last thing I would want to see happen. But but you know, we do live in this world where where these pieces exist. And I would urge people to read, we'll put a link in a political article that shows the amount of lobbying that has been done on behalf of the large for proprietary models. And I don't think you'll find literally the only thing that comes close perhaps and people will think I'm being outlandish. But as SBF who was also lobbying at this kind of level, but this political article shows they have three or four different super PACs. They're putting people they're literally inserting people onto the staffs of the different congressmen and senators to try and influence the outcome here.
是的。这是我最不想看到的事情。但你知道,我们生活在这样一个存在这些利益集团的世界。我想敦促人们去阅读一篇政治文章,里面显示了为了大型专有模式进行的游说工作的数量。我认为你会发现几乎唯一接近的可能是SBF也在这种级别进行游说,但这篇政治文章显示他们有三四个不同的超级政治行动委员会。他们派遣人员直接进入不同国会议员和参议员办公室,试图影响这里的结果。
I think we may be escaped this. Like I think the open source models are so prolific right now that maybe we've gotten past it. And I also think their competitiveness has shown that there's a reason why they would you know, want to stop them. I mean, I think at the time they started, maybe that wasn't clear, but I think it's remarkably clear right now. I also don't believe in the doomerism scenario. Someone who I admire quite quite a bit. Steve Pinker posted a link to this article by Michael Totten where he goes through, I think in a very sophisticated way, the different arguments that I would urge people maybe to read that on their own.
我认为我们可能已经摆脱了这种情况。就像我认为开放源代码模型现在如此盛行,也许我们已经超越了它。而且我也认为它们的竞争性表明他们有理由想要阻止它们。我的意思是,也许在它们开始时这并不清楚,但我认为现在非常清楚了。我也不相信末世论的情景。我非常钦佩的一位人物,史蒂夫·平克(Steve Pinker)发布了一篇迈克尔·托滕(Michael Totten)的文章链接,其中他以非常复杂的方式探讨了不同的论点,我建议人们自己阅读一下。
But yeah, I don't, I don't, for me, if you want to spread the doomerism, let's get people to tell that story that aren't running billion dollar companies that are taking hundreds of millions out and giving it to their employees. I mean, there's a level of bias that's obvious here. And so I'd rather listen to a doomerism argument from someone who's not standing to gain from regulation. Yeah, I mean, I think you saw this tweet from Martin Casado, you know, that was in response to, you know, Vinod comparing open source, you know, would you use open source for the Manhattan Project, you know, which really kind of opened up, you know, this box even more.
但是是的,我不觉得,对我来说,如果你想传播悲观主义,让我们让那些不是在经营数十亿美元公司并从中获利数亿美元并将其分配给员工的人来讲述这个故事。我是说,这里有明显的偏见。所以我宁愿听一个不会因为监管而获益的人讲述悲观主义论点。是的,我想你看到了Martin Casado的推文,他对Vinod比较开源项目做出了回应,你会在曼哈顿计划中采用开源项目吗,这确实更加开启了这个话匣子。
What's your, you know, weigh in a little bit here, just if you're in Washington and you're hearing these things like, you know, we can't allow these types of models to be used on, you know, on things like this, we saw India is now requiring approval, you know, to release models that also, you know, was I think a scary development for people in the open source community. But you know, again, just reinforce like, why should we not be worried about open source AI, AI models? How do they send us to a better place?
在华盛顿听到这些讨论时,你有什么看法呢?比如,我们不能允许这些模型在某些情况下被使用,印度现在要求批准发布模型,这对开源社区的人们来说可能是令人担忧的发展。但是,我们为什么不应该担心开源AI模型呢?它们如何帮助我们走向更好的地方呢?
In the, in the, in the, in the Totten article of Pinker uses an analogy that I just love, which he says, like, you could spread a doomerism, you know, argument that a self-driving car would just go 200 miles an hour and run over everybody. But he says, if you look at the evolution of self-driving cars, they're getting safer and safer and safer. It's, it's not, we don't program the AI to give them this singular purpose that, that overrides all the other things they've been taught and then they go crazy. Like, that's not what's happening. That's not how the technology works. That's not how we use the technology.
在《Pinkoer的Totten文章》中,Pinkoer使用了一个我非常喜欢的类比,他说,就像你可以传播一种悲观情绪的论点,即自动驾驶汽车会以每小时200英里的速度撞倒所有人。但他说,如果你看看自动驾驶汽车的发展,它们变得越来越安全。我们并没有为AI设定这种唯一的目的,超越了它们学到的其他所有东西,然后它们变得疯狂。这并不是发生的事情。这也不是技术如何运作的方式。我们也不是这样使用技术的。
And so I, I think the whole article is great, but I think, you know, and look, I also think Pinker is a really smart human. Like he's also one of the biggest outspoken proponents of nuclear, which is another topic that I think has been wildly, you know, misconstrued. And so anyway, I'm, I'm more of an optimist about technology. These kind of doomerism things go way back to the luddites. Hence, hence the definition of the word, right? And ever since then. And someone else tweeted like, you know, be like telling the farmer, you know, look out for the tractor. Like it's going to ruin, you know, it's just not how our world evolves.
所以我认为整篇文章很棒,但是我认为,你知道的,而且我也觉得平克是一个非常聪明的人。他也是核能的最大公开支持者之一,这是另一个我认为被误解的话题。总之,我对技术抱有乐观态度。这种悲观主义的事情追溯到卢德派。因此,这就是这个词的定义,对吧?自那时以来一直是如此。还有人发推文说,你知道,就像告诉农民注意拖拉机一样。它会毁掉,你知道,这不是我们世界发展的方式。
Well, the reason I think this is so important is because, you know, the competition that's going to come from these models, all the evidence suggests that it moves us to a better place, but not worst place. However, during these moments, right, where, you know, you do have a new thing and it does sound scary. And then you have all these people coming to Washington saying, Hey, we can't allow all this experimentation. We can't allow these open source models. What I worry about is that that can actually win the day like it has in India.
我认为这很重要的原因是,你知道,来自这些模型的竞争将会发生,所有的证据表明,这将让我们走向一个更好的地方,而不是更糟的地方。然而,在这些时刻,你知道,当你面临一个新事物,它听起来确实很可怕。然后你看到所有这些人来到华盛顿说,嘿,我们不能允许所有这些实验。我们不能允许这些开放源模型。我担心的是,这种想法实际上可能会获胜,就像在印度一样。
But you know, I was in Washington last week talking to leadership in both the House and the Senate about, you know, a program near and dear to me called Invest America. But the conversation about AI came up with many senators and many senior leadership folks in the House. And one of them said to me when he was asking about AI, I, you know, I said, I was worried about, you know, excessive government oversight, getting persuaded, particularly as it relates to open source models. And he said, don't worry. He said, you know, we had Sam Altman out here and we know what he's up to. And I thought that was, you know, and he ended by saying, we need competition. Like the way we stay ahead of China is we need competition. So that was highly encouraging to me, you know, from a senior member.
但是你知道,上周我在华盛顿与众议院和参议院的领导人讨论了一个我非常关心的项目,叫做“投资美国”。在与参议员和众议院的许多高级领导人的对话中,涉及到了人工智能。其中一位在问我关于人工智能时,我表示担心政府监管过度,特别是涉及开放源模型。他说:不用担心。他说,我们曾经邀请过Sam Altman,并且知道他在做什么。他最后说,我们需要竞争。保持领先对抗中国的方式就是我们需要竞争。这对我来说是非常鼓舞人心的,来自一名高级成员。
It's interesting. That's so great to hear. And I think, you know, this China thing comes up all the time. Like the one thing that would cause us to get way behind China is if we played without open source and they had like, and then the other thing I would just say is, you know, many academics I talk to are like, I have way more trust in open source where I can get in and see and analyze what's going on. And you know, the other side of this, because we talked about LLMs or, you know, AI competing about them to be to be side to be to see side on the consumer side.
这很有趣。听到这样的话真是太棒了。我认为,你知道的,中国这个问题总是经常出现。如果我们没有开源,他们就会拉开我们很大的差距。我想说的另一件事是,你知道,我和许多学者交流时发现他们更信任开源,因为我可以进入并分析其中发生的事情。我们还谈到了LLM或者AI之间的竞争,他们正在追求消费者的共同利益。
You know, the Gemini release from Google, I think is proof of the type of, you know, the Google Gemini model was much more similar to something autocratic that you might equate with a communist society. Like it's intentionally limiting the information you can have and painting it in a very specific way. And so yeah, I'm more afraid of the price. Yeah, they're effectively imposing a worldview by massaging the kernel here in ways that we don't understand. It's a black box influencing our opinions.
你知道,我觉得谷歌的双子座发布是一种证明,你知道,谷歌双子座模式更类似于你可能与共产主义社会相提并论的一种专制主义。就像它有意地限制你可以获得的信息,并以一种非常特定的方式呈现出来。所以是的,我更担心这个价格。是的,他们通过以我们不理解的方式来设置内核,有效地施加了一种世界观。这是一个影响我们观点的黑匣子。
And you know, I just find it ironic in this moment in time that, you know, the person putting the most dollars up against the open source is somebody we're critical of, you know, Washington was pretty critical of a couple of years ago, which is Zuckerberg. And you know, the fact of the matter is you need to have a million H 100s. He's going to have, you know, hundreds of thousands of B 100s. You need somebody who has a business model that can fund this level of frontier magic on these open source models. And the good news, it appears we have it.
你知道,我觉得在这个特定时刻挺讽刺的,你知道,那个在开源领域投入最多资金的人正是我们批评的对象,你知道,几年前我们还对华盛顿进行了相当批评,那就是扎克伯格。事实是你需要有数以百万计的H 100s。他将拥有成千上万的B 100s。你需要有一个商业模式可以资助这种开源模式的前沿魔法。好消息是,看起来我们已经做到了。
Yeah. That's awesome. I'm thrilled you heard that. You know, the, there was another interesting case over the course of the last couple of weeks that I know you and I, actually one last thing on this, because I just recalled a conversation I was having with the Senator. Like, let's assume, let's assume that you, you, you, you, do Marism's right. And you have to be worried about this. What are the odds? What are the odds that our government could put together a piece of effective legislation that would actually solve the problem? Right. Right. It's low. Well, I mean, I think the cost, you know, the cost to society is certainly greater when you look at, you know, kind of the tail risk of it. But again, you know, how the node frames it, what, what, what I get worried about, I have no problem, you know, in, in him having an active defense and wanting to do everything in open AIs best interest. You know, I just don't want to see us attack technological progress, right? Which open, which open source obviously contributes to en route to that, right? Just compete against them heads up and win heads up. Like that's fine.
是的,太棒了。我很高兴你听到了。你知道,在过去几周中还有另一个有趣的案例,我知道你和我,实际上最后一件事就是,因为我刚才想起我和参议员的谈话。假设,假设你们都对Marism的做法是正确的。你必须担心这个。我们政府真的能够组织起一部有效的法律作品来解决这个问题的概率是多少呢?对,对,很低。我认为,从社会成本角度来看,当你考虑到这种尾风险时,成本当然更大。但是,你知道,节点如何来构思它,我担心的是,我对他有积极的防守和想要对OpenAI的一切都感兴趣并且符合Open source显然有益于这一路而进。只是竞争并取胜是可以接受的。
But let's not try to try to, you know, cap the other guys by taking their knees out before they even get started. So back to what I was saying, you know, speaking of government's role in business, you know, a couple of weeks ago, state of Delaware, the chance record, you know, this judge, Kathleen McCormick, she, you know, pretty shockingly struck down Elan's 2018 pay package. Remember the company was on the verge of bankruptcy. They basically cut a pay package with him where he took nothing if the company didn't improve. But if the company hit certain targets, he would get paid out, you know, 1% tranches of options. I think over 12 tranches, which because the company, right, had this extraordinary turnaround, you know, he achieved his goals.
但是让我们不要试图试图,你知道,通过在其他人开始之前就削弱他们的膝盖来压制他们。所以回到我所说的,你知道,说到政府在商业中的角色,你知道,几周前,特拉华州,机会记录,你知道,这位法官凯瑟琳·麦科密克,她,你知道,相当震惊地废除了Elan的2018年薪酬计划。记得公司当时濒临破产。他们基本上与他达成了一个薪酬计划,如果公司没有改善,他将得不到任何报酬。但如果公司达到了某些目标,他将获得支付1%的期权。我想大约有12个期权,因为这家公司,对吧,经历了这场非凡的转变,你知道,他实现了他的目标。
So now she's kind of Monday morning quarterbacking. She's looking back and she says his pay package is unfathomable. And she said, the board never asked the $55 billion question bill. Was it even necessary to pay him this to retain him and to achieve the company's goals? So of course, this can be appealed to the Delaware Supreme Court and it will be. But you know, in response to this, Elan, and I think many others just said, hold on a second here. What the hell just happened? You know, the state of Delaware has had this historical advantage in corporate law because of its predictability and its predictability wasn't because of the code, but it was because the judiciary, right? There was a lot of precedent in the state of Delaware.
所以现在她有点事后诸葛亮了。她回头看,说他的薪酬方案难以理解。她说,董事会从来没有问这550亿美元的问题。支付他这个数目是不是必要的,是为了留住他,实现公司的目标?当然,这可以向特拉华州最高法院上诉,将会这样做。但你知道,作为对此的回应,埃朗,以及我认为许多其他人,只是说,等一下,到底发生了什么?你知道,特拉华州在公司法上一直有这种历史优势,因为它的可预测性,这种可预测性不是因为法典,而是因为司法,对吧?在特拉华州有很多的先例。
And this seemed to turn that totally on its head. He said he was going to move, you know, incorporation to the state of Texas. You know, we're starting to see, you know, other companies follow suit and other people talking about this. So what was your reaction, you know, you know, seeing, you know, something that was, I think most of us thought was highly unlikely and pretty shocking. Yeah. Well, first of all, I think it's super important for everyone to pay attention to this. I don't, I don't actually think it's just an outlier event. I think it's so unprecedented in Delaware's history that it really marks a moment for everyone to pay attention. And there's a couple of things I would pay attention to. One data point you left out, which came up recently is the lawyers that pursued this case are asking for five or six billion dollars in payment. And it turns out when you bring a derivative suit in the, in, in Delaware, there have been cases where people ask for a percent and the judge gets to kind of decide that and, you know, if you step back and look, the, the, this is a victimless crime.
这似乎完全颠覆了这一切。他说他打算迁移,你知道,将注册地移到得克萨斯州。你知道,我们开始看到其他公司效仿,并有其他人谈论此事。那你的反应是什么呢,你看到这种我们大多数人认为高度不可能且相当令人震惊的事情?是的。首先,我认为每个人都应该关注这件事。我认为这不仅仅是一个偶然事件。这在特拉华州的历史中是如此前所未有,真的让每个人都应该关注。我会关注几个方面。你遗漏了一个数据点,最近出现的是追求此案的律师要求五六十亿美元的赔偿。事实证明,当你在特拉华州提起一个代理诉讼时,有些情况下人们会要求一定的比例,法官会决定这个比例,你知道,如果你退后一步看,这是一种无害的罪行。
And I think that's the thing that makes Delaware look like a kangaroo court here. The everyone knows the lawyer grabs someone that only had nine shares and those nine shares went way up, but it's kind of silly because it's so small. Anyway. So how could, how could a client with nine shares lead to a multi-billion dollar award to a lawyer? And that's only true. If you've created a, a bounty hunter system, you know, a bureaucratic bounty hunter system, there's something California called Paga that's kind of evolved this way. And, and if that's the new norm in Delaware, that's, that's really, really concerning. The other thing that's, that's different here is the stock went way, way up. So I think we've all become accustomed to when stock prices going down these, these litigators, you know, grab a handful of shareholders and bring a shareholder lawsuit and we're like, Oh, yeah. Yeah. Unfortunately, that's become a way of life.
我认为这就是让特拉华看起来像一个袋鼠法庭的原因。所有人都知道律师抓住一个只持有九股的人,而这九股价格大涨,但这有点荒谬,因为数量太小了。总之,那么一个持有九股的客户怎么可能导致律师获得数十亿美元的奖励呢?这只有一个解释,那就是你创造了一个赏金猎人系统,你知道,一种官僚的赏金猎人系统,加利福尼亚有一种叫做Paga的制度就是这样演变而来的。如果这种制度成为特拉华的新常态,那真的非常令人担忧。另一件不同的事情是股价暴涨。因此,我认为我们都习惯了当股价下跌时,这些诉讼律师抓住一大把股东提起股东诉讼,我们会说:“哦,是的。很遗憾,这已经成了一种生活方式。”
But, but to attack companies that go way up, you know, I, I would two things. One, I would offer this pay package. I looked at it in detail to any CEO I work with. And I think they would all turn it down because there was no, there's no cash, no guarantee. And the first trunch was a two X of the stock. So like that's fantastic. I think the biggest problem with compensation packages and you may may tackle that some other day is a misalignment with shareholders where, where people are getting paid when the stock doesn't move. That's RSU's do. And here, by the way, that's the standard in corporate America. We have this grift where people make a ton of money and the stock doesn't do anything. Look at the pay package for Mary Barra at GM. So the first trunch here was if the stock doubled and I would offer that to anyone. I would also say if any other CEO took a package like this, I would in a public company. I would be very encouraged to consider buying a lot of it. And so it's, it may be like one of the most, you know, shareholder aligned and center packages ever, which is exactly what you would think Delaware courts would be looking after. And I assess as well, which is a whole not a whole nother subject. But so it's, I think it's just really bad. And it does show a new side of Delaware, you know, one that they haven't shown before. And so I think everyone has to pay attention. Right. No, I mean, it's, it's shocking.
但是,攻击那些股价大涨的公司,你知道,我会做两件事。首先,我会向与我合作的任何CEO提供这种薪酬方案。我仔细看了一下,我认为他们都会拒绝,因为没有现金,也没有保障。而第一笔收入是股票的两倍。所以这简直太棒了。我认为薪酬方案最大的问题,也许哪天我们会深入探讨,就是与股东的利益不一致,当股票不涨的时候人们依然被支付。这就是 RSU。而在现在,在企业美国,这已经成为标准。我们有这种巧取豪夺的做法,人们赚了一大笔钱,但股价并没有涨。看看通用汽车的玛丽·巴拉的薪酬方案就知道了。所以这里的第一笔收入是股价翻倍的情况下。我也会说,如果任何其他CEO接受了这种薪酬方案,我会非常鼓励考虑买入大量股票。所以这可能是最符合股东利益的薪酬方案了,正是你所期望得到的特许法庭关注的事情。我也检验是否有,这是一个完全不同的话题。但是,我认为这非常糟糕,它展示了特拉华的新一面,是他们以前没有展示过的。我认为每个人都应该关注。没有,我的意思是,这是令人震惊的。
And if you, you know, I was a corporate lawyer in my, my first life, as you know, if you actually go and look at the, the actual corporate law code in the state of Delaware, right? It's almost word for word, the same as Texas, the same as California and so on. The point here is it's not that Delaware has code, you know, a legal code around, around corporations that's so much different than every other state. What has set it apart is it has way more legal precedent, way more trials that have occurred and judges who have interpreted that in a way that is very shareholder aligned, shareholder friendly. So the big, and they're known for letter of the law. So the construction. Correct. And so here we have a moment. And the reason it's so shocking is because it's at odds with all of the precedent that people had come to expect. So I think there are going to be left out, we left out there at 70% shareholder approval. I mean, and there was a low, high, low probability event that happened to happen. And you can't look at that after the fact and say, Oh, it was obvious this was going to happen, you know, right? I think, I think that, you know, if this is, if this stands, so I imagine corporations right now are in holding patterns, right? Elon is moving, you know, reincorporating in Texas. I think a lot of other corporations will stay pending the Delaware Supreme Court appeals ruling, right? If they over torn, overturn this, this judge is ruling, then I think you may be back to the status quo in the state of Delaware.
如果你知道,在我的第一份工作中,我是一名公司律师,你知道的,如果你实际去查看特拉华州的公司法典,对吗?它几乎与德克萨斯州、加利福尼亚州等州的相同,逐字逐句。这里的要点是,特拉华州并不是拥有与每个州都大不相同的公司法典,它之所以脱颖而出是因为有更多的法律先例、更多的审判以及法官们以与股东对齐、友好的方式解释法律。他们以严格遵守法律而闻名。因此,在这里我们有一个时刻。之所以让人震惊是因为这与人们已经习惯的所有先例相矛盾。所以我认为会有人将被置于一边,在70%的股东批准中会被遗漏。我认为这是一个低概率事件,发生了偶然的低概率事件。事后你不能说,哦,这显而易见会发生,对吧?我认为,如果这种情况持续下去,我想企业现在处于观望状态,对吧?埃隆正在搬迁,重新设立在德克萨斯州。我认为许多其他公司将暂时等待特拉华州最高法院的裁决。如果他们推翻这位法官的裁决,那么我认为特拉华州可能会重返现状。
But if they uphold the ruling and deny, I mean, I think Elon said, despite all the goodness that's occurred, saving the company from bankruptcy, this means he effectively gets paid zero for the last five years. I mean, it's such an outlandish outcome. So if it gets, if it gets upheld, I expect you're going to see significant flight from the state of Delaware by people reincorporating these other states that, you know, frankly are pretty friendly as well. Brad, I just thought of something. So if it's upheld and if these lawyers are paid anything as a percentage, anything other than maybe just their hourly fees, so if those two things happen, I would make the argument that every company in Delaware has to move to a different domicile because they could be sued in a future derivative lawsuit for the risk they've taken by staying in Delaware. Oh my God. Oh my God, you're so right. You are so right. Oh, mic drop on that. They, they, you know, so now on the boards that I sit on, I have to warn them that if they stay in the state of Delaware, then they're knowingly and negligently taking on this incremental risk. Absolutely.
但如果他们维持这个裁决并否认,我是说,我想埃隆说过,尽管发生了许多好事,拯救了公司免于破产,这意味着过去五年他实际上没有拿到任何报酬。这个结果太出乎意料了。如果这个裁决维持下来,我预计你会看到很多人从特拉华州搬离,重新在其他友好的州重新注册。布拉德,我突然想到了一件事。所以如果这个裁决维持下来,并且这些律师获得了任何百分比报酬,而不仅仅是他们的小时费用,如果这两件事同时发生,我认为每家公司都必须搬离特拉华州,因为他们可能在未来的派生诉讼中被起诉,因为他们决定留在特拉华州带来的风险。哦天啊。哦天啊,你说得太对了。在我所在的董事会上,我必须警告他们,如果他们选择留在特拉华州,在知情和疏忽的情况下接受这种额外的风险。绝对是这样。
Oh, wow. You know, let's just wrap with this, a quick market check. You know, one of the things I like to do is be responsive to the feedback we get. A lot of people, you know, loved, you know, kind of some of the charts we had put up on, on kind of the market checked on the last show. So you know, we get asked about this all the time. We sat on the prior pod, you know, prices have run a lot this year and the background noise around macro, you know, has not improved. Arguably it's getting a little worse. Inflation's running a little hotter. You know, rates are not expected to come down as much. So I, so I just a quick check on the multiples of companies that we really care about. Microsoft, Amazon, Apple, Meta, Google and Nvidia. And I just want to walk through this really quick. So this is a chart that just shows the multiples between March of 21 and March of 24. Right.
哇,你知道,让我们就用这个来结束吧,快速做个市场检查。我喜欢做的一件事是对我们收到的反馈做出响应。很多人,你知道,喜欢我们上一期节目中放出的一些图表。所以你知道,我们一直被问到这个问题。我们在上一期节目中说过,今年价格涨了很多,而宏观背景上的噪音并没有改善。可以说情况有点恶化。通货膨胀有点高。利率不会下降那么多。因此我只是快速检查一下我们真正在意的公司的估值情况。微软、亚马逊、苹果、美元、谷歌和英伟达。我只想快速走一遍这些。这是一个图表,显示了从2021年3月到2024年3月之间的估值情况。
So if we look at, let's start with, with meta, you know, you can look at that time. There are multiples gone from about 20 times earnings to about 23 times earnings. Right. So it's a little bit higher. Take a look at Google, you know, it's multiples come from, went has gone from about 25 earnings to now down to just below 20 times earnings. Now this is to be expected. I mean, we've been having this debate about whether or not, you know, Google search share is going to go down and the impact that that will have. And so, you know, this is just the markets voting machine at a moment time saying, hey, we hear that debate and we're a little bit more worried about those future cash flows than we were in March of 21, which makes a lot of sense to me. If you look at Apple, it too. And by the way, on that one, I mean, the Gemini release, the world's looking at you with this lens and then you release this thing that, and then you trip. I mean, they basically trip, right. And, and we know they trip because they've apologized for tripping. And so it's just not good. Like it's not confidence inspiring. Well, now you're seeing the drum beats starting, you know, you and I are getting the text, the emails, the drum beats are out and whether Sundar is going to, you know, make it past this moment in time.
因此,如果我们看一下,让我们从meta开始,你知道,你可以看看那个时候。从大约20倍盈利到大约23倍盈利,有多个。对。所以它略高一点。再看看谷歌,你知道,它的倍数已经从大约25倍盈利下降到现在略低于20倍盈利。现在这是可以预料的。我是说,我们一直在就谷歌搜索份额是否会下降以及其影响展开讨论。所以,你知道,这只是市场上的投票机在某一时刻说,嘿,我们听到了这个讨论,我们对未来现金流比我们在21年3月更担忧,这对我来说是有道理的。如果你看看苹果,也是如此。顺便说一句,在这一点上,我是说,双子座发布了,整个世界都在用这种视角看着你,然后你发行了这个东西,然后你绊倒了。我是说,他们基本上绊倒了,对。而且我们知道他们绊倒了,因为他们为绊倒道歉了。所以这就不好了。就像这并不令人信心满满。现在你看到了鼓声开始,你和我都在收到短信、电子邮件,鼓声传出,关于桑达是否能度过这个时刻。
I mean, listen, I think boards have one job, higher fire, the CEO who leads the company forward. Can they execute against the plan? And I think that if I was on the board of Google, that's the question I'd be asking at this moment in time. Not is he a good human being, not is he a smart product guy, not is he a good technologist, not what's happened over the course of the next last 10 years. But at this moment in time, do we have any risk of innovators dilemma? And is this the team? Is this the CEO who can lead us through what is likely to be a tricky moment.
我是说,听着,我认为董事会有一个职责,就是聘请一位能够推动公司发展的CEO。他们能否按照计划执行?如果我是Google的董事会成员,这就是我正在此刻提出的问题。不是他是否是一个好人,不是他是不是一个聪明的产品人,也不是他是不是一个优秀的技术专家,也不是在过去的十年里发生了什么。但是现在,我们是否面临着创新者困境的风险?现在这个团队是否足以让我们通过即将面临的困难时刻?
Just to finish it off, Apple's multiple is a little bit lower, right? That also makes sense to me. You see what's happening in China, some concerns about their, you know, they get $20 billion a year from Google, you know, like what happens to that? In the case of Microsoft, there are multiples a little higher, but you know, again, these multiples are all in the range. And then the final two, you know, Apple's multiple, or I mean, Amazon's multiple is actually quite a bit lower, you know, here. And so that's interesting to me. I actually think the retail business is doing better. I actually think the cloud business is doing better. And now that stock looks cheaper to me. And then Nvidia, of course, is the one that everybody's talking about. This goes back to where we started the show. I mean, if you look at Nvidia's multiple to start the year bill, you know, so hover there right above December 23, it's multiple was had, you know, like a five, 10 year low, right? But why? Because earnings exploded last year from five bucks to 25, you know, bucks.
为了结束这一切,苹果的市盈率稍微低一些,对吧?这对我也是有道理的。你看看中国发生的情况,对他们有一些担忧,你知道,他们每年从谷歌得到200亿美元,你知道,那会发生什么?在微软的情况下,它们的市盈率稍微高一些,但你知道,这些市盈率都在一定范围内。接下来是最后两个,你知道,苹果的市盈率,或者说,亚马逊的市盈率实际上要低得多。所以这对我很有趣。我其实认为零售业务做得更好。我其实认为云业务做得更好。而现在这只股票对我来说看起来更便宜。当然,大家都在谈论Nvidia。这又回到了我们节目开始的地方。我是说,如果你看看年初Nvidia的市盈率,接近12月23日,它的市盈率处于一个5-10年低点,对吧?为什么呢?因为去年收益从5美元增长到25美元。
And multiples obviously come up here a little bit at the start of the year, but you can see it's well below some of its historical really frothy multiples. But I think the question in my mind that we're big and share Nvidia shareholders, like in other people's minds is, you know, is this earnings train durable for Nvidia, right? Are these revenues durable? Have we pulled forward this training data? We showed that chart a couple of weeks ago that we think the future build, build out of compute and super compute of B 100s of everything is longer and wider than people think. And then the interesting thing, like when you see that note out of Klarna last week bill and what they were able to achieve, this is the, this is really the question. At the end of the day, our companies and consumers getting massive benefits out of the models and inference that's running on these chips.
而倍数显然在年初时出现了一点,但你可以看到它远低于一些历史上真正繁荣的倍数。但我认为我心中的问题是,我们作为英伟达股东,就像其他人的想法一样,你知道,英伟达的这种盈利模式是否可持续,对吗?这些收入是否持久?我们是否提前了这些训练数据?我们几周前展示了一张图表,我们认为未来计算和超级计算B 100的发展将比人们想象的更长、更广。然后有趣的是,当你看到上周克拉纳的消息,以及他们所能实现的成就,这真的是问题所在。最终,公司和消费者是否能从这些芯片上运行的模型和推论中获得巨大好处。
You know, if the answer is no, then all of these stocks are going lower. If the answer is yes, they probably have a lot of room to run. But that's the quick, you know, maybe we'll do this, you know, at the end of each of them, do a quick market check. But why don't we leave it there? It's good seeing you. Hey, next time get back out here. Let's do this together again. All right. Take it easy. As a reminder to everybody, just our opinions, not investment advice.
你知道,如果答案是否定的,那么所有这些股票都会走低。如果答案是肯定的,那么它们可能还有很大的上涨空间。但这只是一个快速的,你知道,也许我们每个股票结束时,可以快速市场检查一下。但为什么我们就在这里结束吧?很高兴见到你。嘿,下次再回来吧。我们再一起做这件事。好了,保重。作为提醒,这只是我们的观点,不是投资建议。