Why Vertical LLM Agents Are The New $1 Billion SaaS Opportunities
发布时间 2024-10-04 14:00:41 来源
摘要
As LLM’s become exponentially better it is clear that vertical AI agents are key to the next generation of billion dollar SaaS companies. In this episode of the Lightcone, the hosts sit down with YC alum Jake Heller, the co-founder and CEO of Casetext (which sold to Thomson Reuters for $650 million in cash in 2023) to discuss what it takes to build a successful vertical AI company and overcome resistance from industry veterans and skeptics.
Chapters (Powered by https://bit.ly/chapterme-yc) -
00:00 Coming Up
01:40 Building a successful vertical AI company
06:05 The unique challenges of law and AI
09:24 The turning point for lawyers with ChatGPT
11:25 Finding product market fit in legal
15:04 Entering deep founder mode
20:40 Approaching prompt engineering step by step
25:05 Going beyond GPT wrappers
28:10 Aiming for 100% accuracy
30:48 Thoughts on o1’s capabilities
36:42 Outro
GPT-4正在为你翻译摘要中......
中英文字稿 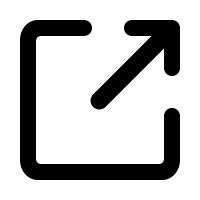
This is our first ever experience talking to this God-like feeling, you know, AI that was all of a sudden doing these tasks that would take me when I practiced the whole day and it's being done in a minute and a half. The whole company, all 120 of us did not sleep for those months before TPD4. We felt like we had this amazing opportunity to run far ahead of the market. That's why you're the first man on the moon. Yeah. Welcome back to another episode of The Lightcone. I'm Gary. This is Jared and Diana. Harge is out, but he'll be back on the next one. And today we have a very special guest, Jake Heller of Case Text. I think of Jake as a little bit like one of the first people on the surface of the moon. He created Case Text more than I think 11, 12 years ago, actually. And in the first 10 years, you went from zero to a hundred million dollars valuation. And then in a matter of two months after the release of GPD4, that valuation went to a liquid exit to Thomson Reuters for $650 million. So you have a lot of lessons about how to create real value from really like large language models. I think you were of our friends in YC, one of the first people to actually realize this is a sea change in revolution. And not only that, we're going to bet the company on it and you were super right. So welcome, Jake. Have it to be here.
这是我们第一次体验与这种神一样的感觉对话,你懂的,人工智能。突然之间,它完成了那些需要我练习一整天才能做到的任务,而且只需一分钟半的时间。整个公司,全体120人,在TPD4发布前的几个月都没有睡觉。我们觉得自己有一个绝佳的机会能遥遥领先于市场。这就是为什么你是第一个登上月球的人。欢迎回到《光锥》的另一集。我是Gary,这是Jared和Diana。Harge不在,但他会在下一集回来。今天我们有一位非常特别的嘉宾,Case Text的Jake Heller。我就像是在月球表面上行走的第一批人之一来形容Jake。他在大约11、12年前创建了Case Text。在前10年里,你们从零到达了价值一亿美元的估值。然后,在GPD4发布后的短短两个月内,估值以6.5亿美元被汤森路透收购。所以你有很多关于如何利用大规模语言模型创造真正价值的经验。我认为你在我们YC的朋友中,是最早意识到这是一场革命变革的人之一。不仅如此,您还将公司押注在这上面,而且事实证明你非常正确。所以欢迎你,Jake。很高兴你能来。
One of the cool things I think about Jake's story and reason why we wanted to bring him on today is that if you just look at the companies that good founders are starting now, it's a lot of vertical AI agents. I mean, I was trying to count the ones in S24. We have literally dozens of the YC companies in the last batch for building vertical specific AI agents. And I think Jake is the founder who is currently running the most successful vertical AI agent. It's by far the largest acquisition and it's actually deployed at scale in a lot of mission critical situations. And the inspiration for this was we hosted this retreat a few months ago and Jake gave an incredible talk about how he built it. And we thought that it would be super useful for people who watched the light cone who are interested in this area to hear directly from one of the most successful builders in this area, how he did it.
我认为杰克的故事很有趣,我们今天邀请他来的原因是,现在优秀的创业者正着手创建许多垂直领域的AI代理。我试着数了数在S24的那些公司,在最近一批YC创业公司中,有几十家都在构建特定垂直领域的AI代理。我认为,杰克是目前运营最成功的垂直AI代理的创始人。他的项目是迄今为止最大的一次收购,并且已经在许多关键任务的场景中大规模部署。这个灵感来源于几个月前我们组织的一次聚会,杰克在活动中做了一场精彩的演讲,分享了他是如何创建这个项目的。我们认为,对于那些看过光锥并对这个领域感兴趣的人来说,能直接听到这个领域中最成功的创建者之一的经验分享,益处非常大。
So how did you do it? Well, first of all, like a lot of these things, there's a certain amount of luck over the course of our decade long journey. We started investing very deeply in AI and natural language processing. And we became close with a number of different research labs, including some of the folks at OpenAI. And when it came time for them to start testing early versions, we didn't realize it was GPD for the time. What was GPD for? We got a very early kind of a view of it. And so, you know, months before the public release of GPD for, you know, we as a company, we're all under NDA all working on this thing. And I'll never forget the first time I saw it, it took maybe 48 hours for us to decide to take every single person of the company and shift what they were working on from, but the projects we were then working on at the time to 100 percent of the company all working on building this new product we call co-counsel based on the GPD for technology. How many people was that? We're about 120 people at the time. So you took like 120 people and completely changed where they were all working on. Yes, yes, yes. In 48 hours. Yes. And for the people watching case texts originally, I mean, had always been in the legal space, you're a lawyer and you built something for yourself. And you know, sort of the first versions of it were actually sort of annotated versions of case law, actually.
那么你是怎么做到的呢?首先,像很多这样的事情,我们在这十年的旅程中也有一定的运气成分。我们开始深入投资于人工智能和自然语言处理领域,并与包括OpenAI在内的多个研究实验室建立了密切关系。当他们准备开始测试早期版本时,我们不知道当时其实是GPD-4。GPD-4是什么呢?我们对它进行了非常早期的接触。所以在GPD-4公开发布的几个月之前,我们公司在保密协议(NDA)下,全体员工都在致力于这个项目。我永远不会忘记第一次看到它的情景,我们花了大约48小时来决定,将整个公司的每一个人从当时正在进行的项目中转移出来,全力投入到基于GPD-4技术的新产品co-counsel的开发上。当时公司有多少人呢?大约有120人。所以你把大约120人全部调整去从事新的工作?是的,是的,是的,在48小时内完成。而对于了解CaseText的观众来说,这家公司最初一直在法律领域运作,你本身就是一名律师,并且构建了一个属于你自己的东西。其最初的版本实际上是案例法的注释版本。
Yeah, that's exactly right. So in the very early origins, the company, the mission of the company, what we're always focused on is how can we build something that brings the best of technology to the legal space as a lawyer? I actually liked the job a lot. The parts of my job that I hated the most was when I had to interact with the technology that lawyers have to use regularly to get the job done. I remember thinking and this is like 2012 when I was at a law firm, if I would want to do something really trivial, I had like a new iPhone at the time, I can go and Google and find like movie times or where's the closest open, high restaurant with vegetarian options. That was super easy. But if I wanted to find the piece of evidence that was going to exonerate my client and make it so he doesn't have to do the jail for the rest of his life or the key legal case, they'll help me win a billion dollar lawsuit. Well, that's going to be like five days in the real until five AM every day. It's like there's got to be a better way. What is the process as a lawyer? You would have to read the stacks and stacks of documents. Pretty much. Yeah. Right before I started practicing, before everything went virtual or like online, you would literally be in a basement with bankers boxes full of documents, reading them one by one by one to try to find all the emails in a company like Pfizer or Google to see if there was potential fraud or.
是的,完全正确。在公司创立的初期,我们的使命始终是关注如何将最好的技术应用于法律领域。作为一名律师,我其实非常喜欢这份工作,但我最讨厌的一部分是与律师们为了完成工作而不得不经常使用的技术打交道。我记得大概是在2012年,我在一家律师事务所工作,如果我想做一些非常简单的事情,比如用新买的iPhone查找电影放映时间或者哪里有提供素食的餐厅,这非常简单。但如果我想找到可以证明我当事人清白的证据,或者能够帮助我赢得数亿美元官司的关键法律案件,那就需要每天熬到凌晨五点,花上五天的时间。作为律师的工作流程是什么呢?你得一页一页地阅读堆积如山的文件。在一切变成电子化或上线之前,你得真的呆在地下室,和放满文件的箱子待在一起,一个接一个地阅读,试图在像辉瑞或谷歌这样的公司中找到所有可能存在欺诈行为的电子邮件。显然,这个过程需要更好的方法。
And then if you wanted to find case law slightly before my time, you'd literally go to the library and open up books and just start reading. And new products were coming out that were some of the first web based research tools, but they're pretty clunky. It was just hard to find the relevant information. You couldn't do control F or I knew this stuff. Basically not. Yeah. And what was interesting about your background is you also happen to be the rare breed of having also computer science training. So this must have driven you nuts. Yeah, exactly. I mean, in the law firm, I'll never forget I was building like browser plugins to go on top of the tools I was using just to make my life more efficient and effective. And actually, one of the reasons I left the law firm to start a company and apply to YC was I got in trouble with the general council who thought, like, hey, why are you spending all your time doing this tech stuff? And also made it the time very clear that that my law firm owns all that technology. So I decided to do something different.
在我那时候以前,如果你想查找判例法,你真的得亲自去图书馆,打开书本开始读。而且,那时也有一些新的产品出来了,是最早的基于网络的研究工具之一,但它们用起来相当笨重。找到相关信息是很难的,你不能用快捷键来查找,基本上没有这些便利。这让我印象深刻的是,你有一个很特别的背景,你也有计算机科学的培训,所以这一定让你感到很烦恼。确实,我永远不会忘记在律师事务所的时候,我开发了一些浏览器插件,用在所使用的工具上,只是为了让工作更高效。实际上,我离开律师事务所创办公司并申请YC的原因之一,是因为总法律顾问对我总是在搞技术这件事情不满,并明确表示这些技术全都属于律师事务所所有。所以我决定去做一些不同的事情。
So do you want to tell us a little bit about the first 10 years of case text, the sort of like long slog in the pre LLM era? One of the lessons here, I think, that I took away from that time period is that when you start a company, you may not get the exact right. You may have like the right kind of general direction. You know, there's a problem. You're trying to solve it, but it could take a very long time to figure out what the solution is. For us, for example, you know, we saw that there was this kind of combined issue of like bad technology and legal sphere, but also like this very like a lot of lawyers use content to do things like research and understanding what the law is. And so we thought, OK, well, we can do the technology better, but how are we going to get this content? And we spent like a couple of years trying to get, as Gary said, lawyers to annotate case law and to provide information.
所以,你想跟我们说说 CaseText 在第一个十年的情况吗?比如说在大语言模型(LLM)出现之前那段漫长且艰难的过程。我从那段时间中学到的一个教训是,创业初期可能会走对大方向,但不一定一开始就能找到正确的解决方案。你会知道有个问题需要解决,但找出解决方法可能需要很长时间。比如说,我们当时发现法律领域有技术上的缺陷,同时又有很多律师依赖内容来进行研究和理解法律。因此,我们意识到技术可以做得更好,但如何获取这些内容是个难题。就像 Gary 说的,我们花了几年的时间,试图让律师们标注判例法并提供信息。
So it's like a UGC site, like the user generating. Yeah, that was a big focus of ours, like the kind of one to punch of better technology, but also better content. We, you know, at the time, our heroes were like stuck over flow and Wikipedia and GitHub and other kind of open source or UGC kind of websites. And it was a total failure. Like we could not get lawyers to contribute their time and information. And I think these are just different populations. The typical Wikipedia editor has more time on their hands than they know what to do with. And so they're adding not all, but many do and they're adding content for free and and altruistically lawyers, bill by the hour, their time is incredibly valuable. They're always running at a time. They had no time to kind of contribute to the UGC site.
所以这就像一个用户生成内容(UGC)的网站,用户自己生成内容。是的,这一直是我们的一个重点,就像一种双管齐下的方法,一方面是更好的技术,另一方面是更好的内容。那时候,我们的榜样是像stackoverflow、Wikipedia和GitHub这样的开源或UGC网站。但这个计划完全失败了。我们根本无法让律师贡献他们的时间和信息。我认为这是因为用户群体的不同。典型的Wikipedia编辑有很多空余时间,不知道该做什么,所以他们免费且无私地添加内容,而律师则按小时计费,他们的时间极其宝贵,总是很忙,几乎没有时间来为UGC网站做贡献。
So we had to pivot and we started investing investing very deeply at the time it was not called AI. It's just like natural processing and machine learning and saw that first of all, we didn't need to create all this UGC like to to replicate some of the best benefits of what our competitors had in these big content databases. Some of it you can basically do even then kind of automated basis. And then also we were starting to create these user experiences that were a lot better than when our competitors could offer based on then at the time, what seems kind of quaint, like AI stuff, like, you know, the same recommendation algorithm that powers Pandora and Spotify's like recommended music. You can use it with a look at basically is this song relates to that song. People listen this also into this and this. Right.
因此,我们不得不转变方向,开始深入投资当时还不叫做人工智能的领域。它更像是自然语言处理和机器学习。首先,我们发现不需要像竞争对手那样创建大量用户生成内容,就能复制他们大型内容数据库中的某些最佳优势。当时,有些事情就已经可以在一定程度上实现自动化。此外,我们还开始创造出比竞争对手更好的用户体验,这基于当时看起来有些古老的“人工智能”技术,比如用于 Pandora 和 Spotify 的推荐算法。这些算法能根据歌曲之间的关系进行推荐:如果人们听了这首歌,也会听那首歌,等等。
Similarly, we looked at, OK, cases that cite to other cases, they all reference earlier opinions, you know, they kind of build out this network of citations and we found ways that we can check a lawyer's work. They'd upload their work so far and be like, well, everybody who talks about this case too and you miss that. So cool experiences like that. But the truth is until the very end until co-council, a lot of what we did were relatively speaking, kind of incremental improvements on the legal workflow. And one of the things that's kind of weird about this is when there's just an incremental improvement, it's actually pretty easy to ignore.
同样地,我们研究了案例之间的引用关系。每个案例都会参考更早的判决,这样它们就构成了一个引用网络。我们找到了方法来检查律师的工作。他们会上传他们的工作进展,我们会指出类似"所有讨论这个案例的人也提到了其他案例,你漏掉了这个"这样的问题。这些都是很酷的体验。但事实上,直到最终合作阶段,我们所做的大多数事情在法律流程上都是相对来说的小改进。有点奇怪的是,当只是一点点改进时,其实很容易被忽略。
A lot of our clients, they would never say this literally, but you kind of this impression, you walk into the room, their office, and you try to pitch them a product and say, this is going to change everything about the way you practice. And they go, well, I make five million dollars a year. I don't want nothing to change. This technology, yeah, it's not I do not want to introduce anything that has the opportunity to make my life at all worse or potentially worse or potentially more efficient because they build by the hour. It was really only after like much later when Chachi GPD came out. You know, at the time we were privately and secretly working on GPD for Chachi PPD came out and all of a sudden every lawyer in America, probably in the world, saw, oh my God, I don't know exactly how this is going to change my work, but it's going to change it very substantially. Like they could feel it.
很多客户并不会直接这样说,但你会有这样的感觉:当你走进他们的办公室,试图向他们推销一个产品,并宣称这将彻底改变他们的工作方式时,他们会说:“我每年赚五百万美元,我可不希望有任何改变。”他们对于新技术的态度通常是拒绝的,因为他们不想引入任何可能会让生活变得更糟或可能带来变化的东西,尤其是因为他们按小时收费。真正发生变化是在很久之后,当ChatGPT出现时,我们当时私下和秘密地在为GPT做研究。而当ChatGPT一问世时,突然间美国,甚至可能是全世界的律师都意识到,“天啊,我不知道这将如何改变我的工作,但它一定会带来很大的变化。”他们能够感受到这一点。
And the same guys and gals were telling us I make five million dollars a year. Why would it change anything about my life? We're like, I make five million dollars a year. This is going to change something. I need to be ahead of this. The technology itself, and we'll get into the second, really changed what we build for lawyers, but also the market perceptions of what was like, what was necessary really changed as well. And for the first time in our 10 years, even before we launched co-council publicly based on GPD for, they were calling us and like, you know, we know you work on AI. We need to get on top of this. What can you show us? What can we work on? And I think it's because the change was on incremental anymore. It was like fundamental. And all of a sudden they had to pay attention. They could not ignore it.
同样的人曾经告诉我们:"我每年赚五百万美元。为什么这会改变我的生活呢?" 我们则认为:"我每年赚五百万美元。这将改变一些事情。我需要走在前面。" 技术本身——我们稍后会详细讨论——确实改变了我们为律师们开发的产品,但同时也改变了市场对什么是必要的看法。在我们十年的历程中,这是第一次,即便是在我们基于GPD技术推出联合顾问服务之前,他们就打电话给我们,说:"我们知道你们在研究人工智能。我们需要跟上这个潮流。你能给我们展示些什么?我们能一起开发些什么?" 我认为这是因为变化不再是渐进式的,而是根本性的。突然之间,他们不得不重视这些变化,无法再忽视了。
I guess the mental model I have for you is there's this concept of the idea maze. The founder goes in the beginning of the maze and they're just like feeling around, like actually in the arena talking to customers, learning like where are the walls, which path to go? Should I go left or right? And then as is actually common for startup founders in the idea maze, you will actually reach a dead end and then usually you have to pivot. Yeah. And then I think you have a very interesting story because you were sort of towards the end of maybe like one of the parts that weren't going to get you all the way to product market fit, but then L M's drop and then it's like the maze got shaken up. Yeah. And then you're actually much closer to product market fit than absolutely anyone else. And so that's why it's not crazy time. Yeah, it's exactly right. That's why you're the first man on the moon. Yeah.
我对你的看法有点像是“创意迷宫”这个概念。创始人进入迷宫的起点,他们像是在黑暗中摸索,实际上是在市场中与客户沟通,学习辨别哪些是障碍、哪条路可以走?应该向左还是向右?对于创业公司的创始人来说,通常会遇到死胡同,这时候就需要转变方向。你的经历很有趣,因为你似乎走到了一条可能无法达到产品市场契合度的路的尽头,但当L M出炉时,迷宫被重新洗牌。而这使得你比其他任何人都更接近产品市场契合。所以,现在不是疯狂的时候。这就是为什么你是第一个登上月球的人。
Yeah. I think there's there's something to that. And the thing is, you know, each time we got progressed to that maze, it felt like maybe now we're a product market fit. You know, we were making real revenue before we launched co-counsel and we had real customers and they said really great things about us. I keep on thinking about this article written by Mark Andreessen in like the early 2000s. I think it's called the only thing that matters. And in it, he describes that what it feels like to have product market fit. He lists things like your servers will go down. You can't hire support people and salespeople fast enough. You're going to eat for a year free at bucks. The kind of famous woodside, you know, diner where where a lot of VCs will take you the the process. And I read that really on in my like, like, you know, career. And I was like, OK, well, that's like hyperbolic. But when we launched co-counsel, it was literally exactly that. Our servers are going down. We could not hire support people fast enough. We can hire sales people fast enough. I ate a lot of bucks. You know, before we were it was a really big day if we're in the ABA journal or some other, you know, legal specific publication, we were on CNN and MSNBC. Like, you know, all of a sudden, everything changed. And that's what real product market fit looks like. I think market markets, even in like 2005 or never the article came out. Exactly right about like in 2023. Can you talk about that crazy time? Because there's only two months from when you launched co-counsel to getting bought for $650 million. So like what happened in those two months? Well, to be clear, the transaction only closed six months after you launched, but it was two months of the conversation started. And so so we started building co-counsel. And for just just to kind of background purposes, the idea we came up with, again, like 48 hours, like a weekend after seeing GPT four was and it's something that is not the kind of sound crazy today, but it felt crazy at the time, which is this AI legal assistant by which we mean it's like almost like a new member of the firm. You can just talk to it, not unlike how you might talk to something like chat or GPT today and give it tasks like I need you to read these a million documents for me and tell me if there's any evidence fraud happening in this company. And then within a couple of hours, it's like I've read all the documents. Here's what the summary is or summarized documents or do legal research and put together a whole memo after researching hundreds of thousands of cases answering the lawyer's initial research question. And so in that sense, it was this like really powerful extension of the workforce of his law firms. That was the concept from the beginning. And we made a very early initial version of it. And we started because we couldn't under our agreement with OpenAI, we could not be public about this product, but they did let us extend the NDA to handful of our customers. And so we started having our customers use it. And so for months before GPT four was launched publicly, we had a number of law firms and like they had no idea they're using GPT four, but they are like, something really special, right? This is actually even before chat GPT. So there is their first ever experience talking to this God-like feeling, AI that was all of a sudden doing these tasks that would take me when I practice the whole day and it's being done in a minute and a half. Right. And so as you might imagine, it was nuts. I mean, first of all, the whole company, all 120 of us did not sleep for those months before GPT four was like public launch. They're very good public launch the product. We felt like we had this amazing opportunity to run far ahead of the market. Something really beautiful happens when everybody's working super, super hard, which is you iterate so quickly past.
好的。我觉得这里面确实有一些值得思考的东西。关键是,每次我们在这个迷宫中前进时,我们都觉得自己可能终于找到了产品与市场的契合点。在我们推出"共同顾问"之前,我们已经在赚取实际收入,并且拥有真正的客户,他们对我们给予了高度评价。我一直在想马克·安德森在2000年代初写的那篇文章,好像叫《唯一重要的事》。他在文章中描述了拥有产品市场契合度的感觉。他提到的一些现象包括:服务器会崩溃,你无法快速招聘足够的支持和销售人员,你将在一个著名的地方免费吃上整整一年。我在职业生涯的早期读到这篇文章,当时觉得这些都是夸张的表现。但当我们推出"共同顾问"时,情况真的是完全一样:我们的服务器不断崩溃,我们无法快速招聘足够的支持和销售人员。我吃过很多次的那个著名餐馆。以前,如果我们能被《美国律师杂志》或者其他法律领域的出版物报道,那都是一个大日子,而现在我们上了CNN和MSNBC。突然之间,一切都改变了,这就是产品市场契合度的真实体现。我觉得即便在2005年或其他任何时间,安德森在文章中对2023年的预测都完全正确。你能谈谈那段疯狂的时光吗?因为从我们推出"共同顾问"到被以6.5亿美元收购,只过了两个月。两个月里到底发生了什么?需要澄清的是,交易完成是在推出后六个月,但是两个对话是在两个月后开始的。
我们开始构想“共同顾问”,背景是看到GPT-4后仅48小时内的一个周末。这个想法在当时听起来很疯狂,但现在似乎并不奇怪。它是一个AI法律助手,就像是一个新的团队成员。你可以直接与它交流,类似于现在你可能会和一个聊天机器人或GPT对话,给它分配任务,比如让它阅读几百万份文件,告诉你这家公司是否存在任何欺诈证据。在几个小时内,助手就会回答:"我已经阅读完了,这里是总结"。或者它可以总结文件、进行法律研究,并整理出一份详细的备忘录,回答律师的初步研究问题。从一开始,这是一个对律师事务所的工作力量的强大扩展。
我们做了一个非常早期的初始版本,并且由于与OpenAI的协议,我们不能公开这个产品,但他们允许我们把保密协议扩展到一些客户。因此,在GPT-4公开发布前的几个月里,我们有一些律师事务所在使用,但他们不知道自己在使用GPT-4——他们只是知道有某种特别的东西。这甚至是在ChatGPT出现之前,所以他们第一次体验这种犹如神一般的AI,完成这些任务的速度让从业律师震惊。在宣布新产品之前,公司全体120人几乎几个月没怎么睡觉。推出产品时,我们觉得自己有一个绝佳的机会能在市场上遥遥领先。每个人都在超级努力地工作,快速迭代,这种美丽的事情就在这种背景下发生。
And actually, I still see some companies out there. They're stuck where we were in the first month of seeing GPT four, right? And I think it's because they're just not like as intensely focused and engaged as we were able to be during those like couple, about six months or so before the public launch of GPT four. You kind of to do this transition, you had to shake the company. You kind of went into deep founder mode. Yeah, because there was a lot of pushback from employees. I was like, oh, this thing was working. Why should we go into through ourselves into the deep end of AI? And tell us about that founder mode moment for you.
其实,我发现现在还有一些公司仍然停留在我们初次接触GPT-4时的状态。我认为,这是因为他们没能像我们在GPT-4公测前那六个月那样,保持高度专注和积极投入。要实现这种转变,你需要彻底振兴公司,就像进入了一种深度创始人模式。当时遇到了不少员工的反对意见,他们觉得原来的东西运作良好,为什么要把自己全力投入到AI的深水区呢?能跟我们谈谈你那时候作为创始人的体验吗?
And so first of all, like this is especially true. We've been running a business for 10 years because they have seen you wander through that maze and and bump into dead ends. And a lot of those folks have been there for most or all that time. Watching, you know, me as the founder saying, we're definitely going this direction, it's definitely going to work. And it's sometimes it doesn't. And you only get so many of those with employees, right? So this was maybe my last one that I had with some of these folks. And they're like, here, Jake goes again with this crazy new technology and some idea we're going to invest deeply in. And yeah, it took some job to convince people.
首先,这一点尤其正确。因为我们已经经营这家企业10年了,他们亲眼目睹了你在这个迷宫中摸爬滚打,不断遇到死胡同。而很多员工在大部分或全部时间里一直陪伴着我们。他们看到我作为创始人在说:“我们一定要朝这个方向走,一定会成功。”然而,有时候并没有成功。你和员工之间这样经历的次数是有限的,对吧?所以这可能是我和其中一些员工一起经历的最后一次。他们心想,杰克又要开始投入这项疯狂的新技术和一些想法了。是的,要说服大家确实花了不少功夫。
And if you imagine like what some of the different roles are, if you're in the go to market role, if you're if you're selling or marketing a product and we're making, you know, we're growing 70, 80 percent year over year, we're between 15 and $20 million in ARR. Things were like terrible, right? That's great. Yeah, we're great. Yeah. We but like so they're like, what's why are we even the board? You know, some of the members like I get this immediately and some of them had to be persuaded, right? And about the founder mode moment, like one thing that really worked for me is I led the way through example, I built the first version of it myself. Wow. Even with 120 person company with like a whole bunch of engineers and lawyers and stuff like before that, you like opened up your like ID and actually built the thing yourself. Oh, yeah.
如果我们设想一下不同的角色,比如说市场拓展岗位,如果你在销售或营销某个产品,并且我们每年以70%到80%的速度增长,年经常性收入在1500万到2000万美元之间。事情看似糟糕,其实不然。对,我们做得很出色。甚至有些董事会成员会质疑我们的存在价值,虽然有些人瞬间明白我们的重要性,但也有些人需要被说服。而关于创始人角色的时刻,有一件事对我特别有效:我以身作则,亲自开发了第一版产品。即便在一个有120名员工,包括众多工程师和律师的公司里,我仍然亲自编写代码,打造了这个产品。
And part of it was the NDA only sent it at first to me and my co-founder. That was it. That was a blessing, that was it. It turned out to be like perfect. And even after we got extended a little bit, we kept it pretty small at first for the first like, you know, a little bit of time. I made my mind within 40 hours, all companies need to do this. But we actually only told the company, I think a week and a half after we first got access. During that week and a half, we built the very first version, like prototype version of this. And again, I won't I've never forget this. The timing is just so funny. Like we saw it on like a Friday, we had it all weekend long, we're working with it. And then Monday was an executive off site where everybody came, all my executives came and they expected to work. We're going to be talking about how we're going to hit our sales target for the next quarter, however, we're talking about none of that. You know, we are talking about something totally different right now. Let me show you something in my laptop. You know, so yeah, I built the first version myself.
部分原因是最初只有我和我的联合创始人收到了保密协议(NDA)。就是这样。这是一个幸运之事,也是一个完美的开始。即使后来我们稍微扩大了一点参与范围,最初的一段时间我们还是保持了很小的规模。在短短的40小时内,我就下定了决心,所有公司都应该这样做。但实际上,我们是在第一次获得访问权限后一周半才告诉公司的。在那一周半的时间里,我们构建了这个项目的第一个版本,也就是原型版本。我永远不会忘记这样的时间节点:我们是在一个星期五看到它的,然后整个周末都在忙着这件事。到了星期一,我们有一个高管的外出会议,所有高管都来了,他们原本期望的是讨论下个季度如何实现销售目标的计划,但我们讨论的内容完全不一样。我对大家说:“让我在笔记本电脑上给你们展示一些东西。”我自己完成了这个第一版本的构建。
But going through that process, me and then a handful of other people, I think was really helpful. And we also brought in customers early and that helped convince a lot of people. As soon as like a skeptical sales or marketing or whatever person or even engineer was on the other line at end of the Zoom call where a customer was was reacting to the product in real time and giving us their honest reactions and like seeing the look on their face. And you have to imagine it's almost hard to imagine that the world was like pre chat GPT. But there are some of these who were seeing that exact idea for the first time. And they were they were just blown away. And that really changed minds quickly. I mean, we saw people go through like existential crises, live, you know, at Zoom calls like, oh, we could see their expression change.
通过这个过程,我和另外几个人一起努力,我认为这真的很有帮助。我们还在早期就邀请了客户参与,这帮助说服了很多人。每当在Zoom会议的另一端,一个持怀疑态度的销售或市场人员,甚至是工程师,听到客户对产品的实时反应并给予他们诚实的反馈时,看到他们脸上的表情,你不得不想象一下,很难想象这是在ChatGPT之前的世界。但当时确实有人第一次看到这样的想法,他们感到非常震惊。这真的改变了很多人的想法。我们亲眼看到,在Zoom会议中,有人经历了类似存在主义危机的时刻,他们的表情发生了变化。
Exactly. On all kinds of ways, it's like, what am I going to do? A lot of the very common reaction amongst the senior attorneys we showed it to was like, well, they got a retired suit. Like, you know, I have to deal with this. And some of this was really driven by GPT for coming out. Like you had access to three. You had access even to two, I think. Yeah. We were in a close relationship. We're with a lot of the labs, but including opening on, they kept on showing us stuff kind of early on in its development. And they're like, well, can you build something with this for legal? And every time we're like, no, this sucks. Like, you know, by by time you got to three and three point five, it was like, OK, well, this is plausible sounding English and it sounds kind of a lawyer. So kudos to that. But it's just making stuff up wildly.
好的。在很多方面,这种感觉就像:“我该怎么办?”我们给一些资深律师展示这个技术时,他们的常见反应是:“好吧,他们已经退休了。”就好像他们必须要应对这个变化。而这种反应在很大程度上是因为GPT技术的发展,特别是GPT-4的推出。之前你可以使用GPT-3,甚至是GPT-2。我们与许多实验室有着紧密的合作关系,包括OpenAI。他们在开发过程的早期阶段就给我们展示了一些成果,并问我们是否可以用这个技术为法律行业开发一些东西。每次我们都会说:“不行,这不行。”但当发展到GPT-3和3.5时,我们觉得这个技术可以形成看似合理的英语,并有点像律师用语,所以值得称赞。但它仍然在编造内容,完全不靠谱。
Like we just did it's very hard to connect it to a real use case, especially in legal where it's so important that you actually get the facts right that you can't hallucinate, you can't even make the wrong kinds of assumptions. And we had to do a lot of work with those earlier models to even get them close to usable. And they just weren't really like one like totem or like one example along the way. Is when GPD 3.5 came out that the study was run and it showed that GPD 3.5 got a 10th percentile on the bar passage. So like it did better than some people actually, but the 10% of them. Yeah, probably the ones are just filling it out randomly, basically.
就像我们刚刚所说的那样,很难将它与实际应用场景,尤其是法律领域联系起来。在法律领域中,准确获取事实是非常重要的,不能凭空想象,也不能做出错误的假设。我们在使用早期的模型时,付出了大量努力才让它们勉强接近可用状态。而当GPD 3.5问世时,人们进行了一项研究,结果表明GPD 3.5在律师资格考试中的表现处于第10百分位。这意味着它的表现比一些人要好,但这些人可能只是随便填写的。
When we got early on the GPD four, we're like, let's run the study again, too. And we work with the open AI, like we were going to confirm this. This test is not in the training set and wasn't totally to test to it. And the test we ran did better than 90% of the test acres. Right. So this is a big difference. And also we started running some tests like, OK, here's like four or five cases to read using those cases, write a memo, respond to this question. And we did a lot of prompt work to get it to essentially just do it accurately to cite the actual things in the in context that we gave it and not make things up. And we're like, OK, well, this is very different than we saw before.
当我们刚接触到GPD四号时,我们决定再进行一次研究。我们与OpenAI合作,想要确认这个测试不在训练集里,并确保测试的真实性。测试结果显示,我们的测试比90%的测试者表现得更好。这是一个巨大的差异。此外,我们开始进行一些测试,比如:展示四到五个案例,通过这些案例写一份备忘录,回答问题。我们做了很多提示工作,确保测试能够准确引用我们提供的上下文,而不是凭空捏造。结果发现,这与我们之前看到的非常不同。
So is a big moment for us. And honestly, I'm not sure what the mindset was of the researchers we were working with. But almost felt like by the time we were having that meeting, it felt like one of those other meetings we'd had in the past where we were getting ready to say, like, this, this is not going to work for legal on trying. And I think they saw us go through maybe some form of the existential crisis on that call that our customers did. You're like, oh, wait, this is super, super, super different. I guess, you know, today we have one. We have chain of thoughts, reasoning. I think a lot of people look at it as it's not merely the text itself, but also the instructions that lead up to the workflow.
这对我们来说是一个重要的时刻。老实说,我并不太确定我们合作的研究人员当时的心态是什么。但几乎感觉在我们进行那次会议的时候,就像我们过去曾有过的那些会议之一,在那些会议上,我们准备好去说,“这不可能在法律上行得通。”我想,当时在电话中,他们可能看到了我们所经历的一种类似于客户所经历的存在危机。突然之间,我们意识到,“哦,等等,这跟之前的完全不一样。”我想,现在我们拥有了一种新的思维链和推理能力。很多人认为这不仅仅是文本本身,还有预先设定的工作流程指示。
But, you know, way at the beginning, nobody knew any of this stuff. How did you start? You had your sort of tests that you had written for previous versions of the model, they outperformed. But then there's this moment where you say, OK, well, now it's something, but what do we do next and how do we do it? So the process that we started with then, and it's actually not too just similar to what we're doing today, it started with a question of like, OK, well, what problem are we trying to solve for the user? Right. The user wants to do research, legal research. So and they went like a memo answering their question with citations to the original source. So like, that's the end result. And then we're like, OK, well, how do we go from that end result, like working backwards almost, what would it take to get there? And what ends up happening a lot with the things that we built for co-counsel, we called them skills, which felt very unique. And at the time, I think a lot of companies now call their AI capability skills.
在一开始的时候,你知道,没人了解这些东西。你是怎么开始的?你有之前版本模型的测试,它们表现不错。但是到了某个时刻,你意识到,好的,现在有了一些东西,但接下来我们该做什么,怎么做?所以我们当时开始的过程,其实和我们今天做的不太不一样,是从一个问题开始的,就是我们要为用户解决什么问题?用户想要做研究,特别是法律研究。他们希望得到一个带有原始出处引用的备忘录来回答他们的问题。所以那是最终的结果。然后我们像是反向推导一样,从那个结果往回推,需要做些什么才能达到那一步?我们为共同顾问构建的很多东西,最终被称为技能,这感觉非常独特。那时,我认为很多公司现在都把他们的人工智能功能称作技能。
So when you're building these skills, it turns out it usually takes a lot of work to go from, like, say, the customer inputs something, say, like a set of documents or a question or what have you to the end result that they're looking for. And the way that we thought about it was how would the best attorney in the world approach this problem? And so in the case of research, for example, the best attorney would get the request safe from a partner and then break that request down into like actual search queries that run against these platforms. And sometimes they use special search syntax. It looks actually pretty like SQL almost, right? So like from the English language query, you have to break it down to these different kind of search queries, maybe a dozen different search queries. You were being really diligent. And then they'd execute the search queries against these databases of law. And they come back with, say, like a hundred results each.
在构建这些技能时,你会发现通常需要大量努力才能从客户输入的内容(比如一组文件或一个问题)到达他们所期望的最终结果。我们考虑的方式是:世界上最优秀的律师会如何解决这个问题?以研究为例,顶尖律师会从合伙人那里接收请求,然后将这个请求分解成具体的搜索查询,在这些平台上运行。有时他们会使用特殊的搜索语法,其实看起来很像SQL语言。因此,你需要从英文查询语句将其分解成不同的搜索查询,可能需要进行十几种不同的搜索查询,以确保万无一失。然后,他们会在法律数据库中执行这些搜索查询,每个查询可能会返回大约一百个结果。
And then, you know, the most diligent, best attorney with sit down just to read every single one of these results that come back, all the case law, statutes, regulations. And you start to do things like make notes and summarize and kind of compile it and outline of what your response might be. Like line by line, paragraph by paragraph, actually. Yeah, it's 100%. And you start like just taking out those like insights or getting from what you're reading and then finally based on all of that work and all those citations you've gathered, et cetera, then finally you put together your, your, you know, research memo and so we're like, OK, well, each one of those steps along the way for the vast majority of them, those were impossible to accomplish with previous technology, but now they're their prompts.
然后,你知道,那些最勤奋、最优秀的律师就会坐下来,逐字逐句地阅读返回的所有结果,包括案例法、法规、条例等。你开始做笔记、总结,并整理出一个大纲,构思你的回应可能是什么样的,逐行逐段地进行处理。确实是100%的投入。然后你会从阅读中提炼出见解。最后,基于所有这些工作和你收集的引文,整理出你的调研备忘录。我们意识到,以前的技术很难完成这些步骤中的大部分,但现在它们变成了一系列提示。
Think step by step. Yeah, think step by step. Yeah, exactly. But we actually broke it down each, each, you know, so getting to the final result may be a dozen or two dozen different individual prompts, each of which might, by the way, be thinking step by step themselves. But and then for that, for each of those prompts, you know, as part of this, like chain of actions you take to get to the final result, we get a very clear sense of what good looks like and we're able, you know, we had a series like a battery of tests before, but this got way more intense where we'd write at first, maybe if he doesn't tests and then if you hundred and if you thousand for every single of those prompts.
逐步思考。对,逐步思考。没错。为了达到最终结果,我们实际上把每个步骤进行了拆分,可能有十几到二十几个不同的细节,每个细节本身也需要逐步思考。然后,对于每一个步骤,在这个为了获得最终结果的链式操作中,我们可以非常清晰地知道什么是好的表现。我们之前有一系列测试,但这个过程变得更加密集。最初可能做几十次测试,然后是几百次,甚至对每个步骤做上千次测试。
So, you know, if the job to be done in the very beginning of this research process, for example, is taking the English language query and breaking it down into search queries, we had a very clear sense of what good search queries look like and wrote like gold standard answers for given this input. This is what the app looks like, right? And so our prompt engineers, and I was one of them at the very beginning, we all just kind of in it together. We're writing these English language prompts to try to, you know, write the test first, basically, and we're with these English language prompts to try to get it. So of a thousand 200 times, they got the right answer 1,199 times or what have you.
所以,你知道,在研究过程的一开始,如果要完成的任务是将英语查询分解为搜索查询,我们对优秀搜索查询的样子有一个非常明确的理解,并为给定输入编写了类似于黄金标准的答案。这就是应用程序的表现,对吧?所以我们的提示工程师,其中一开始我也是其中之一,我们都一起努力。我们编写这些英语提示,基本上是先编写测试,并通过这些提示尝试获得正确的结果。因此,在1200次中,他们有1199次得到正确的答案之类的。
Just sort of like a test driven development. Yeah. Really approach from doing software engineering to prompt. That's exactly right. And the funny thing is I never really believe in test driven development before prompting like I was like, oh, the code works. It doesn't. It's fine. Like you'll see it when you but like with prompting actually, I think it becomes even more important because of the kind of like nature of these LMS that they might go in crazy directions unexpectedly. And so, you know, you might very easily add an incentive instruction to solve one problem you're seeing with these sets of tests and then to break something with sets of tests. And so that exact kind of theory of kind of testing development applies, you know, 10x more, I'd say in the world of prompting.
就像测试驱动开发一样。是的,确实是从软件工程的角度转到提示工程。这确实是对的。搞笑的是,在开始使用提示之前,我从来不是真正相信测试驱动开发的。我之前的想法是代码能运行就行,没问题。但在使用提示时,我认为测试变得更加重要,这是因为这些语言模型的特性,它们可能会意外地朝疯狂的方向发展。所以,你可能会很容易地添加一个激励指令来解决你在这些测试中看到的问题,但也可能会因为这些测试而弄坏一些东西。这种测试开发的理论在提示的世界里,我想可以说重要性提高了十倍。
There's a lot of sort of the naysayers saying that a lot of companies are just building GP rappers and there's not a lot of IP getting built. But it's actually there's a lot of finesse to how you explain all of these. Like you tell us about all of that and how much more there's to be built. Oh, yeah. I mean, I think the thing is we're actually trying to solve a problem for a customer and actually doing the job in our case of like what a young associate might do and do it really well. There are many layers of things you have to add in to actually get the job done. And by the time you like add that all up, you're not like a GPT rapper, you're a full application that may include in our case proprietary data sets like the law itself and our annotations to the law that we added automatically. It may include connections into customer databases.
有很多持怀疑态度的人认为许多公司只是在构建GPT的外壳,并没有创造太多的知识产权。但实际上,解释这些事物是非常有技巧的。比如,你告诉我们关于这些的所有事情,以及还有多少东西需要构建。哦,是的。我的意思是,我们实际上是在为客户解决问题,在我们的案例中,相当于让年轻的助理做好他们的工作。要真正完成这项工作,需要添加很多层的东西。当你把这一切加在一起时,你不再只是一个GPT的外壳,而是一个完整的应用程序。我们可能会包括专有数据集,比如法律本身和我们自动添加的对法律的注释,并可能包括与客户数据库的连接。
In our case and legal, they have these very specific legal specific document management systems, you know, so connecting into those is like very important. It may include something as subtle as like how well you OCR and like what OCR programs you use and how you set those up when you're doing that task of one of the tasks that the co-counsel does, for example, is reviewing large sets of documents. Once you start working a lot of documents, you see like stuff is handwriting all over it and they're like tilted in the scan. And there's this crazy thing that they do in law where they print four pages on one page to save like room and all of the CRS can read it directly across, but actually goes, you know, one, two, three, four.
在我们的案例和法律事务中,他们有一些非常具体的法律专用文档管理系统,因此连接到这些系统是非常重要的。这可能包括一些细微的方面,比如你如何进行OCR(光学字符识别),以及你使用什么OCR程序以及设置这些程序的方式。例如,联合律师的任务之一是审阅大量文件。当你开始处理大量文件时,你会看到一些文件上手写了一些内容,而且扫描时可能有倾斜。在法律界,他们还有一种奇怪的做法,就是为了节省空间,把四页打印在一页上,而所有的OCR软件会直接横向读取,但实际上正确的顺序应该是一、二、三、四。
So by the time you've dealt with like all the edge cases, frankly, not even before you hit the large language model, like everything else up to the large language model, there might be dozens of things you've built into your application to actually make it work and work well. And then you get to the prompting piece and writing out tests and very specific prompts and the strategy for how you break down, you know, a big problem into step by step, kind of thinking and how you feed in the information, how you format the information in the right way. All of that also becomes like, you know, your IP and it's very hard to replicate, very hard to build and therefore very hard to replicate.
所以,当你处理所有边缘案例时,即使是在你使用大型语言模型之前,实际上就已经在应用程序中构建了许多东西,以使其正常工作并且工作良好。接下来,你需要处理提示部分,编写测试和非常具体的提示,以及如何将一个大问题分解为一步一步解决的策略,以及如何输入和正确格式化信息。这一切都变成了你的知识产权,很难被复制,并且很难构建,因此也很难被再现。
It's all the business logic, which is all even all the very successful SAS companies with very specific domain. You need very, very custom esoteric niche integrations like plug into this esoteric law database. Yeah, absolutely. Two things that I think about all the time, it's like basically all SAS for a while was just like a SQL wrapper, right? Like if you think about like various companies like Salesforce, they've built that business logic around basically just databases and connections between like tables in a database and sometimes bridging that gap between something that like either a very technical person can do, but most people can't and making accessible or bridging that gap between them that almost works like you can do a lot of cool demos in chat GPT without building a line of code, but that almost works and works, you know, 70% of the time, but going to 100% of the time is a very different kind of task and people pay $20 a month for the 70% and maybe $500 or $1000 a month is in that actually works depending on the use case, right? So there's a lot of value gained going that last mile or 100 miles, whatever it is.
这全部都是业务逻辑,甚至是那些在特定领域非常成功的SaaS公司也是这样。你需要非常定制化的、专业领域的小众集成,比如接入一些特殊的法律数据库。是的,绝对的。有两件我常常想到的事情:一段时间以来,几乎所有的SaaS都像是一个SQL的包装器,对吧?比如说,像Salesforce这样的公司,他们围绕数据库和数据库中的表之间的连接构建业务逻辑,有时候这种工作是非常技术化的,普通人难以做到,而他们的任务就是让这些技术可访问或填补技术和普通人之间的鸿沟。你可以在ChatGPT中无需编写代码就做很多很酷的演示,但要做到从大概70%的正确率到100%的正确率是非常不同的任务。用户可能愿意为70%的正确率每月支付20美元,而愿意为某些情况下真正能工作且一直工作的版本支付500或1000美元。所以,无论这条路有多长,最后的那些努力都能为你带来很大的价值。
Yeah. Can you talk about how you went from 70% to 100% because I think the other knock on this technology that we hear a lot is like, Oh, these albums hallucinate too much. They're not accurate enough for real world use. But as you said earlier, like the use case that you're working on is a mission critical use case. There's like a lot at stake if the agent gives bad information to lawyers who are working on important court cases. How did you make it accurate enough for lawyers who are conservative by nature to trust it? This test driven development framework first one goes a long way because you can start seeing patterns and why it's making a mistake and then you add instructions against that pattern and then sometimes it still doesn't do the right thing and then you kind of really ask yourself, OK, well, was that being super clear in my instructions? Am I including information? Doesn't it doesn't it shouldn't see you or too much or too little information for it to really get the full context?
好的。你能谈谈你是如何将准确率从70%提高到100%的吗?因为我们经常听到关于这项技术的批评,比如说这些系统有太多错误,不够准确,无法在现实世界中应用。但就如你之前提到的,你所从事的应用场景是一个关键任务场景。如果系统给参与重要法律案件的律师提供错误信息,后果会很严重。你是如何让它足够准确,让本质上保守的律师也能信任它的?基于测试驱动的开发框架是走出的第一步,因为你可以开始发现出错的模式,然后针对这些模式添加说明。不过有时它仍然不能正确执行任务,这时你需要真正问自己,我的说明是否足够清晰?我是否包含了过多或过少信息,使得系统无法真正理解完整的背景?
And usually like these things are pretty intelligent. And so usually you can kind of root cause while you're failing certain tests. And then build to a place where you're actually passing those tests and just getting it right, you know, and one of the things we learned is after passes, frankly, like a hundred tests, the odd that it will do on any random distribution of like user inputs that is 100,000, 100% accurately is like very high.
通常情况下,这些东西都相当智能。所以你通常可以找到未通过某些测试的根本原因。然后逐步改进,直到你真正通过那些测试并正确完成。我们学到的其中一个经验是:在通过大约一百次测试之后,它在任意随机分布的用户输入(比如10万次)中能达到100%准确率的可能性非常高。
One of the things that strikes me that is tricky, like many founders we work with are very tempted to just raw dog it. Yeah. It is like no e-vals, no test driven. We're just like vibes only prompt engineering. And maybe I mean, you switched over to this very quickly then. Like, was it just obvious from the beginning? You're like, we just can't do it that other way. We should not raw dog any of these prompts.
这段话的意思是:让我感到棘手的一点是,我们合作的许多创业者很容易被只依靠直觉的方法吸引。他们倾向于不进行评估,也不做测试驱动,只依靠感觉来进行提示设计。或许你很快就转变了方法,从一开始就认为不能那样做。我们的提示设计不应该仅仅依靠直觉。
Yeah, I think I think the biggest thing first of all depends on the use case. For a lot of things that we were working on, for better or for worse, there was a right answer. And if you get the wrong answer, lawyers are not going to be happy about it. You know, I had been a lawyer myself, but also been signed lawyers for a decade. Every time we made the smallest mistake and anything we did, we heard about it immediately, right? And so I had that voice in my head, maybe, but I was going through this process. And that that was the learning from the 10 years of slogging through pre LMS, you're like, no, it has to be a hundred percent.
是的,我认为,首先,这取决于具体的使用场景。在我们处理的许多事情中,不论结果好坏,往往都有一个正确答案。如果你给出了错误答案,律师们是不高兴的。我曾经也是一名律师,而且在过去十年里一直与律师们合作。每次我们犯哪怕是最小的错误,都会马上听到反馈。所以,或许在我心里有个声音一直在提醒我。当我经历这个过程时,我就会想起这十年埋头工作的经验教训,就是事情必须百分之百正确。
Oh, yeah. Oh, yeah. That's probably true of way more domains than we realize, actually. It could be because I know the thing that we're thinking about a lot is you can lose faith in these things really quickly, right? You have one bad experience, especially if it's your first bad, your first experience is bad. And you're like, you know, maybe we'll check on this AI stuff a year from now, especially if you're like a busy lawyer, not a technologist.
哦,是的。哦,是的。实际上,这可能在远比我们意识到的更多领域中都成立。这可能是因为我知道我们考虑的事情很多,你可以很快对这些事物失去信任,对吧?你只要有一次不好的经历,尤其是如果你的第一次经历就很糟糕。你可能会想,或许一年后再来看看这个AI的东西,尤其是如果你是一个忙碌的律师,而不是技术专家。
So we knew you had to make that first encounter the first week really, really work for the lawyer or else they're not going to invest in it deeply. So let's talk a bit about open AI 01 because it is very different model. I mean, up to this point with GPT4 and all that previous generation, the analogy in terms of the intelligence is sort of the kind of system, one thinking in the Daniel Kahneman type of intelligence, right? Yes, this whole economic theory, you want the Nobel prize around this. Someone thinking is just very fast is kind of these decisions that humans make very intuitively and based on patterns and elements are fantastic at that. But they're terrible at the executive function because what I'm hearing with all the stuff that you're describing is kind of you're just giving the LLM executive function is like, how do you think it's right? How do I manage it? Really that slower thinking? And I think 01 is exciting. We haven't seen things built yet because it just got announced a few days ago, right?
所以我们意识到,你必须让律师在第一周的首次接触中真正投入,否则他们就不会深入参与。因此,让我们来谈谈开放AI 01,因为这是一种非常不同的模型。到目前为止,包括GPT-4及其之前的版本,其智能可类比为丹尼尔·卡尼曼所描述的“系统一”思维。是的,这一经济理论为他赢得了诺贝尔奖。“系统一”思维非常快速,类似于人类直观地基于模式和元素做出决策,但在执行功能方面表现很糟糕。根据你所描述的内容看来,无论是怎样给大型语言模型提供执行功能,它就像是在问:该怎么思考才是正确的?我该如何管理?这真的是一种更缓慢的思维过程。我认为01非常令人兴奋。由于它几天前才发布,我们还没有看到基于它的开发成果。
I think it's getting to that system to thinking. And I think this has been a big area research, which I saw a lot in a new reps a year ago where a lot of the researchers were excited to unlock this because this is the missing piece to where AGI. Let's talk about what are your thoughts on 01 and how this changes. So first of all, I think 01 is a very impressive model. Like with other things, we gave it the kinds of tests that we knew were failing and the degree of it's not just math, the degree of thurnis, precision intelligence applied to some of these questions. And sometimes it's the stuff that you wouldn't wouldn't expect you need a super smart model to do.
我觉得现在系统正在转变为一种新的思维方式。我认为这一直是一个重要的研究领域,一年前我在一个会议上看到了很多相关研究,很多研究人员都很兴奋能攻克这个问题,因为这就是通往通用人工智能(AGI)的缺失部分。让我们来谈谈你对01这个模型的看法以及它带来的变化。首先,我认为01是一个非常令人印象深刻的模型。就像其他事物一样,我们对它进行了各种我们知道会失败的测试,但它不仅仅是在数学上表现出色,还有在面对某些问题时所应用的深度精确的智能。有时候,它解决了你认为不需要超智能模型的那些问题。
Like in one of the tests that we run, we give it lawyers, real legal brief, but we edited very slightly some of that lawyers quotations to the case to make it a wrong quotation or wrong kind of summarization of his case. It's like 40 page legal brief. You alter things with just adding the word like not can change the meaning of something entirely, right? And then we give the full text of the case as well to the AI. And we say, well, what did, you know, what did the lawyer get wrong about this case of anything? And literally every LLM before that would be like, nothing. It's perfectly right. And it's just not a precise thinker about some of the very nuanced things that we altered about the brief to make it slightly wrong.
就像我们的一个测试中,我们给AI提供了一份律师写的法律简报,这是一份真实的文件,但我们对律师引用案件的部分进行了非常细微的修改,导致引用或摘要变得错误。这份法律简报大约有40页。你只需添加一个词,比如“不”,就能彻底改变意思,对吧?然后,我们也把案件的完整文本提供给AI,并询问“这个律师在这个案件中有什么地方搞错了吗?”此之前的每一个大语言模型都会回答:“没有,一切都正确。”但其实它们对我们在简报中做的那些细微错误修改并不敏感,缺乏对这些细节的精准分析能力。
And one got like immediately, like you said, like it thinks actually for a while, like it sits there for a minute or like, is it something it's thing on, you know, like, but then it starts answering and it's like, oh, well, you know, change an and to a neither nor. So those are the kinds of tests that you kind of expect even, frankly, earlier AI, like, LLMs to be able to pass, but just could not. And all of a sudden, one is even doing these things that take like, like precise detail thinking. Obviously, we don't have the internals on how a one really works. We have, you know, this broad idea of chain of thoughts.
翻译成中文:
正如你所说,其中一个立刻反应过来了,但其实它好像需要思考一会儿,像是停顿了一分钟,或者说,它在琢磨一些东西。不过接着它开始回答,像是“哦,你可以把‘and’换成‘neither nor’。”这些是你期望甚至是早期AI,比如大型语言模型 (LLMs) 都能通过的测试,但事实上却不能。突然之间,有一个AI居然可以处理这些需要非常精确思考的事情了。显然,我们并不了解一个AI内部是如何运作的,但我们对一种思维链的概念有一个大致的了解。
Seemingly, we know that if open AI had a giant corpus of internal monologue of people thinking through doing things step by step, one would be even a lot better. It sort of rhymes with the thing you did to put your first step on the moon, right? Like, yeah, it rhymes with break it down into, you know, chunks where you can get to a hundred percent accuracy instead of just throw it all in the context window and, you know, maybe magically it will work. Yeah. Do you think that that's what's happening then? There's a shot that they've had, you know, maybe change what their contractors are doing instead of just doing, you know, input in answer out. They're doing input in how would I think about solving this problem and then answer out, but then it's, you know, the interesting thing is then it's kind of limited by the intelligence of the people writing those instructions.
看起来,如果OpenAI有一个包含人们按步骤思考做事过程的巨大语料库,那么AI性能可能会更好。这和你们当初逐步实现人类首次登月的过程有些相似,对吧?也就是说,把任务分解成可以实现百分之百准确的小块,而不是仅仅把所有信息一次性放入上下文窗口,希望它能神奇地解决问题。你觉得这就是他们现在在做的事情吗?可能他们已经改变了合同工的工作方式,不再只是“输入问题,输出答案”,而是“输入问题,思考如何解决,然后输出答案”。有趣的是,这种方式会受到撰写这些思考过程的人的智力水平的限制。
And one of the things that we're investigating for it's worth with O1 is can we prompt it to tell it what to think about during its thinking process and inject, like again, like we've hired some of the best lawyers in the country. How would the most of the best lawyers in the country think about solving this problem and maybe, you know, we have no conclusive evidence from where the other yet that this dramatically improves things. It's so early and just just not have time yet has passed. There's a chance that one of the new prompting techniques with O1 is teaching it not just like how to answer the question, what can the answer look like, but how to think.
我们正在研究的一件事是,是否可以通过提示来引导O1在思考过程中去想什么。比如说,我们雇佣了一些全国最优秀的律师。大多数顶尖律师会如何考虑解决这个问题呢?目前,我们还没有确凿的证据表明这种方法能显著改善问题。因为这一研究还处于早期阶段,还没有足够的时间过去去验证。然而,有可能一种新的提示技术可以教O1不仅仅是如何回答问题或者答案的形式,而是如何思考。
And I think that that's another really interesting opportunity here is injecting domain expertise or just your own intelligence. I'm just so thankful because I think you're sort of sharing the breadcrumbs and where there are a great many other spaces where this technology is just beginning. I mean, you go to pretty much any company. People have no concept of what's just happened. Yeah, they actually literally still repeat all of those sort of tired tropes of, oh, you better be fine tuning or all of these. I mean, these things are just not connected to like what we're seeing day to day with startups and founders trying to create things for users.
我认为这里还有一个非常有趣的机会,就是注入领域专长或者你自己的智慧。我非常感激,因为我觉得你在分享一些线索,指出了许多这个技术刚刚开始的领域。我是说,几乎走进任何一家公司,人们都还没意识到刚刚发生了什么。实际上,他们仍在重复那些陈旧的观点,比如“你最好进行微调”之类的话。这些想法根本没有连接到我们每天看到的那些创业公司和创始人如何为用户创造事物的实际情况。
What I'm kind of glad for is that we get to actually share this news, like this knowledge because like even the things we talked about, you know, hey, you probably do e-vals. Like there's a lot of alpha and getting to 100 percent, not just 70 percent. These are sort of the breadcrumbs that will actually go on to create all of the billion dollar companies, maybe thousands of them actually. We hope so. I mean, I think that you're about to see a lot of other fields like law really level up when you don't have to spend, you know, millions of dollars in six months, literally in a basement reading document by document by document.
我感到欣慰的一点是我们能够实际分享这个消息和这些知识。就像我们讨论过的一些事情,例如,你可能会进行评估。实现100%的成功,而不仅仅是70%,正是这些关键线索将会帮助创立所有市值达到数十亿的公司,也许实际上有成千上万这样的公司。我们对此充满希望。我认为你将会看到很多其他领域,比如法律,将会真正提升。当你不必在地下室逐页阅读文件,并在六个月内花费数百万美元时,这些领域会有很大的进步。
Right. When you actually can just get past that and get just the results. Now you're thinking strategically and intelligently and the unlock for these companies, I mean, they currently pay again, millions of dollars in salaries for these jobs to be done, each of them. Right. So for any company to come out with a AI that can do even 80 percent of that, the value is really there. And I just want to encourage people to not kind of give up based on those tropes, right? Like, Oh, it hallucinates too much. It's too inaccurate. It's too whatever.
好的。当你真正能够超越那些障碍,只关注结果时,你就在战略性和智能性地思考。这些公司现在每年都花费数百万美元的工资来完成这些工作。想想如果有一家公司能够推出一款人工智能,即使只完成80%的工作,它的价值已经显而易见。我想鼓励大家不要因为一些常见的说法就放弃,比如“它出错太多”或者“它不够准确”等等。
There's a, for example, if anything, it's like there's a path and you can do it. And there's some good news in that, you know, what the jobs aren't going to go away. They'll just be more interesting. That's what I think. Yeah. Well, with that, we're out of time, but Jake, thank you so much for being with us. Thanks for having me. See you guys next time.
例如,有一条路,你可以做到。而且有个好消息,那就是工作不会消失,只是变得更有趣。这就是我的看法。好的,时间到了,Jake,非常感谢你和我们在一起。谢谢你邀请我。下次再见。